1. Introduction
Nowadays, energy access continues to be limited, with 759 million people worldwide still without access to electricity and 2.6 billion continuing to rely on polluting and unhealthy energy sources for cooking [1], the majority in rural communities [2]. This lack of energy access has worsened with the current Covid-19 pandemic, which has stalled various investment projects in the global energy sector, once again moving humanity away from meeting the goal of ensuring energy access for all [3]. Before the pandemic, significant advances in energy access had been made, linked to developing new generators and the efficient use of energy resources. These advances were achieved through the implementation of different energy systems which seek to be sustainable and generate the most negligible environmental impact, among which the following stand out:
Microgrids have been presented as a solution for electrification in isolated systems using non-conventional energy sources [4]. One such case is [5], where a methodology for the design of a rural microgrid was developed and validated with data from 10 rural communities in Nigeria. Similarly, in [6], the design of a rural microgrid is examined, integrating a methodology for demand estimation. Another alternative for rural microgrids is presented in [7], where a microgrid model is proposed for the electrification of a rural bank.
Cogeneration can be achieved using renewable and non-renewable energy sources or directly using a renewable energy source such as biomass. An example is Pakistan, which uses sugarcane bagasse (leftover cane pulp) in cogeneration processes, contributing to the sustainable development of the population, a third of which is located in rural areas [8]. Similarly, in sub-Saharan Africa, wood processing residue is used for cogeneration processes to produce energy for both rural and urban areas [9]. A further case study can be found in Jamaica [10], where, as in [8], sugarcane bagasse is used in cogeneration processes.
Trigeneration uses fuel for power generation taking advantage of the hot exhaust gases to produce cool and heat [11]. As with cogeneration, energies such as biomass can be used, generating not only heat and electricity but also cooling in Non-Interconnected Zones (NIZ), as shown in [12]. Other studies, such as [13], show the planning of trigeneration systems for agriculture in greenhouses located in remote areas.
Multi-energy systems (MES) address the challenges of energy provision in a global manner, integrating different energy vectors and improving the use of the primary energy source [14]. These systems are gaining ground as a solution to the energy supply issues in industrial areas [15] and are also considered a potential solution for NIZ [16].
With the implementation of MES, challenges emerge in the search for optimal operation of these complex systems so that they function adequately [16]. That is why, in the first instance, they need to be planned optimally to ensure that the system meets the requirements expected.
For this, the use of optimization tools that enable the adequate planning of these systems is vital to ensure they are a solution to the challenges of energy provision. Thus, [17] presents a mixed integer linear programming model for planning a generic structure for intelligent multi-energy systems. Similarly, the work carried out in [18] presents two of the most common ways to plan in an MES: i) Using existing systems and ii) Planning from scratch. In this case study, two-stage mixed integer linear programming is used to optimize the MES configuration, and the distributed renewable energy sources are considered.
Other studies, such as [19], show the planning of an MES prioritizing the use of thermal storage in the heating network and the heat load. For the planning, an optimization model with mixed integer linear programming is proposed with cost reduction as the objective. Similarly, in [20], a mixed-integer linear programming model is developed for an MES with long-term energy storage. Additionally, in [21], an integral nonlinear mixed integer programming model is used to determine the best combination of the generators to satisfy the overall energy demands of a set of buildings, seeking to prioritize using clean and renewable energy.
The research related to MES planning is mainly focused on urban [22] and industrial areas [15], which are connected to conventional energy grids and do not take into account the concept of distributed energy systems [23]. Similarly, the MES described above does not take advantage of the full energy potential available, which for NIZ is primarily renewable [24].
Based on the studies presented above, this paper proposes a new approach for MES planning in NIZ, with the following contributions: i) Proposal of a methodology with an optimal multi-objective approach for MES planning in isolated areas, sizing their integral energy resources; ii) A comparative analysis performed with an energy solution based on the planning of an electric microgrid; iii) The reduction of economic costs and pollutant emissions positioned as key objectives. Considering distributed energy systems as an alternative solution to the problems of energy provision in NIZ. The proposed scheme uses of local existing renewable energies available in order to ensure the proper functioning of the system.
In addition, the planning process is carried out utilizing an operation time of 30 years in such a way that it is possible to observe how the system to be implemented will behave and thus plan it optimally. Therefore, a multi-objective particle swarm optimization algorithm (MOPSO) is implemented. The generators used are solar panels (PV), wind turbines (WT), batteries (BT), biogas generator (GB), diesel generator (GD), geothermal pump (BC), absorption chillers (CA), solar collector (ST).
At the end of the process, a range of optimal options emerges, giving the decision-maker the o opportunity to prioritize cost or emission minimization according to their specific needs. In addition, solutions can be selected using the sum of expert criteria that align with the planners' interests.
The next part of this paper is organized as follows:
Section 2 presents the proposed general model and the mathematical representation. Section 3 describes the optimization model. Section 4 presents the application of the model in a case -study. Finally, Section 5 presents some key conclusions from the model presented.
2. Proposed methodology
The MES planning model is implemented, considering the operation. The first stage is a site characterization, where it is necessary to determine the energy demand, consisting of electricity, cooling, and heating loads. As well as determining the quantity of primary energy resources available and their types, such as irradiance levels at the site, wind speed, and biomass quantities, it is also important to identify the energy sources which can be brought to the site, such as diesel, natural gas or LPG. In the second stage, the generators to be implemented are defined depending on the available energy resources and the energy demand to be met. The mathematical models of the generation assets are then described. In the third stage, the objective functions are optimized, and their mathematical models are defined. In the fourth stage, given the characteristics of the multi-energy system, a multi-objective MOPSO algorithm is proposed to optimize the objective functions. These functions, which by their nature are contrary to each other and are in different dimensions, as is the case of costs and emissions. Finally, a Pareto frontier is obtained with different results to guarantee a range of solutions. These are optimal while respecting the dimensions of each objective function, finding a vector of decision variables that complies with the restrictions imposed, and optimizes the vector function. Fig. 1 shows the proposed methodology.
2.1Mathematical models considered for the MES planning
For the planning of the system, the mathematical models of the generators and the mathematical models of the objective functions will be presented.
2.1.1 Mathematical models of generators
The power generation models for both conventional sources and renewable systems are presented. The models used in this paper prioritize the use of renewable energies as the main generators, having photovoltaic generators, biogas generators, wind generators, solar thermal generators, low enthalpy geothermal generators, and cold generators; in addition, a non-renewable diesel generator is used as a backup for the system, as well as a battery system.
Photovoltaic generator model
Eq. 1 is used for calculating the electrical power generated.
Where G(t) is the hourly solar irradiance, A PV is the area of the PV module, and ηPV and ηPV is the efficiency of the PV module and the DC/AC converter.
Solar collector model
The thermal power generated by the solar collector was calculated using Eq. (2).
Where AST is the solar collector area, n0 is the optical efficiency, G(t) is the hourly solar irradiance, a1 is the heat loss coefficient, Tc is the average temperature, and Ta is the ambient temperature.
Diesel generator model
Eq. (3) shows the calculation of electrical power generated.
Where ηeGD is the electrical efficiency of the generator, αGD is the lower heating power of the diesel, and FGD is the amount of fuel consumed by the generator each hour.
Eq. (4) calculates the thermal power of the generator.
Where ηtGD is the thermal efficiency of the generator, αGD is the lower calorific value of the biogas, and F GD is the amount of fuel consumed by the generator every hour.
Geothermal heat pump model
The following Eq. is the calculation of the generated thermal power:
Where QCBC is the thermal power delivered by the heat pump, EER is the coefficient of performance for the said pump, and Pe is the electrical power consumed by the heat pump to heat or cool, depending on its operating model.
Absorption chiller model
The following Eq. calculates the thermal power delivered by the absorption chiller.
Where QFCA is the thermal power that the absorption chiller can deliver, QCCA is the amount of heat required by the chiller to deliver that power, and EER CA is the coefficient of performance for the chiller.
Wind turbine model
The calculation of the electrical power delivered by the wind turbine is estimated using Eq. (7) and depends on the wind speed present in the generator's area.
Where P_WT is the generator output power, PR is the rated power of the wind generator, V is the wind speed present in the area, VR is the rated wind speed, VC is the lower wind cut-off speed, and VF is the upper wind cut-off speed of the wind turbine.
Battery model
The system of electrical storage with the charging and discharging of the battery is given by Eq. (8).
Where SOC is the state of charge of the battery, SOC0 is the initial charge level of the battery, n is the battery’s efficiency, C is its capacity, and is its nominal discharge current.
Biogas generator model
Eq. (9) is used to calculate the electrical power output of the biogas generator.
Where neGB is the electrical efficiency of the generator, ∝GB is the lower calorific value of the biogas, and FGB is the amount of fuel the generator consumes per hour.
Eq. (10) describes the thermal power output of the biogas generator.
Where ntGB is the thermal efficiency of the generator, ∝GB is the lower calorific value of the biogas, and FGB is the amount of fuel consumed by the generator per hour.
2.2 Mathematical models of the objective functions.
Once the models of the different generators have been described, It is essential to know the initial investment, operating, and maintenance costs, as well as the environmental impact of the operation, construction, and transport of the equipment to be installed in order to make the project economically viable and with the least environmental impact. Therefore, the functions to be minimized are as follows:
Initial investment costs
One of the components of the objective function is the project’s initial investment costs, which correspond to those incurred when acquiring the system’s assets. This cost is calculated according to Eq. (11).
Where noPV is the number of panels, CPV is the cost of these panels, including the inverter, noST is the number of solar collectors, CST is the cost of each solar collector, noWT is the number of wind turbines, CWT is the cost of each turbine, noGB represents the number of biogas generators, CGB is the cost of each biogas generator, noGB is the number of diesel generators, CGD is the cost of each diesel generator, no BT is the number of batteries, CBT is the cost of each battery, noCA is the number of absorption chillers, CCA is the cost of each chiller, noBC is the number of geothermal heat pumps, CBC is the cost of each heat pump.
Operating and maintenance costs
Operating and maintenance costs are derived from operating the system as planned. Operating costs are incurred from the moment the system is commissioned until the end of its useful life, and maintenance costs seek to ensure the proper functioning of the equipment that is operating so that it lasts for the stipulated period. The calculation of costs is as follows:
Where COMPV is the annual operating and maintenance cost of the panels, COMST is the annual maintenance cost of the solar collectors, COMWT is the annual operating and maintenance cost of the wind turbines, COMGB(t) is the annual operating and maintenance cost of the biogas generator, COMGD is the annual cost of the diesel generator, COMBT the annual operating and maintenance cost of the batteries, COMCA the annual operating and maintenance cost of the absorption chiller, COMBC is the annual operating and maintenance cost of the heat pump.
Considering both the initial investment costs and the operating and maintenance costs, the objective cost function is
Quantity of carbon emissions
To mitigate the environmental impact, the algorithm implemented minimizes the objective function of emissions, which is calculated in Eq. (14):
Where PPVmax is the maximum power that each solar panel can produce, EPV is the quantity of emissions produced in the construction phase of each solar panel, QSTmax is the maximum power output of the solar collector, and EST is the quantity of emissions produced in the construction of the solar collector.
3. Optimization model
Having identified the objective functions and restrictions for the planning of an MES in a NIZ identified, considering the thermal and electrical demands, the equipment and capital investment required, the operating and maintenance costs, and its environmental impact. This study proposes the use of a MOPSO algorithm which has been widely used and its effectiveness demonstrated in solving planning problems in the energy sector [25]. A MOPSO is a tool which, due to its high convergence speed, and good computational performance, demonstrates great flexibility and practicality in solving complex optimization problems that seek optimal solutions with multiple variables and objectives, objectives that are, by their nature, at odds with one another [26]. In the planning of MES in isolated areas, a MOPSO serves as a valuable support for decision making, presenting a range of optimal solutions which can be used to answer the planning problem.
Next, the structure of the optimization model is presented, with the proposed algorithm similar in structure to [27]. The algorithm begins by creating an initial population which complies with the established generator capacity constraints. This initial population is a combination of the different generators that can be installed. Once this is established, an optimal operation of the system that meets the energy demand is carried out. Once the system is planned and operated, the optimization process is carried out, and the resulting dominated solutions are eliminated. Subsequently, these nondominated optimal solutions are stored, and the number of iterations is verified before displaying the Pareto frontier that the decision-maker will use to plan the system. Fig. 2 presents the general optimization framework.
4. Case-study
In this section, the proposed optimization methodology is followed in order to verify its effectiveness in the planning of an MES in a NIZ. The zone is Bahía Málaga located in the Pacific region of Colombia in the department of Valle del Cauca. It is expected that this tool will help decision-making in order to improve the energization processes in places where the service is inefficient, or in some cases non-existent, or where it generates high levels of pollution due to the use of primarily fossil fuels.
4.1 Study area
In Colombia, approximately 500,000 users do not have access to electricity services [28], the energy required in many cases for refrigeration processes, lighting, and electronic equipment such as cell phones. Connecting populations in NIZ to an electricity supply is a key objective for the government, and different generation systems and various policies have been developed [28,29]. One of these populations is the Miramar Communitarian Counsel, a community of 165 inhabitants living in 34 houses, located in Bahía Málaga in the Colombian Pacific region. The community’s main economic activity is fishing, which has an energy consumption of 48kWh/day.
4.2 Architecture of the multi-energy system
A multi-energy microgrid is modeled, that given the energetic and geographic characteristics of the site has two cogenerators (diesel and biogas), two electrical generation systems (photovoltaic and wind), three thermal generation systems (solar collectors, geothermal heat pumps, and absorption chillers), and an electrical storage system (batteries), Fig. 3 illustrates the process of the multi-energy microgrid.
The input data of the system correspond to the site's electrical and thermal demands and the potential energy sources present in the area, including those that can be introduced to the site Fig. 4 shows the electrical load profile, the thermal load is constant (2kWh), Fig. 5 and Fig. 6 show the solar radiation and wind speed in each hour in a day respectively. The average daily energy demand considered in the study was 78.77 kWh/day, of which 48.0 kWh/day corresponds to fishing activities which require cooling, and the community in other activities consumes the remaining 30.77 kWh/day. The maximum expected electrical load at 19:00 hours is 5.46 kW, and the thermal load is constant during the day with a value of 2kW. The average daily solar radiation is 3.5 kWh/day, and the maximum daily solar radiation in summer is 4.0 kWh/square meter. The worst month regarding renewable energy availability has a maximum of 3.67 hours of sunshine. The average wind speed is between 1.9 m/s and 2.74 m/s.
Tables 1 and 2 below show the capital costs and the capacity of the assets installations.
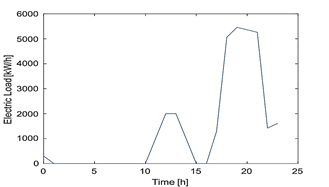
Source: Adapted from Martínez RE, Bravo EC, Morales WA, García-Racines JD. A 2020
Figure 4 Electrical load profile.
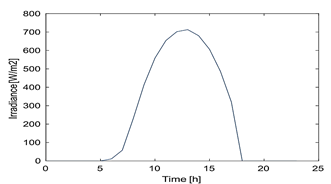
Source: Adapted from Martínez RE, Bravo EC, Morales WA, García-Racines JD. A 2020
Figure 5 Solar irradiance profile: Bahía Málaga.
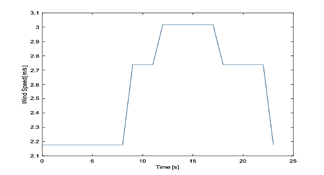
Source: Adapted from Martínez RE, Bravo EC, Morales WA, García-Racines JD. A 2020
Figure 6 Wind speed profile: Bahía Málaga
Table 1 Capital costs of assets energy installation
Name | Value | Unit | Description |
---|---|---|---|
CBC | 1200 | USD | Capital costs per BC |
CCA | 9000 | USD | Capital costs per CA |
CST | 550 | USD | Capital costs per ST |
CGD | 0 | USD | Capital costs per GD |
CPV | 279.89 | USD | Capital costs per PV |
CWT | 1261.68 | USD | Capital costs per WT |
CBT | 731.18 | USD | Capital costs per BT |
Source: Adapted from Martínez RE, Bravo EC, Morales WA, García-Racines JD. A 2020
Table 2 Capacity of assets energy installations
Name | Value | Unit | Description |
---|---|---|---|
QBC | 21 | kW/h | Maximum power of each heat pump |
QCA | 17,6 | kW/h | Maximum power of each absorption chiller |
QST | 2,497 | kW/h | Maximum power of each solar collector |
PGD | 10 | kW/h | Maximum power of each diesel generator |
PPV | 0,295 | kW/h | Maximum power of each solar panel |
PWT | 1 | KW/h | Maximum power of each wind turbine |
CBT | 2,49 | kW/h | Rated battery capacity |
Source: Adapted from Martínez RE, Bravo EC, Morales WA, García-Racines JD. A 2020
Results & Discussion
To solve the MES planning problem proposed in the case study, the MOPSO algorithm was executed with the parameters described above on a PC with Windows 10 operating system, with 8GB of RAM and a Core i7 3.6 GHz processor. MATLAB R2021B software was used to carry out the simulation. The control criteria were set as follows: number of particles = 50; number of iterations = 500; objectives = 2.
Solving the proposed multi-objective optimization problem yields the optimal Pareto frontier with 57 results, presented in Fig. 7. As previously discussed, multi-objective optimization provides a range of possibilities that will enable the decision-maker to select an optimal solution, depending, in this case, on the costs and emissions of the system to be installed. In addition, the planning considers the operating costs as a constraint in supplying both thermal and electrical demand, thus ensuring that the number of generators is the minimum possible.
As can be observed in Fig. 7, as costs increase, emissions decrease. The increase in cost is due to the use of renewable energy generators, which have an initial capital investment cost, unlike the diesel generator, which is considered to have zero initial investment cost for this case study because it is already installed in the zone.
In order to carry out a clearer analysis, the following five optimal solutions are chosen based on the Pareto frontier and the following criteria:
Lower costs
First solution with renewable energies
Average costs and emissions
Energization provision with renewables only.
Lower emissions
Solution 1: includes the existing diesel generator and an absorption chiller to take advantage of the waste heat from the combustion gases emitted from the generator and thus supply the thermal load. This is the most economical solution, but it is also the most polluting due to the use of the diesel generator as the only source of electricity production and the waste of energy that occurs when the cogeneration process is not carried out. Fig. 8 show this solution.
Although Solution 1 produces high emissions, they are still lower than those produced in a solely electric microgrid for an isolated area, such as the grid planned in [30]. Table 3 compares Solution 1 from [30] with Solution 1 proposed in this paper, using the same case study where, as mentioned, a 43.9% reduction in emissions is observed by implementing MES in the planning of energy solutions for isolated areas. In addition, investment and operating costs were also reduced by 87.2% when using these systems.
Table 3 Results comparison
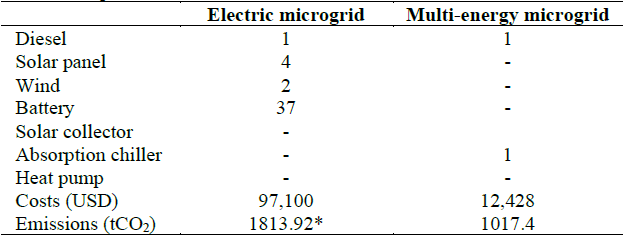
*The value reported by the authors in the results does not correspond to the methodology they propose. In this table, this value was modified according to the methodology.
Source: own elaboration
Solution 2: Also includes the use of the existing diesel generator as the only source of electrical energy, but this time accompanied by a geothermal heat pump. This combination of renewable and non-renewable energies results in 3% higher capital investment costs of 14,755 USD, but a 10% reduction in emissions, producing 1813.92 tons of CO2 compared to 1017.4 tons in the MES Solution 1. Fig. 9 show this solution.
This reduced environmental impact is because it is unnecessary to burn fuels to have a heat source as the heat is derived from the subsoil (shallow geothermal energy). This provides constant heat throughout the year, ensuring a robust cooling system in which 1kW of electricity can generate 5kW of cooling, supplying the site's demand.
Solution 3: proposes a greater presence of renewable energies (solar and shallow geothermal), which, along with diesel, share the electricity production. It is also important to note that the heat pump becomes an important feature of the solution in order to supply the cooling demand of the site because, as mentioned above, it has low power consumption, and the subsoil is its heat source. Fig. 10 show this solution.
In addition, the use of solar panels to generate electricity reduces emissions to 642.35 tonnes of CO2, which in turn reduces the fuel consumption of the diesel generator. However, despite the reduction in fuel consumption, the total investment cost increases.
Solution 4 shows an optimal alternative where the use of renewable energies predominates for both electrification and cooling. It is important at this point to note that these energies are present in the area, which is why they do not depend on external factors to reach the site, but the availability of irradiance may vary depending on weather conditions, hence the importance of battery use to ensure a constant energy supply. Fig. 11 show this solution.
This renewables-only solution is also a solution included in the planning of the electric microgrid mentioned above [30], where the electric microgrid has a 77.4% greater cost and 91.8% higher emissions than a multi-energy microgrid, as shown in Table 4.
Table 4 Results comparison
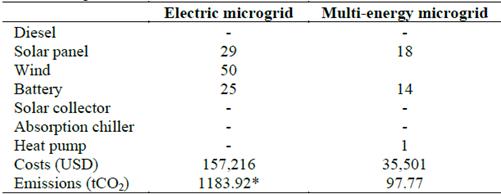
*The value reported by the authors in the results does not correspond to the methodology they propose. In this table, this value was modified according to the methodology.
Source: own elaboration
Solution 5: produces 90.6% tons of CO2, less than the alternatives to be installed according to the solutions given for the MES, but it is also 76.8% more expensive. Something similar happens with the microgrid where emissions are the lowest for this solution, but this time without having the highest costs, as demonstrated in Table 5. Despite this, the MES is still 84.24% less polluting and 49.3% more economical for the solutions shown in Table 5. Fig. 12 show this solution.
Table 5 Results comparison
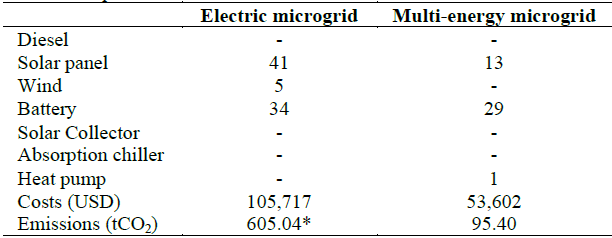
*The value reported by the authors in the results does not correspond to the methodology they propose. In this table, this value was modified according to the methodology.
Source: own elaboration
As renewables enter, batteries, by necessity, appear. There is also a null use of wind turbines by the multi-energy microgrid due to the low wind speeds present at the site.
Conclusions
The proposed model considered the use of a multi-objective optimization algorithm for the planning of an MES with two objectives: that it included more than two renewable energy sources, included a storage system, and also integrated a set period of operation. The system was designed so that the results could support an expert in making decisions regarding the installation of MES systems in NIZ, thus ensuring energy access to these communities.
The integrated use of multiple energy sources in a single system has great potential in NIZ if planned and operated adequately, as shown in this study. Most of these remote communities have been found to have sufficient renewable energy resources to make the energization process possible and to meet inhabitants’ energy demands if they are integrated into MES. Such integration would significantly reduce the use of conventional energy sources, which, in many cases, depend on fuel that is difficult and costly to deliver to such zones.
This methodology, compared to other similar methodologies, considers optimal planning and operation for the integral scaling of energy resources, including the use of three renewable energy sources, highlighting the use of shallow geothermal systems for air conditioning processes.
The results show that it is possible to provide energy access in a NIZ using only renewable energies and storage systems. The results also underline the importance of an accurate evaluation of the energy resources available at the site to avoid incurring unnecessary expenses that increase the costs and emissions associated with the system. For this reason, in this case, study, it was not advisable to use wind turbines given the low wind speeds.
In comparison with the reference case study, the MES shows better economic and emissions results. In addition, its installation requires less space, and, as per the traditional microgrid, it predominantly includes the use of renewable energies.