INTRODUCTION
Rice is a vital crop, providing over 21% of global caloric needs (Fitzgerald et al., 2009). The "Green Revolution" in the 20th century significantly increased rice productivity through the breeding of new cultivars and the application of nitrogen (N) fertilizer (Pingali, 2012). However, excessive N use has negative environmental impacts, including greenhouse gas (GHG) emissions (Martínez-Dalmau et al., 2021). Improving rice nitrogen use efficiency (NUE) is crucial to reduce N fertilizer application and mitigating environmental consequences.
Strategies for enhancing NUE include agronomic approaches like site-specific nutrient management (SSNM) and alternate wetting and drying (AWD) (Mofijul et al., 2016; Chivenge et al., 2021), as well as genetic approaches targeting N-related genes (Sonoda et al., 2003; Hu et al., 2015). Advances in sequencing and genomic tools facilitated the introduction of molecular breeding techniques such as masker-assisted selection (MAS), genetically modified organisms (GMOs), and gene editing (GE) (Collard et al., 2008; Wang and Han, 2022). Currently, clustered regularly interspaced short palindromic repeats and Cas9 nuclease (CRISPR/Cas9) system has become a widely used GE technology for its simple structure and flexible applicability (Zhang et al., 2019).
Previous studies in Arabidopsis thaliana have shown that knockout (KO) mutation of AtAAP2 function decreased seed protein levels (Zhang et al., 2010), but enhanced N allocation and improved the amino acid level in leaves, leading to higher photosynthesis efficiency, carbon fixation ability, and seed yields (Perchlik and Tegeder, 2018). AtCRF4 is a transcription factor (TF) involved in N uptake and assimilation in A. thaliana, engaged in N signaling in shoots and roots (Brooks et al., 2019). Over-expression mutants of AtCRF4 showed decreased N uptake, root biomass, primary root length, and lateral root number, due to inhibition of SNZ and CDF1, two TFs that are involved in N uptake and their expression is controlled by CRF4 (Varala et al., 2018). Therefore, inhibiting AtCRF4 expression could enhance N uptake efficiency by increasing SNZ and CDF1 expressions, which may increase NRT2.1 expression - another key nitrate transporter.
This research aimed to improve rice agronomic NUE (ANUE) by knocking out rice homologs of AtAAP2 and AtCRF4 using CRISPR/Cas9 and evaluating agronomic performance in the field.
MATERIALS AND METHODS
Creation of the KO rice lines by gene editing
The AAP2-KO and CRF4-KO rice lines were created in the National Agriculture and Food Research Organization (NARO) in Japan by identifying their homologs through bioinformatic research. Their homologs in rice were identified by BLAST search on RAP-DB (https://rapdb.dna.affrc.go.jp/) and SALAD Database (https://salad.dna.affrc.go.jp/salad/) based on the amino acid sequences of the candidate genes on TAIR (https://www.arabidopsis.org/) for A. thaliana. 4 AtAAP2 homologs (LOC4324999, LOC4327987, LOC4336246, LOC4338844) and 1 AtCRF4 homolog (LOC4326729) with certain expression level were identified in rice genome. The target sequences for the gRNA of target genes were selected based on the exon regions and PAM sequence locations, and CRISPR/Cas9 binary vectors with guide RNA (gRNA) were designed for each target gene (Fig. 1).
Agrobacterium tumefasiens competent cell EHA105 was transformed with the plasmids (binary vectors) through electroporation. The transformed Agrobacterium was cultured and selected on solid LB medium with hygromycin. Surviving colonies were stored as glycerol stock. Rice callus induction was achieved on solid N6D medium, followed by infection with transformed Agrobacterium on solid AB medium and co-cultivation on solid 2N6AS medium. After infection, rice calli were sterilized, transferred to N6D medium with hygromycin and meropenem for selection. From those survived calli, DNA samples were extracted to detect candidate calli for regeneration. The selected calli were transferred onto ReIII regeneration medium with meropenem. Regenerated plants were transplanted onto a hormone-free medium, and their DNA was analyzed via PCR and capillary electrophoresis for KO confirmation. Both AAP2-KO and CRF4-KO lines were successfully obtained, and T1 and T2 seeds were sent to the International Center for Tropical Agriculture (CIAT) in Palmira, Valle del Cauca, Colombia for evaluation of agronomic parameters and ANUE. The experimental lines were put in genotyping before the seed shipment (Suppl. Tab. 1).
Design of confined paddy field experiments
A paddy field experiment was conducted at CIAT, following the field design and management from (Selvaraj et al., 2017). To induce soil N depletion, three cycles of maize cultivation without fertilizer application were performed in previous seasons and soil samples were taken from each pool for analysis before transplanting. The experimental field had a split-plot design with three replicates. 14 lines including control were selected for the field experiment based on the mutagenesis location and types (Tab. 1). Seeds were sown on June 24, 2022, and transplanted on July 15 (21 days after sowing, DAS) with 20 cm gaps between plants and 25 cm gaps between rows. Statistical analysis was carried out on seven randomly selected plants per plot after the harvest on October 18 (118 DAS).
Table 1. Rice lines for the field experiment.
No. | Line name | Description |
---|---|---|
1 | AAP2-25-1-4 | AAP2-KO |
2 | AAP2-30-8-1 | AAP2-KO |
3 | AAP2-30-8-4 | AAP2-KO |
4 | AAP2-30-8-10 | AAP2-KO |
5 | CRF4-14-2 | CRF4-KO |
6 | CRF4-22-2 | CRF4-KO |
7 | CRF4-25-1 | CRF4-KO |
8 | AAP2-25-1-3(2)-Pl-2 | AAP2-KO segregant |
9 | AAP2-30-8-1-Pl-1 | AAP2-KO segregant |
10 | AAP2-30-8-4-Pl-1 | AAP2-KO segregant |
11 | CRF4-14-2-Pl-1 | CRF4-KO segregant |
12 | CRF4-22-2-Pl-1 | CRF4-KO segregant |
13 | CRF4-25-1-Pl-1 | CRF4-KO segregant |
14 | WT Nipponbare | Control |
Nitrogen fertilizer was applied in the following treatments: (i) N 0% pool (N0%) received 0 kg ha-1, (ii) N 50% pool (N50%) received 90 kg ha-1, and (iii) N 100% pool (N100%) received 180 kg ha-1. The rate of 180 kg ha-1 for N100% was based on the farmers' practice in Colombia (Berrío et al., 2002). Actual N fertilizer weights were adjusted accordingly. In N100%, urea was applied in three splits, while N50% received half the amount following the same application regime as N100%. Other nutrients were applied as basal fertilizer at the standard rate in Colombia.
For statistical analysis and ANUE calculation, seven randomly selected plants from each plot were harvested. Eight agronomic parameters were examined: plant height (PH), stem number (SN), panicle number (PN), panicle length (PL), filled grain weight (FG), empty grain weight (EG), dry biomass weight (DB), and 1,000-grain weight (1000GW).
ANUE calculation
ANUE is an NUE index calculated using yields (kg ha-1) from the N-applied and non-fertilized control fields. It assesses the yield contribution of the N application by comparing it to the control. ANUE range is typically 10-30 kg kg-1, with a value of 25 or higher indicating efficient management or low soil N supply (Congreves et al., 2021). The ANUE formula (Eq. 1) is derived from (Craswell and Godwin, 1984)
where, F = fertilized crop, C = unfertilized control.
NDVI and NDRE analysis
Plant monitoring by vegetation indices (VIs) was carried out during the paddy field experiment based on the protocols by Selvaraj et al. (2020). Normalized difference vegetation index (NDVI) and normalized difference red-edge (NDRE) were the VIs used to measure plant health and density. NDVI uses the near-infrared (NIR) and red (RED) spectral bands to measure greenness, while NDRE employs the red edge (REDGE) spectral band provided by MicaSense (Boiarskii and Hasegawa, 2019). A drone DJI MATRICE 600 and a multispectral camera MicaSense Altum (MicaSense, Seattle, Washington, USA) were used to take aerial pictures. Ground control points (GCPs) were installed for georeferencing, and QGIS (https://www.qgis.org) was used to designate the regions of interest (ROIs) in the aerial pictures. The vegetation indices were extracted on CIAT Pheno-i (http://pheno-i.ciat.cgiar.org). Statistical analysis on NDVI and NDRE was conducted at 60 DAS (heading), 70 DAS (flowering), 81 DAS (milky-grain), and 88 DAS (grain-filling).
The formulas for NDVI (Eq. 2) and NDRE (Eq. 3) are as follows (Tucker, 1979; Barnes et al., 2000).
Statistical analysis
The statistical analyses were carried out on Statistical Analysis System SAS 9.4 (SAS Institute Inc., Cary, NC, USA). The mixed model was employed for the analysis of the field experiments, assuming N treatment, genotype, and interactions of N treatment and genotype as fixed effects, and the blocks as random effects. Duncan’s multiple range test was used to detect significant effects in the variation on the agronomic parameters and ANUE between all experimental lines. Meanwhile, Dunnett's test was used for NDVI and NDRE analysis to compare the extracted VI values between WT and KO lines at P = 0.05 in both tests.
Inserted marker hpt cassette analysis
Leaf samples were collected during the field experiment at CIAT for genomic DNA extraction to detect the remaining CRISPR/Cas9 cassette by PCR for hpt gene, the selection marker used in this study. Seven plants were randomly selected from each line in block 1 of N100% on September 1st, 2022 and their flag leaves were collected. Genomic DNA from the samples was extracted based on the protocol by Risterucci et al. (2000) and modified by M. Lorieux in 2002. The dissolved genomic DNA of each sample was stored at -20°C until genetic analysis by PCR. To detect hpt gene, primers HPT-F3 (5´-AGT-TCG-ACA-GCG-TCT-CCG-ACC-TGA-3´) and HPT-R1 (5´-TGC-CGT-CAA-CCA-AGC-TCT-GAT-AGA-GT-3´) were used, which amplify a 792 bp fragment. The PCR mix contained 2X GoTaq Master MIX (Promega, Madison, WI, USA), 0.5 μL of genomic DNA, and 0.25 μM each HPT-F3 / HPT-R1 primer. The conditions of the thermocycler were: 98°C for 3 min, 35 cycles of 98 °C for 15 s and 68°C for 1 min were repeated. PCR products were checked on 1.2% agarose gel with SYBR Safe DNA Stain (Thermo Fisher Scientific Inc., Waltham, MA, USA).
RESULTS AND DISCUSSION
Field experiment of the KO lines in a paddy field for agronomic traits and ANUE
The soil analysis before the transplanting showed the average soil pH was 8.48 and the average POXC content was 11.81 g kg-1 which is low. The average NH4 + and NO3 - contents were 7.711 and 5.944 mg kg-1, respectively.
In the field experiment, visually noticeable phenotypic differences were observed between WT and KO lines. The KO lines, whether AAP2-KO or CRF4-KO, exhibited shorter plant height, a greater stem number, and greener color during the grain-filling stage (Fig. 2).
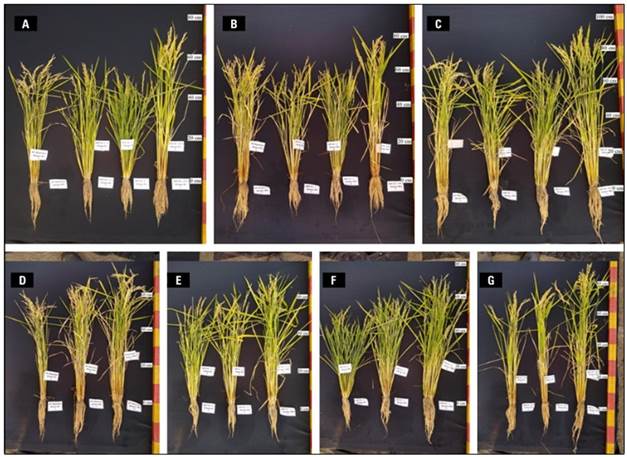
Figure 2. Rice plants from the second paddy field experiment on October 1st (99 DAS) by N treatment; from left to right: WT, AAP2-25-1-4, CRF4-22-2 (Original GE line), and CRF4-22-2-Pl-1 (segregant line) in 0% N pool (N0%) (A), 50% N pool (N50%) (B), and 100% N pool (N100%) (C). By genotypes, WT (D), AAP2-25-1-4 (E), CRF4-22-2 (F), and CRF4-22-2-Pl-1 (G), from left to right: N0%, N50%, and N100% in each picture.
Significant differences were observed between genotypes (G), N levels, and G x N interactions in FG, EG, PH, SN, PN, and DB. PL did not show any G x N interaction (Suppl. Tab. 2). KO lines generally displayed increased EG, SN, PN, and DB, along with reduced FG, 1000GW, PH, and PL (Fig. 3). The ranges of SN, PN, and DB in AAP2-KO and CRF4-KO lines were 118-228%, 183-313%, and 130-175% compared to WT, respectively (Suppl. Tab. 2). The AAP2-KO segregant and CRF4-KO segregant lines exhibited different tendencies, with generally higher 1000GW, PH, and DB, and lower SN compared to WT (Suppl. Tab. 2). Most lines responded well to N applications in terms of DB, SN, PN, and FG, however, WT and the segregant lines were more sensitive to N application compared to the original AAP2-KO and CRF4-KO lines (Suppl. Fig. 1).
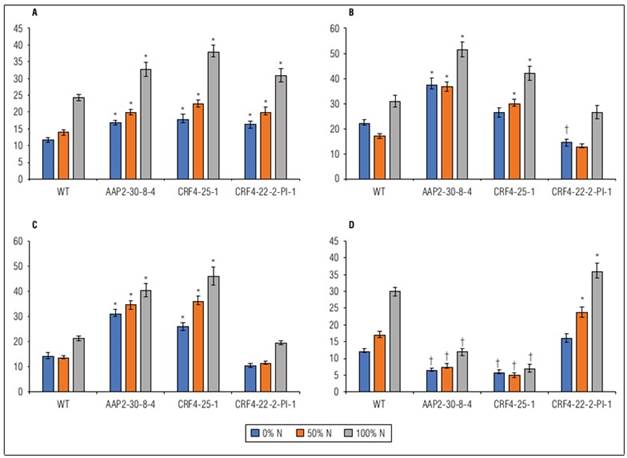
Figure 3. Means of WT, AAP2-30-8-4, CRF4-25-1, and CRF4-22-2-Pl-1 on dry biomass weight g/plant (A), stem number (B), panicle number (C), and filled grain weight g/plant (D) under different N application regimes in the second field experiment. Values are means±SE of 21 plants. * indicates a significantly higher value and † indicates a significantly lower value at P<0.05.
ANUE of the KO lines in the field experiment
ANUE was calculated after collecting agronomic parameter data. Significant differences were observed in genotypes, N application rates, and G x N interactions in ANUE (Tab. 2). All the original AAP2-KO and CRF4-KO lines exhibited significantly lower ANUE than WT, regardless of N application rates. The segregant lines, such as CRF4-22-2-Pl-1 at N50% and N100% and CRF4-25-1-Pl-1 at N50%, demonstrated higher ANUE than WT, although these were not significant. Overall, the field experiment showed a superior increase of ANUE in WT and the segregant lines with increasing N application, meanwhile, the reaction to N application was weaker in the KO lines (Suppl. Fig. 2).
Table 2. Agronomic nitrogen use efficiency (ANUE) calculated from the field experiment.
Genotype | Treatment | Agronomic nitrogen use efficiency (ANUE) |
---|---|---|
WT Nipponbare | 50% | 13.85±2.78 |
WT Nipponbare | 100% | 19.57±1.25 |
AAP2-25-1-4 | 50% | 3.10±0.11† |
AAP2-25-1-4 | 100% | 4.99±1.02† |
AAP2-30-8-1 | 50% | 5.68±2.91† |
AAP2-30-8-1 | 100% | 5.27±0.61† |
AAP2-30-8-4 | 50% | 2.60±2.47† |
AAP2-30-8-4 | 100% | 5.92±1.23† |
AAP2-30-8-10 | 50% | 4.81±0.14† |
AAP2-30-8-10 | 100% | 7.16±0.12† |
CRF4-14-2 | 50% | -0.33±1.49† |
CRF4-14-2 | 100% | 3.63±0.32† |
CRF4-22-2 | 50% | 0.90±0.64† |
CRF4-22-2 | 100% | 1.91±2.54† |
CRF4-25-1 | 50% | -3.17±1.38† |
CRF4-25-1 | 100% | 1.26±0.39† |
AAP2-25-1-3(2)-Pl-2 | 50% | 3.76±0.77† |
AAP2-25-1-3(2)-Pl-2 | 100% | 4.42±0.39† |
AAP2-30-8-1-Pl-1 | 50% | 9.20±2.08 |
AAP2-30-8-1-Pl-1 | 100% | 16.86±3.92 |
AAP2-30-8-4-Pl-1 | 50% | 11.85±2.13 |
AAP2-30-8-4-Pl-1 | 100% | 17.96±3.47 |
CRF4-14-2-Pl-1 | 50% | -0.53±2.83† |
CRF4-14-2-Pl-1 | 100% | 19.02±3.91 |
CRF4-22-2-Pl-1 | 50% | 16.96±3.13 |
CRF4-22-2-Pl-1 | 100% | 22.23±3.42 |
CRF4-25-1-Pl-1 | 50% | 20.21±12.12 |
CRF4-25-1-Pl-1 | 100% | 19.51±0.21 |
Genotype (G) | ** | |
N level (N) | ** | |
G x N | ** | |
CV % | 63.57 | |
Mean | 5.57 |
Values are means ± standard error (SE) from 21 plants. * significantly higher than wild type (WT) (P<0.05), ** significantly higher than WT (P<0.01), † significantly lower than WT (P<0.05).
NDVI and NDRE analysis in the field experiments
There were no significant differences in NDVI and NDRE values between genotypes at 60 DAS, 70 DAS, and 81 DAS, except for some plots at 60 DAS and 81 DAS (Suppl. Tab. 3; Suppl. Tab. 4). However, NDRE detected more differences at 88 DAS, with 11 lines of KO and KO-segregated lines exhibiting higher NDRE values than WT under different N conditions (Fig. 4). The dynamics of NDVI were similar between genotypes and N levels, while in NDRE, the dynamics between N levels were more distinct (Suppl. Fig. 3), and differences between genotypes were evident at certain time points. These findings align with the visual observations in the field, indicating that AAP2-KO and CRF4-KO lines retained their greenness for a longer duration compared to WT, particularly after flowering and grain filling.
Genetic analysis of CRISPR/Cas9 cassette
The existence of the hpt between original AAP2-KO lines varied, and all the original CRF4-KO lines possessed it as confirmed in the previous genotyping before the seed shipment (Fig. 5). Regarding the CRF4-KO segregant lines, the hpt gene was detected from six of the seven samples in CRF4-14-2-Pl-1 and CRF4-25-1-Pl-1, and all seven samples in CRF4-22-2-Pl-1. However, among the AAP2-KO segregant lines, AAP2-30-8-1-Pl-1, AAP2-30-8-4-Pl-1, and AAP2-25-1-3(2)-Pl-2 had the hpt gene in four, two, and three of the collected seven samples, respectively.
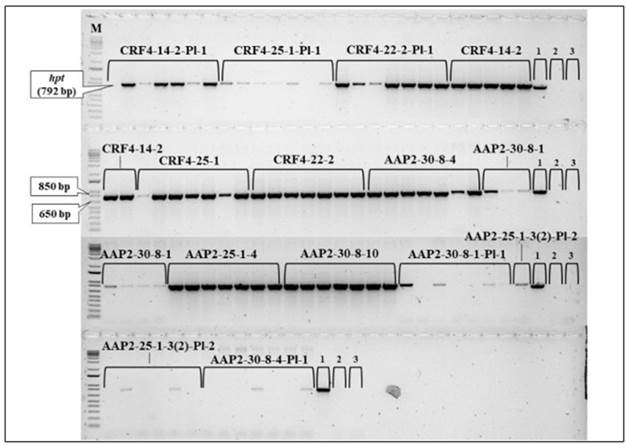
Figure 5. hpt detection by PCR between the rice lines in the field experiment. 1.2 % agarose gel with SYBR Safe DNA Stain (Thermo Fisher Scientific). The numbers in the figure indicate: 1, plasmid with hpt (positive control); 2, WT Nipponbare (negative control); 3, distilled water (control negative). M: 1Kb PLUS DNA Ladder (Invitrogen), 0.5 ug uL -1 .
Knockout of AtAAP2 and AtCRF4 homologs delayed senescence of rice plant
In our study, we used CRISPR/Cas9 to knock out the homologs of two selected A. thaliana genes in rice. The AAP2-KO and CRF4-KO lines exhibited prolonged greenness during the grain-filling stage, as indicated by NDVI and NDRE analysis (Fig. 4). This "stay-green" phenotype may contribute to increased biomass or yield (Borrell et al., 2001). However, the impact of “stay-green” is still debated in previous studies, with both positive (Borrell et al., 2000; Fu et al., 2009) and negative (Cha et al., 2002; Zang et al., 2022) effects reported. The AAP2-KO and CRF4-KO lines may possess a type B or C stay-green phenotype (Thomas and Howarth, 2000), as they showed similar VI dynamics to the wild type during vegetative growth but higher NDVI or NDRE values later on (Suppl. Tab. 3; Suppl. Tab. 4). The knockout of AtAAP2 and AtCRF4 homologs likely affected the N pathways in the rice plants, as senescence involves chlorophyll degradation and N remobilization (Zang et al., 2022).
One possible explanation for the observed features in the KO lines is that the knockout of the target gene(s) disrupted the translocation of N and photosynthetic products from the source to sink tissues. Another hypothesis suggests that the phenotypic features in CRF4-KO lines may be due to interactions between NRT2.1 and other nitrate transporters. AtCRF4 regulates the expression of the high-affinity nitrate transporter NRT2.1 (Varala et al., 2018), and previous studies have shown that OsNRT2.1 requires the partner protein OsNAR2.1 to enhance its N uptake activity (Feng et al., 2011). Altered N uptake was not observed when OsNRTs were overexpressed under the constitutive S35 promoter (Katayama et al., 2009). Transgenic lines of OsNRT2.1 with different promoters exhibited varying levels of agronomic nitrogen use efficiency (ANUE) and dry matter translocation to grains (Chen et al., 2016). The complete knockout of the AtCRF4 homolog in rice likely led to an abundant expression of nitrate transporters throughout the plant body due to the lack of the TF that represses their expression and might induce an overexpression-like condition. In a similar experiment, transgenic lines with OsNRT2.1 overexpression under a constitutive promoter displayed shorter plant height and lower yield (Chen et al., 2016), although not as extreme as observed in our CRF4-KO lines. These findings align with our observations.
The AAP2-KO and CRF4-KO lines did not exhibit improved ANUE compared to WT, likely due to lower yield and limited response to N application (Fig. 3; Tab. 2). The cause of this reduced yield is still uncertain. One possible explanation is that delayed senescence hindered N remobilization, leading to lower yield (Zang et al., 2022). Alternatively, lower seed setting rates could have inhibited shoot senescence by lacking the destination for N remobilization, as rice leaf senescence primarily occurs before plant death to facilitate nutrient transfer to seeds (Lee and Masclaux-Daubresse, 2021). Moreover, genetic variations among AAP2-KO genotypes might contribute to yield fluctuations and lower ANUE (Suppl. Tab. 1). The increased stem and panicle numbers in the KO lines could be related to the "stay-green" trait, which has been shown to positively correlate with fertile stem numbers (Luche et al., 2015). There is a study that observed similar trends with higher panicle numbers and shorter plant heights in stay-green lines (Sakuraba et al., 2015). Overexpressing the low-affinity nitrate transporter OsNPF7.2 also enhanced the tiller number and grain yield (Wang et al., 2018), suggesting interactions between stay-green trait, tiller number, and nitrate transporters in rice.
The observed phenotypic difference in the segregant lines might be due to the recovery of fertility as most of them showed normal or higher yield per plant than WT (Suppl. Tab. 2). However, detecting the genetic differences between the original KO and segregant lines can be challenging since they may be due to somaclonal or off-target mutations, although our gRNAs were designed to reduce the possibility of this unfavorable event (Miyao et al., 2012; Zhang et al., 2015). Detecting genetic mutations in non-target regions is usually more laborious than sequencing the target regions (Shillito et al., 2021).
CONCLUSION
Both AAP2-KO and CRF4-KO rice lines showed distinct phenotypic features from WT such as shorter plants, increased stem and panicle numbers, and dry biomass weight in the two field experiments. The KO lines also showed delayed leaf senescence; thus, it is evident that the KOs of the target genes affected the N cycles and pathways in rice plants. However, neither AAP2-KO nor CRF4-KO showed better yield or ANUE than WT. This study demonstrated the usefulness of GE technology in gene evaluation and highlighted the effects of AtAAP2 and AtCRF4 genes in the plant N cycle.