INTRODUCTION
Next-generation sequencing (NGS) technologies include DNA sequencing and its derivatives, which are based on in-depth, high-throughput, and in-parallel methods. In this review, second-generation sequencing (SGS) and third-generation sequencing (TGS) techniques are treated as NGS. Relative to Sanger-first generation sequencing, NGS technologies are highly efficient, rapid, and cheaper and allow the sequencing of hundreds of gigabases in a few hours (Barba et al., 2014; Kulski, 2016). As a reference, the sequencing of the human genome, accomplished in 2003, took 13 years and cost about USD 2.7 billion (International Human Genome Sequencing, 2004). Today, it is possible to sequence genomes with sizes similar to the human genome in less than a day for less than USD1000 (NIH; Hernandez, 2018) TGS applications have also increased recently; however, these promising technologies are still in the initial phase of the application.
Having been available for approximately 15 years, NGS has become a widely used tool in all life sciences research. Substantial improvements have been seen in costs, sequence accuracy, and yield (Goodwin et al., 2016). Specifically, the increased use of high-throughput sequencing technologies has resulted in an exponential increase in the number of sequenced genomes, metagenomes, and transcriptomes from a wide variety of species. NGS platforms produce an enormous amount of data, the analysis, and interpretation of which has provided new insights and knowledge of molecular bases of human and plant diseases and in plant resistance to both biotic and abiotic stresses. Further, NGS has profoundly impacted the development of medicine, agriculture, and industry. NGS is part of the large scale technologies applied to the study of a whole collection of biomolecules present in a given cell, tissue, organ or organism and these are termed as "omics" and include the study of DNA (genomics), RNA (transcriptomics), proteins (proteomics), lipids (lipidomics), carbohydrates (glycomics), and other metabolites (metabolomics) as well as integrated systeomics (Kulski, 2016; Stagljar, 2016).
The application of NGS and 'omic' technologies in plant virology came into common use around 2009 (Hadidi et al., 2016) and included several approaches. Specifically, in the following text, nucleic acid sequencing and proteomics will be discussed in detail. Although NGS has been widely applied worldwide, in Colombia, its application is still at an early stage. Despite significant advances made in the application of genomics to plant virus identification and characterization, other omics have been little explored. Thus, in addition to introducing the current status and advances in NGS technologies and their main applications, this review will show that the reduced costs and extensive use of these technologies throughout the scientific community have increased their accessibility to everyone, even to developing countries, such as Colombia. Therefore, we wish to encourage Colombian researchers to implement and increase the use of high-throughput technologies.
NGS PLATFORMS
NGS technologies include several platforms that enable the sequencing of millions to billions of DNA or cDNA fragments. The lengths of the sequenced DNA fragments and sequencing methods vary based on the platform used. At present, there are seven major sequencing platforms (Table 1). According to the sequencing method, these technologies can be grouped as follows: The first group is composed of DNA sequencing technologies that previously required a PCR step for cDNA or DNA amplification. Currently, this group is constituted by both first and second-generation sequencing technologies, such as the GS FLX 454 sequencer (Roche Diagnostics Corp., Branford, CT, USA), which was discontinued, the Illumina platforms (Illumina Inc., San Diego, CA, USA), which are the most widely used, the ABI SOLiD System (Life Technologies Corp., Carlsbad, CA, USA), and the Ion Personal Genome Machine (Life Technologies, South San Francisco, CA, USA). The second group is composed of those technologies that are based on direct single-molecule sequencing (without a preceding PCR amplification step). These technologies are considered third-generation platforms by most authors (Heather and Chain, 2016) and include the HeliScope (HelicosBioScience Corp., Cambridge, MA, USA), which filed for bankruptcy in 2012, the PacBio single-molecule real-time (SMRT) system (Pacific Biosciences, Menlo Park, CA, USA), which is the most widely used third-generation technology, and the GridION, MinION and PromethION Nanopore platforms (ONT) (Oxford Technologies), which are the most promising sequencing technology. The last platform was tested by end-users in a trial in 2014 (Loman and Quinlan, 2014) and is hoped to revolutionize DNA sequencing as it allows the use of small portable devices, production of very long reads in concise times, and low costs; however, this technology has poor-quality profiles (Heather and Chain, 2016; Rang et al., 2018).
Table 1 Summary of Next-generation DNA sequencing technologies platforms and its main characteristics.
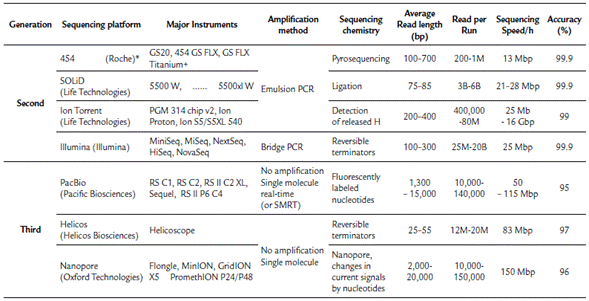
*Discontinuated; M: Million; B: Billion
Second and third-generation sequencing applied to omics studies includes the complete sequencing of genomes, transcriptomes, metagenome, amplicon sequencing, and other specific categories, such as sequencing for large-scale polymorphism discovery, bisulfite-treated DNA, methylation in genomic DNA, chromatin immunoprecipitation sequencing (ChIP-Seq) to determine protein-DNA interactions, and others. As follows, we focus and describe in detail the application of complete sequencing of genomes, transcriptomes, and metagenomes in plant virology.
NGS APPLIED TO PLANT VIRAL GENOMICS
Plant viruses are causal agents of several plant diseases; therefore, plant virus is responsible for significant losses in crop production and trade (Nicaise, 2014; Hadidi et al., 2016). As a consequence, its detection and diagnostic is a crucial step to determine a specific disease etiology involving a virus symptomatology and for its respective crop management program. For virus detection and characterization, standard methods have been used such as electron microscopy, serological, and molecular tests (Pecman et al., 2017). However, due to the high variability found among plant viruses, these methods do not always work (Hadidi et al., 2016). The application of High-Throughput Sequencing Technologies to obtain viral genomes has had aid highly to overcome this challenge. Since 2009, when the first viral genomes were obtained using NGS, viral genomics has had an enormous impact on the identification and discovery of novel viruses and viroids and also in refining the characterization and diagnosis methods of previously identified viruses (Barba et al., 2014; Hadidi et al., 2016; Blawid et al., 2017; Pecman et al., 2017).
Conventionally, genomics investigates a complete set of DNA of a given organism, including but not limited to its gene and intragenic sequences, functions, annotations, and structure (Blawid et al., 2017; Pecman et al., 2017). Genomics has progressed in parallel to the development of NGS platforms, and it has been widely applied to all life sciences. However, in viral genomics, this concept should be redirected to fit specific viral characteristics, including its variable genetic material composition, host-dependent nature, and small genome size (ranging from 2.6 kb to 19.3 kb) (Hull, 2014).
Since nucleic acid from viruses and plant are often in a mixture in which viral sequences are a tiny proportion, enrichment of viral sequences is a crucial step to be considered before sequencing. The protocols will depend on plant virus genetic material, which may be composed by a single-stranded RNA (ssRNA), double-stranded RNA (dsRNA), single-stranded DNA (ssDNA), or double-stranded DNA (dsDNA) (Hull, 2014). The main methods used for plant viral genome sequencing is viral metagenomics or viral meta-transcriptomics using modifications to virus sequence enrichment or using ultra-deep sequencing directly or from purified viral particles.
Viral metagenomics or viromics refers to those methods employed for the detection of all viruses present in a given sample using sequencing technologies (Roossinck et al., 2015). Metagenomics is the sequencing of environmental DNA, comprised of tens to thousands of organisms. In metagenomics analysis is common the use of conserved sequences, usually ribosomal RNA sequences regions; however, in viruses, there are not universally conserved genes or regions. Therefore, in viral metagenomics, the total acid nucleic (DNA or RNA) from virus-infected plants are obtained. Nevertheless, the optimal condition is to minimize (as much as possible) contamination by host genetic material. Thus, several adjustments in sample preparation protocols have been included.
These approaches improve viral sequence enrichment after sequencing. However, some of these could present disadvantages or are limited to a specific group of viruses. In addition to these approaches, the depletion of ribosomal RNA for plant ribosomal RNA elimination, the enrichment of Double-Stranded (ds) RNA using cellulose or lithium chloride useful for dsRNA viruses sequencing, sequencing from small interfering RNA (sRNA) and RNA or DNA isolation directly from partially or totally purified viral particles, known as Viral Associated Nucleic Acid (VANA), are included (Roossinck et al., 2015; Adams and Fox, 2016; Pecman et al., 2017; Jeske, 2018).
The isolation of nucleic acids and the enrichment of viral sequences are followed by quality assessments, cDNA library construction, sequencing using shotgun sequencing or whole RNA sequencing (RNA-Seq), and Bioinformatics analyses. Sequencing steps, except for cDNA fragmentation, which is the first procedure for SGS, both SGS as TGS have in common the library construction, in which cDNA templates are prepared and modified using procedures according to the requirements of a specific sequencing platform. It is essential to mention that for TGS, DNA isolation is a crucial step; hence the objective is to obtain the longest reads as possible, therefore a high DNA quality and integrity is required (Kchouk et al., 2017). Following, when a genome sequencing project is planned, estimated costs should include both the library construction and sequencing. In developing countries, whole sequencing projects by cost-benefit and time economy usually are led as a service with an external company or in collaboration with foreign universities. Currently, costs quoted by sequencing companies are between USD 500 (for SGS platforms) to USD 1500 (for TGS platforms), including library construction, sequencing, and genome assembly.
Sequencing platforms applied to viral genome sequencing has had a fast evolution, and in a short time, platforms such as GS FLX 454, which was highly used in the past, currently is discontinued. Further, Illumina platforms such as MiSeq and HiSeq series have taken advantage in the market place; however, its main limitation lies in the short length of the reads they generate which hindrances the genome assembling of viruses present in similar samples sharing a high degree of sequence identity. In contrast, TGS platforms such as PacBio offer very long read; yet, they have a lower throughput, higher error rates, and higher costs per sequenced base (Rhoads and Au, 2015). Although for TGS, the Throughput has significantly increased in the last years, its major drawback continues to be its high error rates (mainly in ONT) (Villamor et al., 2019). Nevertheless, the use of TGS to obtaining plan-virus genomes is highly promising.
Recently, a new method combining PCR-free, circular DNA enrichment (RCA) and TGS (SMRT-PacBio, Pacific Biosciences Inc.) named as CIDER-Seq (Circular DNA Enrichment Sequencing) was successfully applied to obtain the full-length sequence of a DNA-viruses without the requirement of an assembling step and therefore reducing the bias that the short length reads assembly generates in the SGS technologies (Mehta et al., 2019). In the last two years, the use of ONT technologies to sequence plant-virus genomes has been increasing. The MinION device was first applied for detecting whole genomes of virus maize streak virus, maize yellow mosaic virus and maize totivirus associated with maize lethal necrosis symptoms (Adams et al., 2017), latter on 2018 was used for detecting plum pox virus in plum plants (Bronzato Badial et al., 2018) and for detecting the yam viruses Dioscorea bacilliform virus, yam mild mosaic virus and yam chlorotic necrosis virus (YCNV) (Filloux et al., 2018). In 2019 MinION also was efficiently used for detecting the Wheat streak mosaic virus (WSMV), Barley yellow dwarf virus (BYDV) and Triticum mosaic virus (TriMV) in wheat plants (Fellers et al., 2019) and for detecting a novel bipartite begomovirus infecting cowpea plants (Naito et al., 2019).
Another crucial variable to consider is the time elapse from sample collection to data collection and analysis. Time taken for DNA isolation could comprise several months, sequencing process including the shipment, take less than a month, and here is necessary to highlight that the step which could be the most arduous and time-demanding is the bioinformatics analysis, which requires computational expertise and full dedication to data curation, annotation, and genomic structures analyses.
Globally, several advances have been achieved, and novel viruses have been identified using NGS. Applications of NGS for detection of plant viruses using different approaches in sample preparation have been well reviewed (Barba et al., 2014; Roossinck et al., 2015; Hadidi et al., 2016). However, in Colombia, the application of NGS in plant virology has been little reviewed. In the country, agro-industry is one of the most important sectors for economic development, and viruses represent a severe problem for crop production (Rodríguez et al., 2016). The economic proposal for agricultural sector 2006-2020 is based on ten groups with exporting potential, which includes mainly exotic and tropical fruits (Rodríguez et al., 2016). Thus, plant virus genomics, which has had an excellent performance in the last years, has been focused on these products.
Several studies (Table 2) involving the use of NGS for genome sequencing (complete or partial), detection, and characterization of RNA viruses infecting potato (S. tuberosum and S. phureja) (Gutiérrez-Sánchez et al., 2014; Villamil-Garzón et al., 2014; Gutiérrez et al., 2016; Muñoz et al., 2016; Vallejo C et al., 2016), peanut (Gutiérrez Sánchez et al., 2016), rice (Jimenez et al., 2018), cassava (Carvajal-Yepes et al., 2014), exotic fruits, such as Solanum quitoense (Gallo et al., 2018), Solanum betaceum (Gutiérrez et al., 2014), Physalis peruviana (Gutiérrez et al., 2015), Passiflora edulis (Jaramillo Mesa et al., 2018; Jaramillo Mesa et al., 2019), and horticultural products (Gutiérrez et al., 2017; Muñoz Baena et al., 2017) have been included. The above studies have been conducted using mainly total RNA isolated from symptomatic virus infected plants followed by depletion of rRNA and whole RNA sequencing. Plant viral genome reconstruction has been obtained by combining de novo transcriptome assembly for filtering contigs and sequences related to the virus and genome reference based on transcriptome assembly; however, few studies (Carvajal-Yepes et al., 2014; Jimenez et al., 2018) have been conducted using sRNA from virus-infected tissues.
Despite of advances achieved in the detection and identification of RNA viruses infecting plants in Colombia, additional studies are still needed for the discovery of DNA viruses. Colombia possesses a wide diversity of plants with potential for agribusiness. However, viral diseases are also a significant challenge, and many of these diseases have remained uncharacterized. Therefore, the advent of NGS sequencing is an opportunity to advance the discovery of novel plant viruses in Colombia.
NGS IN TRANSCRIPTOMICS TO ELUCIDATE PLANT-VIRUS INTERACTIONS
Considering that some viruses contain RNA as genetic material and some viruses transcribe their DNA genetic material into RNA, whole transcriptome sequencing, as discussed above, has been widely used to obtain the viral genome from plant virus-infected transcriptomes. Besides, another critical application of transcriptomics in plant virology is the study of a given plant-virus interaction. Plant viruses have small genomes coding just for a small number of pivotal proteins; thus, processes such as virus replication and movement are carried out by hijacking host cell components.
Virus components interact and disturb the plant process through the entry and cell infection. A battle between virus and plant defense systems begins, and the success of viral infection will depend on the plant's ability to resist the virus attack. When the virus overcomes the plant responses, the plant became susceptible, and disease symptoms are triggered. Therefore, in plant-virus interactions, a high transcriptome reprogramming is given, and several sets of genes are modulated in response to the viral infection (Hanley-Bowdoin et al., 2004; Bengyella et al., 2015; Zanardo et al., 2019). The study of the molecular bases of plant-virus interaction is essential for finding genes or pathways involved in plant defense or critical components for virus infection, replication, or movement. Taken together, this knowledge is pivotal for crop improvement programs directed at plant resistance to viral diseases (Hillung et al., 2016; Zanardo et al., 2019).
Among approaches for transcriptome study, currently, whole transcriptome sequencing commonly called RNA-Seq is one of the most widely applied. This technique is aimed to study of all sets of transcripts expressed in a given organism in a given condition (Mosa et al., 2017). Overall, the methodology applied to the plant-virus interaction consists of total RNA isolation from plant-infected or virus-inoculated plants and plant-virus free or mock-inoculated samples, followed by the cDNA library construction and subsequent sequencing using an NGS platform. The most widely used platforms for RNA-Seq are Illumina Hiseq 2000, 2500, and 4000 series. However, with the introduction of the NovaSeq series, also Illumina platforms, this picture probably will change in brief. The current costs for RNA-Seq range from 250 to 450 USDs for library construction and sequencing of approximately 20-40 M reads per sample. Similar to the genome sequencing, after sample preparation, the sequencing process is fast and could take about one month, while bioinformatics analyses could take several months depending on the expertise.
RNA-Seq has been used to find insights in several plant-virus interactions, including model plants such as Arabidopsis thaliana (Sun et al., 2016; Wu et al., 2016), plants of agronomical importance such as Oryza sativa (Blazquez et al., 2013; Wong et al., 2015), Zea mays (Chakrabarty et al., 2018), Manihot esculenta (Fang et al., 2014; Amuge et al., 2017; Anjanappa et al., 2018), Solanum tuberosum (Goyer et al., 2015; Stare et al., 2017) and tropical fruits such as C. papaya (Madroñero et al., 2018). However, to date (04-102019), a Google Scholar search, the NCBI PMC database, and the Web of Science using the queries "plant", "virus", "interaction", "transcriptome", "RNA-Seq", and "Colombia" with different combinations did not yield any results for plant-virus interactions research developed in Colombia.
PROTEOMICS TO BETTER UNDERSTAND PLANT-VIRUS INTERACTIONS
The term proteomics has been used to denote the "PROTein complement of a genOME". It groups all techniques employed for protein studies in a given condition which involves protein characterization localization, interactions, post-translational modifications and others (Wilkins et al., 1996). Currently, proteomics has allowed identifying a complete set of proteins, present in a particular organism submitted to changes in physiological activities and/or caused by external factors which, is technically termed as "sub-proteomes" (Peck, 2018; Zaynab et al., 2018). Plant proteomics became widely applied around 2000, and its use has increased thanks to the advent of NGS technologies. However, transcriptome, and proteome are not always correlated this lack of correlation is mainly due to the post-transcriptional regulatory processes; however post-translational modifications also play a crucial role. For that reason, proteome and transcriptome should be taken as complementary tools.
Usually, proteomics could be used to identify proteins from purified virus, virus-enriched samples, or using plant virus-based expression systems. However, in plant-virus interactions, the most common application is using total proteins from infected tissues. Like transcriptomics, in proteomics, the qualitative or quantitative proteome profile of a specific plant previously submitted for virus infection is obtained. Further, an analysis to identify the differentially abundant proteins is conducted in non-infected cells as compared with infected cells. This approach allows identification of host or viral proteins that play critical roles in infection or that evade host defense mechanisms (Xu and D. Nagy, 2010).
For proteomics, it is crucial to know the preparation method and properties of the sample and protein extract. In order to cover a wide range of sample types, improve data coverage, and obtain accurate quantification, different methods have been developed for proteomic analysis, which owing to its high sensitivity can detect changes in the abundance of proteins in different types of samples (Li et al., 2012).
Proteomics is classified into three main groups: I) Expression proteomics studies which are addressed to the investigation of protein expression. These studies can be conducted using conventional (Chromatography, ELISA Western blotting) and/or advanced techniques (Gel-based approaches, Mass spectrometry, Edman sequencing). II) Structural proteomics studies are analyzed using high throughput techniques (X-ray crystallography) or bioinformatics tools for protein structure prediction. These last are very well reviewed in (Roy and Zhang, 2012). Even when this work is from 2012, contains several bioinformatics tools which are still widely used. III) Functional proteomics, which is the focus of this review, comprises the quantitative techniques which allow to understand the protein functions as well as elucidating unknown molecular mechanisms, protein interactions, and associations of an unrevealed protein with partners from a given protein complex (Chandrasekhar et al., 2014).
In plant virology, as described above, it should be taken into account that viruses encode small proteomes (1-2500 proteins) and that the proteomes of viruses and plants are determined by the virus-host protein interaction, wherein the virus focuses on ensuring its infective replication and the plant focuses on blocking the virus infection. Plant responses to virus infections are speedy, and a drastic change in the protein accumulation is triggered in the whole plant, this protein accumulation provides the crucial clues to understand the plant-virus interaction and the resistance mechanisms to the virus infection (Kundu et al., 2013; Varela et al., 2017; Souza et al., 2019). On the other hand, leaves are a principal organ for studying plant-virus interactions, because these generally exhibit necrotic patches or morphological variations that allows to visually detect the first infection symptoms (Di Carli et al., 2010; Kundu et al., 2013; Varela et al., 2017; Souza et al., 2019). However, not always symptoms are evident, what makes then even more necessary the implementation of reliable and specific techniques, such as proteomics to assess the protein levels and interactions under such conditions (Mochida and Shinozaki, 2011; Mosa et al., 2017).
Not-targeted proteomics is the most commonly used technique. In this technique, proteins are identified through a process known as a shotgun, in which proteins are digested in small peptides using different proteases. The obtained peptides are analyzed by Liquid chromatography-mass spectrometry (LC-MS). However, this approach could produce unreliable and irreproducible results (Li et al., 2012). The most widely applied method for the simultaneous identification and quantification of proteins is the label-free method. Label-free is a low-cost and straightforward method which do not require the use of stables isotopes carried in the sample, instead, the signal intensities (peaks areas) or spectral counts of peptides belonging to a specific protein are correlated with the protein amount present in a sample obtained under different conditions or treatments (Li et al., 2012). Nonetheless, label-free presents poor yields in the quantification of low abundant proteins (Choi et al., 2008; Vowinckel et al., 2014).
Recently, chemical or enzymatic methods for protein or peptides labeling using different isotopes tags in vitro or in vivo have been developed. These tags include the ICAT (Isotope-coded affinity tag), which, after protein digestion, the C-terminal of peptides is tagged using the isotope H218O and ReDi (reductive dimethylation) and the N-terminal and lysine lateral chain of peptides are tagged by reductive demethylation. However, some authors have suggested that methods based on isobaric chemical labeling such as iTRAQ (Isobaric Tags for Relative and Absolute Quantitation), Stable isotope labeling by amino acids in cell culture (SILAC) and TMT (Tandem Mass Tag) have been found to be most successful when applied to samples previously analyzed by LC-MS and IMAC (Immobilized metal affinity chromatography, a strategy for enriching phosphorylated peptides) (de la Fuente van Bentem et al., 2006; Jayaraman et al., 2012).
Around the world, proteomics has been widely applied to study plant-virus interactions. It includes crops of economic importance, such as maize and Soybean (Wu et al., 2013; Pavan Kumar et al., 2016), and also plant models, such as tobacco and tomato (Di Carli et al., 2010; Lin et al., 2015; Alexander and Cilia, 2016; Megias et al., 2018). For those readers interested in deepening, Souza et al., (2019) present an excellent review of proteomics applied to several studies of plant-virus interactions. In Latin-America, proteomics is less addressed; basically, the country that is leading the research studies on structural, expression and functional proteomics in plant-virus interactions is Brazil. Some examples of researches conducted in Brazil are the study of the chloroplast proteomic profile in Tomato blistering mosaic virus (ToBMV) and Nicotiana benthamiana interaction (Megias et al., 2018), the host proteomic response to Citrus tristeza virus (Dória and Pirovani, 2019) and to the Papaya meleira virus complex (PMeV) (Soares et al., 2017). In Colombia, however, similarly to transcriptomics, proteomics has been little explored. To date (16-07-2019), a detailed search on Google Scholar, the NCBI PMC database, and the Web of Science, using the queries "plant", "virus", "interaction", "proteomics", "functional proteomics" and "Colombia" with different combinations did not yield any results for functional proteomics applied to plant-virus interactions research developed in Colombia.
BIOINFORMATICS RESOURCES AND OMICS TOOLS FOR PLANT-VIROLOGY STUDIES
There are many bioinformatics tools and resources that ease experimental workflows. Each omic has its specific bioinformatics pipeline; however, there are common databases and resources for analysis of data from all omics. Here, the main tools, bioinformatics pipelines, and databases for public data acquisition and data visualization focused on viruses and plants are introduced.
Overall, for plant virus genome discovery, after sequencing, bioinformatics is used for sequence quality analysis and trimming, de novo assembly of contigs, and similarity-based searches for finding virus-related scaffolds and contigs. Once viral contigs are obtained, these are used for an extending virus genome contigs using de novo assembly or reference genome-based assembly (Blawid et al., 2017). Currently, there are several open-source, free, and fee-based software (Table 3), used for bioinformatics analyses of plant viruses. Among the licensed software, the most commonly used include CLC Genomics Workbench, Geneious, and DNASTAR. Although these tools offer a large number of resources for genome and transcriptome analyses, there are also freely available software with more user-friendly interfaces, such as VirAmp (Wan et al., 2015), which is a tool included in the Galaxy project, and VirusTap (Yamashita et al., 2016), which was released on 2016. For those who are familiar with command lines and prefer to have access to additional sets and parameters, multiple open source assemblers are also available, which are described in detail elsewhere (Blawid et al., 2017).
When the objective is the analyses of plant-virus interactions, similar to the common virus discovery strategy, bioinformatics analyses are carried using the transcriptome data. However, in this approach, the focus is the plant instead of virus sequences. In comparison to the virus-genome transcriptome-based discovery, the study of plant-virus interactions is directed to find all transcriptome alterations caused by virus infection in the plant. Therefore, quantitative analyses are prioritized, and a higher depth in sequencing is required. For bioinformatics analyses, there are also licensed software and free web interface-based resources such as Galaxy (Goecks et al., 2010) and RobiNa (Lohse et al., 2012) available, which are including pipelines for RNA-Seq. However, because of a large amount of generated data, its processing demands high computational power. Thus, it is recommended to use programs that are run on UNIX-like operative systems and directly using a command line interface that exposes all the parameters of the different packages/tools. Overall, transcriptome analyses of plant-viral interactions involve analysis of sequence quality and trimming, transcriptome assembly, annotation, quantification, and differential expression analyses between infected and control plants.
For reference genome-based transcriptome reconstruction, the most commonly used short reads mappers are TopHat2 (Kim et al., 2013), Star (Dobin et al., 2013), and HiSat2 which is the replacement of TopHat nowadays (Kim et al., 2015). For de novo transcriptome assemblers, the commonly used versions included Trinity (Haas et al., 2013), SOAPdenovo-Trans (Xie et al., 2014), Trans-ABySS (Robertson et al., 2010), IDBA-tran (Peng et al., 2013), and Oases (Schulz et al., 2012). Once transcriptome assembly is completed, before the differential analyses of gene/transcript expression, the abundance levels of genes should be estimated. Unlike genome sequencing, in the transcriptome, sequence coverage is indicative of abundance levels. Therefore, the developed methods for quantification are based on the reads counts or normalized reads counts belonging to a given exon, gene, or transcript.
These methods are grouped into two categories: union exons and transcript-based. While programs such as featureCounts (Liao et al., 2013) and HTSeq-count (Anders et al., 2014) are based on the union exon method, programs such as Cufflinks (Trapnell et al., 2012), RSEM (Li and Dewey, 2011), BitSeq (Glaus et al., 2012), and the recently implemented programs such as Sailfish (Patro et al., 2014), RapMap (Srivastava et al., 2016) , Kallisto (Bray et al., 2016), and Salmon (Patro et al., 2017) are transcript-based methods. However, these last are approaches based on algorithms such as pseudo-alignment, lightweight mapping, or quasi-mapping. For these methods, the reads are broken in short-sequences named as k-mers. These characteristics increase the accuracy and efficiency of these quantification methods.
Once the expression levels are estimated, these data are incorporated in programs developed to conduct differential expression analysis. These programs use different statistical methods in their analyses. Programs such as edgeR (Robinson et al., 2009), baySeq (Hardcastle and Kelly, 2010), DESeq (Anders and Huber, 2010; Love et al., 2014), these programs is spreadsheets or plain text files containing and Cuffdiff (Trapnell et al., 2013) are widely used for the ID of genes or transcripts and its corresponding conducting differential expression analysis. The output of statistical data.
Table 3 Commonly and friendly-interface omics Tools and web resources used in the study of plant virology.
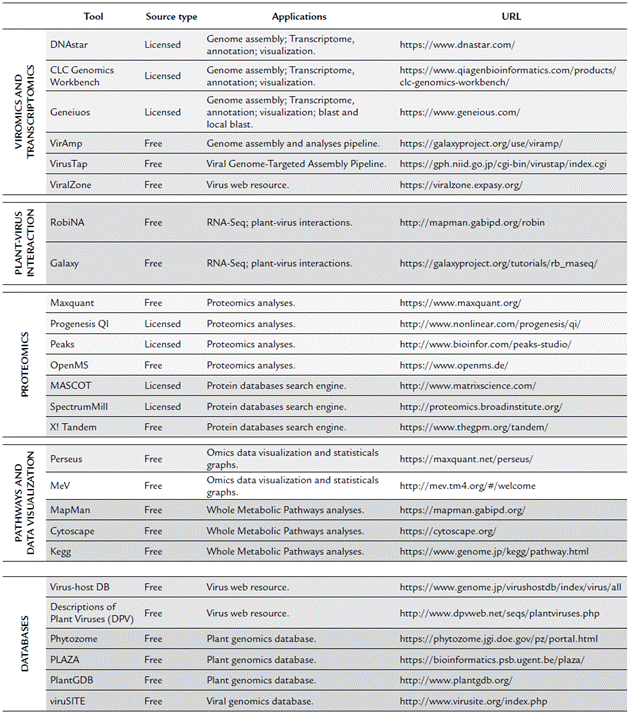
For proteomics analyses, data analysis is highly dependent on the used Mass Spectrometry instrument, therefore, the raw files generated can be very variables; however, among the best software for complement quantitative analyses are the MaxQuant (Tyanova et al., 2015) and Progenesis QI (licensed), which are used to analyze, identify, and quantify high-throughput mass spectrometry data. For PEAKS (licensed), this software is used to identify peptides and posttranslational modifications from de novo sequence peptides analyses. OpenMS (Röst et al., 2016) is an open source resource based on C ++ libraries for LC-MS data analyses. Subsequently, using the obtained mass spectrometry data, proteins can be identified by querying in peptide sequence databases using licensed software such as MASCOT, SEQUEST, Phenyx, SpectrumMill, and IdentityE or open source resources such as X! Tandem (Bjornson et al., 2008) and OMSSA (Geer et al., 2004). The final output of these programs is also filed with the protein ID and corresponding statistical and quantitative data.
Using the output files obtained from proteomics and transcriptomics analyses, data visualization, and pathways analyses for those who are familiar with the R language could be conducted using several R packages. However, there are also free friendly interface tools, which require as input, a file with the gene/transcript or protein IDs and results of the quantitative analyses. This group of free resources include Perseus (Tyanova et al., 2016; Tyanova and Cox, 2018) and MeV (Howe et al., 2011), these programs possess several tools for statistical graphs, Heatmaps, cluster and functional enrichment analyses. For metabolic pathways analyses, among the most widely used programs are MapMan (Thimm et al., 2004), Kegg (Kanehisa and Goto, 2000) and Cytoscape (Shannon, 2003). This last, although is a generic tool for the analysis and visualization of network data, is a powerful resource which works with plug-ins and applications for several tasks including plug-ins for metabolic pathways analyses such as MetScape, MetaNetter 2, GeneMANIA and KEGGscape.
Last but not least, for data storage and acquisition, there are also many public repositories. Among these, there is available the International Nucleotide Sequence Database Collaboration (INSDC) which is a collaboration between the DNA Data Bank of Japan (DDBJ), The European Bioinformatics Institute (EMBL-EBI) and The National Center for Biotechnology Information (NCBI) for making available and gathering the most extensive public repositories of nucleic acid sequence data around the world. NCBI also provides more specific resources such as The Reference Sequence (RefSeq) collection (O'Leary et al., 2016), and the Sequence Read Archive (SRA), which store a large number of NGS bio-projects both transcriptome and genome data. Another useful Database for sequences storing and analyses is the Protein Data Bank (PDB) (Rose et al., 2012) which contain the Protein Data information about the 3D shapes of proteins, nucleic acids, and complex assemblies.
More specifically, databases for the study of plant virology include those focused on virus genomes, such as the International Committee on Taxonomy of Viruses (ICTV). Although this database does not include sequence information, it is a useful tool for determining an officially reported virus and its taxonomy. The ViralZone (Hulo et al., 2011) is a web resource that combines information about the virus host range, replication cycle, and virion structure with genomic and proteomic sequences. The Virus-host DB (Mihara et al., 2016) is a good resource for finding virus sequences by searching the virus name or host taxonomy. Other databases specific for plant viral genomics are the Descriptions of Plant Viruses (DPV) (Adams, 2006) and viruSITE (Stano et al., 2016). Among the databases that contain plant genome sequence information and tools for annotation are Biomart, Blast, Bioextract, Phytozome (Goodstein et al., 2012), PlantGDB (Dong, 2004), and PLAZA (Proost et al., 2015).
Taken together, all these resources highly facilitate work with large amounts of data generated by large-scale omics approaches and help to provide biological context and an explanation for some given phenomena from computational analyses.
FUTURE PERSPECTIVES
NGS technologies and 'omic' technologies have opened the door to immense knowledge regarding the genomics, transcriptomics and proteomics of organisms. Further, these advances have helped discover the high diversity between and within species. The genetic analysis of a unique gene become in an analysis of multiples genes, an analysis of a complete genome. NGS enables the determination of the complete genetic sequence even of various interacting organisms. The challenge, however, lies in establishing the relationship between them. In Colombia, the use of NGS is very scarce, and even more so for bioinformatics analyses. Currently, bioinformatics analyses are a bottleneck for progress in Colombian research because these are time-demanding and require qualified personnel; for instance, the comparative genome analyses are increasingly delayed regards to the massive amounts of data that are deposited in databases globally. To facilitate workflow, Figure 1 summarizes the general process to follow for plant virology studies using NGS technologies. Based on the reports of Colombian research teams that have used NGS technologies and our own experience, it is necessary to design strategies to extensive the use the NGS and 'omic' technologies in the country. However, it is more urgent to increase the number of researchers trained to perform bioinformatics studies and to create and strengthen collaborations for genome sequencing projects.
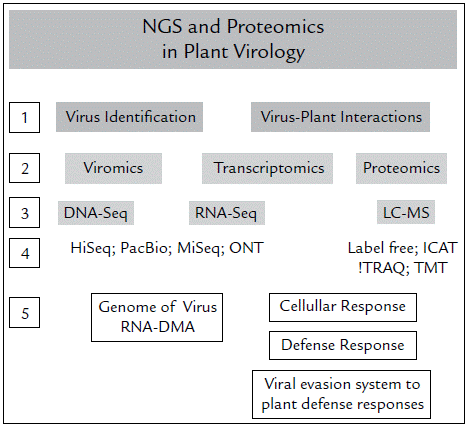
Figure 1 Strategies (NGS and proteomics) for the study of viruses in plants. First, the objectives must be established: identify viruses or evaluate how they interact with plants (1). According to this, you must choose which is the most relevant strategy between Genomics, Transcriptomics and / or Proteomics (2) and which is the technique (3) and platform (4) most suitable in terms of costs, throughput and quality of information collected and evaluated. Finally, with the data analysis you can identify the viruses (DNA or RNA) present in your sample, or establish the cellular responses, the defense mechanisms established by the plants against virus, and vice versa (5).
The use of NGS and "omics" technologies in the study of plant-virus could allow to the Colombian researchers to analyze the complete view of any biomolecules into the organism, a complete information of genome, how many, which and where are located the virus genes in the host, which and how many of them are being transcribed and translated. Viral genomics allows to identify new viruses and to establish the relationship among them. The comparative genomic analysis will reveal the genetics mechanisms of entrance and movement of virus into the cell. Also, the proteomic allow us to know the changes in the host protein profile induced by the expression of the virus proteins. Taken together, omics used as complementary tools are crucial for revealing the molecular mechanisms determining resistance or susceptibility, as well as finding genes with critical roles in the viral infection processes which can be used in crop improvement programs.