1. Introduction
With the increasing number of work accidents, which might lead to death, occupational safety and health have been widely discussed, as they affect both industries and families, impacting on companies’ productivity, social security costs and especially the workers’ health itself 6,31. In addition, dealing with uncertainties and problems within organizations is a way to keep the organization active in the market 13.
According to the International Labor Organization (ILO), every 15 seconds a worker dies as a result of illness or work-related accidents and 151 workers suffer some type of occupational accident 17. In Brazil, between 2012 and 2020, 5,589,837 accidents were reported and 20,467 of those occurrences resulted in death, as demonstrated in the Occupational Health and Safety data. Thus, it is estimated that a worker dies every 3h 51m 28s 33.
In 2020, there were about 63,447 accidents on Brazilian federal highways, of which 51,865 were fatal 8. Additionally, an analysis of the time series of occupational accidents and illnesses from 2008 to 2014, showed the transport, storage and mailing/shipping services were the subsectors of economic activity with the highest accident rates, not to mention that they presented, at that time, an upward trend 31.
The National Institute of Social Security (NISS), responsible for ensuring victims’ rights, annually makes the Work Accident Communication (WAC) data available to the public, since 2008 2. Given the volume of data produced on accidents, occupational diseases and deaths, data mining (DM) is an alternative to develop preventive programs and policies 15,27. The use of data mining and machine learning facilitate the transformation of a large volume of data into useful information, as well as the detection of patterns and trends, making it possible to predict and discover parameters for occurrences through analysis 28,38.
The analysis of research related to data mining and Occupational Safety and Health (OSH) allows finding highly relevant studies focusing on cases of death in the occupational environment. In this regard, several studies have been carried out in other countries considering which factors can lead workers to die 21,23,32. Mining techniques applied to the analysis of occupational data can assist the organizations decision-making in terms of management 4,22.
Occupational injuries, illnesses and deaths emerge in high economic costs for individuals, employers, the state and, therefore, society in general. An overview of the financial consequences of occupational injuries and illnesses provides governments and organizations with relevant data that can be useful for the development of OSH policies. Furthermore, insights into these costs can raise awareness of the magnitude of the problem and, thus, contribute to an efficient allocation of resources for OSH 34.
In Brazil, drivers of heavy load vehicles and passenger transport are part of a significant activity for the country’s economy. However, the working conditions offered to these professionals often do not reflect the economic relevance of their activity 12. Improving traffic safety and reducing the number of road accidents are essentials 10, because when it comes to developing countries, this problem has become a major social and economic burden, as death, disability, and long-term health care result in a large social security cost. Road accidents, which mostly involve low or middle-class individuals, have become as critical as any disease, and pose a risk to society in general 30.
The aim of this study is to make use of classification data mining techniques to analyze occupational accident records in the Brazilian transport sector, emphasizing the relationship of deaths with other factors linked to work accidents. The research, used on the WAC database, is divided on a quarterly basis. For this application, months between July-September 2018 to July-September 2020 were selected to provide an overview of the issue in the transport sector. This period represents the scenario of two complete years, with the same characteristics data. In previous and subsequent years, the variables were different and may not be compared. Twelve data mining techniques were applied, and the results were compared using some metrics to show which algorithms performed the best. As a result, it is expected to understand what are the main work accident factors that are related to the workers death.
2. Theoretical framework
2.1. Work accidents
The ILO estimates that, in 2017, expenditures linked to accidents, work-related illnesses and deaths totaled about $3 trillion, which represents about 4% of the world GDP 7,18,36. Work accidents can often result in injuries, illnesses and death, and there are numerous records of occupational accidents from a long time ago. OSH has become pivotal when it comes to ensuring workers' rights, thus, minimizing the risks faced by them in their work environment 19.
The term ‘Occupational Safety and Health’ is related to a set of legally required standards and procedures that aim to prevent accidents and occupational diseases, therefore, reducing risks for workers and, consequently, boosting the performance of organizations 24.
According to Law No 8,213, from 1991, Article 19, occupational accidents are the ones that happen to individuals while they are performing a work task assigned by their employer, resulting in bodily injury or functional disturbance that may lead to death, as well as permanent or temporary loss or reduction of their work capacity. They can be categorized as commuting accidents, typical accidents, and work-related illnesses 9.
Commuting accidents are those that take place even outside an individual’s workplace, and at times beyond their working hours, be it on their way from home to work or vice-versa. Occupational illnesses, in turn, are the result of working conditions that are harmful to individuals’ health. They can be caused, for instance, by accidental contamination 6.
A typical accident is any unforeseen and undesirable occurrence related to work 1. Death, apart from physical and emotional damage, is a possible consequence of work accidents, besides being the most serious type of occurrence 29.
Incidents related to the work environment must be recorded as soon as they occur. This is important for statistical control by federal agencies, in addition to ensuring support for workers through the National Social Security Institute (NSSI) 2,9. In the OSH area, Work Accident Communications (WAC) are one of the main sources of information and data on work accidents. WAC records cover the entire country and are an important material for dimensioning the work accidents issue. The data stored in the WAC system are clustered and updated every three months and made available on the Brazilian Federal Government's Open Database. This huge dataset makes it possible to distinguish the most frequent factors in the occurrences.
During 2020, the NSSI registered about 446.9 thousand work accidents in Brazil involving employees in standard employment relationships, a decrease of approximately 30% compared to 2019 33. Although statistics on fatal accidents at work keep being produced, studies evaluating workers’ deaths in Brazil are scarce, especially considering that most data refer only to insured workers 27.
2.2. Work accidents in the transport sector
Professional drivers are daily exposed to a variety of environmental stressors, which makes them more likely to have work accidents. According to a report by the World Health Organization (2018), road traffic killed 1.35 million people in 2018. Among the external causes that kill the most, traffic accidents occupy the first position. Traffic accidents are the most common external cause of death 24,37.
In 2020, 5,287 lives were lost in accidents on Brazilian federal highways that cost approximately R$ 10.22 billion. The car was the type of vehicle responsible for the highest number of accidents (44.2% of the total), followed by motorcycles (31.8%) and trucks (17.6%). And collisions were the most common type of accident 8.
The National Classification of Economic Activities (NCEA) places Road Freight Transport (Group 49.30) in Section H, which is composed of industrial companies that operate in Transport, Storage and Mailing. According to data from the 2017 Social Security Statistical Yearbook, Road Freight Transport (NCEA 2.0 H 49.30-2) is, on average per year, responsible for 55% of all work accidents comprising the statistics of motorized road vehicles involved in accidents 33.
Due to a progressively faster pace of work and increasingly strict demands, individuals end up performing tasks on the edge. In Brazil, the work of professional drivers is considered very dull due to a stressful routine. In addition, such professionals face adverse conditions and long working hours, not to mention that many of them end up making use of illicit drugs and are vulnerable to violence and urban adversities 25.
In Brazil, the payment based on distance covered or on-time deliveries can lead drivers to work for longer periods, which results in less time to take breaks and, thus, rest. Decreased sleep quality and long hours behind the steering wheel significantly contribute to the occurrence of accidents 12. So, accidents related to the transport sector are considered a public health issue, since it is a growing social problem that directly influences the quality of life of society in general.
2.3. Data mining
Due to technological advancements and the spread of the internet, the number of data generated has increased significantly. Big data is a concept whose most common contemporary meaning alludes to a massive set of stored data, which can be accessed on numerous devices. Therefore, a resource to deal with these large sets is data mining 38.
Data mining is an interdisciplinary sphere that combines machine learning, statistical analysis, visualization and identification of patterns and trends within certain data 5. It differs from statistical techniques in terms that, instead of checking hypothetical patterns, it uses the data themselves to find those patterns. According to Berson and Thearling (1999), even though approximately 5% of all relationships can be found by statistical methods, DM can discover other relationships previously unknown, that is, the remaining 95% 2. It can also be described as part of the process known as KKD (Knowledge Discovery in Databases), which is responsible for searching for patterns among a set of used information 11.
DM is often characterized by its ability to perform certain tasks, such as classification, association, clustering, description, and regression 14,16,20,35. In this sense, no technique is able to solve all data mining problems. Different methods serve different purposes, and each method has advantages and disadvantages. Familiarity with the techniques is necessary to make it easier to choose one of them according to the problems that have been presented.
3. Methodology
The study has an applied nature, since it seeks to generate knowledge and solutions aimed at OSH, using data and models already known. It has a quantitative approach, using data mining for further analysis. It is also descriptive, as it presents connections between the variables in the data set and the workers deaths. This research is divided in four stages: planning; data collection and preprocessing; application of mining techniques; and analysis of results.
In the planning stages, the theme was contextualized based on the literature about OSH, transport sector and data mining. The search in the literature in the area of OSH was not done in a systematic way, but rather seeking to understand the scenario of accidents in the transport sector and how data mining could support this process.
In the collection and preprocessing stage, the WAC dataset from NISS was used due to its national coverage, reliability and ease of access. The period analyzed comprised July/2018 to September/2020 and Microsoft Excel and Power BI software were used to support and pre-processing. The data were organized considering 25 attributes or variables. Also, a preliminary analysis showed the presence of duplicated information or information not relevant to the study, which allowed for a reduction of the number of attributes to be considered. 8 variables dependents related with the accident and 1 variable independent (the death) were selected to compose the model.
Within the period in question, 990,870 instances/records were computed. However, considering the focus of the research, only data corresponding to the transport sector were selected. For selection of data from the transport sector, the National Classification of Economic Activities (CNAE) variable was used as a basis. Accidents with CNAE code 4900 to 5399 were filtered. As a result, the dataset was reduced to 65,049 instances, which allowed for a more detailed analysis. Finally, 603 records out of the entire dataset indicated death.
By analyzing the dataset, it was possible to see that some attributes can be classified in many ways, which would increase the complexity and execution time of the algorithms used. In order to properly execute the mining techniques, all attributes in string format were categorized and adjusted into categorical format.
During the mining stage, death and non-death data were collated, which resulted in a balanced number of occurrences reported by 1,206 records, of which 50% had death as an outcome. The selection of no-death data was random. The balanced dataset was constructed so that the model could be trained, without generating a bias in the classification by the algorithm. In order to apply data mining, we used the Jupyter Notebook environment with models developed in the Python language.
The balanced dataset was processed with the following algorithms: Bagging, Extra Trees, Random Forest, Stacking, Voting, XGBoost, Decision Trees, K-Nearest Neighbors, Logistic Regression, Naive Bayes, Neural Networks and Support Vector Machine. Moreover, the results of the techniques were analyzed by using five metrics, namely Accuracy, Precision, Recall, F1 score and ROC/AUC curve.
4. Results and discussion
4.1. Data collection and preprocessing
The attributes chosen for the research were date of birth (age), sex, the first level of Brazilian Occupation Classification (BOC) according to the guidelines of the Ministry of Labor and Employment (MLE), location (state of the employer's city/town), death/no death, type of accident, affected part of the body, cause of the accident and nature of the injury. Workers’ age at the time of the accident is not part of the information listed by the WAC dataset. However, it can be calculated based on the victims’ date of birth and the day when the accidents took place.
4.2. Application of data mining techniques
To predict the occurrence of deaths, the ‘death/no death’ factor was chosen to be the dependent variable, while the others were used as independent variables. The balanced dataset was performed by using twelve techniques to apply the model. Six of them were ensemble (Bagging, Extra Trees, Random Forest, Stacking, Voting and XGBoost) and the other six non-ensemble (Decision Trees, K-Nearest Neighbors, Logistic Regression, Naive Bayes, Neural Networks and Support Vector Machine). The results of the techniques were compared based on accuracy, precision, recall, F1 score and ROC/AUC curve.
Through the train_test_split function of scikit-learn, the dataset was divided into 70% training and 30% testing. The training set was composed of 844 instances, while the test set had only 362. After characterization, the dataset was submitted to data mining techniques, which enabled us to analyze the results of the metrics and the execution time of each technique. Due to the large number of ‘no death’ occurrences, ten random sets were generated to be executed, and only the ‘not obtained’ data were altered. Table 1 highlights the results of the ten executed data sets and computational time of the data mining techniques.
Table 1 Results of the metrics and computational time of the techniques.
Technique | Accuracy | Precision | Recall | F1 score | ROC/ AUC | Time (s) |
---|---|---|---|---|---|---|
Ensemble | ||||||
ET | 0.882 | 0.876 | 0.890 | 0.883 | 0.941 | 6.122 |
RF | 0.876 | 0.879 | 0.879 | 0.879 | 0.943 | 5.930 |
XGB | 0.891 | 0.891 | 0.891 | 0.891 | 0.951 | 7.031 |
BA | 0.873 | 0.883 | 0.861 | 0.871 | 0.936 | 14.03 |
VO | 0.827 | 0.825 | 0.830 | 0.827 | 0.908 | 5.995 |
ST | 0.890 | 0.887 | 0.889 | 0.887 | 0.953 | 7.199 |
Non-ensemble | ||||||
SVM | 0.817 | 0.847 | 0.777 | 0.810 | 0.878 | 5.907 |
LR | 0.782 | 0.773 | 0.799 | 0.786 | 0.850 | 6.060 |
KNN | 0.821 | 0.825 | 0.808 | 0.816 | 0.874 | 5.919 |
NB | 0.891 | 0.866 | 0.919 | 0.892 | 0.951 | 5.762 |
DT | 0.845 | 0.852 | 0.846 | 0.848 | 0.850 | 5.923 |
NN | 0.814 | 0.832 | 0.797 | 0.812 | 0.893 | 7.273 |
Source: The authors.
It is possible to highlight that the techniques categorized as ensemble had better results in terms of accuracy, precision and ROC/AUC metrics. As for those listed as non-ensemble, they had better performance in recall metrics and F1 score, besides having the shortest computational time. Considering the ensemble techniques, XGBoost (XGB) achieved the best metric results, with the exception of ROC/AUC, whose best result was associated with Stacking (ST), and the shortest computational time was due to Random Forest (RF). Regarding the non-ensemble techniques, Naïve Bayes (NB) had the best metric results, including the shortest computational time.
5. Discussion
The metrics results were also analyzed based on graphs, configured for each of the five metrics in relation to the applied techniques. Figs 1 and 2 show the relationship between metrics and ensemble/non-ensemble techniques, respectively.
The analysis of accuracy, precision, recall and F1 score (Fig. 1) shows that the XGBoost technique stood out and had the highest performance. Only for the ROC/AUC metric was there a different result, since the stacking technique achieved better results. Although the XGBoost technique stood out in most scenarios, the results were very close for all metrics, with the exception of voting, which had lower values in all cases. It is also possible to notice that the percentages of the ROC/AUC metric were higher than those of other metrics. As for non-ensemble algorithms, the Naïve Bayes technique stood out (Fig. 2).
In order to improve the performance analysis of the metrics, the techniques with the best results, XGBoost (XGB) and Naïve Bayes (NB), were selected for the explanation stage with an artificial intelligence algorithm, the SHapley Additive exPlanations (SHAP).
The results of the ensemble technique, XGBoost (XGB) (Fig. 3A: XGBoost) and the non-ensemble technique Naïve Bayes (NB) (Taure 3B: Naïve Bayes) at the explanation stage indicate that the nature of injury (fractures, multiple lesions, crushing etc.) is the most relevant factor for predicting death. Work accidents whose outcome is death are also explained by the part of the body affected. That is, work accidents in road transport commonly result from frontal collisions of motorized road vehicles (cause) with a great impact, causing serious injuries in areas of the body such as head and trunk, as well as systems and apparatuses (part of the body affected).
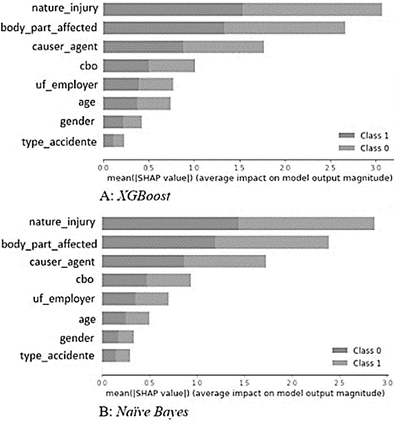
Source: The authors.
Figure 3 Categories of attributes and their relevance for the XGBoost and Naïve Bayes results.
On the other hand, sex, type of accident (typical, on the road or illness), state where the company is based, age and BOC are not significant attributes for predicting deaths in the transport sector or characterizing the incidence of occupational accidents in the aforementioned sector, as seen in Fig. 3A: XGBoost and Fig. 3B: NB.
6. Conclusion
In this research, in order to predict deaths in the transport sector, 12 data mining techniques were applied to a set of 1,206 occupational accident records, available by the WAC database. The study relied on data partitioning, where 70% referred to training and 30% to testing. The algorithms XGBoost (ensemble group) and Naïve Bayes (non-ensemble group). were identified with the best performance based on their results regarding accuracy, precision, recall, F1 score and ROC/AUC metrics.
The results determine the attributes that have greatest influence on the notification of work accidents followed by death. This information can help employers to implement more effective prevention programs aiming to reduce accidents in the transport sector. In addition, these attributes can assist assemblers in the development of motorized road vehicles, focusing on drivers’ safety. And the use of data mining techniques linked to occupational safety and health data results in information able to support decision-making in both public and private organizations.
The main limitation faced by this study has to do with the data used, since some variables in the selected dataset did not present values, their categories could not be identified. A model comprising a greater number of variables would probably present more accurate results. Therefore, a suggestion for future studies is the use of data mining techniques to compose the explanation stage with another artificial intelligence algorithm