Introduction
The interest in high order finite elements methods (FEM) in different areas has increased significantly during the last decade, especially in engineering. FEMs provide good accuracy with a reduced computational cost and save resources in trials usually very expensive in both time and money.
Multiple software can be used for the simulation of detonations. Some of them, such as LS-DYNA (LSTC, 2019) or ANSYS, offer general finite element solvers for different kinds of formulations (Lagrangian; Smooth Particles Hydrodynamic - SPH; Particle Blast - PB; Multi-Material Arbitrary Lagrangian Eulerian - MM-ALE); or methods (Load Blast Enhanced - LBE; explosive Equation of State - EOS) related with explosives or their effects. Others, like ProsAir (Forth, 2012), are standalone compressible Computational Fluid Dynamics (CFD) codes developed specifically for assessing blast loading and blast waves with finite volume method. ProsAir uses the AUSMDV Riemman solver, an improved advection upstream splitting method (AUSM) together with the MUSCL-Hancock integration scheme to achieve a second order of accuracy.
In a numerical study, discretization error estimation would be desirable due to the dependency of the solution on the mesh size. This error is present in our solution, even when the results obtained agree with real data, e.g. field test or laboratory experiments. Another error present in the results is the modelling error itself, which is related to the simplifications made to the physical problem or the mathematical implementation of various factors (e.g. boundary and loading conditions, material properties, or constitutive equations) (Anderson et al., 2007). The Grid Convergence Index - GCI (Roache, 1994) is a method to estimate the discretization error even when the successive mesh refinements are not integer multiples. This technique is defined as an error percentage, providing a confidence bound in which the numerical solution will likely to be. Several authors used the mentioned GCI in computer fluid dynamics (Jin and Shaw, 2010; Liang and Tao, 2017; Ndebele and Skews, 2019). However, it is less mentioned in discrete methods (Kwaśniewski, 2013; Schwer, 2008), with no sources found for shockwaves produced by explosive detonation. According to Roache (1994), the GCI method would be recommended for shocks and other discontinuities despite further experience with complex shocked flows is needed. In addition, Huang et al. (2012) highlighted the need of further research to contrast simulated results with field data and their validation.
To verify the efficiency and accuracy of the GCI method, the present work presents a comparison of both ProsAir and LS-DYNA approaches with the data obtained from experimental studies of blastwave using homemade explosive. To reproduce the explosive behavior in this software, the TNT equivalent is necessary, since the results are better with the accuracy of this calculation. Therefore, this work also has served to validate the methodology used in that calculation (Chiquito et al., 2019).
Field blast testing program
A total of 18 tests with different explosives were conducted. However, the results in terms of energy released by the explosive were too low for five out of the six mixtures used. In this work, only the mixture that resulted in a higher TNT equivalent in pressure was considered. More information about the tests is available in the paper by Chiquito et al. (2019).
The explosive selected in this work was a mixture of ammonium nitrate with aluminum. The technical ammonium nitrate used in the tests is usually produced for industrial purposes and it is basically pure ammonium nitrate with high porosity. The specifications provided by the manufacturer ensure that a minimum of 98,5% is ammonium nitrate. The aluminum powder was added to the ammonium nitrate at 10% by weight. The aluminum used was already in powder form with a size of 230 microns and a purity of at least 98%. All mixtures were done inside a plastic bag and introduced in powder-free latex gloves, easily found in any store. The charge was approximately spherical in shape with 15 cm diameter and it was hanging on a rope at 46 cm from the ground in all tests.
In order to measure the shock wave produced by the explosive studied, five high frequency PCB® pressure sensors were placed on a flat ground around the explosive in two concentric circles whose radios were 3 and 5 m. Two sensors were located at 3 m from the charge (called P1 and P2) and three at 5 m (P3, P4 and P7) with an angle of 0°, 45° and 90°, as Figure 1 shows.
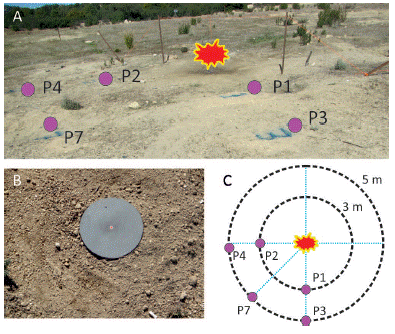
Source: Authors
Figure 1 Experimental setup: (A) Photograph of the field settings; (B) cup used to place the pressure sensors, ensuring that they do not move; and (C) detail of the pressure sensor location and distances.
After three tests with the same explosive, six signals were available at 3 m, while there were nine signals at 5 meters. The obtained TNT equivalent based on pressure and then used in the numerical models had a weighted average of 5 measurements at 3 m and 9 measurements at 5 m, as one of the measurement at 3 meters was useless. For signals treatment, a code in MATLAB® was developed based on least squares method to fit the modified Friedlander Equation. With this fitting, the key parameters of the positive phase can be extracted. See Chiquito et al. (2019) and Rigby et al. (2015) for more details. Finally, the TNT equivalent is calculated and the value obtained was 0,855.
Methodology
The success of modelling an event using any technique that require a discretization of the problem, like finite volumes or elements or CFD, depends strongly on the chosen mesh size. Consequently, a convergence study known as Grid Convergence Index (GCI) was carried out in order to find the proper mesh size for this kind of computational models.
The Grid Convergence Index (GCI)
The choice of finite time and space domain introduces a discretization error into simulation. Therefore, a numerical solution can only be treated as an approximation to the real one. In order to find how big this error is depending on the mesh size, the Grid Convergence Index (GCI) method is applied. Presented first by Roache (1994), the GCI can be defined as the relative error bound, i.e. the measure of how far away our computational result is from the asymptotic numerical value. It indicates the error band in which our error is located and how big it could be. Besides, it shows how much the solution will change with a greater refinement of the mesh, as low GCI values will indicate the computational solution near the asymptotic one. If the solution is already good enough, smaller meshes will not result in big different solutions. Assuming that, in general terms, the results obtained with a finer mesh are better although some authors have shown that this is not always the case (Alañón et al., 2018). This technique provides some key advantages when compared with others: an analytical solution is not required, it provides a confidence bound on the estimated error band, and can be used with a minimum of two mesh solutions (though it works better with three).
In the traditional processes of illustration of the convergence error, the analytical solution (exact) was used to determine the error, and then the range of convergence was determined graphically. However, in practical problems, the exact solution is usually unknown. Most traditional discretization methods assume a relation between the analytical solution f exact and its numerical approximation f(h), and then calculate the discretization error E(h), using the Equation (I) and neglecting higher-order terms when the mesh is appropriately refined (Roache, 1994):
where h is a measure of the mesh discretization, A is a constant and p is the rate of convergence. Therefore, three unknowns remain: the constant A, the convergence ratio p, and the exact solution f exact . Estimating these unknowns is the basis of the GCI method.
In this case, the application of the GCI was done with three mesh refinements with a constant grid refinement ratio. The meshes used in this study (called C, D and E) resulted in the following ratio, r = h c /h D = h D /h E = 2. The biggest one was called "Mesh C - h C " (12,5 mm), followed by "Mesh D h D " (6,25 mm), and the smaller one, "Mesh E - h E " (3,125 mm). To obtain an estimation of the order of convergence, the concept used in Equation (1) is applied to the three mesh sizes CDE, where h C > h D > h E . Then, the unknown constant A can be eliminated and the unknown p can be obtained (Schwer, 2008):
Additionally, once the order of convergence is known, an estimate of the analytical result can be calculated by finding the asymptotic solution for h approaching zero and using the two finest grids from the threesome CDE (Schwer, 2008):
After some algebraic work, and starting from Equation (3), it is possible to relate the relative error (ε) of the finer meshes to the mesh (r) and convergence (p) ratios. Details of this can be found in Kwaśniewski (2013) and Schwer (2008). However, the definition of the relative error is as follows (Schwer, 2008):
which never should be taken as an estimate of the relative error of the methodology, since it does not reflect r or p in the formulation.
The expression that gives the error value of the GCI is presented in Equation (5), whose solution can be expressed as a percentage (Schwer, 2008).
where F s expresses the safety factor multiplying the relative error term, based on the application of the GCI in different situations, but always with CFD (Roache, 1994). This error is an estimate of the finest mesh used, relative to the numerical (converged) solution as, in general, the exact solution is unknown. The value of this factor is 3 when two meshes are studied, or 1,25 when there are three or more meshes. The latter value was used in this particular research. This safety factor represents the 95% confidence for the estimated error bound.
Finally, the extrapolated (or computational) solution, called f* ED for the finer mesh combination, provides an estimate of the numerically asymptotic solution (Schwer, 2008):
This methodology can be applied only when all grids are within the asymptotic range and then Equation (6) is valid asymptotically (also the extrapolated solution for other mesh combination). Based on the idea that the asymptotic range of convergence requires the ratio between errors and mesh spacing to be constant, it is possible to verify if it is met by comparing the values oí two given GCI, as three meshes are available (Kwaśniewski, 2013).
Finally, as the GCI only gives the bound within the error that should be, Equation (8) shows the procedure to obtain the range with a 95% confidence that the converged solution should be (Schwer, 2008).
Pros Air modelling process
The first program used to reproduce the field test was ProsAir - PROpagation of Shocks in AIR (Forth, 2012). This is a computational fluid dynamics program developed by Craníield University, which simulates the effects oí the detonation of an explosive charge, using a high-resolution, finite volume scheme.
The configuration and use of ProsAir is relatively simple. First, there are four tabs: general settings, spherical geometry, cylindrical geometry and 3D geometry. In the general settings tab the number of CPU cores to be used and the atmospheric conditions (101 325 Pa; 288 k) can be defined, among other less important set-ups. Then, in the spherical and cylindrical geometry tabs, the parameters to be plotted (the overpressure) and its intervals can be assigned. The spherical geometry has been used to mesh the explosive and the cylindrical one to mesh the air. Moreover, the mesh cannot be visualized, but it is a regular structured square. Besides, in the spherical geometry tab, the explosive type and its mass are set. Other control parameters are also established, like simulation time, CFL (or Courant-Friedrichs-Levy) safety number equal to 0,5, cell size (for the explosive, not the one used in the GCI), domain size (defined as 7 m in radial direction and 4 m high - see Figure 2) and type of boundary. Finally, the cylindrical geometry tab has the same control parameters as the spherical one, including the cell size (the one used to define the air - the same as defined in the GCI) and the height of blast.
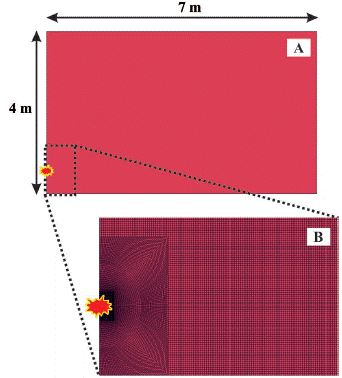
Source: Authors
Figure 2 Model domain: (A) Numerical model domain in LS-DYNA. Note that the ProsAir domain is the same; however, the mesh cannot be seen in the software until modelling has been done. B) Mesh detail of the source point with a butterfly mesh to ensure correct wave propagation at the initial stages.
The ProsAir computational tool was used to reproduce the experimental tests defined above based on the TNT equivalent calculated for the explosive used. In order to check its behavior, the prediction of the pressure caused by the blast wave of a specific spherical TNT mass in two different ranges from the center of the explosion (3 and 5 m) was compared with the GCI prediction, and then with the real (field) data. The data provided by the software depends on the equation of state (EOS) defined for the explosive and the input data used by the program. On the one hand, ProsAir does not allow to modify the Equation of State (EOS) and the manual does not specify what it is. On the other hand, the input data are: density 1 600 kg/m3, detonation velocity 6 730 m/s and heat of explosion 4 520 kJ/kg.
As mentioned before, the ProsAir software is based on finite volume method, and then it depends on the mesh size. Usually as the mesh size is changed, the pressure also varies, as well as the time consumed by the computer to finish the simulation. As it is not always possible to predict the behavior of the shock wave modelling, the study of mesh convergence (GCI) becomes fundamental.
LS-DYNA modelling process
The second software used for comparison is the finite elements software, called LS-DYNA. This software, in comparison with ProsAir, requires a lot of training and knowledge in order to be used with confidence. It has many different options, but that also increases its complexity. In this case, the general 2D multi-material arbitrary Lagrangian-Eulerian (MMALE) technique was used. The *CONTROL_ALE card was set with Van Leer and half-index-shift advection algorithm of second order accuracy (METH=2). The alternate advection logic was also used, as it is generally recommended for explosives simulation.
The air was meshed as an axisymmetric domain equal to the one defined in the ProsAir (7 m long and 4 m high rectangle - see Figure 2). The explosive was set with the card named *INITIAL_VOLUME_FRACTION_GEOMETRY, defining the container geometry by a sphere and following similar previous works (Mobaraki and Vaghefi, 2015; Rebelo and Cismasiu, 2017). As the data is required by volume, knowing the TNT equivalent and the density of the TNT introduced into the model (Table 1), the explosive volume (m3) was calculated. The chosen meshing technique was the butterfly one, in order to ensure the good spherical shape of the shock wave as other authors suggest (Lapoujade et al., 2010 and Rigby et al., 2014). The necessary data for the EOS used in this case, the traditional Jones-Wilkins-Lee (Lee et al., 1973), are shown in Table 1.
Table 1 LS-DYNA inputs for the cards *MAT_HIGH_EXPLOSIVE_ BURN and *EOS_JWL, see Equation (9)
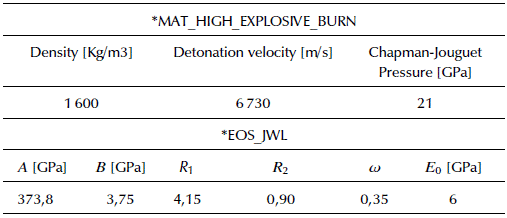
Source: Lee et al. (1973)
where V is the relative volume, E the internal energy, and ω the Grüneisen coefficient.
The air was modelled as a perfect gas using the material *MAT_NULL and including a density of 1,29 kg/m3, an initial internal energy of 0,25 MPa, and a specific volume of 1 (Huang et al., 2012). The card used to describe the equation of state for the air was the *EOS_LINEAR_POLYNOMIAL, where C4 = C5 = 0,4 and the rest of constants is equal to zero (Huang et al., 2012).
Results and discussion
Optimal mesh size estimation
The objective of this phase is to find an optimal grid able to reach enough precision to study the pressures caused by a free air explosion. Table 2 shows the real pressure obtained in three tests (five measurements) at 3 meters (mean value and standard deviation, after the ± symbol), and LS-DYNA and ProsAir pressure results for the three meshes used. The shortest distance of 3 meters has been chosen for comparison and validation of the data produced by both software, based on the TNT equivalent calculated. As some authors suggest, at lower distances the calculation is more difficult due to the presence of the highest overpressure and impulse (Huang et al., 2012; Rose, 2001). This point is considered as the most adverse situation and the one that will set the best mesh.
Table 2 Pressure peaks of the three mesh sizes for each solver in comparison with the empirical result
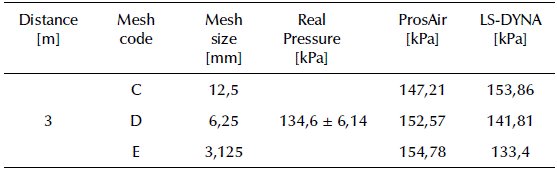
Source: Authors
A similar procedure to fit the pressure signals with a Friedlander equation has been done with both data set, ProsAir and LS-DYNA. Analyzing the behavior of the results given by LS-DYNA, we can easily observe a wider range of results than in ProsAir. In each refinement, the variation in the outcome gets closer to the converged solution for LS-DYNA, while a refinement of the ProsAir model just gives a systematically higher pressure. The wide dispersion of LS-DYNA means that this solver has a bigger dependence on the solution with the mesh size than ProsAir. The relevance of choosing a correct mesh using LS-DYNA is a key factor that can make the difference to provide good results. Regarding calculation time for the same mesh size, ProsAir was significantly faster than LS-DYNA.
Table 3 sets the results of the application of the GCI method to the outputs provided by LS-DYNA and ProsAir for the 3 meters pressure peak. In general terms, shocks, non-linear flux limiters and other discontinuities invalidate the basis of GCI (Taylor series in Richardson extrapolation). However, if these phenomena exist at small scale and with simple patterns, the GCI method seems to be valid and recommended (Roache, 1994). In any case, checking if the asymptotic ranged has been reached is generally enough to apply with certainty the GCI method.
Table 3 Results of the application of the GCI method to the outputs provided by LS-DYNA and ProsAir (values at 3 meters)
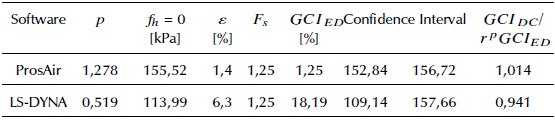
Source: Authors
Table 3 shows the calculations for each mesh: the order of convergence p, the estimated asymptotic solution f h = 0, the value between subsequent solutions ε, the safety factor (set in 1,25 for three or more meshes) and the GCI for the meshes E and D. It also shows the confidence interval whereby there is a 95% confidence that the converged solution is within this range, and the verification that all calculations are in the asymptotic range of convergence (Equation 7).
The formulations made with ProsAir, which requires reduced computation load, have lower GCI error value (GCI ED ) as shown in Equation (5) and higher convergence order (p) than the LS-DYNA model. This difference is because the results with ProsAir change less with each refinement of the mesh than in LS-DYNA, resulting in an estimation of p that seems to be better. The GCI method provides a different estimation of the asymptotic solution and confidence interval for both solvers. This is logical since the behavior of the software, in this case, is opposite when the mesh changes. The ProsAir estimation ranges from 152,84 to 156,72 while the values for LS-DYNA range from 109,14 to 157,66 presenting a wider range where solutions can be found. In fact, when comparing this confidence interval with the data provided in Table 2, it is possible to affirm that not all the results obtained with ProsAir are within the interval, while all the meshes made with LS-DYNA are in the range.
Finally, it is been verified that all solutions are within the asymptotic range of convergence, so the GCI method can be properly applied, as GCI ED and GCIDC are compared and the values obtained are similar to 1 (Table 3). Additionally, it is observed that all the estimated asymptotic solutions for the finest meshes are between the 95% confidence intervals. Remark that all the values shown in the table are estimates, and, for instance, despite the real order of convergence used with the solver LS-DYNA p = 2, the estimator (p - Table 3) gives a different value. The same happens with the ProsAir. As it is an estimator, the order of convergence is calculated by converging to different asymptotic solutions.
Although the analysis is being carried out on an extremely complex event, such as the shock wave, it can be stated that the GCI is a method that can work despite its dependence on the software used. From the analysis made, the better mesh size is the finest and equal to 3,125 (mesh E) in both, ProsAir and LS-DYNA.
Comparison of the simulation with field data
Table 4 shows the pressure peak results obtained for both software at two different distances (the smaller one, E), thanks to the GCI method. Real pressure peaks are also shown with its standard deviations (after the ± symbol) for 3 and 5 meters. If the pressure is within the measured limits in the field it is signalized with wml in the table, otherwise the relative error is displayed right next to the solution.
Table 4 Comparison of the numerical results with real data and its respective relative errors
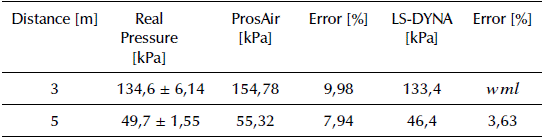
Source: Authors
On the one hand, the LS-DYNA model gave better results, closer to the experimental pressures. One of them (3 m) was within the measurement limits and the other one had a relative error not exceeding 5%. On the other hand, the ProsAir results differ to a larger extent. Nevertheless, none of the results surpasses the 10% error, which is a very acceptable outcome in the engineering field.
For example, pressure history at 3 and 5 meters from the experiments ProsAir and LS-DYNA is compared in Figure 3. The timing and shape of the overpressure peaks were captured accurately by the simulations. In terms of arrival time, ProsAir tends to go faster, while LS-DYNA behaves in the opposite way. The impulse, defined as the effect of the pressure over some target for a specified time period, is not compared here since the equivalent used was for the pressure peak. However, in Figure 3 it is possible to support that the impulses could be estimated with confidence, knowing that ProsAir overestimates the impulse and LS-DYNA underestimates it. These results are in accordance with others in terms of LS-DYNA and Air3D (similar to ProsAir) behavior (Huang et al., 2012; Rigby et al., 2014; Trajkovski et al., 2014).
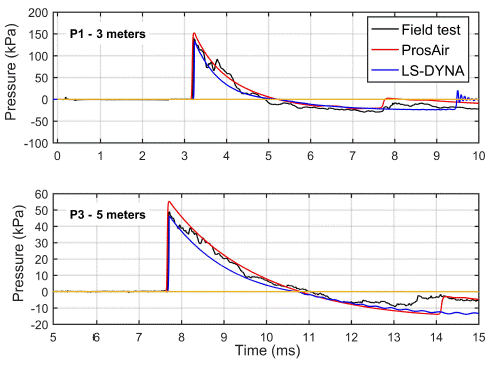
Source: Authors
Figure 3 Comparison of experiments results: ProsAir and LS-DYNA analysis. Tests number 3 signals in P1 and P3 (see Figure 1).
In general terms, the TNT equivalent used here appears to be in the lower range when used in LS-DYNA, while it is in the higher range for ProsAir. The TNT equivalent used here mixed data from two scaled distances, for this reason, the error changes with the distance. It is well-known that the TNT equivalent depends on the distance (Swisdak, 1975). However, as mentioned by Rose (2001), this observation is generally overlooked by most researchers because it adds a level of complexity to already very complicated problems, which makes the whole process of prototype or numerical modelling almost insoluble.
Conclusions
Evidently, the problem of suitable TNT equivalence will continue to make difficult the applicability of experimentally - and numerically-based results in the future. However, this work should serve to reduce the lack of existing experimental and numerical data in this field, in order to improve the understanding of the TNT equivalent and its use in modelling.
The mesh refinement study is the first step in the verification of numerical models. The presence of shocks, other discontinuities or singularities can difficult grid convergence studies. A priori, the GCI results (if the real result is unknown) would seem more consistent for ProsAir. This is normal because the GCI is designed for CFD. However, the results obtained for a complex finite element program as LS-DYNA are satisfactory.
The peak pressure results obtained using LS-DYNA and compared with field data are better. However, the calculation time used is much longer. The use of one program or the other depends on the available calculation capacity, the user knowledge and the required precision.
Regarding the mesh refinement, the variation in the outcome gets closer to the converged solution for LS-DYNA, while a refinement of the ProsAir model just gives a systematically higher pressure. The relevance of choosing a correct mesh using LS-DYNA is a key factor, making the difference to provide good results. The results provided here allow us to select the proper air mesh for future or more complex simulations involving, for example, explosives and structures.