1. Introduction
The design of more rational and efficient structures under certain objectives is a constant search by designers and researchers, which can be achieved through structural optimization. This process could be didactically divided into 2 cores: the structural part, i.e. structural modelling, analysis and design, and the optimization part: problem formulation, objective function(s), use of optimization methods, and the like. Both are important, because if a good mathematic formulation is not achieved or adequate optimization methods are not selected, the structural results obtained will not be rational, and the whole process loses its essence.
In structural optimization, due to some peculiarities such as the use of discrete variables, for example, the use of meta-heuristic methods to carry out the optimization process is frequent. These methods, within which the evolutionary strategies (EA) are located, present a stochastic behavior, making each process unique, so a number of tests are needed to obtain reliable results related to the performance of the method in a certain problem. These strategies present parameters that regulate their operation, and it is difficult to establish their appropriate values for specific problems. For this reason, it is necessary to make an adjustment of these parameters. This process is named parameter tuning [1,2]. It is often extremely difficult and laborious due to the great computational time consumed, especially when modeling, analysis and structural design software and a super programming language are linked to generate the entire automated procedure using API functions (CSi API SAP2000-MATLAB in this study).
To perform this expensive task, it has been decided to look for functions that simulate the main features of real problems (number of variables, generated response surface, space of admissible solutions, and so on), when it is not necessary to introduce the modeling, analysis and design part. The solutions are evaluated only in an analytical function, and not in a complex process. For this, the use of the benchmarks functions is recommended. After studying the method in this function, it will be applied to a real case study (Fig. 1) with the best operational parameters found.
On the other hand, one of the shortcomings detected in the literature review is the authors' non-inclusion of the soil-structure interaction (SSI) in modeling. For this reason, the analysis of structural results will be focused on the importance of taking into account static-SSI (SSSI), and the differences when it is not introduced. In our investigations, SSSI is integrated using the Winkler hypothesis and the methodology proposed by [3], adapted to our conditions [4,5].
2. Materials and methods
2.1 Mathematical formulation of the optimization problem
The mathematical formulation of the optimization problem is one of the essential steps of the process, where the objective function(s), design variables, constraints, and assigned parameters are defined.
For this case, as the first step is to simulate a real problem of structural optimization, the Ackley function is chosen as the objective function (see Fig. 2), with 14 design variables. Constraints would be only the limits of movement of variables, or explicit constraints. In the case of real problems, there are other constraints to be met, which we call implicit. Some of them are related to the fulfillment of the Limit States (strength and serviceability). Constraints associated with strength criterion are immediately satisfied when the design is performed. The others, when they are not met, the objective function is penalized.
2.2 Objective function
Firstly, the study will be carried out on a function which generates response surfaces with the presence of several local optima, as it is often the case in structural optimization. Ackley function (Fig. 2b) has been adapted to simulate what happens in real cases (Fig. 2a) and it is taken as the study function to perform parameter tuning.
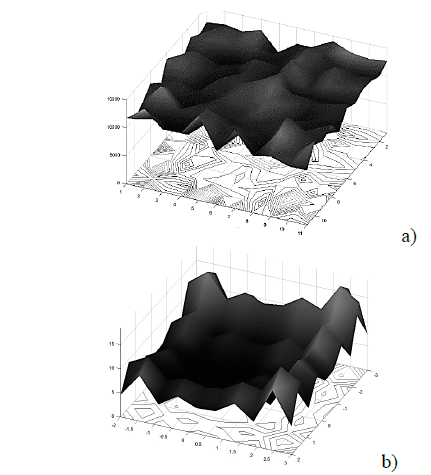
Source: The authors.
Figure 2 Searching for problems similar to those generated in structural optimization, a) response surfaces generated in real problems, b) adaptation of the Ackley function to simulate real problems.
The practical objective function is the total direct cost of the structure (beams, columns and foundations), taking into account the formwork, the elaboration and placement of steel (longitudinal and stirrups) and concrete, and the cost of excavation and refill in foundations [4,5]. For more information, check the previous references.
2.3 Variables
The real case has fourteen discrete variables. For beams and columns, the variables are the dimensions of the cross section. For foundations they are the rectangularity, and for materials the compressive strength of the concrete (f’c). Other characteristics depend on f’c (E, density, etc.). Two geometry groups are established for beams (4 variables), three for columns (6 variables), three for foundations (3 variables) and one variable for the quality of the concrete.
In variables associated with beams’ height, for example, the interval would be defined by L/18≤p≤L/10 (L is the beam span), varying in steps of 0.05 m. For example: for 8m long beams, the possible solutions would be: p=[0.45, 0.50, 0.55,…,0.80].
In the case of the foundations, rectangularity is the variable analyzed, i.e., division of size along "X" axis (L) and size along "Y" axis (B). Therefore, each variable has a different number of possible solutions and influences the objective function in a different order. In addition, its optimal values will not necessarily be located in similar positions within all possible positions. This situation is reflected in the Ackley function using the constraints.
2.4 Constraints
Constraints play a fundamental role, because, although they will only be limited to establish the ranges of movements of the variables (in optimization of the Ackley function), they will allow us to make the function more difficult to optimize. This can be explained as follows: if we set equal movement intervals for the studied function, all the variables would have the same tendency, and the convergence of the algorithm towards the global solution would be faster. Furthermore, each variable would take the same values and its influence on the objective function would be the same. However, if we simulate the optimization of real problems and we set different intervals for each variable, the problem would increase in complexity and its solution would not be so obvious.
From the Ackley function, it is known that its global minimum is, for the interval -8≤Xi≤8, located in Xi=0 (Xi are the design variables), with a value of f(Xi)=0. Knowing this, and with previous results obtained in the optimization of the real structure, it will be matched, in addition to the number of values per interval, the position of the best solution, or optimal value of each variable (Fig. 3).
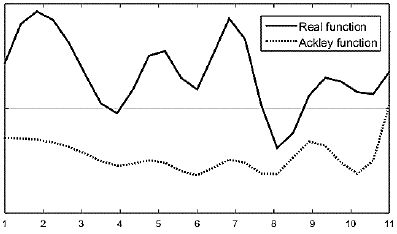
Source: The authors
Figure 3 Using constraints to establish similarities between Ackley and real function. The graphs are cuts made on the response surfaces in Fig. 2. It can be seen how the location of the global optimum is matched.
For example, for the group of beams explained above, the optimal height p(1) turned out to be 0.50 m (in the real case previously studied), so the interval of p(1) for the study function would be -1≤p(1)≤6, and so on in dependence on each variable.
2.5 Evolutionary algorithms
One of the most effective approaches to solve complex optimization problems is the evolutionary one [6,7]. Among the most commonly used methods of this type for solving problems associated with structural optimization we have: Genetic Algorithms (GA) [8-10], Simulated Annealing (SA) [11-13], Particle Swarm Optimization (PSO) [12,14], and others.
The MATLABv15 software was used in the programming and application of these algorithms, specifically the toolbox of evolutionary strategies YPEAv1.0 [15]. To choose from initial 11 evolutionary algorithms, 30 tests were performed for each method on the study function, obtaining average performance curves (APC) or average fitness in function of the iteration number. In Fig. 4, it can be seen that the method with the best behavior was BBO. The other techniques tested were: Artificial Bee Colony (ABC), Bat Algorithm (BA), Covariance Matrix Adaptation Evolution Strategy (CMA-ES), Differential Evolution (DE), Firefly Algorithm (FA), GA, Harmony Search (HS), Imperialist Competitive Algorithm (ICA), PSO and Teaching Learning-Based Optimization (TLBO). The parameters in each method were adjusted according to obtained results, using a simple trial and error method.
2.6 Biogeography-based optimization
BBO is a relatively new method, so its diffusion and application is not very widespread, although some authors have used it for structural optimization [16,17].
Biogeography studies the geographical distribution of biological organisms. Mathematical models of biogeography describe how species migrate from one habitat or island to another, how new species arise, and how species become extinct [18].
For a better understanding of the process it should be clarified that each species represents the value that each variable takes within a possible solution, that is, each habitat has a quantity of species equal to the number of variables. The algorithm consists of the following steps:
1) A random initial set of habitats with a uniform distribution is created. This initial population is sorted according to HSI. Each time a new population is conformed, it is assigned a value of μ and λ, which are the coefficients of emigration and immigration respectively. Solutions or habitats with best HSIs have higher values of μ and lower values of λ, and vice versa.
2) From here the algorithm starts the process with the best habitats, changing according to their parameters μ and λ (migration), as well as the probability of mutation:
Starts from the best habitats to the worst. Within each habitat, it is analyzed from species to species, in case of migration. This process is performed as follows: each species of each habitat is checked based on its λ coefficient, that is, to perform immigration. Therefore, the species of the first habitats have little chance of entering this process, although this possibility increases when considering worse solutions (higher values of λ).
Once a species enters this process, another species is selected to immigrate to the solution being worked on. The selection of this species is based on μ, and the selection process is performed using roulette wheel selection.
With the species selected, immigration starts, which is not the substitution of one by the other, but a combination of both, performed as in eq. (1).
Here:
ActSpec: Species being analyzed.
ImmSpec: Species selected to carry out immigration.
α: The acceleration coefficient (or Alpha), related to the magnitude of the change to be made in the analyzed species.
In addition to this, species can mutate, with a certain probability of mutation represented in eq. (2).
Where:
σ: Mutation step size (0.05 per default).
N(0,1): Random number with 0 mean and standard deviation 1.
After every iteration, σ decreases, modified by mutation step size damping (0.99 per default).
Once the entire population is analyzed, the new population is formed. To do this, a number of the best solutions of the previous population (before being transformed) are chosen and added to the best of the new population. This is similar to elitism used in GA. The number of solutions taken from the previous population is given in eq. (3).
Where:
KeepRate: Coefficient that represents the percentage of the previous population that will enter the new one.
PopSize: Population size.
Thus, the new population is made up of the best previous and new solutions, proceeding to organize the new generated population and continue with the iterative process.
2.7 Parameter tuning
It is widely recognized that the performance of an optimization algorithm can be improved by parameter tuning [19-21], but this task can be difficult to implement.
In general, there are two main performance measures for EAs: one is related to the quality of the obtained solution and the other to the speed of the algorithm. Most performance metrics used in evolutionary computation, if not all, are based on variations and combinations of these two. Solution quality can be naturally expressed by the fitness function the EA is using. As for algorithm speed, execution time or search effort needs to be measured [19,21].
Due to the stochastic nature of EAs, it is necessary to perform a certain number of tests to obtain a good distribution and a good measure of the method’s performance. In this way, we can get some measures allowing us to make a decision about the quality of performance [21]:
These values are also defined as utility, and can be taken in their simple form or a combination of them that allows a better understanding of a method (or its configuration) when facing a given problem. For our study, the utility taken will be the MBF. The APC will also be used [22].
2.8 Experimental design
The experiment is designed based on the proposal of [2]. Parameter tuning is performed by fixing a value of certain parameter, while the others take random values. This proposal offers the possibility of combining each analyzed parameter with all the possible values of the other ones, creating all possible combinations of values and finding the best one. Ten thousand tests will be performed for each parameter value. The parameters analyzed are: PopSize, KeepRate, Alpha and MutationProb. PopSize will take the following values: 20, 25, 30, 35, 40, 50, 75 and 100; KeepRate can be: 0.05, 0.10, 0.15, 0.20, 0.25, 0.30, 0.35 and 0.40; Alpha: 0.60, 0.65, 0.70, 0.75, 0.80, 0.85, 0.90 and 0.95, while MutationProb will take the same values as KeepRate. Once the results on the study function have been obtained, the tuned method will be applied to the real problem, on which this experiment was inspired in order to compare it with the methods used in previous investigations.
2.9 Results and discussion
To be consistent with the proposed experiment, the results will be expressed using the APC of the 10000 tests for each value. Simple box plots are also used, taking into account the maximum and the minimum final values that the method found in the 10000 tests and the MBF as well.
For structural results, two types of soil will be used for the inclusion of SSSI, one predominantly cohesive (soil 1) and the other predominantly frictional (soil 2) (see Table 1).
2.10 Study function
Population size is an essential parameter for any EA. The quality of the results and the consumption of the process depend largely on its value. In the case of BBO, and unlike other methods, it seems to work well with relatively small population sizes.
Fig. 5 shows that, depending on the number of iterations, the efficiency of the values of this parameter varies: for the first iterations, with larger population sizes, better results are obtained. In subsequent iterations, small population sizes ensure better performance, although they have a marked tendency to stay in local optima, so, for final iterations, again large population sizes are better. Finally, the best performing population size turned out to be 75.
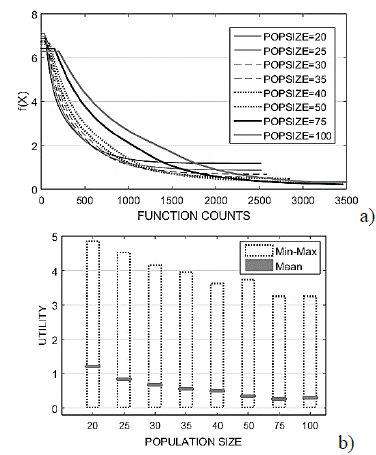
Source: The authors.
Figure 5 Graphical results for PopSize. a) Average performance curve. b) Simple box plots.
According to Fig. 6, the best KeepRate value is 0.40, but it can be verified that this parameter does not have much influence on the method’s behavior.
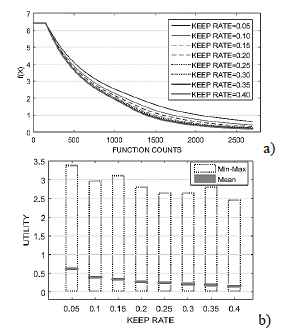
Source: The authors.
Figure 6 Graphical results for KeepRate. a) Average performance curve. b) Simple box plots.
Acceleration coefficient (Alpha) regulates, in some way, the magnitude of the change produced in the immigration process. In Fig. 7, it can be seen how 0.95 ensures much better results than the rest of the values. This parameter is possibly the most influential one in the method’s performance (in addition to PopSize), since even using random values of the others, the global optimum was found on 98.86 % of the tests. It means that, by fixing optimal values of the other parameters, the method could always find the global optimum. This gives the possibility to establish smaller population sizes to ensure faster convergence obtaining good results.
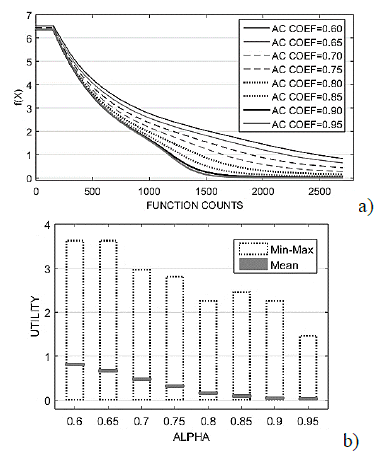
Source: The authors.
Figure 7 Graphical results for Alpha. a) Average performance curve. b) Simple box plots.
In Fig. 8, it can be clearly seen that 0.40 as probability of mutation is the best option, especially for more advanced function counts. This guarantees to give more diversity to the process, and with this, to avoid falling into local optima.
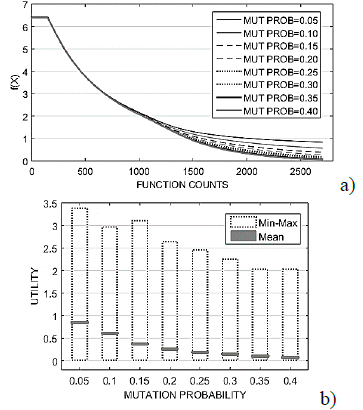
Source: The authors.
Figure 8 Graphical results for MutProb. a) Average performance curve. b) Simple box plots.
It seems, considering the results, that for this particular optimization, the method offers better solutions with parameter values that make it to show a more dynamic behavior, i.e., with many changes between habitats and with a great magnitude of these changes.
In general, with the resolution of this experiment certain conclusions can be drawn regarding the use of BBO in discrete structural optimization. One has previously mentioned, and it is the dynamism of the method, and the need to establish values of its parameters which increase that dynamism. On the other hand, one of the essential objectives of this type of optimization is to ensure a fast convergence of the method ensuring good solutions. It is due to how extensive these processes could be, and BBO seems to be excellent for this because it performs well with relatively small population sizes. In addition, KeepRate does not have high influence on the method’s performance.
2.11 Application to the real case study
The case study has previously been analyzed in other investigations [4] (Fig. 1). It has 14 discrete variables and 1.5 x 1010 possible solutions.
The results of the real case study optimization process (with soil 1) using BBO with its tuned parameters is compared to simple GA and simple PSO, just as had previously been used in [4]. In Figure 9 it can be seen the superiority of BBO, and the dynamism mentioned above because this method constantly improves its solutions.
2.12 Structural results
Structural results will be expressed in span/height (L/h) and reinforcement ratios (ρ) in the case of beams, and rectangularity (division of the dimensions in the direction of “x” and “y” axis, in that order) for columns and foundations. As mentioned, this is about comparing the use of SSSI in modeling in general and optimization in particular, due to it is a factor that significantly increases the cost of the optimization process, but it has been proved that the results can be quite significant when it is not included.
Table 2 summarizes the most important results for each type of element. In the case of the beams, it can be seen how by including the SSSI, they become much more requested elements from the point of view of design forces. For predominantly cohesive soil (soil 1), the same dimensions are obtained as when SSSI is not included. The differences lie in ρ, with increments of up to 0.59 % in the case of the top reinforcement of group 2.
Table 2 Summary of the most significant structural results for real case study
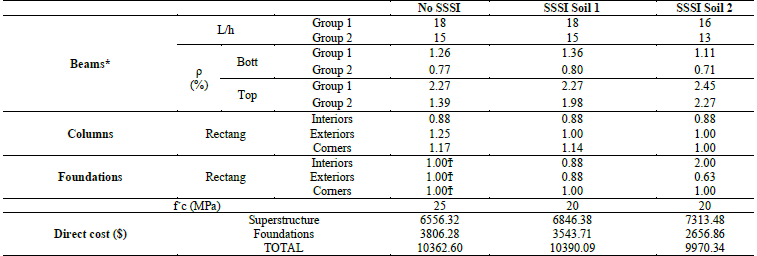
* Two most representative design groups are represented
Ϯ Foundations are not taken into account in modeling (no SSSI) but they are, at the end of the optimization process, designed, and their cost is calculated
For the predominantly frictional soil (soil 2), differences are much more significant, since they also increase the optimal heights (L/h ratio decreases), and even then, there is an increase in ρ, reaching changes of 0.29 %. In general, when SSSI is not included, the superstructure is 4.42 % less expensive than the case of the soil 1 and 11.55 % than the case of the soil 2. This does not mean that by not including this aspect a more rational design of the superstructure is obtained, but that is not safe enough, with differences of more than 10 %, measured by the direct cost. These structures designed without including some aspects in modeling (such as SSSI) are prone to rapid deterioration and the consequent need for extra maintenance, significantly increasing the cost during their life cycle.
Previous results can be explained by the influence of the analyzed soils properties. For predominantly cohesive, with frequently less bearing capacity than frictional ones, during foundation geotechnical design in first Limit State (bearing capacity), larger dimensions are obtained in relation to the seconds. This situation determines that soil behavior under foundation in predominantly cohesive soils stays in the linear zone (curve 1-2 in Fig. 10), for load combinations during second Limit State check (settlement). In predominantly frictional soils, the behavior generally remains in the nonlinear zone (curve 2-3 in Fig. 10), thus causing, even for similar loads in similar foundation designs, significant differences in settlements. These differential settlements, even in the admissible range, cause a redistribution of design forces in the superstructure. The beams are the most affected elements, especially in the supports zone (top reinforcement). These settlements do not exist in classic supports (fixed or pinned).
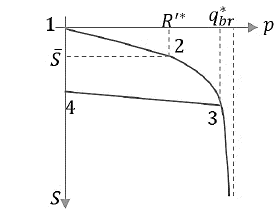
Source: The authors.
Figure 10 Pressure-Settlement (p-S) soil behavior curve under foundation: q * br , design soil bearing capacity as pressure; R'*, design limit pressure for linear behavior; s̅ settlement for p=R'*; 1-2, linear behavior during loading; 2-3, non-linear behavior during loading; 3-4, behavior during unloading.
For the analysis of columns and foundations, essential aspects must be taken into consideration. Lateral loads are resisted by sway frames and not by shear walls, so the columns will be the main responsible for providing the horizontal stiffness of the structure. On the other hand, "x" axis is the critical direction to bending due to gravitational loads, while for horizontal loads (wind), it is "y" axis, so, the most critical global direction to bending is not an element of obvious appreciation.
In the case of the interior columns, which do not present bending due to gravitational loads (symmetrical floor plan, in geometry and loads), they have their largest dimension in the “x” direction, supporting the stiffness of the structure facing the wind load. The other groups are either square (there is no predominance of bending in any direction) or rectangular supporting the critical direction facing the gravitational loads.
Regarding foundations, most are either square or rectangular supporting the critical wind direction. There is the case of the interior foundations in the soil 2, whose bigger dimension is in the “x” axis, since no other element prioritizes stiffness in that direction, which also presents a considerable area for wind load.
For the quality of the concrete, it is important to analyze that, generally, elements that work at bending fundamentally do not need high f´c values, the opposite for elements that work with a predominance of axial force. When SSSI is not included in the optimization process (the foundations are neither modeled nor taken into account in the total cost), the best global value turns out to be 25 MPa. Only beams and columns are included here, being these opposing elements groups the ones which face different types of forces. When SSSI is included, another group of elements that mainly work at flexion, as foundations, is introduced into the process, in such a way that the global result is to use concrete of 20 MPa for the entire structure.
3. Conclusions
As part of a strategy to reduce the cost of the parameter tuning process in the optimization of reinforced concrete structures, the Ackley function was adapted to simulate real problems, and it was taken as a study function. In optimization tests on this function using eleven EAs, BBO was proved to be the most effective strategy. After tuning four parameters of this method, it was found that BBO is very appropriate to face these problems (discrete optimization), characterized by great dynamism in its behavior. The tuned method obtained the following configuration: PopSize=75, KeepRate=0.40, Alpha=0.95 y MutProb=0.40.
From the structural point of view, it was found that the inclusion of SSSI, an aspect usually disregarded by researchers and designers, provides significant results in respect to when it is ignored. The difference is smaller in cohesive soils than in frictional ones. For the former, the difference obtained in direct costs of the superstructure is equal to 4.42 %. For the latter, this difference rises to 11.55 %. In the case of beams, L/h ratios around 15 are recommended, with ρ values up to 2.45 %. Columns and foundations get their most rational configuration with rectangularity of up to 1.15 for the firsts, and 2.00 for the seconds. In columns, this rectangularity is generally conditioned by the predominance of bending in one direction over the other due to gravitational loads. In foundations, what generally determines the rectangularity is the critical direction of the wind load.