Introduction
The WOM communication channel is a common way of creating enduring customer value through the influence of online communities (Kumar and Reinartz, 2016; Li and Du, 2017; Li et al., 2018a; Rintamäki and Kirves, 2017). Nowadays, digital marketing strategies have become a global tool for WOM recommendations which come from family, friends, and social networking (Li et al., 2018b). Sharing information contributes to the awareness process and it has even affected on a greater scale the purchase process. At first the exposition may be seen attractive and can encourage consumers to buy and further share information about their experiences (Misirlis and Vlachopoulou, 2018; Wang and Zhang, 2017).
This situation gradually generates consumption patterns that change by slowly increasing the purchase of similar products. This could be related with friends and acquaintances’ products reviews, and, as a result, the message can reach millions of consumers through multiple exchanges and interactions (Françoise and Tosan, 2018). Other studies agree that the informal exchange of information through personal conversations can influence consumer choice and decision-making (Lee et al., 2017; Wouters and Kirchberger, 2015; Wu et al., 2018). Compared to traditional advertising, WOM communications have big advantages such as significantly lower costs and much faster propagation; this is particularly true for online social networks (Li and Du, 2017; Li et al., 2018b; Relling et al., 2016). In this context, what is the function of WOM for online brand communities and what role does it play on food consumption?
It is becoming increasingly difficult to ignore the importance of the Internet as a marketing strategy. Thus, Internet-based strategies are having a viral effect on customers. Research into these strategies has been carried out by several studies (De Pelsmacker et al., 2018; Li et al., 2018b; Peres et al., 2010). Although diffusion modeling has been researched extensively, there are new potential directions that can be explored in emerging economies, web-services, social networks, and complex service product structures (Peres et al., 2010). For instance, the agent-based models only help to understand the role knowledge plays in diffusing competitive brands (Jiang et al., 2016), but do not help to understand the complexity approach to market systems (Cosenz and Noto, 2016; Herrera-Ramírez et al., 2017). This paper carried out a simulation model to understand brand development and the viral market effect on online communities which allows market penetration to be analyzed or adopted in complex service product structures.
The diffusion of technology is a process where new products are spread within and across economies through innovation (Korres, 2012; Lee et al., 2015; Walrave and Raven, 2016). Hereby, this paper attempts to analyze market penetration or adoption using simulation scenarios for brand development; this contrasts with other studies which uses dynamic structure for modeling through integrating the development of products with an innovation process (Ford and Sterman, 1998; Walrave and Raven, 2016). Our work contributes to market penetration modeling from product development dynamics and viral market effect on close-loop perspective.
Studies on the innovation implementation process suggest that three new constructs (including motivation threshold) characterize the dynamics of implementation (Repenning, 2002). Our paper carried out an analysis of viral markets which provides insights into the motivation threshold. Thus, the importance of studying the effect of WOM on market dynamics through simulation scenarios allows viral marketing to be implemented and then the effects of market policies can be understood.
Advances in technology and the ways people connect in virtual environments have led to an exponential growth in the amount of WOM information accessible to consumers. Social networking can be a powerful tool to guide the collective moral judgment. Although some authors discuss the relevance of online groups and communities, they state that the community has been at the heart of the Internet since its inception. The simple status of individuals as members of a green products group can generate a common identity. Social interaction, such as chat rooms and customer feedback, has the potential to increase consumer influence. The importance of the Internet as a marketing strategy provides space for a wider range of relationships between people who recognize that they have something in common with a sense of community and solidarity.
A social network is a platform that groups users together so that they can establish friendships and interact with each other based on interests they have in common. Social networks connect users through sharing thoughts and building friendships. The high degree of privacy among users facilitates WOM marketing. Information is quickly dispersed on a social network and it is generally highly valued by the recipients. For this reason, social networks are commonly used for WOM marketing (Balaji et al., 2016; Li and Du, 2017; Park et al., 2017).
This paper develops an analysis of the effect of WOM through viral marketing for product development. Simulation methodology is used with dynamic systems that show consumer behavior in two different time scenarios that relate to product development and the spread rate of viral marketing.
In the following section, literature on WOM in online communities is discussed, the method, data, and results obtained are then presented. Finally, the paper concludes with a discussion and conclusions for managers and policy makers.
An overview of WOM in online communities
WOM is perceived to be a credible and trustworthy tool and it plays an important role on the web, blogs and social networks which can employ several WOM activities such as: community marketing, referral programs, viral marketing, and guerrilla marketing (Agag and El-Masry, 2016; Li et al., 2018a; Wu et al., 2018). In this sense, WOM has an intentional influence on consumer-to-consumer communications through marketing techniques. It is influenced by the desire to help others: to warn of bad experiences that consumers have suffered (Balaji et al., 2016; Chu and Sung, 2015; Standing et al., 2016). Some studies have affirmed that WOM is one of the most influential communication channels and are used more than other marketing communication channels. They also expect WOM’s influence to continue due to an explosion in communication channels (Chu and Sung, 2015; Krush et al., 2016; Sicilia et al., 2016).
As multiple applications of the Internet have been developed, purchasing commodities online has become more popular and easier to access (Lee et al., 2017). The importance of choosing a correct strategy for viral marketing in digital media is reflected in Figure 1, where it is evident that the number of digital transactions are increasing year-by-year. This trend is expected to continue over coming years.
In the field of product planning and development, WOM can play an important role in the purchase process because the product idea is not yet established in the minds of consumers, therefore, they require information to decide whether to make a purchase. Thus, the WOM is an informal communication channel between customers and consumers making a purchasing decision (Basri et al., 2016; Lim and Chung, 2011). According to Foroudi et al. (2018), consumers with a high motivation to process information are willing to accept recommendations and switch from declared attribute preferences, but they only choose optimal products. Moreover, WOM can predict the aggregate demand when a product is being pre-launched, which is why it is essential to make people talk frequently and favorably about the product to the right people (Lee et al., 2017).
Statistics show that WOM is more credible than other marketing techniques; however, Ho and Dempsey (2010) examined one of the critical factors, namely Internet users' motivations, to tell others about online content. In her study, motivation was associated with the viral marketing of online content. Also, people endorse a product or service to their families, friends, or colleagues, but most influencers have no personal interest in the product itself. Most people seek advice from family and friends to reduce the possibility of making a wrong decision.
WOM, either for a brand or a product, is developed by three key market drivers: the functional, the social, and the emotional (Lovett et al., 2013). The functional and social factors are relevant in the online-based WOM, while the emotional link is more important in the offline-based WOM. However, it is important to determine how methods can influence positively or negatively (Balaji et al., 2016). Online review platforms can effectively aggregate positive, negative, and neutral opinions into readily accessible online WOM, which has become a critical source of information that is reshaping the behavior of online consumers by reducing product uncertainties (Wu et al., 2018). A negative view is more counterproductive in sales than a positive one; this is because the positive opinions are less valued than the negative ones, possibly because people often tend to speak positively (Chen and Lurei, 2013). One way to achieve positive WOM is to provide certain types of gifts as products or Point of Purchase (POP) material; this allows the WOM to relate positively to the target market (Berger and Schwartz, 2011; Reimer and Benkenstein, 2016). In conclusion, organizations must work to improve their image through holistic marketing, which will determine and transmit the concept that customers have regardless of the type of relationship or contact with the company.
Unfortunately, empirical evidence on measuring WOM in sales is currently limited. This raises the need to measure the effects of WOM over time. Figure 2 details the first authors who measured the effects of WOM on the market. The very first authors to address the empirical evidence were Katz et al. (1955) who conducted a self-report of surveys. The results showed that WOM is twice as effective as radio ads, four times more than sales staff, and seven times more than print ads (Trusov et al., 2009). Coleman et al. (1966), realized that WOM contagion effects disappear when traditional marketing strategies are used. In early 2000, a Web-based study on message inferences was performed, which stated that the more comments a product could get, the more sales it is going to obtain (Chevalier and Mayzlin, 2006). Customers attracted through WOM strategies will spread the WOM and generate twice as many new customers (Villanueva et al., 2008).
In short, a satisfied customer has the potential to influence many people; therefore, the development of WOM allows a greater impact on the market to be generated than any other advertising project (Chen et al., 2017; Eelen et al., 2017; Mishra et al., 2016). However, the strategy must be accompanied by an honest and authentic message that not only promotes the goods or services but also presents the concept of what they represent. The brand must create customer engagement (Harrigan et al., 2018; Prentice and Loureiro, 2018); therefore, a client who knows the brand has the ability to share more complete and detailed information.
Simulation modeling
The methodology developed is based on simulation modeling with system dynamics and takes into account the points raised by Forrester (1997), Mollona (2008) and Sterman, (2000)). Simulation models with system dynamics were used to analyze the behavior of innovation management in product marketing penetration. This is useful to the extent that customer dynamics are shown over a period of time (Peres et al., 2010; Repenning, 2002; Walrave and Raven, 2016).
All the computer work was carried out using Stella Software. The design of the simulation model was based on customers making a change in their agribusiness companies and supply chain model (Herrera-Ramírez et al., 2017; Orjuela-Castro et al., 2017). It was decided that the best methodology for this research was system dynamics modeling due to the complex service of product structure on close-loop.
The model constructed includes four levels of variables which measure the number of new customers, the potential market, consumer base, and lost customers. The levels of variables are regulated by product development time, the market share that a consumer base has, and the rate of marketing penetration through viral marketing strategies. In Figure 3, the simulation diagram shows changes from the potential market level to the consumer base or lost customers. The proposed model is used to give an answer to the following question: In terms of market dynamics, what are the WOM´s determining factors which allow the use of viral marketing in product development?
The structure of this model allows to understand the behavior of market dynamics that are associated with times and content presentation (viral marketing). The simulation model presents two main variables for the WOM-Viral Marketing model. Equation 1 represents the consumer rate over time, which allows understanding the delay in the decision making of consumers within the product development time.
Equation 2 shows the relationship between sales accumulated over time and possible sales (Michelfelder and Morrin, 2005):
Where,
ft = (change in cumulative sales at time t) / (potential sales),
r = rate of penetration of potential sales,
M = (total potential sales) / (all buyers), or market saturation percentage.
Equation 3 presents the WOM commercialization process considering a WOM marketing campaign that begins at time (t) = 0 and ends at time (t) = T. It defines the target market for the campaign at time (t) as the set of consumers and potential consumers involved in the campaign at time (t). XM (t) denotes the size of the target market at any moment. Due to the impact of many known factors, XM (t) is often uncertain. Let M (t) denote the expectation of XM (t), (Li et al., 2018b).
Simulation tests were carried out on customer and customer acquisition rates. The design shows four scenarios of simulation within product development according to WOM effect (viral market) and the time it takes to develop a product. To increase the reliability of measurements, each simulation scenario was tested by using marketing managers from the different agribusiness companies that were consulted. Figure 4 provides the strategy to simulation model used in this case. The first stage determines the actor`s system customers of agribusiness companies. Stage two begins by laying out the relationship between actors’ systems. Stage three, assesses the variables used in the simulation model. Finally, stage 4 shows the simulation scenarios with the WOM effect.
Results
The results were derived from the case study of marketing penetration of a new product developed in agro-food. Four different scenarios were designed for the time it takes to develop a brand and the marketing penetration level or viral marketing.
Table 1 shows the summary of the four scenarios designed to assess the time it takes to develop a brand and disclosure index (viral marketing). The experimental design was based on determining development times for agro-industrial products with more or less, eight months and a market penetration of approximately 50%. Marketing managers provided the simulation scenario data from the different agribusiness companies that were consulted; thus, the level of the potential agribusiness market represents the number of wholesale customers each company has. Moreover, new customers’ behavior shows the number of consumers who enter the agribusiness market. The study was based on one-hundred major agribusiness customers.
Table 1 Results of simulation scenarios for WOM and brand development time.
Scenario | Brand development time | Viral marketing | Potential Market | New customers |
---|---|---|---|---|
Pessimistic (1) | High (> 8 months) | Low (> 50%) | 46 | 137 |
Pessimistic (2) | High (> 8 months) | High (<50%) | 27 | 147 |
Optimistic (3) | Low (<8 months) | Low (> 50%) | 60 | 108 |
Optimistic (4) | Low (<8 months) | High (<50%) | 38 | 119 |
Source: Authors’ own elaboration.
The first pessimistic case scenario shows more time for brand development and a high level of diffusion and marketing penetration (intensive marketing strategies). Figure 5 shows the effect that time has on product development for the consumer base and that the potential market increases. However, the number of consumers who stop buying the product also tends to increase, which implies that the number of consumers within the actor´s system quickly stop buying the product; therefore, the level of marketing penetration will not be enough to increase the number of consumers. On comparing the results of the consumer base and lost customers, it can be seen that the potential market significantly decreases. A possible explanation for this might be the increase in brand development time.
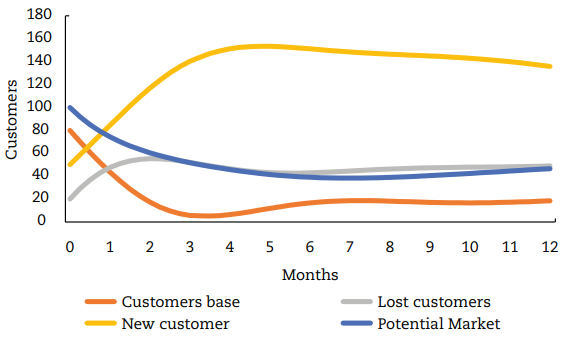
Source: Authors’ own elaboration.
Figure 5 Scenario 1: More time spent on brand development and increased market penetration.
On the other hand, if there is an increase in product development time, the product may also suffer from low marketing penetration as well as less intensive and more targeted strategies. In this scenario, the number of consumer increases as does the number of consumers who stop buying the product. Figure 6 shows the effects of a decrease in viral marketing strategies. The effect of the reduction in viral marketing strategies generates an increase in the potential market and potential consumers of the goods and/or services. This implies an increase in the number of customers and an increase in the number of consumers who stop buying the product. This remains constant over time and in the future this will have effect on the number of consumers, generating the need to create a new but less intensive market strategy. Scenario 2 shows that there was no significant difference when considering new customers minus lost customers. However, these results show that the customer base increase was due to a viral market increase.
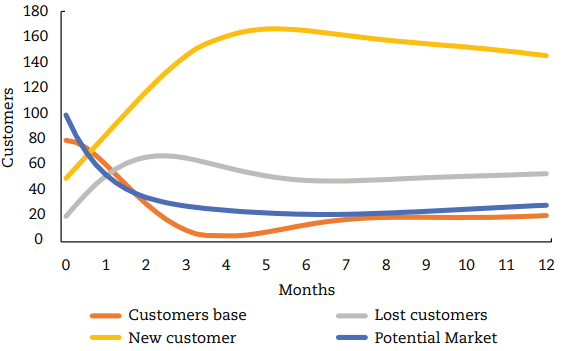
Source: Authors’ own elaboration.
Figure 6 Scenario 2: More time on brand development and low market penetration.
Figure 7 shows the optimistic scenario that envisages a shorter time for brand development and a less intense and more targeted marketing penetration strategy. This scenario considers that an increase in the cost of product development is offset by a more targeted economic strategy. The potential market increases, but the number of consumers decreases, which implies a larger drop that occurs when the development time is greater. This means that the increased costs for brand development in the shortest possible time do not imply that the product or service will have a considerable impact on potential consumers. The results from scenario 3 show that the decrease in new customers is linked to the lost customers.
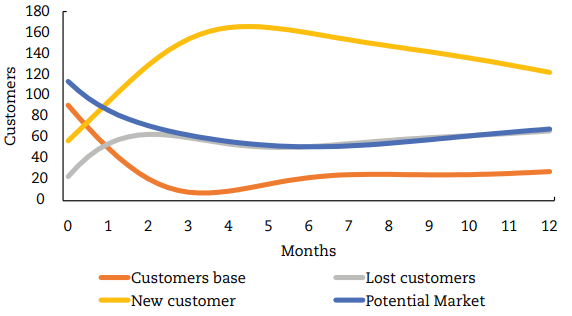
Source: Authors’ own elaboration.
Figure 7 Scenario 3: Shorter time spent on brand development and low market penetration.
The last modeling scenario shows the effect of a shorter time for product development together with an increased market penetration (optimistic). Figure 8 shows the rise in consumers and potential consumers due to the increase in market penetration and decreased time to develop products; this grows the size of the market as a larger number of consumers are obtained. This scenario is highly dependent on consumer retention time and new products developed being highly attractive. Comparing the results from the potential market and consumer base, it can be seen that there was no significant difference with scenario 2. A possible explanation for this might be the change in the viral market effect.
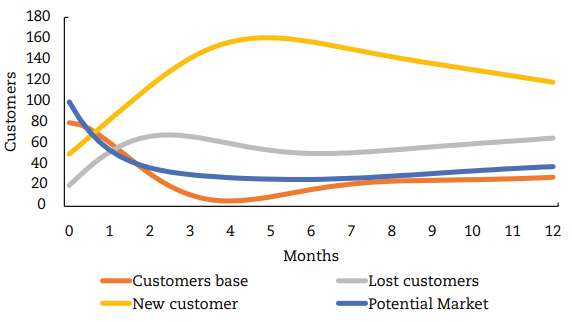
Source: Authors’ own elaboration.
Figure 8 Scenario 4: Shorter time in brand development and increased market penetration.
The following paragraph details a sensitivity analysis that was undertaken for the following parameters: product development time and product’s rate of spreading through viral marketing strategies (WOM).
Sensitivity analysis
The sensitivity analysis for the product disclosure through viral marketing index parameter shows that the number of consumers increases and their tendency to decrease is delayed within a dissemination range of 55% to 90% (inclusive). Within the 55% to 10% dissemination range, consumers decrease faster, and the tendency to recover (steady state) is slower and minimal (illustrated in Figure 9).
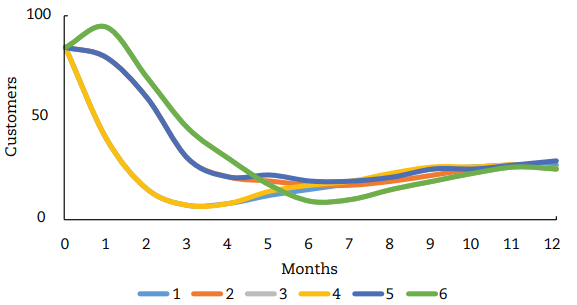
Source: Authors’ own elaboration.
Figure 9 Sensitivity analysis for market penetration index WOM (viral marketing).
In the case of the sensitivity analysis for the product development time parameter, for a shorter time-period (a product development of seven to eight months), the number of potential consumers is lower compared to a greater number of months in which the product is developed (8-9 months). The Figure above shows an increase in consumers who stop purchasing the product due to an increased development time for new innovations and more robust products. Figure 10 shows the behavior of sensitivity analysis.
Discussion
The results show the need for marketing strategies that allow the marketing penetration of a product to be linked to constant and innovative development (Gök and Peker, 2017). This allows the number of consumers to significantly increase, which will allow the number of new potential consumers to be greater. However, the above is only possible if the capacity to develop a product in the shortest possible time is improved. Therefore, parallel strategies with flexible designs must be created, and the production time must be reduced.
It is important to emphasize that viral strategies allow the continued and sustainable dissemination of products that are characterized by low consumption time (less than two months). Furthermore, increased viral strategies are no guarantee of a product developed over a longer period of time having a high impact (Ho and Dempsey, 2010).
Product development time regulates the increase or decrease in potential consumers but generates an effect on consumers who stop buying the product. These, over time, become the product’s target market. This suggests that there are consumer loyalty strategies and that there is a decrease in the amount of time in which the consumer abandons the product.
Based on the former, the usefulness of simulating results lies in analyzing the behavior of consumers who have been exposed to WOM strategies in the agro-food industry. Similarly, the time it takes to develop food products is characterized by the variety and complexity of perishable foods (Orjuela-Castro and Adarme-Jaimes, 2017; Orjuela-Castro et al., 2017).
Conclusions
Modeling by using a simulation methodology of system dynamics allows various scenarios presented in WOM strategies to be analyzed. The complexity of the market and consumer dynamics must be viewed from the point of view of: constant innovative product development, the product’s market penetration, and its dissemination through marketing strategies that enable the customer to become attracted to a product or service (Bianchi, 2016). Despite the system dynamics methodology being suitable for this research, it has some limitations regarding the data collection process for the simulation model and the validation process with decision makers.
The results show that product development time should decrease in order to increase consumer retention and new potential consumers. Therefore, an increase in potential consumers allows innovative products that are constantly being generated to be attracted, which then becomes a proper market strategy. The product development time is an important stage in which effective WOM strategies should be planned.
In terms of attraction strategies and product marketing penetration, it is essential to determine the degree of attraction generated by the consumer (Alvarez-Milán et al., 2018). The results from modeling the simulation using system dynamics show the importance of the contagion degree to attract and retain consumers; this also includes consumers who have left the product and then return to the target market. It is important to analyze the behavior of these consumers as the degree of customer attraction must be intensive and constant over time.
The simulation performed with systems dynamics shows the complex relationships between actors and consumer states; however, the integration of other marketing or engineering techniques may improve the analysis of the relationships that underlie marketing strategies and customer behavior.
The usefulness and relevance of the developed model addresses the changing of consumer states through simulation and allows us to understand the behavior of agribusiness products in the field of new product development, its dissemination, and disclosure. The impact of research will allow the future integration of techniques that demonstrate the impact of WOM strategies as well as innovation and product development time in environmental, social, and economic areas of society.