Services on Demand
Journal
Article
Indicators
-
Cited by SciELO
-
Access statistics
Related links
-
Cited by Google
-
Similars in SciELO
-
Similars in Google
Share
Revista Colombiana de Ciencias Químico - Farmacéuticas
Print version ISSN 0034-7418On-line version ISSN 1909-6356
Rev. colomb. cienc. quim. farm. vol.40 no.1 Bogotá Jan./June 2011
Artículo de Investigación Científica
A quantitative structure - toxicity relationship of drugs on rat
Una correlación cuantitativa entre la estructura molecular de fármacos y la toxicidad en ratas
Amir Separham1, Mohammad-Ali Eghbal2, Elnaz Tamizi3, Abolghasem Jouyban4*
1 Tuberculosis and Lung Disease Research Center, Tabriz University of Medical Sciences, Tabriz, Iran
2 Biotechnology Research Center, Tabriz University of Medical Sciences, Tabriz 51664, Iran
4 Drug Applied Research Center and Faculty of Pharmacy, Tabriz University of Medical Sciences, Tabriz 51664, Iran
* Corresponding author. E-mail: ajouyban@hotmail.com, Fax: +98 411 3363231.
Received: February 20, 2011 Accepted: March 22, 2011
SUMMARY
A quantitative structure toxicity relationship (QSTR) is proposed to correlate the toxicity of drugs on rat after intravenous administration. The computational descriptors of 319 drug molecules are calculated using HyperChem software and regressed against LD50 of drugs collected from the literature. Correlation coefficient (R), F value and average percentage deviation (APD) between calculated and experimental LD50 are used to evaluate the accuracy of the proposed QSTR model. The best QSTR model is:
LD50=-639.254+3.773SAA-4.786VOL-21.050HE-50.753log P-51.440REF+121.219POL+12.932MASS+0.011TE-95.494HOMO
N=319, R=0,748, F=43
where, SAA is surface area (approximate), VOL molar volume, HE hydration energy, log P is the logarithm of partition coefficient, REF molar refractivity, POL polarizability, MASS molecular weight, TE total energy and HOMO energy of the highest occupied molecular orbital. The APD of a number of drugs are very high and this resulted in high APD for the data set. These drugs include busulfan, calcitriol, epinephrine, triaziquone etc. and could be considered as outliers. After excluding these data points, the model is:
LD50=-740.217+4.050SAA-5.138VOL-21.909HE-50.713log P-49.662REF+120.843POL+12.742MASS+0.010TE-106.513HOMO
N=309, R=0,751, F=43
Small changes in the model constants showed that the model is robust and could be considered as a predictive model. Because of various toxic mechanisms, high discrepancy in reported LD50 of some drugs from different references, high APD value could be justified. As an example, LD50s of dixyrazine are 37.5 and 3.75 in different references. After excluding the outliers, APD reduces to 977%. The APD could be considered as acceptable over range if the experimental discrepancies between reported LD50 from different laboratories are kept in mind.
Key words: Quantitative structure-toxicity relationship, Rat, Drug, LD50
RESUMEN
En este trabajo se plantea una correlación cuantitativa entre la estructura molecular de fármacos y la toxicidad (QSTR) en ratas después de la administración intravenosa. Se calcularon los descriptores computacionales de la estructura molecular de 319 fármacos utilizando el programa HyperChem. Los descriptores computacionales fueron relacionados matemáticamente contra los respectivos valores LD50 tomados de la literatura. Para evaluar la precisión del modelo QSTR propuesto se utilizaron el coeficiente de correlación (R), el valor F y el porcentaje de desviación promedio (APD) entre los valores calculados y experimentales de LD50. El mejor modelo QSTR es:
LD50=-639.254+3.773SAA-4.786VOL-21.050HE-50.753log P-51.440REF+121.219POL+12.932MASS+0.011TE-95.494HOMO
N=319, R=0,748, F=43
Donde, SAA es el área superficial (aproximada), VOL es el volumen molar, HE es la energía de hidratación, log P es el logaritmo del coeficiente de reparto, REF es la refractividad molar, POL es la polarizabilidad, MASS es la masa molar, TE es la energía total y HOMO es la energía del orbital molecular más altamente ocupado. El valor de APD de un cierto número de fármacos es muy alto y esto incidió en un alto valor de APD para el total de compuestos estudiados. Estos fármacos incluyeron busulfán, calcitriol, epinefrina, triaziquona, entre otros, por lo que fueron considerados como descartables. Después de excluir estos puntos, el modelo obtenido es:
LD50=-740.217+4.050SAA-5.138VOL-21.909HE-50.713log P-49.662REF+120.843POL+12.742MASS+0.010TE-106.513HOMO
N=309, R=0,751, F=43
Con algunos pequeños cambios en las constantes el modelo demostró ser robusto y por lo tanto se consideró como altamente predictivo. El alto valor de APD obtenido podría justificarse gracias a los diferentes mecanismos de toxicidad y a la gran dispersión de los valores de LD50 presentados en la literatura. Como ejemplo podría citarse el caso de la dixirazina, para la cual se han reportado valores de LD50 de 37,5 y 3,75 según las diferentes fuentes bibliográficas. Después de excluir esos compuestos, el valor APD se reduce a 977%, el cual podría considerarse como aceptable si se tienen en cuenta las discrepancias experimentales encontradas en los valores de LD50 que han sido reportados por diferentes laboratorios.
Palabras clave: Correlaciones cuantitativas estructura-toxicidad, Ratas, Fármacos, LD50.
INTRODUCTION
The first step in the development of a new drug is the synthesis of a potential new drug molecule or extraction of a candidate chemical compound from natural sources. The safety and efficacy of a drug have to be investigated before its marketing. These investigations start from in vitro studies and continue for most of the biologic effects of the molecule which should be characterized in animal studies before human drug trials. Human testing must then go forward in three conventional phases before the drug is considered for final approval of marketing. A fourth phase of data gathering follows after approval for general use.
Enormous costs, from 100 million to over 500 million USD, are involved in the development of a successful new drug. These costs are spent for searching new molecules and a huge number of candidates (5000-10000) may be synthesized for each successful drug, basic and clinical studies and promotion of the ultimate candidate molecule. It is primarily because of the economic investment and risks involved that most of the new drugs are now developed in the laboratories of pharmaceutical companies. At the same time, the incentives to succeed in drug development are equally enormous (1).
It is important to recognize the limitations of preclinical testing. These include the following: (A) Toxicity testing is time-consuming and expensive. During the last decade, the total cost of preclinical pharmacology and toxicology studies was estimated to be at least 41 million USD per a successful drug and 2-5 years to collect and analyze data. (B) Large numbers of animals are used to obtain preclinical data and there are limitations for animal studies in some societies. Scientists are properly concerned about this situation, and progress has been made toward reducing the number of animals required while still obtaining valid data. In vitro methods such as cell and tissue cultures are increasingly being used, but their predictive capacity is still severely limited. (C) Extrapolation of toxicity data from animals to humans is not completely reliable. For any given compound, the total toxicity data from all species have a very high predictive value for it's toxicity in human. However, there are limitations on the extent of the available databases. (D) For statistical reasons, rare adverse effects are unlikely to be detected, just as in clinical trials (2).
Finding an active compound with maximal pharmacologic effects and minimal adverse and toxic effects is the most important goal of drug development investigations. Different mechanisms are involved in the toxicity of a compound and the tools on understanding such a complex phenomena are still very limited. Although different mechanisms are effective and different receptors are involved in toxic responses, but the outcomes of toxicity are death; disability and/or mutations.
The severe adverse and toxic effects result in decreasing the chance of further progress of introduction of the new drugs. In addition to the experimental investigations, the computational methods could also be employed in order to determining the safety and efficacy of the new candidates. By considering that drugs toxic effects result from their physico-chemical reactions with biological systems, basically, there must exist a relationship between physico-chemical characteristics of drugs and their toxic effects.
This relationship could be determined for the kinetic or dynamic effects of physicochemical properties of drugs. Statistical analyses of drug data, for correlating toxicity with chemical structure and proposing mathematical models with ability of toxicity prediction, makes the drug discovery investigations faster and more cost-effective. These models could be applied for predicting toxicity of new compounds based on their structures (3).
Quantitative structure - activity (QSARs) and quantitative structure - toxicity (QSTRs) relationships have provided a valuable approach in research into the toxicity of organic chemicals in such studies (4).
One and two parameter QSTR equations were obtained to describe the cytotoxicity of isolated rat hepatocytes induced by 23 catechols in which LD50 represents the catechol concentration required to induce 50% toxicity after 2 h incubation. The reported QSTR equation was:<

where log P represents the logarithm of octanol/water partition coefficient.
Excluding three catechols from the original data set improved the QSTR equation between the catechols log LD50 (µM, 2h) and their log P values. The presence of outlirers implies that factors or specific mechanisms other than log P are involved in their cytotoxicity. Therefore, they obtained the two-parameter QSTR equation in order to describe the correlation between the cytotoxicity of the various catechols, their lipophilicity (log P) and their degree of ionization (pKa1) towards isolated rat hepatocytes:

Moridani et al. (5) excluded one catechol from the data set and improved the QSTR equation between the catechols log LD50 (µM, 2h), and physico-chemical properties log P and pKa1 values. QSTR models can be used as a powerful tool for understanding the toxic mechanisms that may be common among a group of similar compounds such as catechols. This is possible by first deriving the QSTR equations correlating different physico-chemical parameters to catechol toxicity. Then these QSTR equations can be applied to other biological endpoints that contribute to the toxic mechanisms; i.e., transport, metabolism, and target damage, so as to determine which endpoint is affected by the same catechol physico-chemical parameters that contribute to the toxicity. This information would be valuable to a research group that uses catechol functional groups for drug design and the optimization of a catechol molecule for a desired physico-chemical characteristic (5).
In another investigation on LD100 of some drugs in human subjects, the following relation was reported,
LD100 data of drugs were collected by measuring blood concentration of drugs obtained from the suicides victims. In Eq. 3 NVE is the number of valence electrons. To further improve Eq. 3, the toxicity data of 41 different drugs were applied and Eq. 4 was reported:


In this model, five drugs were considered as outliers and removed to improve the QSTR model between LD100, NVE and log P. Drugs with Cl and Br substitutions fit well with this equation. In two recent models log P is one of the parameters that is determining the absorption of drugs from gastrointestinal tract and also drug penetration to the CNS (6).
The aim of the present study is to provide a model for prediction of drug toxicity based on acute toxicity data (LD50) on rats and extend this model for toxicity evaluation of new drug candidates.
MATERIALS AND METHODS
Toxicity data (LD50 mg/kg) of drugs (N=319) on rat after intravenous administration were collected from the literature (7-9). Then physico-chemical descriptors of the molecules were calculated using HyperChem® software (10). These descriptors were: surface area approximate (SAA), molecular weight (MASS), molar volume (VOL), total energy (TE), hydration energy (HE), molar refractivity (REF), logarithm of partition coefficient (log P), polarizability (POL), dipole moment (DM) and energy of the highest occupied molecular orbital (HOMO).
Details of the collected data including the drug name, LD50 values and the calculated descriptors are listed in Table 1. LD50 was used as dependent variable and other descriptors as independent variables and the best equation with consideration of correlation coefficient (R), percentage deviation (PD), F value and average percentage deviation (APD) was developed. The PD and APD were computed by Eqs. 5 and 6, respectively.


where, N is the number of data points. All calculations were carried out in SPSS (11) environment using the written programs through this project.

RESULTS AND DISCUSSION
Preliminary analysis was carried out employing all independent variables by backward regression command of SPSS software. The resulting equation was:
The above equation with applying 319 experimental data of different drugs was resulted. Relatively high correlation coefficient (R) and F value with the significance level of < 0.0005 reveal that, the model is able to fit the toxicity data of drugs on rat after intravenous administration. APD for some data points for Eq. 7 was high because of existence of the outliers which were listed in Table 2. After excluding the outlier data points, the resulting equation was:

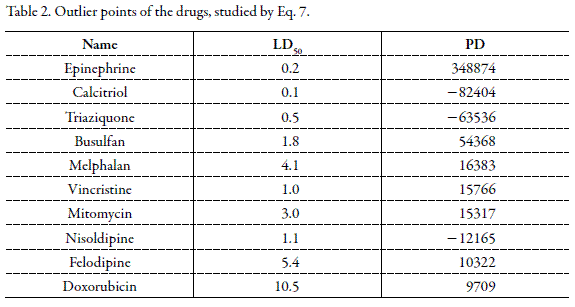
For providing practical application of Eq. 8, Hodge and Sterner scale was applied. Based on this classification, drugs are divided into six groups from highly toxic to harmless (12). Table 3 shows the APD values for different groups and number of data in each group. Considering Table 3, with increasing toxicity of the drugs, predictive ability of the model is decreased. From statistical viewpoint, this limitation results from two factors: (A) Number of data in high toxic group is very small and (B) Effect of very small numerical values of high toxic drugs on the total LD50 mean is negligible and lead to the reduced accuracy of the equation.

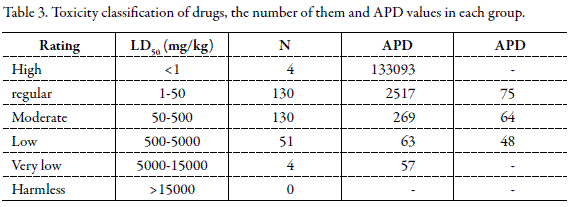
In another numerical analysis, the proposed equation was trained by data of groups with higher than 50 data points (N>50) and then, the APD values were calculated and reported in Table 3. As shown, the overall APD reduces to ∼ 60 %, however, one should be able to detect the class of the candidate concerning Hodge and Sterner classification and then to use this prediction method.
Any error in measuring or reporting the experimental toxicity data is another source of prediction error. In some cases, the numerical difference of the reported LD50 for a single drug, on a given animal and a common administration route is about 1500 folds. For example, LD50 of benzyl benzoate in rat after oral administration is 1.7 mg/Kg (7) and 2800 mg/Kg (9). The PD between two experimentally reported data is equal to 164600 %. Table 4 shows some of different data for LD50 of drugs in rat after intravenous administration.
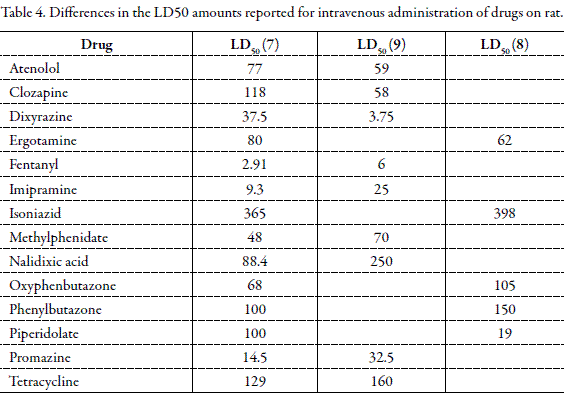
As a conclusion, a quantitative structure toxicity relationship (QSTR) was proposed to correlate toxicity of drugs on rat. The proposed model is robust and could be considered as a predictive model. Because of various toxic mechanisms, high discrepancy in reported LD50 of some of drugs from different references, high APD value could be justified. After excluding the outliers, APD reduces to 977%. The APD could be considered as acceptable error range if the experimental discrepancies between reported LD50 from different laboratories are kept in mind.
REFERENCES
1. S.A. Billstein, How the pharmaceutical industry brings an antibiotic drug to market in the United States, Antimicrob. Agents Chemother., 38, 2679 (1994). [ Links ]
2. B.G. Katzung, "Basic and Clinical Pharmacology." McGraw-Hill Publishing Company, New York, 2004, p. 67. [ Links ]
3. J Feng, L. Lurati, H. Ouyang, T. Robinson, Y. Wang, S. Yuan and S. S. Young, Predictive toxicology: Benchmarking molecular descriptors and statistical method, J. Chem. Inf. Comput. Sci., 43, 1463 (2003). [ Links ]
4. P.V. Khadikar, K.C. Mather, S. Singh, A. Phadnis, A. Shrivastava and M. Mandaloi, Study on Quantitative Structure - Toxicity Relationship of benzene derivatives acting by narcosis, Bioorg. Med. Chem., 10, 1761 (2002). [ Links ]
5. M.Y. Moridani, A. Siraki, T. Chevaldina, H. Scobie and P.J. O'Brien, Quantitative Structure Toxicity Relationship for catechols in isolated hepatocytes, Chem. Biol. Interact., 147, 297 (2004). [ Links ]
6. C. Hansch and A. Kurup, QSAR of chemical polarizability and nerve toxicity. 2. J. Chem. Inf. Comput. Sci., 43, 1647 (2003). [ Links ]
7. A. Kleemann, J. Engel, B. Kutscher and D. Reichert, "Pharmaceutical Substances", Thieme, Stuttgart, 2001. [ Links ]
8. I. Sunshine, Physical-toxicological and analytical data. In "Handbook of Analytical Toxicology", Edited by I. Sunshine, Chemical Rubber Co., Cleveland, 1969, pp.1-123. [ Links ]
9. I.S. Rossoff, "Encyclopedia of Clinical Toxicology: A Comprehensive Guide and Reference", Parthenon Publishing Group, Boca Raton, 2002. [ Links ]
10. HyperChem 7.0, Molecular mechanics and quantum chemical calculation package, HyperCube Inc., Ontario, 2002. [ Links ]
11. SPSS 11.5, Statistical Package for the Social Sciences, SPSS Inc. Chicago, 2001. [ Links ]
12. J. G. Carbonell and J. Siekmann, "Knowledge Based Intelligent Information and Engineering Systems", Springer-Verlag Berlin, Heidelberg, New York, 2008, Vol. 1, p. 570. [ Links ]