INTRODUCTION
Soil moisture is highly variable over time and space, which is known as spatio-temporal variability. This variability exerts a strong influence on the development of vegetation cover, which is crucial when analyzing all related processes in the soil-plant-atmosphere interaction (Zhu et al., 2019).
According to Pan et al. (2008), variability in soil moisture is influenced by rain seasonality; in rainy months (PPT>ET), topography and vegetation are the main factors, and, in less rainy months (PPT<ET), soil texture has the greatest influence. Jia and Shao (2013) found that the vegetation factor has the highest correlation with variability in soil moisture. Joshi and Mohanty (2010) showed that soil texture and topography are highly related to variability in soil surface moisture.
Colombia is a very diverse territory, influenced by two oceans, the Pacific and the Atlantic. In addition, the Amazon contributes large masses of moisture. These factors, along with the territorial movement of the Intertropical Convergence Zone (ITCZ) and its interaction with the topography of the Andes mountain range, result in highly diverse mesoclimates and microclimates (Jaramillo and Chaves, 2000). This situation is responsible for seasonal variations in precipitation and the general climate, with direct influence on soil moisture on different time scales. In summary, soil moisture results from the interaction of precipitation (intensity and frequency), soil cover conditions, slope and physical properties of the soil, mainly texture and porosity (Zhang et al., 2014).
Precipitation, both frequency and intensity, temperature and wind speed are climatic variables that are associated with the temporal dynamics of soil moisture and its resulting flows (Hong et al., 2021).
Precipitation is the most important climatic force for soil moisture content and its seasonality, which depends on topography and land cover, generating surface runoff that can strongly influence soil moisture (Huang et al., 2013).
The persistence of soil moisture results in autocorrelation over time that can be used to quantify the temporal variable memory. Persistence is important because soil moisture is related to precipitation, where air temperature oscillations are a robust signal for predicting precipitation (Yang and Wang, 2019).
Beljaars et al. (1996) found that soil moisture varies slowly in relation to atmospheric elements, with variations that persist for several weeks to several months in deep soil layers. Moisture content influences current climatic conditions and those for coming months because of its high persistence.
On the other hand, soil slope and physical factors, such as texture and vegetation cover, influence the rate of water infiltration through a soil profile. Huang et al. (2013) pointed out that increases in rainfall intensity do not necessarily lead to greater water infiltration into the soil and may even, depending on the aforementioned factors, reduce water infiltration.
Slope (slant degree) has two defined behaviors with respect to moisture response. Firstly, an increase in the slope percentage decreases the infiltration rate of water in the soil, which can increase surface runoff. Secondly, the effective precipitation (rainwater that infiltrates the soil profile) decreases as slope increases (Assouline and Ben-Hur, 2006; Mu et al., 2015).
Likewise, vegetation cover is key since it influences the exchange of energy and water although little attention has been paid to this relationship. Assessing the effects of soil cover and use patterns on soil moisture is difficult because each soil cover and use changes soil properties in terms of evapotranspiration and interception of rainfall, causing variability in soil moisture conditions and intervening in other processes, such as infiltration and runoff (Fu et al., 2003; Chen et al., 2009; Cleves et al 2016; Liu et al., 2020).
Vegetation type and canopy density and uniformity contribute to variations in soil moisture on different spatial and temporal scales. The influence on soil moisture is more dynamic than topographic factors. Soil moisture variability is lower with a full canopy cover and higher with a partial vegetation cover (Gaur and Mohanty, 2013).
The heterogeneity of soil affects the distribution of moisture in the soil profile because of physical factors such as variations in texture, organic matter content, structure and porosity. Each of these physical factors affects the dynamics of water transmission and retention. Inherent conditions in the formation of soils can present significant variations in moisture over short distances with variations in porosity and soil structure, which influence the flow of water through a profile, with a significant effect on the pattern of moisture distribution (Martínez-Fernández, 2021).
The main objective of this study was to characterization soil moisture dynamics under the topographies, physical soil conditions, vegetation cover and climatic classifications of Colombia,focusing on agricultural regions (UPRA, 2017). The reference included data from automatic data stations in the national network administered by IDEAM, with around 20 years of records, from 2001 to 2020.
MATERIALS AND METHODS
Study area and data: The study area included the Colombian Continental territory, with a surface area of 1,142,748 km2; specifically, the area of the agricultural frontier defined by Unidad de Planificación Rural Agropecuaria of Colombia (UPRA in Spanish), with altitudes from sea level to 3,500 m, was used.
The hourly meteorological data (2001-2020) used in this study were obtained from the agrometeorological network of the Instituto de Hidrología, Meteorología y Estudios Ambientales (IDEAM in Spanish), with 105 stations distributed in agricultural areas with soil moisture sensors at 10, 30 and 50 cm deeps. The meteorological variables considered were mean temperature (°C), accumulated rain (mm), days with rain (number days), hours with rain (number hours), soil temperature (°C), sunny hours (hours) and relative humidity (%).
The physical conditions of the soil were extracted from general studies on soils by department, at a scale of 1: 100,000 (IGAC, 2014), and the information on vegetation cover was obtained from the Land Cover Map, at a scale of 1: 100,000, prepared by IDEAM and IGAC, developed with the Corine Land Cover methodology (IDEAM, 2015).
Homogeneity tests:Before carrying out a study on the seasonal behavior of soil moisture, it must be ensured that changes observed in the series are derived from natural dynamics in the variable and its interaction with atmospheric and physical conditions.
A series may cease to be homogeneous if the measuring station undergoes changes in instruments, site conditions, or recording system or observer (Saladié et al., 2004). Soil moisture is sensitive to these changes. Therefore, a technique that verifies this hypothesis is needed.
The homogeneity of the soil moisture daily series for the three (3) depths was verified with the SNHT test (Standard Normal Homogeneity Test), using the single station test or absolute test, which uses the data from the same station since soil moisture is highly influenced by physical variables and location. The network of stations is not dense enough to have neighboring stations that share characteristics. According to Haimberger (2007), for recording periods less than five (5) years, the seasonal cycle of the variable must be considered, and daily data should be used instead of monthly data. Therefore, the analysis used daily data.
The SNHT test calculates the differences between two groups of data, comparing the means in the series of differences before and after a potential break point. The point that divides the sample into two parts varies, but the time interval remains fixed. Haimberger (2007) detected two drawbacks: 1) the method tends to indicate breaks near the edges of the time interval, and 2) the position of a break point is poorly estimated in the presence of a periodic signal. The author modified the algorithm for a more reliable estimate of annual variability using daily data, allowing the use of missing data and guaranteeing sampling of annual seasonality before and after potential breakpoints.
For this study, since the precipitation seasonality and its effect on soil moisture were known, a period of 350 d was analyzed to detect ruptures using the statistical software R and the package "SNHT", developed by Browning under the Robust rupture detection configuration (Browning and Schneider, 2017), as indicated in figure 1.
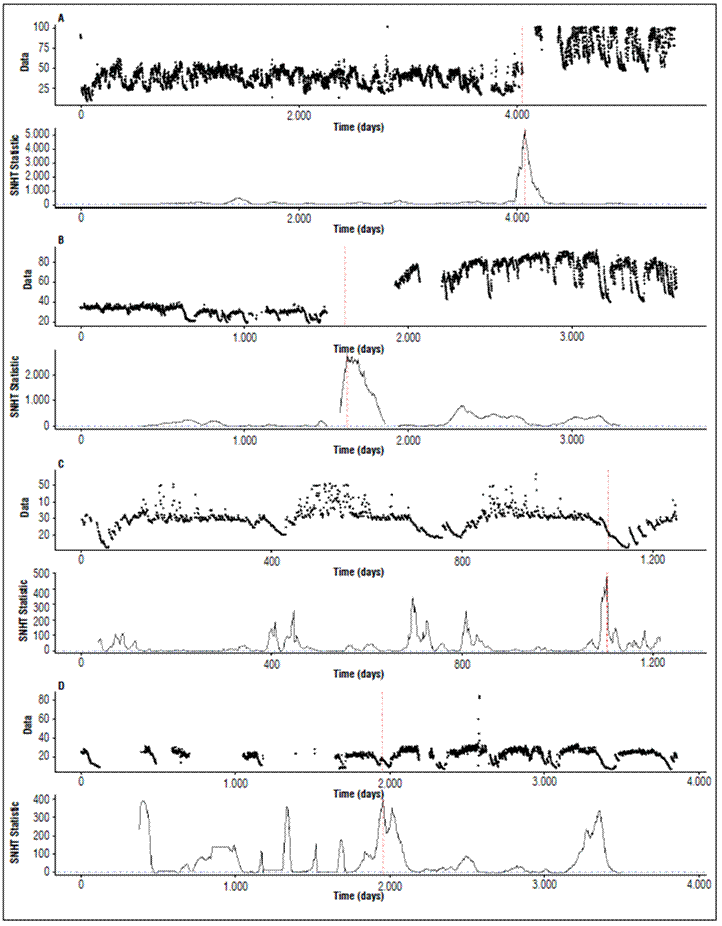
Figure 1. Examples of SNHT homogeneity tests for some of the IDEAM Network Stations, the higher the SNHT score, the greater the difference between means.
Correlation analysis of soil moisture: The variable soil surface moisture, at a depth of 10 cm, was correlated with the atmospheric variables (accumulated precipitation, hours with rain, mean temperature, maximum temperature, and minimum temperature) and soil temperature on different time scales (daily, decadal and monthly).
To analyze the temporal influence of atmospheric variables during the period of available soil moisture data, the non-parametric rank correlation test proposed by Spearman (1904) was used, which evaluates the intensity of the relationship between two variables. This coefficient varies between -1 to 1; the closer it is to -1, the stronger the inversely proportional relationship, and the closer it is to 1, the greater the directly proportional relationship. Values close to 0 indicate little or no relationship.
Soil moisture is a fundamental variable in the soil-plant-atmosphere interaction and is influenced both by atmospheric variables and physical, topographic and plant cover variables. To determine the influence of atmospheric variables on the temporal dynamics of moisture, dispersion graphs were made between the soil moisture and other variables with data availability for the three aggregated time scales: a) daily, b) decades (periods of 10 d) and c) monthly.
Persistence analysis: In order to determine the persistence of this variable over time, an autocorrelation analysis quantified the temporal variation of soil moisture at different depths, determining the degree of influence of the physical conditions and station location on the soil moisture memory. Therefore, the evaluation of the soil moisture persistence grouped the physical variables and positioning of the meteorological stations, calculating the median and indicating the influence of physical conditions on the temporal persistence of this variable.
RESULTS AND DISCUSSION
Seasonal analysis of soil moisture: Figure 2 shows the seasonal behavior of soil moisture during the observation period 2001-2020, according to data from the IDEAM network.
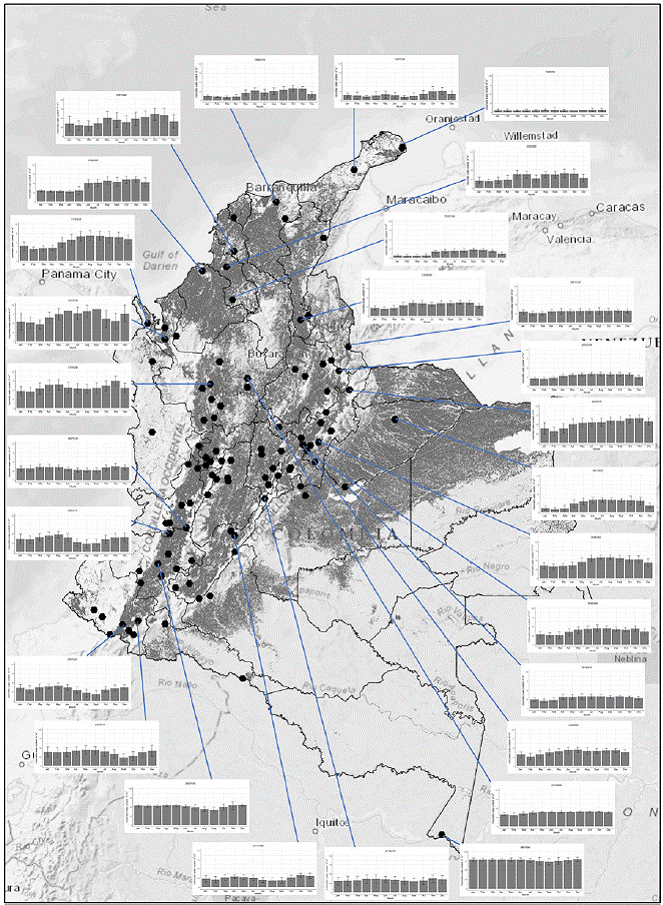
Figure 2. Seasonal behavior of soil moisture (2001 - 2020), the shaded region is the agricultural frontier, established by UPRA. Source: Own elaboration based on data from the IDEAM station network.
The Toromana station (12.08352 N, -71.21094 W), located in Uribia, Department of La Guajira, registered the lowest moisture content in the soil, with mean values of 6-7%. This station is located on the north coast of Colombia, on the border with Venezuela, and is characterized as an arid, desert area, where rainfall is very scarce, with an annual accumulation of 291 mm.The station with the highest moisture content was Puerto Nariño (-3.780305 N, -70.362638 W), located in the municipality of Puerto Nariño in the Department of Amazonas, with an mean annual moisture value between 73-80% and 3,048 mm of accumulated rainfall annual.
The Colombian Pacific region is among the rainiest areas of the planet. Moisture in this region ranges between 32% for the less rainy months (January-March) and 65% soil moisture for the more rainy months (May-August) in the northern zone (Unguia-Choco), with annual rainfall of 2,711 mm.
The southern zone sees 43% moisture in the less rainy months (June-August) and up to 55% for the more rainy months (October-December) in El Tambo (Cauca), with annual rainfall of 4,410 mm. The results for the soil moisture variability in Colombia agrees with those reported by Hoyos and Rodríguez (2020), who indicated that the climate of Colombia is complex, given the heterogeneity of its relief, where, in an area of a little more than 1,100.000 km2, there are diverse climate classifications, ranging from very rainy areas to very arid areas, warm lowlands to cold mountainous areas, and coasts with strong winds and interior areas with calm winds.
Homogeneity test of soil moisture series: Inhomogeneities were identified with a significance level of 0.1 for the depth closest to the surface (10 cm); 83.3% of the moisture series were homogeneous series. With a significance level of 0.05 for the depth of 10 cm, 73% of the series were homogeneous, and, with a significance level of 0.01, 37.1% were homogeneous. This trend was similar for the three (3) depths (Tab. 1). Farsi and Mahjouri (2019) and Vazifehkhah and Kahya (2019) suggested a homogeneous series with the SNHT test and a significance of 0.05 in monthly and annual groupings with series greater than 30 years of records. The test was carried out with series at a daily level with a period close to 20 years, so a significance of 0.1 was adequate for analyzing the dynamics of the variable.
Table 1. SNHT homogeneity test of soil moisture series.
Depth | P<0.1 | P<0.05 | P<0.01 |
---|---|---|---|
10 cm | 83.3 % | 73.3 % | 37.1 % |
30 cm | 75.8 % | 66.3 % | 36.8 % |
50 cm | 68.3 % | 60.0 % | 38.3 % |
Influence of atmospheric variables on the temporal dynamics of soil moisture
Precipitation has a positive relationship with soil moisture, both in intensity and frequency of rainfall events (Fig. 3). This means that the greater the number of rainfall events and intensity, the higher the surface moisture content of the soil will be. This ratio tends to be higher when applied to decades or months. Since the soil moistening process requires time that depends on physical conditions and vegetation cover that, in turn, influence the rate of this process, a lag may be seen between a precipitation event and a sensor's response to an increase in soil moisture, as reported by Zhao et al. (2020), Letian et al. (2012) and Zhang et al. (2009).
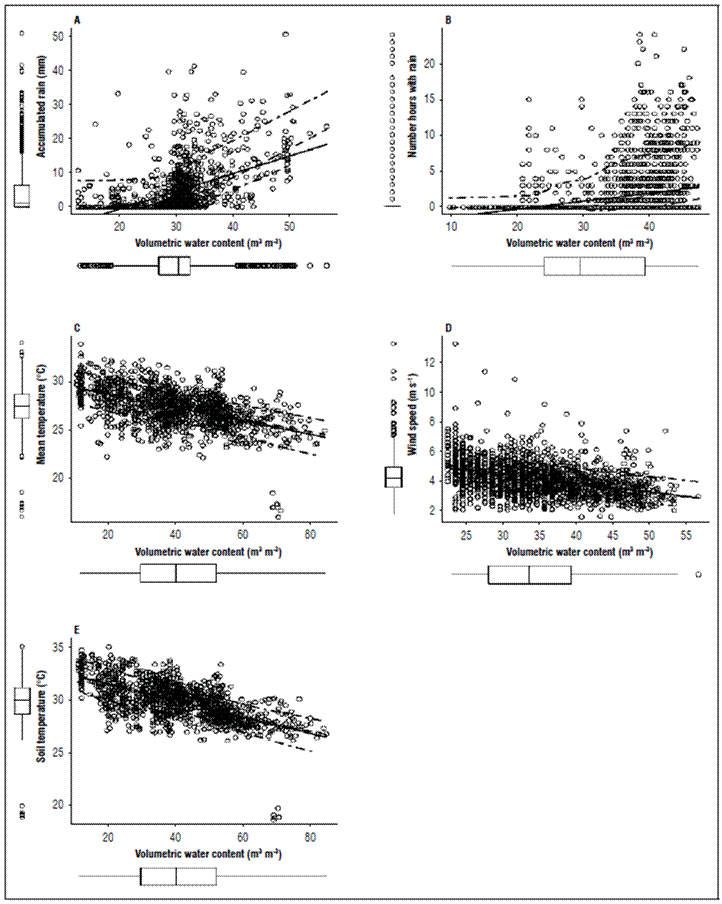
Figure 3. Soil moisture dispersion vs. atmospheric variables. A, precipitation; B, hours rain; C, temperature air; D, velocity wind; E, temperature soil.
Soil moisture has a negative relationship with the variables of air and soil temperature, as well as with the magnitude of wind speed, indicating that, when these variables increase, there is a decrease in the moisture content in the closest profile. On the surface, this relationship is more evident over the course of days and months; Radha et al. (2017) recorded a strong influence from atmospheric variables, such as evaporation, temperature and rainfall, on soil moisture temporal dynamics, results that confirm the relationships observed in this study.
Studies carried out by Cao et al. (2017) in mid-latitudes found that increases in temperature are related to increases in soil moisture conditions because the soil thawing process generates a greater response to moisture as the result of an increase in the upper electrical conductivity of liquid water with respect to water in the solid state (permafrost). In Colombian agricultural regions, soil does not freeze, and temperature tends to be quasi-stable throughout the year. In dry months, temperature tends to increase very little, favoring the evaporation process with vegetation cover that negatively affects the moisture content of soils (Hoyos and Rodríguez, 2020).
Analysis of the soil moisture persistence: Soil moisture has persistence at different depths of more than 80 d. The depth with the lowest persistence is closest to the surface. When analyzing the effect of soil texture on the persistence of moisture at 10 cm, it was observed that the coarse texture registered the lowest persistence, between 85-95 d on average, indicating that this texture favors the passage of water through the soil profile. This behavior was also evidenced at the depth of 30 cm, showing the lowest persistence with about 150 d. For the depth of 50 cm, there was no loss in persistence as a function of time for this variable on average in the annual cycle (Fig. 4A).
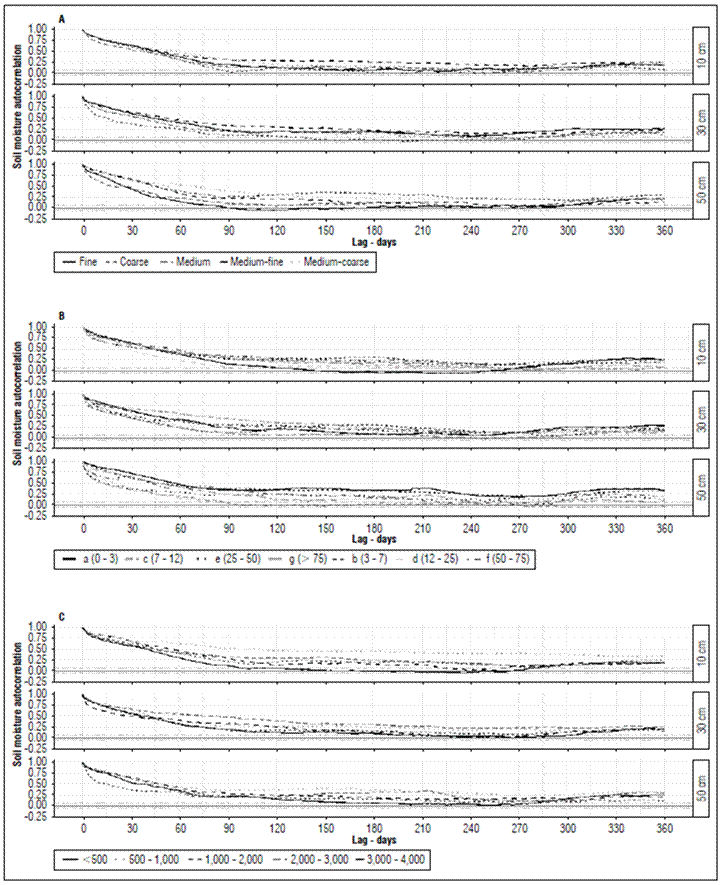
Figure 4. Temporal autocorrelation of soil moisture with physical conditions of the stations, IDEAM Network. A, soil texture; B, terrain slope; C, altitude (m a.s.l.); D, vegetation cover; E, climate classification.
These results suggest that water that tables close to the surface in humid climates (regions with frequent rainfall during most of the year) or bodies of water close to the sensor can influence it. The soil texture with the greatest persistence was the medium-fine texture, with more than 300 d. This behavior was evidenced at the depths 10 and 30 cm.
The influence of slope on persistence of the soil moisture variable in the first 10 cm of depth was seen in 12-25% slopes with about 90 d, followed by 0-3% slopes with 120 d. At 30 cm, the slopes with lower persistence were 3-7% and >75% (Fig. 4B).
The influence of altitude on persistence (Fig. 4C): Stations located below 500 m a.s.l. registered lower persistence, with about 100 d on average. At 30 cm, the persistence of this variable increased in the stations located below 500 m a.s.l., with 240 d.
The results differed from expectations based on the literature (Huang et al., 2013) since the highest correlations were observed with low slopes, where there is little runoff and rainfall infiltration is favored, but condition dynamics in Colombia, its location in the ITCZ, the interaction between trade winds and mountain ranges result in increased rainfall that increases with altitude, causing more frequent rainfall in highlands with steep slopes. For this reason, higher correlations were recorded with higher slopes (Jaramillo and Chaves, 2000).
The vegetation cover exerted a direct influence on soil moisture with the evapotranspiration process. At 10 cm, the persistence of moisture in bare soil or with very little vegetation was 55 d (Fig. 4D), followed by forests and forest plantations with a soil moisture persistence of 140 days on average.
This may have been due to the high capacity for extracting moisture from soils in forests. At a depth of 30 cm, secondary vegetation, forests and forest plantations registered lower persistence, with 80 and 200 d, respectively.
The greatest meteorological force on soil moisture was exerted by precipitation, both in intensity and frequency of rain events. The persistence analysis with climatic classification showed that dry climatic conditions or lower contributions of precipitation presented lower persistence of this variable (Fig. 4E). Similar results were reported by Martínez-Fernández et al. (2021), who stated that coarse textures lower the memory of precipitation events on the ground, with less variability over time.
Seasonal variability of soil surface moisture. Figure 5 shows the decadal and monthly behavior of soil moisture at 10 cm with the weather conditions. A marked relationship was evidenced with all atmospheric variables, indicating that atmospheric conditions are related to each other, with a positive relationship between soil moisture and accumulated precipitation variables, number of days with rain and air humidity. A negative relationship existed for the number of hours of sunlight and the mean temperature.
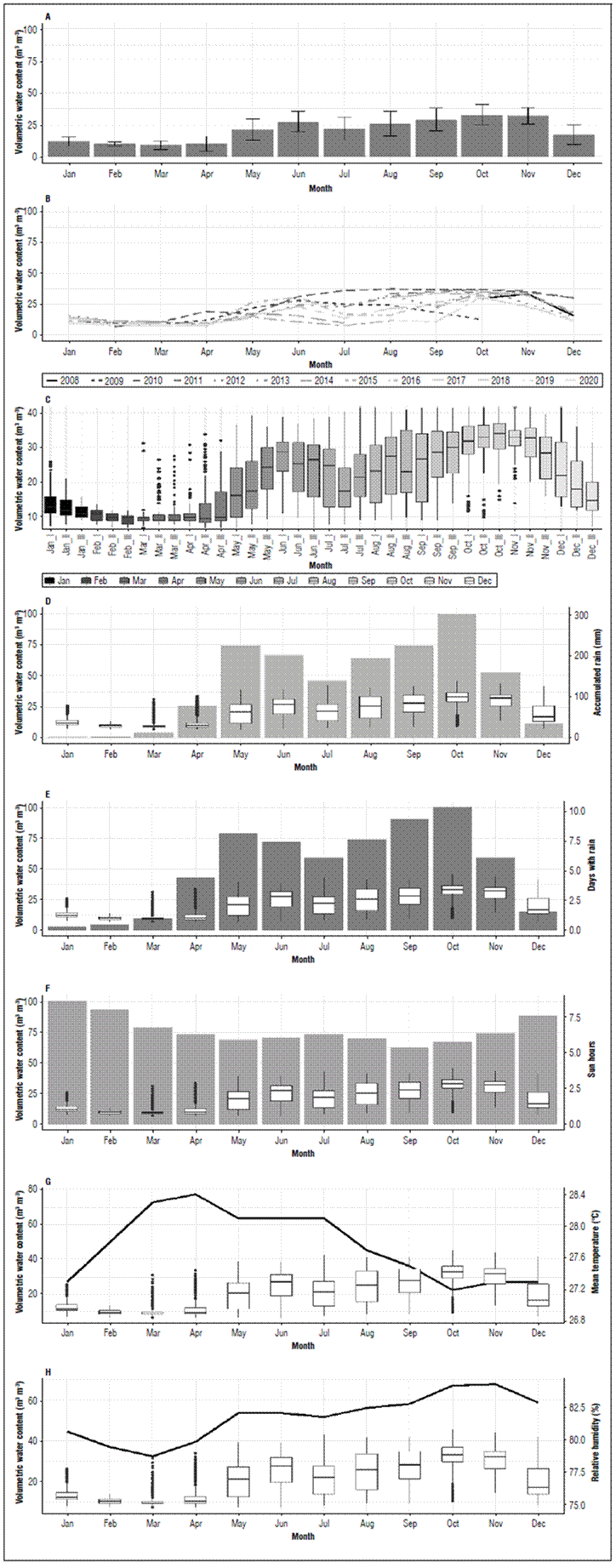
Figure 5. Relationship between atmospheric variables with soil moisture. A, monthly variability of soil moisture; B, monthly soil moisture; C, decadal variability of soil moisture; D, soil moisture vs. monthly accumulated precipitation; E, soil moisture vs. days with rain; F, soil moisture vs. sun hours; G, soil moisture vs. mean temperature; H, soil moisture vs. relative humidity.
Soil moisture presented a high interannual variability with a high response to precipitation in the years when an anomalous rainfall was recorded, as evidenced in the soil moisture. When performing the analysis with historical decades, the greatest variability was recorded for the rainy months, which did not happen for the less rainy months, where the variability of soil moisture was low.
The minimum values of soil moisture tended to be very constant between years. The moisture for the less rainy months decreased to a certain value and stabilized, a value that was reached every year regardless of the intensity of rainfall, suggesting that the moisture storage capacity in the soil was limited not only by the absence of precipitation but also by the physical condition of the soil, which does not facilitate continued moisture losses or requires a lot of energy and time to achieve further decreases in the soil moisture content because of the inverse logarithmic behavior of this variable. These results differ from the results described by Yetbarek et al. (2020), who found that, in agricultural regions, soil moisture dynamics are influenced to a greater extent by the physical conditions of a soil and vegetation cover than by weather conditions.
Climate classification analysis and results: The Spearman correlation analysis of the positive correlation values indicated directly proportional relationships, and negative values indicated inversely proportional relationships between the soil moisture variable and the other atmospheric variables. The climatic classification showed that, for the precipitation variable, the highest rs correlation (P<0.05) occurred in the number of hours with rain, 0.31, as compared to the accumulated precipitation with 0.28. The atmospheric conditions with the highest correlations between the hours with rain and the soil surface moisture included the humid classifications with 0.31 and 0.30 for the dry classifications,respectively. For the time scale, the highest correlation occurred at the month level, with 0.34, followed by daily with 0.33 and decadal with 0.25.
For the relationship with air temperature, the highest correlation was recorded for the group of days, with 0.29, followed by monthly, with 0.28. For the climatic classifications, the warm groups registered the highest correlation, with 0.32, followed by the temperate classifications, with 0.33. The lowest correlation was registered for the cold climatic classifications, with 0.24.
The influence of mean wind speed on soil moisture was greater for the dry climatic classifications, with a correlation of 0.34, and the humid climatic classifications had 0.24, indicating that wind speed contributed to the drying process of the soil, with greater influence in areas with a dry or semi-dry climate.
For the analysis period, the highest relationship was in the monthly grouping, at an rs of 0.32, suggesting that the wind in regions with low volumes of precipitation, higher temperatures and high wind speeds increased the loss of moisture in the surface profile of the soil with greater intensity.
Soil temperature presented a negative correlation with soil moisture at the same depth, resulting in a higher correlation for dry climates, with 0.20; the humid climates had 0.16. For the analysis period, the highest correlation was registered with the daily data, with 0.28, with a decadal value of 0.22.
This high daily relationship was due to the proximity of the moisture and temperature sensors since they were at the same depth in the soil profile, which was not observed in the aggregations of decades because of the high volatility of moisture values, reaching the saturation point of the soil when precipitation events were registered. The temperature saw little variation in this period because of the nature of this variable.
Influence of physical conditions of soil, topography and vegetation cover
Soil texture. The highest correlation according to the textural classification was registered for the number of hours with rain for the monthly analysis period, with rs = 0.36. For the decade level, it was 0.35.
For the accumulated precipitation, the highest correlation was evidenced for the data grouped at the monthly level, with 0.33. A correlation of 0.42 was observed between the number of hours with rain and the coarse and medium-coarse texture. 0.29 was recorded for fine and medium fine textures, and 0.34 was seen for medium textures.
The results indicated that, with thicker textures, a greater response is registered for the number of hours with rain in the increased moisture. For accumulated precipitation (daily, decadal and monthly), the fine and medium-fine textures had a correlation of 0.28, the medium textures had 0.29, and the medium-coarse and coarse textures had a correlation of 0.41.
This behavior suggests that, for an increase in soil moisture, rather than accumulated precipitation, the most relevant factor is exposure time of the soil to the event. The greater the exposure is, the greater the amount of moisture that can infiltrate the soil profile is.
With thicker textures, the infiltration process is faster, and a direct response of precipitation is generated in the soil moisture content, which does not happen with fine textures because the ratio is lower on a daily scale. Water takes longer to infiltrate fine textures because of the reduced space between soil particles and the surface tension of the water. Similar results were reported by Martínez-Fernández et al. (2021), who elucidated the role that soil texture plays in soil moisture dynamics, showing an inverse relationship between coarse textures and soil moisture content.
Ground slope. When performing the correlation analysis between the soil moisture condition and precipitation typified by slope ranges, the results indicated that slopes less than 7% had a correlation of 0.21 with accumulated precipitation for daily aggregation, 0.35 for decades, and 0.34 for monthly aggregation.
The mean slopes between 7-50% registered a correlation of 0.21 for daily data, 0.28 for decades, and 0.22 for monthly data. For steep slopes >50%, the correlations were 0.26 for daily data, 0.29 for data grouped by days, and 0.30 for monthly data. With low slopes <7%, the lowest correlation was with the number of hours with rain for the daily period, 0.21, which was 0.30 for decades and months. The higher correlations were recorded with steep slopes >50%, where the correlation reached 0.26 for the daily scale, 0.35 for decades and 0.36 for the monthly data.
Altitude: Depending on the altitude of the station, the correlation with the accumulated precipitation was 0.37 for decades and 0.44 for the monthly aggregation in stations below 500 m a.s.l. The correlations tended to decrease with height. Stations between 500-1,000 m asl presented correlations of 0.32 for decades and 0.21 for the monthly data.
The correlations continued to decrease as a function of height, with the exception of stations located above 3000 m a.s.l., where there was an increase in correlations with accumulated precipitation, 0.33 for data aggregated in decades and months.
When analyzing the frequency of rainfall through the number of hours with rain and its relationship with altitude, stations <500 m a.s.l. in the decadal grouping presented a correlation of 0.44, with 0.52 in the monthly aggregation. Stations between 1,000 - 2,000 m asl recorded 0.38 on the decadal scale and 0.34 on the monthly scale.
The correlation increased to 0.36 in the decadal aggregation and 0.42 at the monthly level for stations between 3,000-4,000 m a.s.l. These results differed from those found by Huang et al. (2013), who reported higher correlations with low slopes. However, for Colombian conditions, the results are similar to those found by Jaramillo and Chaves (2000) where Colombian topography, the influence of the ITCZ, and other regional and local phenomena cause slope to modulate the regime, distribution and amount of rainfall in an area.
Vegetation cover: Higher correlations between soil moisture and accumulated precipitation were evidenced for the covers: crops-pastures, mosaic-pastures-crops and secondary vegetation, with correlations for the decadal aggregation of 0.37, 0.31 and 0.33, respectively.
For the monthly aggregation, the correlations with the accumulated precipitation were 0.34, 0.33 and 0.39, suggesting that medium size covers, crops and secondary vegetation had a greater response to accumulated precipitation. The bare soils (without coverage) and urban areas presented lower responses to precipitation, with correlation indices lower than 0.19.
The correlation between soil moisture and number of hours of rain had an increasing relationship as a function of plant cover. This relationship was lower with bare soils (rs = 0.08) on the monthly scale.
The results indicated that soil moisture increases progressively as a function of the development of cover, passing through pastures and crops until reaching a correlation of 0.49 for secondary vegetation. The higher the degree of cover and plant development is, the greater the relationship between the frequency of precipitation and its response to soil moisture is. Other approaches to the relationship between soil moisture and plant cover can be seen in the study by Wyattet al. (2021), who evidenced a relationship between the soil moisture condition and the type of cover, suggesting that, in grasslands, where most sensors are located, the soil moisture condition differs depending on the density of the vegetation cover in very short distances, where the change in conditions is attributed to a greater extent to the development of the vegetation cover.
CONCLUSIONS
The spatial distribution of soil moisture is highly heterogeneous because of the heterogeneity of rainfall distribution in Colombia and physiographic factors.
The soil moisture content was determined mainly by the texture of the soil, where medium and medium-coarse textures registered higher percentages of volumetric moisture, possibly because of the higher ratio of meso and macropores, which can fill up during constant rainfall, causing high soil moisture.
Variables such as soil texture, slope, altitude and vegetation cover are modulators of soil moisture dynamics that influence the persistence of this variable on different time scales, from 55 days to more than 360 days. The greatest atmospheric force on the soil moisture content at a depth of 10 cm was precipitation although it was not the only variable that modulated this content.
The soil moisture memory was high, and the physical, topographic and vegetation cover variables greatly influenced the rate of soil wetting and drying.
Prolonged persistence of this variable makes it possible to use soil moisture as a climate predictor in the short and medium term.