1. Introduction
In the field of labor, in recent decades Colombia has undergone important transformations, for example, the greater schooling rate of women has generated a sustained increase in their participation and insertion into the labor market (Castellani and Arango, 2016). Despite this, when compared with other Latin American countries or countries with similar per capita income levels elsewhere, female unemployment in Colombia continues to be one of the highest in the region. In addition, compared to men, women register lower levels of occupation and participation in the labor market, a situation that although it is not exclusive to this country, deepens the already pronounced existing inequalities (Lora, 2016).
As shown in Figure 1, the female unemployment rate had been declining, except for the year 2020 probably as the result of the economic crisis generated by the COVID-19 pandemic that negatively impacted women´s employment and working conditions According to the Departamento Administrativo Nacional de Estadística (DANE), that year 61.78% of men were employed compared to 38.29% of women. Likewise, the unemployment rates show a disparity: 12.83% of men were unemployed while the rate for women was 20.62%.
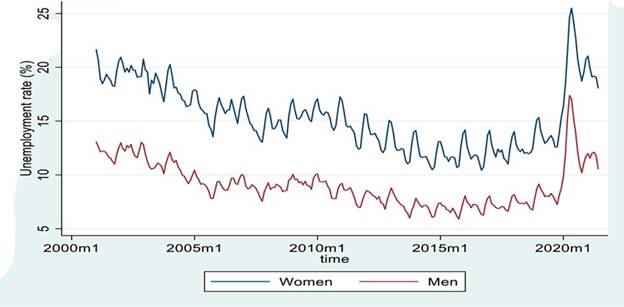
Source: Authors’ own elaboration based on the GEIH 2001-2021.
Figure 1 Unemployment rate by gender in Colombia (%) 2001:01 - 2020:06
Since statistics on the labor market in the country have been available, the gender gap in the unemployment rate -which is defined as the difference in percentage points (pp) between the female and male unemployment rate- has been at least 4 pp (Tenjo, Álvarez, and Jiménez, 2016). Figure 2 shows the evolution of this gap for the period 2001:01 - 2020:06. At the beginning of this century, the gender unemployment gap reached 8.5 pp in favor of men and decreased in the following years, generally remaining below 7.0 but above 5.9 pp, a number that continues to be alarming. Just during the period 2012-2019, the gap remained below 5.8 pp. However, the arrival of COVID-19 had a greater impact on the jobs occupied by women and increased the gap against them again by more than 8 pp, thus reversing the small but important progress reached in the last two decades.
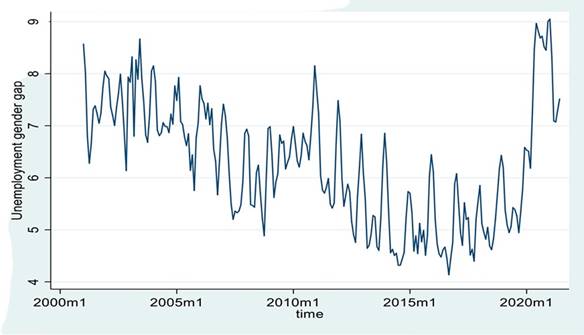
Source: Authors’ own elaboration based on the GEIH 2001-2021.
Figure 2 Unemployment gender gap in Colombia (percent points) 2001:01 - 2020:06
Women’s entry into the labor market generates positive effects on their lives by allowing them not only to change their productive and reproductive roles but to become economically empowered, reducing the gender gap in unemployment rates which, in turn, could significantly increase the Gross Domestic Product (GDP) of a country (Meza, 2018). For this reason, understanding the behavior and determinants of the unemployment gender gap is fundamental to the achievement of equal opportunities and the full and equitable development of an economy.
The purpose of this research is to analyze the evolution of the unemployment rate gender gap in Colombia during the period 2001:01 - 2021:06, seeking to adjust a model to forecast its behavior and identify its volatility. A gradual narrowing of the gap is expected, although at a slower speed than desired, in a scenario of growing volatility. We start by presenting the results of similar research carried out at the Latin American and global levels in the field of study. Next, to model the evolution of the gender gap, the methodology of Autoregressive Integrated Moving Average models with seasonal component (SARIMA) and Generalized Autoregressive Conditional Heteroscedasticity models (GARCH) are exposed. The results obtained for these models are then presented and explained, followed by the discussion and the conclusions.
2. Literature review
Unemployment is conceived as a social and economic problem that is detrimental to the well-being of households. This disruptive event in the labor career has disproportionately influenced vulnerable groups, such as women, the elderly, and ethnic minorities, among others. Specifically, the gender gap in both the employment and unemployment rates has aroused great interest in academia, institutions, and governments that seek to achieve equal opportunities between men and women. While it is known that changes in the structure of employment, globalization, and technological advances have managed to mitigate these gaps, the patterns of these indicators still show significant differences, and gender inequality in the labor market is still observed in many countries. Thus, the exploration of the evolution of these variables and their forecasting is essential for decision-making and the formulation of public policies that recognize this social and economic problem and are interested in reducing it.
Albanesi and Sahin (2018) study gender differences in unemployment in the U.S. from a long-term perspective. Their main hypothesis is that women´s opportunity cost is higher and more dispersed than men´s and that women are more likely to have a change in that opportunity cost due to impacts such as poor health or disability of themselves or family members, pregnancy or childbirth, changes in household income, among others. When analyzing the unemployment gender gap defined as the difference between the female and male unemployment rate for the period 1948-2014, the authors observe that such a gap, which had been positive, practically disappeared in 1980, remaining stable during the last 60 years; however, during recessions, there is an increase in male over female unemployment. Using a calibrated search model of three variables (employment, unemployment, and non-participation) for the years 1978 and 1996, they examined whether the composition of the labor force and the industry can explain the evolution of this gap. The results reflect that the narrowing of the unemployment gender gap is largely explained by the convergence in male and female attachment to the labor force and that the increase in male unemployment relative to female unemployment in recessions is due to differences in gender distribution in the industrial sector during the last years.
Similarly, Gharehgozli and Atal (2020) analyze the gender wage inequality at the different deciles in the U.S. for the period 1986 - 2016. To have an understandable and comparable measure of analysis, they used the Big Mac index as a unit of measurement. Their results reveal that the overall ratio of women’s to men’s wages in 1986 was 53%, which increased to 67% in 2016, indicative of a very slow decline in the wage gap over time. They also find that a larger employment gap is associated with a smaller wage gap, especially at the lowest wages, and that gender wage inequality increases with the deciles of the wage distribution. Finally, a negative correlation is observed between the unemployment rate and the employment gender gap, which infers that the male employment rate is more affected by economic crises.
Kovalenko and Töpfer (2021) analyze the effects of business cycles on unemployment rates and labor force participation of men and women, the gender wage gap, and GDP and the consumer price index in the short, medium, and long term, for the U.S. during the period 1979-2019. For this, they resort to a structural vector autoregressive model (SVAR), with seasonally adjusted variables and restrictions to identify macroeconomic shocks (demand, technology, wage bargaining, labor supply, and specific shocks to labor supply by gender). Their results indicate that labor demand and supply shocks only affect the wage gap in the short and mid-term, although not in a statistically significant way. On the other hand, a wage bargaining shock has positive effects on the wage gap only in the short term, however, this effect is only relevant in the period 1979-1999, suggesting that after the year 2000 this variable has had limited significant effects on income equality. Another notable feature is that a technology shock not only triggers economic growth but also helps reduce the wage gap. Finally, like Albanesi and Sahin (2018) and Gharehgozli and Atal (2020), the study points out that male unemployment responds more to economic cycles than female unemployment.
Millán, Santos, and Pérez (2015), analyze the female labor market in Spain based on information for the period 2002:2 - 2014:4. Using ARIMA models, they predicted the number of employed and unemployed women in the last three quarters of 2014 and through a logit model, the probability of getting a job. The results show an optimistic outlook for women with a decreasing trend in the unemployment rate due to an increment of the female labor force by about 81,249 women for the fourth quarter of 2014; however, the authors state that these numbers are not enough. The results of the logit model show that women who are most likely to obtain a job in Spain are the youngest, with better educational levels, without children, and with part-time jobs in the tertiary sector.
For his part, Khan (2020) evaluates gender differences in college graduates and unemployment rates in Saudi Arabia using an ARIMA model with annual data from 1996 to 2018. The author concludes that although there is an increasing number of women graduating from universities, their unemployment rate is significantly higher compared to men. Thus, according to the ARIMA forecast, the female unemployment rate in 2015 should be about 33%, much higher than the male one, which is an obstacle for the country that aspires to become one of the most technologically advanced economies in the world.
A higher probability of falling into unemployment given the economic cycle or other variables does not necessarily imply a lower probability of returning to work and recovering wages. Mooi-Reci and Ganzeboom (2015) propose an interesting perspective in which they examine wage declines after unemployment, known as “unemployment scars”, and how this phenomenon operates by gender, social groups, and economic conditions. The study was conducted in the Netherlands for the period 1985-2000 and used a fixed-effects panel data model, with the logarithm of hourly re-employment wages as the dependent variable, and unemployment indicators, human capital, demographic, and job characteristics, as well as macroeconomic measures as covariables. Among the main findings is that unemployment causes significant salary losses for both men and women, with the scars being higher in the latter after the first unemployment and less pronounced in the following ones, while in men these effects vary throughout the unemployment periods. This could be because, after the first interruption, women move to sectors more favorable to motherhood. Finally, discriminatory practices in re-employment are more likely to develop for older workers, in precarious economic conditions, or migrants.
In the same line of thought, Manzoni and Mooi-Reci (2020) study how labor market inequalities accumulate over time after an initial period of unemployment in Germany for the period 1984-2005. The data used comes from the German Socioeconomic Panel, and the methodology used consists of a hybrid model that combines the strengths of the fixed and random effects models, with employment status as the dependent variable and unemployment, sociodemographic and macroeconomic indicate that the negative effects generated by unemployment, on average, alleviate throughout the career and disappear for men, but not for women for which, the quality of the career increases at the beginning, but eventually slows down until it finally stops or decreases. This infers that the scars in the German labor market are more pronounced in women.
According to the literature, business cycles affect the employment and unemployment rates of men and women differently, especially in times of crisis. The Covid-19 pandemic has had multidimensional impacts on the society and the economy, and unemployment has skyrocketed due to the restrictive measures imposed and the closures of companies and staff cuts, especially affecting women who, in addition, had to assume a higher burden of domestic work and care. Recently, Dancausa, Millán, Hernández, and Jimber, (2021) used an ARIMA model to analyze the Spanish labor market from a gender perspective during the 2009 economic crisis and the health crisis of Covid-19. Specifically, they analyze the gender wage inequality of women´s glass ceiling. Starting from the Labor Force Survey, which contains monthly information on the number of women working and considering a data set for the period 2008:1 to 2020:4, the authors found a slightly increasing trend in the female labor demand. However, they also found evidence of the persistence of inequalities in employment and unemployment rates, wages, and job authority opportunities. In this sense, assuming that the pandemic is controlled and that there is no border closure, they predict that by 2021 there will be a growth in the labor market for women.
As for the case of Colombia, Méndez (2021) carried out a time series analysis of the main indicators of the female labor market in the period 2007-2019, forecasting their behavior and evaluating their relationship. Using DANE’s Great Integrated Household Survey, the author applies the Box-Jenkins methodology to monthly data on target underemployment rate by skills, global participation rate, and unemployment rate. The results indicate that the underemployment rate and the global participation rate would tend to increase during the first quarter of 2020 but would eventually decrease; the unemployment rate would move oppositely, decreasing at the beginning and then increasing, but moderately. However, variables did not behave as forecasted given the contingency situation resulting from the Covid-19 crisis, in which the female population was affected the most.
According to Gharehgozli and Atal (2020) and Khan (2020), men and women do not get the same by acquiring greater human capital, women and men do not obtain the same probability of working in a good job with a good salary as they accumulate human capital. That is, as progress is made in the occupational hierarchy and wage deciles, the gender gap against women becomes more pronounced. This discriminatory dynamic is foreseen from higher education institutions where horizontal segregation is observed in the selection of and continuity in the career, especially in those with greater demand in the labor market, with better salaries and better working conditions. To analyze the gender gap in academic programs of Science, Technology, Engineering and Mathematics (STEM), Giral (2021) adjusts an ARIMA model for the number of graduates of higher education institutions in Colombia in the STEM area during the period 2001-2018, based on semi-annual data from the National Higher Education Information System (SNIES). Their results indicate that, although the percentage of women who access and graduate from higher education programs has increased in recent decades, their participation continues to be segregated in certain careers. The forecasts obtained in the study are positive and allow a glimpse of a reduction in the gap, which indicates a more favorable outlook for women in terms of employment.
Although the incorporation of women into the labor market has become one of the most important phenomena of the last century, gender gaps in this area continue to exist. Therefore, and given that institutions and governments must formulate and implement policy actions aimed at reducing and subsequently eliminating these inequalities, it is important to have rigorous and timely research carried out from a gender perspective to identify the progress reached and the possible panorama of the coming years. Gender equality in the labor market, as well as in the social, economic, and political fields, contributes to the improvement of the quality of life and is a fundamental link for the achievement of development and progress. As long as equal conditions for men and women are not achieved, there will be an inefficient use of the labor resource.
3. Methodology
This study uses information on the monthly unemployment rate for men and women estimated by the DANE based on the Great Integrated Sample Survey (GIHS) of the period 2001:01 - 2021:06. The unemployment gender gap is calculated as the difference between the female and male unemployment rates. To model the evolution of this gap, we resort to an AutoRegressive Integrated Moving Average model with a seasonal component (SARIMA) applied to the series in logarithms, while its volatility is analyzed based on a Generalized AutoRegressive Conditional Heteroskedasticity model (GARCH).
The modeling procedure follows the steps indicated by Box and Jenkins (1970) but includes the volatility component (Engle (1982) and Bollerslev (1986)). Thus, we start by identifying a tentative SARIMA model for the mean based on the graphical analysis of the series, its correlograms, and the results of a unit root test; next, we proceed to adjust the proposed model and evaluate its results, seeking to verify that the assumptions are met. If necessary, the originally proposed model is modified until satisfactory results are obtained. Finally, following a similar procedure but based on the squared residuals of the model finally adjusted, we proceed to identify and estimate a GARCH model for the analysis of the series.
3.1. SARIMA model
The Box-Jenkins (BJ) methodology, technically known as ARIMA(p,d,q), was initially proposed by George Box and Gwilym Jenkins in 1970 to forecast time series based on their probabilistic properties. In these models, the variable is explained by p lags of its historical values (autoregressive processes, AR) and q lags of its previous stochastic error terms (moving average, MA) applied to stationary series, that is, their mean and variance are constant, and their covariance does not vary over time. However, most series are not stationary and must be differentiated, in other words, are integrated of order d [I(d)] (Gujarati and Porter, 2010). Thus, an ARIMA(p,d,q) is an ARMA(p,q) model applied to a series that needs to be differentiated to become stationary.
On the other hand, many series show a seasonal behavior, which usually occurs with series with a periodicity of less than a year; this seasonal component must be considered to properly model the series. Thus, it is necessary to identify not only the lags in the regular part but also in the seasonal part, i.e. a SARIMA(p,d,q)(P,D,Q) model, which in general terms is given by:
where Yt is the variable under study, et is a white noise error term, d and s correspond to the order of differentiation in the regular and seasonal component, respectively, while m, the φs, and the qs are the parameters to be estimated.
3.2. GARCH model
Generalized AutoRegressive Conditional Heteroskedasticity models (GARCH) developed by Bollerslev (1986) are an extension of the AutoRegressive Conditional Heteroskedasticity models (ARCH) developed by Engle (1982). In this model, Bollerslev proposes a conditioned variance that depends not only on the lagged values of the squared errors, as in the ARCH(q) model, but also on p lagged values of the variance itself. The usefulness of the GARCH(p,q) models in univariate time series analysis is that, by having a more flexible lag structure, it allows predicting the volatility in the short and mid-term and ensures that the properties and behavior of the estimators do not change over time. The equation for the conditional variance (σt 2) is given by:
In this study, we resort to a GARCH(p,q) model to capture the volatility of the unemployment gender gap in Colombia for the period under study.
4. Results
In the period of study, the average unemployment gender gap was 6.26 pp against women, with a minimum of 4.13 pp in September 2016, a maximum of 9.04 pp in January 2021, and a standard deviation of 1.17 pp. In addition, the coefficient of asymmetry is positive, implying that the distribution of the data is skewed to the left, that is, the series presents higher frequencies of values below the mean (Table 1).
Table 1 Descriptive statistics of the unemployment gender gap
Gap | |
---|---|
Observations | 246 |
Mean | 6,26 |
Standard deviation | 1,17 |
Minimum | 4,13 |
Maximum | 9,04 |
Assimetry | 0,22 |
Kurtosis | 2,21 |
Source: Authors’ own elaboration based on the GEIH 2001-2021.
4.1. Results of the SARIMA model
To estimate the SARIMA model, the logarithm of the gender gap (lgap) is used to lower the variance of the series and facilitate the analysis. The simple and partial correlograms of the series presented in Figure 3 show a monthly seasonal component; in the former, an exponential fall in the regular component is observed, indicative of stationarity, while the linear fall in the seasonal component indicates non-stationarity. According to the Augmented Dickey-Fuller unit root test with no trend, the series is not stationary, with a p-value of 0.1265.
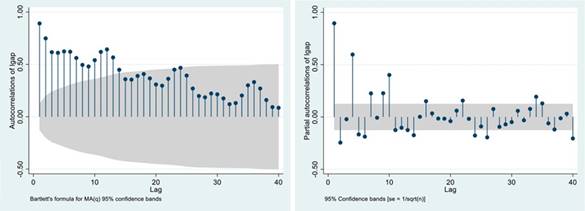
Source: Authors’ own elaboration based on the GEIH 2001-2021.
Figure 3 Simple and partial correlograms of the unemployment gender gap (logarithm)
According to the correlograms for the series in differences, after one difference in the regular component, the seasonal pattern becomes even more evident, while one difference in the seasonal component makes the series stationary, with an exponential fall in the simple correlogram concluding that the series needs one seasonal difference of order 12 only; also, the correlograms indicate the presence of an autoregressive process in the regular part and a moving average process in both the regular and the seasonal part (Figure 4).
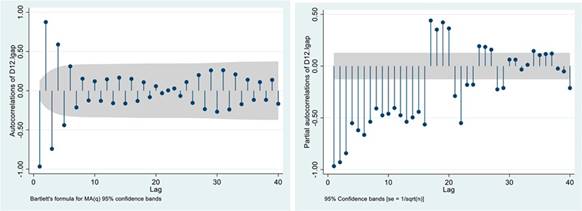
Source: Authors’ own elaboration based on the GEIH 2001-2021.
Figure 4 Simple and partial correlograms of the unemployment gender gap (logarithm): seasonal difference
Based on these results, a SARIMA (2,0,2)(0,1,1,12) with no constant is proposed. The results of the estimated model are shown in Table 2.
Table 2 Results of the SARIMA (2,0,2) (0,1,1,12) model
S12.lGap | Coefficients | Standard deviation | Z | p-value |
---|---|---|---|---|
ARMA | ||||
AR | ||||
L1 | 0,2177 | 0,0573 | 3,79 | 0,0000 |
L2 | 0,5600 | 0,0643 | 8,70 | 0,0000 |
MA | ||||
L1 | 0,9969 | 0,0387 | 25,76 | 0,0000 |
L2 | 0,8012 | 0,0495 | 16,18 | 0,0000 |
ARMA12 | ||||
MA | ||||
L1 | -0,8147 | 0,0467 | -17,43 | 0,0000 |
Sigma | 0,0517 | 0,0021 | 24,30 | 0,0000 |
Source: Authors’ own elaboration based on the GEIH 2001-2021.
According to Bartlett´s test, with a p-value of 0.0885, it can be concluded that the residuals of the model are white noise. Likewise, the inverse root test of a polynomial shows that all roots are within the unit circle, proving that the process is stationary (Figure 5).
Finally, regarding the forecast of the model, a good fit is observed, with the estimated series (represented by the red line), behaving similarly to the observed variable (blue line). Figure 6 illustrates the original unemployment gender gap in Colombia and the fitted values since March 2015, including a forecast until December 2021 to better appreciate the results obtained.
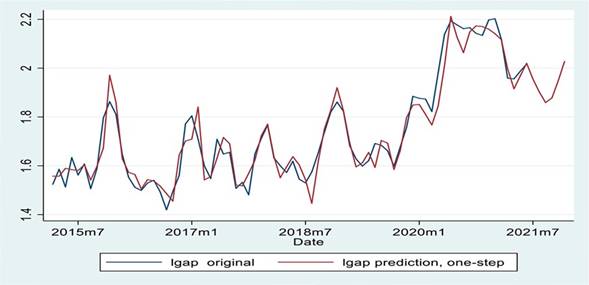
Source: Authors’ own elaboration based on the GEIH 2001-2021.
Figure 6 Forecasting from the estimated SARIMA (2,0,2) (0,1,1,12)
According to the forecast for the next six months (from July to December 2021), it is estimated that the unemployment gender gap will tend to decrease; however, by December it is expected to increase again given the seasonal dynamic shown by the series.
4.2. Results of the GARCH model
To identify the existence of a GARCH component and estimate it to account for the volatility of the process, the variance of the residuals of the estimated SARIMA model is analyzed. As shown in Figure 7, in the first years of the series greater volatility of the unemployment gender gap is observed and the histogram indicates a leptokurtic distribution of these squared residuals, which implies a high degree of concentration around the central values. It is also observed that the data has a positive asymmetry with a long tail to the right, which allows us to infer that the variance is not constant and, therefore there are heteroskedasticity problems.
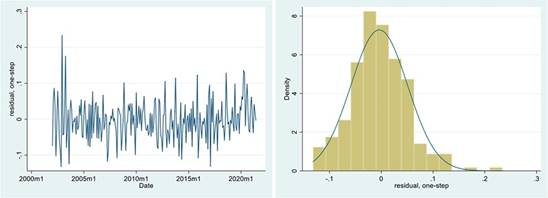
Source: Authors’ own elaboration based on the GEIH 2001-2021.
Figure 7 Residuals of the estimated SARIMA (2,0,2) (0,1,1,12) model
The AutoRegressive Conditional Heteroskedasticity (ARCH) test, whose null hypothesis states that there is no ARCH effect, is rejected with a p-value lower than 0.05 and considering lags 1 and 4-10 of the squared residuals, which corroborates the presence of an ARCH component. In this sense, to estimate the volatility of the series, we propose a GARCH(1,1) model with no constant for the squared residuals of the SARIMA(2,0,2)(0,1,1,12) model.
As shown in Table 3, the coefficients of the ARCH and GARCH terms are positive and statistically significant, and their sum less is than one, indicating that the previous behavior of the gap may influence the current volatility.
Table 3 Results of the GARCH (1,1) model
Res | Coefficients | Standard deviation | Z | p-value |
---|---|---|---|---|
ARCH | ||||
L1 | 0,1403 | 0,0546 | 2,57 | 0,010 |
GARCH | ||||
L1 | 0,6007 | 0,1512 | 3,97 | 0,000 |
Cons | 0,0000 | 0,0003 | 2,08 | 0,037 |
Source: Authors’ own elaboration based on the GEIH 2001-2021.
Once the model for the variance is fitted, it can be observed higher volatility during the first years of the period of study which decreases and remain stable after that, to finally going back to higher fluctuations after the year 2015 (Figure 8).
5. Discussion
According to the literature review and the results obtained from the SARIMA-GARCH model, it is possible to highlight some important conclusions. On one hand, the unemployment gender gap in Colombia has remained positive against women throughout the period of study with a very slight tendency to decrease. Even though the effects of the Covid-19 pandemic dramatically increased this gap, when detailing the behavior of the series before this crisis, a slow but stable decline is observed going from 8.6 pp in January 2001 to 6.5 pp in January 2020, which represents a fall of only 2.1 pp in nineteen years. On the other hand, unlike Albanesi and Sahin (2018), Gharehgozli and Atal (2020), and Kovalenko and Töpfer (2021), who found that the male unemployment rate is more affected by economic crises than the female unemployment rate, in the case of Colombia the female unemployment rate responded to a greater extent to the economic crisis than the male one, which translates into a greater gender gap during recessions. This behavior is consistent with that found by Dancausa et al. (2021) and Méndez (2021) when analyzing the period of the Covid-19 pandemic. After the lockdown restrictions were relaxed, the gap remained higher than before the pandemic.
When analyzing the female and male unemployment rates separately, it is observed a similar decline in both series; however, this behavior keeps women in a disadvantageous position since the former rate falls at a relatively lower speed and the rise of the unemployment rate of this group due to the health crisis was higher that of men. This infers that the scars of unemployment are relatively more pronounced in the case of women, presenting greater difficulties in re-entering the labor market, which coincides with the results of Manzoni and Mooi-Reci (2020).
Another particular feature of the unemployment gender gap is that it presents a seasonal dynamic, whit higher values in the first and last months of the year and smaller values in the other months, with a slight peak in June. This behavior is influenced to a greater extent by the female unemployment rate which responds the most to seasonal patterns. That is, along the year, the female unemployment rate presents greater volatility that the male´s, the latter being more stable. Finally, the forecast of the series reflects that in the following six months after the end of the period studied, the gap will show a tendency to decrease, to eventually increase again given this seasonal component.
It is important to highlight the increase in variance that the series has shown in the last years, making it more susceptible to economic cycles and seasonal patterns and, therefore, achieving an increasingly unstable narrowing of the gap. For this reason, central governments along with the private sector must commit themselves through affirmative actions that strongly achieve an accelerated and sustained closing of the gap, especially at a time when the economic crisis induced by the pandemic generated a setback in the limited progress that had been reached in the last decades.
6. Conclusions
Unemployment is widely seen as a social and economic problem that has a negative impact on society; historically, women have been most impacted by this situation, affecting not only their economic autonomy but also the economy of countries in general. Gender gaps in the labor market are a structural problem that requires the attention of governments so understanding the behavior of the unemployment rate is essential for the achievement of equal opportunities and the full and equitable development of any country. In this research, we use the unemployment rates of men and women estimated by the DANE based on the Great Integrated Household Survey to analyze the evolution of the unemployment gender gap in Colombia in the period 2001:01 - 2021:06 and fit a SARIMA-GARCH model to estimate its average behavior and determine its volatility.
The gender gap was calculated as the difference between the female and the male unemployment rate for the period considered, and the behavior of this series was initially analyzed through descriptive statistics and the estimation of a SARIMA (2,0,2) (0,1,1,12) model which was eventually used to estimate the gender gap for the following six months (2021:07-2021:12). Likewise, through a GARCH(1,1) model, the variance of the residuals of the model was examined to evaluate the volatility of the series.
As expected, the results indicate that the unemployment gender gap in Colombia showed a slight tendency to decrease before the COVID-19 pandemic; however, this progress has been almost insignificant, with a reduction of only 2.1 pp between the period 2001:01-2020:01. This small advance was nullified by the externalities of the pandemic in the labor market, resulting in an increase in the gender gap in the unemployment rate of 2.5 pp between 2020:02 and 2020:12, affecting women to a greater extent who in turn have not managed to fully recover after the restrictive measures imposed by the pandemic were relaxed, since by 2020:06 the gap had increased by 1 .5 p.p., that is, 1 pp above the level before the crisis. The model forecasts that in the following six months the series will continue to decrease by approximately 0.6 pp, to increase again by approximately 1.1 pp in December due to the seasonal component of the series.
Likewise, in the last years, the volatility of this gap has gradually increased, probably since female unemployment seems to be more vulnerable to economic and seasonal cycles, as well as to contingencies of environmental, political, or health nature, as is the case of the COVID-19 pandemic, directly harming women. This infers that, even though the unemployment gender gap had been decreasing until the year 2019, its instability has been growing especially since the year 2015, which implies that efforts to close the gap have not yielded the expected progress, with poor results.