1 Introduction
Colombia, despite the economic growth of the last decades, continues with structural and business management limitations that slow down the sustainable improvement of the productive efficiency of its companies. In particular, entities such as Corpoica, currently AGROSAVIA [1], in their diagnosis of the agricultural sector, show the imbalances in productivity and technical inefficiency between economic activities and the departments of the country, attributable to the limited use of technological packages, to insufficient investment in science, technology and innovation, among other aspects, which restrict an optimal use of productive factors.
In this direction, authors such as Quintero, Prieto, Barrios and Leviller [2] have focused their studies on knowing the individual and associative contribution of production factors that affect productive efficiency and to give recommendations for improvement in business management as a competitive strategy. Farrell [3] was a pioneer in defining productive efficiency as the result of price efficiency and technical efficiency; furthermore, together with other authors as Debreu [4] and Koopmans [5], they indicated that this efficiency is a relative concept that starts from the comparison with other possible use alternatives of production resources (in input selection or cost reduction) that allow the maximization of the results. Besides, its valuation is given from the production ceiling or efficient frontier obtained in the selected sample.
According to Battese and Coelli [6], there are worldwide two known ways to evaluate the production function: deterministic (mathematical) and probabilistic (stochastic). The current research chooses to deepen in the study of the latter through an empirical estimation of the Cobb-Douglas type production functions and the comparison of the elasticities of the output to variations of different production factors in the sample of agricultural companies.
There is considerable variability in studies that inquire about the effects of production factors on the productive efficiency of the company, its sector, and territory from the use of the stochastic frontiers methodology. For example, Mungaray and Ramírez [7] explained the positive contribution that formal and informal human capital makes in the operations of low-value-added Mexican companies with financial, technological, and market access limitations. While Domínguez [8] and Albornoz [9], on current assets, highlighted the importance of disposing of sufficient working capital for the stability of the company in aspects such as meeting their financial obligations in the short and medium terms and having timely access to discount opportunities provided by their commercial suppliers, among others.
More recently, Jara [10] found 40% technical inefficiency in a group of companies in Latin America (which included Colombia) and that were linked to the primary activity. Further, regarding business size, large companies have better efficiency percentages on an increasing scale and with higher levels of income compared to small and medium-sized companies; therefore, this author suggests differential strategies that increase productivity and sectoral competitiveness.
Two specific studies in Colombia based on the function of production costs in cassava, cotton, and coffee farms, led to defining the maximum quantities of product that can be obtained with a given volume of inputs and whose results supported the presence of limitations in the competitiveness of the agricultural sector for the maximum number of products available [11,13].
Considering what was mentioned above, we infer that research on productive efficiency in the national agricultural sector and on the peculiarities of its production factors is limited to guide improvements in business management and sector planning, and hence, to define strategies that stimulate territorial growth. Consequently, the aim of this study was to evaluate the behavior of the productive efficiency of agricultural companies in Colombia, starting from a hypothesis to be tested: the efficiency percentages of the national agricultural sector vary depending on the geographical location and business size.
2 Methodology
Following the methodology described by Battese and Coelli [14], this research performs a stochastic evaluation of the production function to estimate the average technical efficiency of the sample of selected companies in the Colombian agricultural sector. This allowed knowing the optimal combination of inputs for the definition of the stochastic frontier and obtaining the technical efficiency indicators that show the gap between this frontier and the production technology of the companies in the sector.
We started by understanding the efficient frontier as the maximum theoretical response that can be obtained from a mix of possible inputs in a productive unit [15]. As anticipated, this measurement allows us to temporarily compare each productive unit with the best one that has been observed in practice (frontier) within a given group of companies, economic sector, or region. However, the measurement of the efficient frontier has limitations in terms of the affectation caused by the context where it is measured, the use of the technology used, and the restrictions of the production scheme [6].
For the measurement of the production function returns, the Cobb-Douglas function was selected, which reflects the constant returns and a multiplicative relationship at the rate of the inputs and outputs that are involved. This type of function was selected, given its operational simplicity and the fact that there are no restrictions on the value and possibility of variation of the production frontier. Below, the linearized Cobb-Douglas type equation with estimates of the β parameters and the technical efficiency component is presented:
Where i represents the i-th producer; j is the input considered, and Y represents the production. D ℎ refers to the dummy variable to control location effects, the subscript h indicates the department where the company is located, and vi groups the variables in production that are random and beyond the control of the company (e.g., adverse climatic effect such as a pluviometric regime of prolonged drought, low to complete absence from work due to sickness, among others). Further, ui are non-negative random variables higher than zero, and unobservable associated with the technical inefficiency of production. In the original model, it is assumed that each ui is distributed independently as an asymmetric, seminormal distribution with a mean of zero and the σ2u variance. The technical efficiency will be total when ui = 0, with the presence of technical inefficiency when ui> 0, regardless of the value taken by vi.
The model assumes that the variables are independently and identically distributed (i.i.d) as a Normal with zero mean and constant N variance (0, σ2v). An error term (εi) can also be considered from the values of vi and ui described above, so εi = vi - ui
From the minimum quadratic adjustment, the stochastic frontier model of the mean production function was estimated, with the maximum-plausible estimation using the Davidon-Fletcher-Powell algorithm [16]. This allowed obtaining estimators of the λ parameters (coefficients between the standard deviations of vi and ui) and σ2 (sum of the variances of those error terms). On the other hand, the γ parameter measures the production proportion of the variance attributable to technical inefficiencies, and, therefore, it is susceptible to being corrected through improvements in the management of the production factors. The ˠ parameter is estimated as ˠ = λ2/ (1+ λ2) = σ2u/ (σ2u+ σ2v), so that 0 ≤ ˠ ≥ 1 [17].
The technical efficiency indices for each company are calculated using the equation ETi = exp[-E(ui/ εi)], where 0 ≤ ETi ≤ 1, and E(ui/ εi) is the expectation of the term ui, conditional to the εi value, whose value provides an estimator of that error term, being able to be obtained using the formula developed by Jondrow et al. [18].
It should be noted that the elasticities associated with the coefficients expressed in the natural logarithm variables show the increase in productivity output. Moreover, using a contrast test, it was possible to establish the variations in scale returns (constant, increasing, or decreasing) in all the regressions of the study that used equation 2.
The inefficiency term was modeled according to the department in which the company is located, while the classification by business size was made based on the number of employees that the company has. In order not to overestimate or underestimate business production, the calculation of the inventory differences of the production reported in total sales by the agricultural companies and which were reported in their business records was used.
Finally, the statistical processing of the production frontier and the stochastic (random) frontier were performed with the R software, version 3.5.2 (2018-12-20) - "Eggshell Igloo" Copyright (C) [19]), using the FRONTIER package (Tim Coelli and Arne Henningsen, 2013).
2.1 Data and variables
The stochastic frontier model was determined to assess the contribution of total factor productivity to the economic growth of a sample of 410 commercial agricultural companies in Colombia that carry out 20 economic activities according to the National Classification of Economic Activities (NACE Rev. 2) that can be seen in Table 1.
The subsectors with the highest business participation are other cattle and buffalo farming (17.32%), support activities for agriculture (17.07%), the cultivation of sugar cane, and agricultural production combined with livestock production (each with 10.49%). On the other hand, by business size, 78.53% is concentrated in medium- and large-sized companies, while the remaining 21.46% are small companies.
The analyzed information was extracted from those companies that make periodic reports of their financial balances in the Mercantile Registers of the Colombian Chambers of Commerce, and that are collected in the ORBIS Database (accessed at Universidad Complutense de Madrid, Spain [20]), excluding micro-enterprises (according to the criteria of Ministerio de Comercio, Industria y Turismo [21]), or those who had financial problems. The geographical representation of the study includes 25 departments of the country (including the special district of Bogotá) and leaving out the departments of Amazonas, Arauca, Boyacá, Guainía, Guaviare, San Andrés and Providencia, Vaupés, and Vichada. In particular, it should be noted that 50% of the agricultural companies in the study were concentrated in the department of Valle del Cauca (36.48%) and Bogotá (27.03%).
As for the temporality, it only corresponds to the year 2015 given the limitations for obtaining panel data; this is a restriction of the current study that would allow contributing to the evolutionary analysis of agricultural efficiency or technological change in the country.
The inputs or model entries correspond to the variables related to the productive factors of labor and capital (measured in thousands of dollars), whose characteristics are described in Table 2.
It should be noted that the selection of the variables described above obeys to the theoretical foundations and empirical verification of their effect on increasing profitability, innovation, and the competitiveness of companies. For example, current assets offer sufficient working capital for the normal development of the operational management processes of the company, and allow taking advantage of the commercial opportunities offered by its suppliers [8,9]. For its part, intangible assets, is a non-physical productive resource that allows obtaining improvements in production technology through new productive knowledge, including patents, trademarks, franchises, goodwill, and investment in research, development, skills and tacit knowledge of workers or the organization [22]. Meanwhile, the variable number of employees shows the human capital that the company possesses, its level of training, and the experience of the personnel available to the productive unit.
3. Results
This section includes two parts. The first part defines the production function of the Colombian agrarian sector, and the second one estimates the stochastic frontier at the general level and by company size, together with the identification of the departments that have the most inefficient companies in the sector.
3.1 The agricultural production function for Colombia
Table 3 shows the estimation of the sectoral production function, its elasticities, and the significance of the variables with the most significant contribution to the generation of agricultural output.
Table 3 Estimation of the production function for the agricultural sector

(*), (**), (***) represents a significance lower than 10%, 5%, and 1%, respectively
Source: Elaborated by the authors
The production factors used explain 50.21% of the income of the agricultural companies of the country. In particular, a significance of less than 1% was obtained for the factors number of employees and current assets, with elasticities of 0.76275 and 0.08749, respectively; therefore, we deduce that increases of 1% in the labor production factor and business liquidity generated income increases of 0.76% and 0.08%, respectively.
3.2 Stochastic frontier functions
With the variables used in the production function in the previous section, the stochastic frontier [6] was modeled in Table 4 for the national agricultural sector at a general level and by business size, in addition to its inefficiency by departmental location.
Table 4 General stochastic frontier of the Colombian agricultural sector

(*), (**), (***) represents a significance lower than 10%, 5%, and 1%, respectively
Source: Elaborated by the authors
The results allow us to affirm that the agricultural companies of the country are 52.52% efficient, with a significance of less than 1% in the same variables of the production function, added to the contribution in the significance of less than 5% comprised by intangible assets. The estimators obtained at a general level allow us to affirm that the sector has decreasing returns of scale in the sum of the coefficients of its elasticities (0.73), a scenario that in the long-term does not project increases in the technical properties of the national agricultural sector. Furthermore, the efficiency parameters (γ = 0.7007) and the variance (σ2) have a significance of less than 5% and 1%, respectively.
On the other hand, this model allowed testing the hypothesis of the existence of efficiency variations by business size and by its location; promptly, the most inefficient departments in the country are Norte de Santander and Putumayo, both borderline departments of Colombia.
Table 5 presents the lowest average technical efficiency value in the study (47.85%), with high significance (1%) in the same production factors obtained at a general level, with significant variance (σ2) of less than 1% of the variables in production that are random and beyond the control of the company. The sum of the model estimators indicates decreasing returns to scale of less than one unit (0.77), with a significance efficiency (γ = 0.6504) of less than 5%. This is an opportunity to improve the business management of these productive factors.
Table 5 Stochastic frontier of small businesses

(*), (**), (***) represents a significance lower than 10%, 5%, and 1%, respectively
Source: Elaborated by the authors.
Fig. 1 shows heterogeneity in the efficiency behavior of small agricultural companies in the country, with the highest frequencies in the range of 0.9 and 1.0 of efficiency.
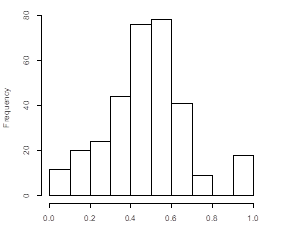
Source: Elaborated by the authors.
Figure 1 Mean efficiency of small agricultural companies distributed by frequency
The Table 6, which included medium- and large-sized companies presented high significance (1%) in the factor number of employees and current assets (like the general group), although it also reflected a significance of less than 5% in the factors of intangible and fixed assets. This group was the only one that made use of all the production inputs that have been linked to the production process in a significant way. This explains why a higher mean efficiency margin within the Colombian agricultural sector (68.37%) was reached; further, the production variance parameters obtained were less than 1% (γ), and the σ2 was attributable to technical inefficiencies and random error. Therefore, there are plenty of possibilities of being positively affected by improvements in the management of production factors. Fig. 2 concentrates more than 50% of medium- and large-sized companies in the average efficiency range of 0.4 and 0.6, although it also reports companies that manage to operate on the efficient frontier. The most inefficient region was Santander, the department that borderlines with Venezuela and also borders the most inefficient department of the group of small companies (Norte de Santander).
Table 6 Stochastic frontier of medium and large companies

(*), (**), (***) represents a significance lower than 10%, 5%, and 1%, respectively
Source: Elaborated by the authors
4. Discussion
Unlike the results found by Jara [10] for Latin American countries, the Colombian agricultural sector, independent of its business size, makes better use of the productive resources and capital (50%), without obtaining satisfactory average efficiency margins to be competitive; hence, the key to making the best use of their production inputs lies in business management. By company size, the results obtained coincidence with this same author, particularly regarding the low technical efficiency indexes of small agrarian companies; furthermore, results obtained in the current study also agreed with Moreno and Castro [23] in the favorable performance found in the average efficiency of the large national agrarian companies. Along these same lines in Mexico, Mungaray and Ramírez [7] indicated increases in the productivity of personnel linked to small businesses that access technology transfer and capacity-building programs. Just as in Colombia, leading entities in the national agricultural sector have shown that the low-efficiency margins are due to the limited access and linkage of technologies to the sector (Corpoica, currently AGROSAVIA [1]).
Consequently, the study verifies the vast opportunity to improve the productive efficiency of the Colombian agrarian sector and especially, to increase the decreasing returns to scale of the production frontier of the small companies in the sector, from the implementation of business management strategies, such as higher availability of qualified human resources linked to the productive and institutional support apparatus, providing greater accessibility to liquid money in the operational year of the company, with an expansion in the investment in intangible assets and rights subject to economic valuation by the company.
Regarding the effect of the location of the company, the agricultural departments with the highest technical inefficiency are borderline regions with countries such as Venezuela, Ecuador, and Peru. This leads to explaining that their inefficiency is due to the high labor informality margins in the sector, the devaluation of the currency, and tax collection losses due to the entry of smuggled goods, among other aspects, which subsequently motivate its empirical research.
5. Conclusions
The estimation method for the stochastic frontier functions used for the Colombian agricultural sector allowed estimating the sectorial efficiency by business size and by departmental location.
At a general level, the agricultural companies showed medium positive efficiency levels and with high significance in the productive factors number of employees, current assets and intangible assets; therefore, these factors are key to a more significant contribution to the increase in returns to scale of the agricultural business efficiency of the country. In particular, the medium and large companies in the sector use all their inputs that were part of the study to improve productive efficiency, that is, that the management carried out by the entrepreneur in this group is more successful in obtaining higher income.
The business size within the Colombian agrarian sector reports margins in its productive efficiency, being the group of large companies the ones with the highest incomes; besides, at the departmental level, inefficiency factors were found based on the business location, particularly marked in regions bordering countries such as Venezuela, Ecuador, and Peru. This leads to recommending the generation of differential public-private policies for the national agricultural sector (by size and location of the company), linked to small business management strategies in various areas such as i) higher quality and local supply of qualified personnel, ii) increase the R+D+i and technology transfer generation environments, and iii) encourage official and unofficial mechanisms of access to credit for higher liquidity in the short term.