INTRODUCTION
The loss of homeostasis causes pathophysiological imbalances that alter morbidity rates during all stages of lactation in cows selected for milk production (Martens, 2020). Contributions have been made from different areas of knowledge to the understanding of physiological changes in order to propose alternative solutions and thus, improve the health and profitability of livestock systems (McGuffey, 2017).
The transition period corresponds to a phase of deep physiological changes during peripartum in dairy cattle, which normally occurs between the last three weeks of gestation and the first three weeks postpartum (Martens, 2020). This period is important because the success of lactation and the resumption of reproductive activity depend on it. Several types of research have been conducted to determine the diagnostic or predictive value of metabolic or hematic components in dairy cows (Kim et al., 2020) to understand the effects on homeostasis. In tropical conditions, animals are exposed to stressors (presence of hemoparasites, low nutritional quality forages, adverse environmental conditions that make a difficult adaptation) that cause chronic stress, thus generating individual metabolic or hematic responses and making difficult their integral analysis.
Cellular components of blood tissue can be influenced by several factors such as health events, age, parity, days to calving, milk production, and season of the year (Cozzi et al., 2011). These factors should be considered to establish metabolic disease criteria or to determine physiological homeostasis state.
In tropical conditions, water balance handling is a constant challenge for livestock, but especially for dairy cows due to the high and permanent water excretion through milk secretion. Hematocrit (Packed Cell Volume, or PCV) has been proposed as a tool to evaluate the potential response to caloric stress in cattle (Sejian et al. 2018) and its determination constitutes a simple, economical, and rapid method to directly estimate the degree of animal dehydration thus allowing a timely response to avoid negative effects on productive processes. Likewise, the determination of hemoglobin concentration provides information to discern, together with hematocrit, possible clinical situations of anemia, whose incidence is high in the peripartum period in grazing cows under tropic conditions, in some cases with the presence of hemotropic agents (Florio-Luis et al., 2012).
Despite the existence of evidence on the importance of the immune response in the transition period, partly related to the polymorphonuclear cellular components associated with it (Trevisi & Minuti, 2018), this is not yet clear for dairy cattle in the tropics, for the effect of modifications in the hematic and metabolic profiles associated with the breed type and the time of the year (De Vasconcelos et al., 2020).
The analytical study of physiological, environmental or genetic variations, could be done through multivariate statistics methods that allow to model the relationships between several variables simultaneously and perform exploratory analyses without the fulfillment of initial assumptions (cluster or principal components). Other techniques make possible to evaluate the effect of one or more factors on a set of dependent variables such as multivariate analysis of variance (MANOVA). However, in this case, the set of variables or the residuals obtained by the model must follow an exact or approximate normal distribution (Di Rienzo, et al., 2017; Oppong & Agbedra, 2016). Machine Learning models also provide a useful tool for variables prediction in multiple areas of knowledge. In this case, predictive models can be developed without prior knowledge of the relationships between variables, allowing non-normal distributed variables to be included in the analyses (Van der Heide et al., 2019).
This work was conducted aiming to analyze different statistical tools to determine which components of the production systems have the most significant influence on hematic parameters of dairy cows grazing in low tropical conditions and to propose alternatives for the identification of factors that allow inferring the state of hematic dynamics.
MATERIALS AND METHODS
The use of experimental animals was institutionally approved by the animal research ethics committee at Universidad Nacional de Colombia (CP-FCA 2018). Data from six experiments conducted during close up dry cow, the transition period and the first lactation phase in adult cows were analyzed. The cows were of different breeds and managed in various low tropic conditions between 3'30 to 4'10 N and 76'21 to 76'46 W, corresponding to a tropical dry forest zone according to Holdridge’s life zones classification (Holdridge, 1967).
Table 1 shows the breed group analyzed and the type of grazing system. Intensive systems presented the highest milk production, followed by semi-intensive systems and the lowest production was for the silvopastoral system. The breed groups were classified: 1, Multiracial with Holstein base and Gyr (F1 of Gyr for Holstein); 2, 100% Holstein and 3, Lucerna (synthetic breed -33% Shorthorn, 33% red Holstein and 33% Hartón del Valle.
Table 1 Breed and type of grazing in the systems analyzed.
System | Breed | Milk production level | Grazing type |
1 | Holstein x Jersey x Gyr | Medium | Semi-intensive |
2 | Holstein | High | Intensive |
3 | Holstein x Gyr | Medium | Semi-intensive |
4 | Holstein x Gyr | Medium | Semi-intensive |
5 | Holstein x Brown Swiss x Gyr | High | Intensive |
6 | Lucerna | Low | Silvopastoral |
In general, the cattle that were part of the analysis were managed in rotational grazing based on star grass Cynodon plectostachyus. In the intensive systems, grazing was carried out most of the day and 18% protein concentrate was used as supplement, at a rate of one kilogram of concentrate per four liters of milk. In the semi-intensive systems, with more spaced rotations in time, so it is likely that forage was presented a higher percentage of Neutral Detergent Fiber (NDF) and low protein content, it was supplemented at a rate of one kilogram of concentrate (16% protein) per five liters of milk.
The silvopastoral system had a similar forage base but associated with leucaena (Leucaena leucochepala), with a supplementation based on rice bran (Oriza sativa) and wheat fiber residue (Triticum vulgare L) at a rate of one kilogram per six liters of milk. The average milk production was 12.5 liters/day, ranging from 9 to 20 liters/day in all systems. It was classified as high production when the adjusted average per lactation for the system exceeded 4600 liters (intensive system), medium production in the interval between 4599-3700 liters (semi-intensive systems) and low production (silvopastoral system) when lactations below 3700 liters were recorded.
For the study of hematological variables, blood samples were obtained by coccygeal venipuncture through a vacutainer system and vacuum tubes with Tetra sodium Ethilene Diamine Tetraacetate (EDTA) as anticoagulant, and without this. The blood samples with anticoagulant were used to perform blood smear and determine hematocrit. Initially, the EDTA tube was shaken gently for one minute, then samples were transported refrigerated to the laboratory where hematocrit analysis was performed by the microcentrifugation technique and the blood was smeared and stained with Wright's dye and buffer solution. The manual count for identification and quantification of white blood cells (neutrophils, lymphocytes, monocytes, eosinophils, and basophils) was performed by microscopic reading at 100X. Hemoglobin was determined using the cyano-methemoglobin technique with commercial reagent IHL (Cali, Colombia) and reading it in a semi-automatic equipment. The serum samples were used to analyze non-esterified fatty acids (NEFA) and total proteins (TP) by colorimetric enzymatic tests with commercial RANDOX reagents (Crumlin, UK) in a semiautomatic RAYTO® chemistry equipment (Shenzhen, China).
The information from six experimental processes was collected to establish the analysis database was considered. In this way, and after removing atypical values, 415 records were obtained from 48 animals, from which a total of 10 blood samples were collected. The collection periods were two in prepartum (30 and 15 days before calving) and eight after calving, with a sample on the second day postpartum at 15 days intervals until day 105 postpartum.
Statistical analysis. In a first phase, each variable in the database was box-plotted to identify and eliminate outliers in physiological data. To reduce data variation produced by the effect of including information from different experiments, a hierarchical cluster analysis was carried out by sampling period (n=1-10) to group the periods in which the hematological variables presented similar behaviors during peripartum.
In the analysis the variables were standardized, and the average linkage classification algorithm and Euclidean distance were used. The number of clusters formed was determined to guarantee the presence of more than 20 animals per cluster and by the biological behavior associated with the transition period and first lactation phase. Subsequently, descriptive statistics were estimated within each cluster.
The behavior of hematological variables between clusters was evaluated simultaneously through a multivariate analysis of variance, which considered the effect of clustering as a classification factor. Histograms were made to evaluate the normality of the variables, and those that presented a different behavior were transformed to a logarithm or square root to guarantee an approximately normal distribution (Oppong & Agbedra, 2016). The Wilks, Pillai, Lawley-Hotelling and Roy statistics were established as significance criteria. Hotelling’s rule (P<0.05) was used for means comparison of the linear combination of hematological variables. Similarly, Spearman´s rank correlations were estimated between variables and were considered significant at P < 0.05. The analyses were carried out with Infostat software (Di Rienzo et al., 2017).
To establish models that allow to estimate the hematocrit and hemoglobin levels from other hematological variables, classification analyses were performed using the Random Forest algorithm. For this purpose, hematocrit and hemoglobin variables were categorized according to "normal" values (hematocrit range between 24 and 37%; hemoglobin range between 8 and 15 mg/dL) and "abnormal" values (hematocrit < 24 to >37; hemoglobin <8 to >15) as reported for cattle in tropical conditions (De Vasconcelos et al., 2020). The hematological variables used to estimate hematocrit levels were Non-Esterified Fatty Acids (NEFA), hemoglobin, TP, neutrophils, lymphocytes, monocytes, eosinophils and basophils. In the estimation of hemoglobin levels, the variables used were the same, except that the hematocrit variable was added to the predictors.
The Random Forest algorithm creates a set of decision trees where each one predicts the categorical variable to finally indicate which classification was the most common. Each tree is created from a bootstrap sample which is obtained by taking random samples with replacement cases from the original database. For the construction of each tree the technique takes a random sample of predictor variables at each split point (node) to select those that best split the data.
The performance of each tree in the Random Forest analysis can be evaluated by the way the model classifies the cases that were not considered in the bootstrap samples ("out-of-bag"). The error in classification is known as out-of-bag error (Nguyen et al., 2020). In this study, Random Forest models with 500 and 1000 trees were constructed for each case (hematocrit and hemoglobin).
Similarly, different numbers of variables were evaluated to define the best information division in each tree. Those models with the lowest out-of-bag error were selected. Confusion matrices were also estimated to evaluate the accuracy of the model and the Gini index or MDG -Mean Decrease Gini- (average impurity reduction) to identify the relative importance (influence) of each variable when estimating hematocrit and hemoglobin levels. The analyses were carried out in the Rstudio Team (2020) package version 4.0.2.
RESULTS AND DISCUSSION
Figure 1 shows the dendrogram resulting from cluster analysis where four well groups were defined: Group one: 30 days prepartum; Group two: 15 days pre and postpartum (close up dry cow); Group three: calving; Group four: 30 to 105 days postpartum.
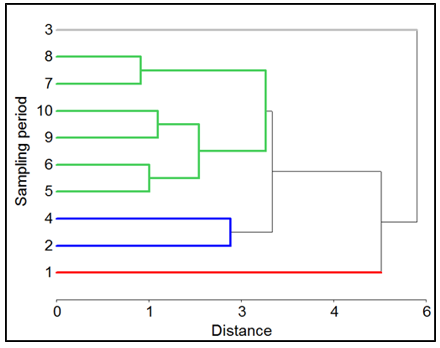
Figure 1 Dendrogram of cluster distribution according to hematic variables in ten lactation periods (1,2=prepartum; 3=partum; 6 to 10= postpartum) established during the transition period and first lactation phase in grazing dairy cows under low tropical conditions.
Descriptive statistics of hematological variables for cluster are shown in table 2. On day 30 prepartum, hematocrit and hemoglobin were high, while neutrophils were low. On the other hand, during 15 days pre- and post-partum, an increase in neutrophil count (24.4 106µL-1) and a reduction in eosinophils (5.3 106µL-1) were observed. At calving, an increase in NEFA concentration, neutrophils, monocytes and basophils counts and a reduction in eosinophils were observed. From 30 to 105 days, hematocrit and hemoglobin were low, in relation to the other clusters.
Table 2 Descriptive statistics within the clusters established by the behavior of hematic variables.
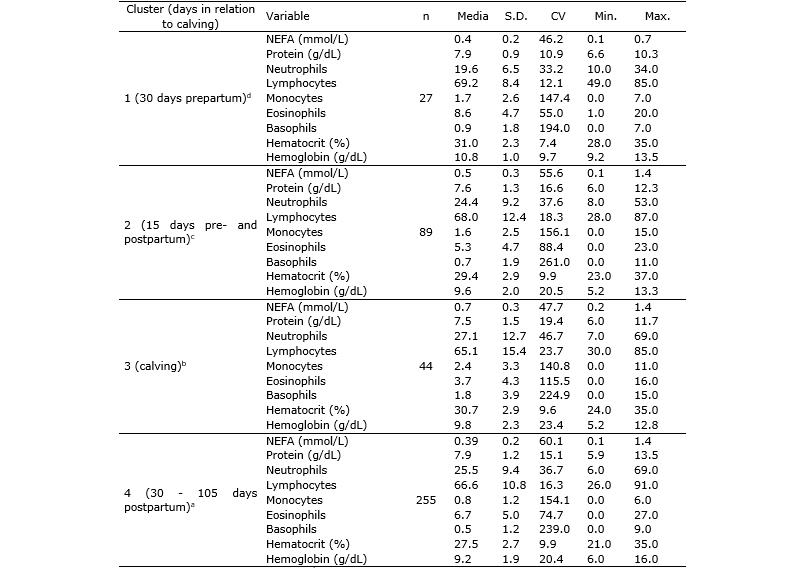
Neutrophils, lymphocytes, monocytes, eosinophils, basophils (106 μL-1). Significant differences (p< 0.05) are indicated by different letters in superscript in each cluster
Spearman correlations between hemoglobin, hematocrit, hematic cellular components and the two metabolic variables are shown in Table 3. In general, no significant relationships were observed between them.
Table 3 Spearman correlations between metabolic and hematological variables (estimated values and their significance are below and above the diagonal, respectively).
VA | NEFA | TP | NEUT | LYMP | MONO | EOSI | BASO | PCV | Hb |
NEFA | 1.00 | 0.01 | 0.03 | 0.00 | 0.00 | 0.00 | 0.89 | 0.00 | 0.00 |
TP | -0.13 | 1.00 | 0.01 | 0.00 | 0.10 | 0.06 | 0.00 | 0.82 | 0.00 |
NEUT | 0.10 | -0.14 | 1.00 | 0.00 | 0.37 | 0.13 | 0.69 | 0.51 | 0.00 |
LYMP | -0.16 | 0.21 | -0.85 | 1.00 | 0.00 | 0.00 | 0.00 | 0.50 | 0.00 |
MONO | -0.15 | -0.08 | 0.04 | -0.18 | 1.00 | 0.26 | 0.00 | 0.53 | 0.00 |
EOSI | 0.19 | -0.09 | 0.07 | -0.48 | -0.06 | 1.00 | 0.00 | 0.81 | 0.00 |
BASO | -0.01 | -0.18 | 0.02 | -0.23 | 0.20 | 0.15 | 1.00 | 0.77 | 0.36 |
PCV | 0.32 | 0.01 | 0.03 | -0.03 | -0.03 | 0.01 | 0.01 | 1.00 | 0.47 |
Hb | 0.40 | -0.26 | 0.19 | -0.27 | -0.15 | 0.26 | 0.04 | 0.47 | 1.00 |
Abbreviations: VA: Variable; NEFA: Non-Esterified Fatty Acids; TP: Total Protein; Neut: neutrophils; Lymp: lymphocytes; Mono: monocytes; Eosi: eosinophils; Baso: basophils; PCV: Packed Cell Value; Hb: Hemoglobin.
Ninety-three point seven percent (93.7%) of the animals showed hematocrit with a normal mean value of 29.64%, with values ranging between 24 - 37%. Seventy-four point four percent of the animals exhibited normal values for hemoglobin, with a mean value of 9.84g/dL. The behavior related with the “out-of-bag” samples is presented in Table 4.
Table 4 Confusion matrix for the prediction of the animals considered in the “out-of-bag” samples.
PREDICTION | HEMATOCRIT | HEMOGLOBIN | ||
Normal | Abnormal | Normal | Abnormal | |
Normal | 389 | 3 | 309 | 15 |
Abnormal | 12 | 11 | 26 | 65 |
Out- of- bag error (%) | 3.64 | 9.88 |
The use of the Random Forest technique allowed finding the variables that significantly affect the prediction of hemoglobin and hematocrit. Their importance (influence) is presented in Figure 2 and shows the participation of the two metabolites analyzed as well as the hematic cellular components through MDG.
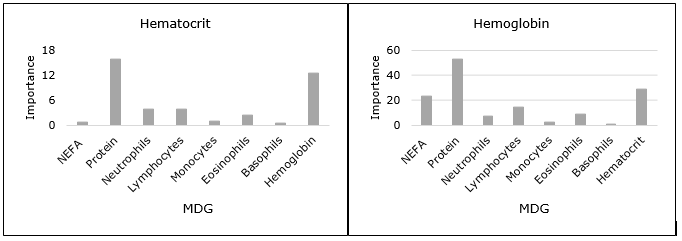
Figure 2 Importance of hematological variables to predict hematocrit and hemoglobin levels through the Random Forest models.
Variables described in the literature as blood cellular components (lymphocytes, monocytes, neutrophils, eosinophils, and basophils) and those related to blood dynamics such as blood cell volume (hematocrit) and gas transfer capacity (hemoglobin) were analyzed in the present study. Due to the close relationship between energy and protein metabolism, and their changing behavior during the transition period and the first phase of lactation, NEFAs, and TPs variables were also analyzed as an indicator of energy and protein metabolism, respectively concordant with Krupa et al. (2018), in monitoring during the transition period and the first phase of lactation.
Cluster analysis is a technique that allows analyzing a set of cases simultaneously and form groups according to their similarities (Di Rienzo et al., 2017). The estimation determined in the clusters for periods is logical (Figure 1) given that the situations associated with peripartum are clearly differentiated with three different clusters and a cluster where reunite five to ten lactation periods were grouped, which in terms of lactation corresponds to another productive phase.
As shown in Table 2, groups formed during the transition period included in the analysis, presented different hematic behavior at 30 days prepartum, calving and the days closest to calving (15 days pre- and postpartum). These results indicate that there are homeorhetic adjustments around calving as it is known for this period (Martens, 2020). Similar variations for these periods were reported by Campos et al (2008). The determination of specific physiological characteristics in peripartum allows a better intervention of animals in production to ensure a timely assessment of their health status (Aleri et al., 2016).
Periods five to ten, grouped in a cluster, correspond to the lactation phase between days 30 and 105 postpartum. No significant variations were found between them or between periods five and ten. This can be interpreted as a stability of blood dynamics at extreme moments of the first lactation phase once the transition period has ended. In this cluster, lower hematocrit and hemoglobin values were observed, which coincides with the results that have been presented by other authors (Cozzi et al., 2011; Campos et al., 2008) for the same lactation phase.
The multivariate analysis of variance for the simultaneous study of hematologic variables between the clusters formed, through the Wilks, Pillai, Lawley-Hotelling and Roy’s statistics, the presence of significant differences (P<0.05). Hotelling’s comparison test showed that the mean behavior of the linear combination of the hematological variables was different for each grouping (P<0.05). The hematological indicators analyzed show a behavior in which it is evident that the alterations in the peripartum period have a marked impact on them. These physiological variations can be caused by homeostatic changes and the presence of plasma cortisol associated with peripartum (Martens, 2020; Campos et al., 2008).
Regarding the breed composition within the clusters analyzed, it was observed that the multibreed component was the most numerous (70% of the individuals), while purebred groups comprised 30% of the animals in equal proportion (15%) for Holstein and Lucerne breeds. This shows that hybridization strategy to seek resistance and rusticity to the tropics has been consolidated in the dairy cattle population in Latin America. Within the clusters, proportionality was observed in the participation of racial groups. There were no differences in hematological behavior between breed groups or effect of grazing type, nor was there any effect of grazing type. In this sense, Radkowska & Herbut (2014) reported that the type of management applied to cattle does not generate an alteration in blood parameters.
Correlations presented between variables stands out that for both NEFA and TP, the relationships with hematological variables were inverse, which would indicate that metabolic processes do not develop in parallel with cellular processes in blood. Under the conditions of the study, it is accepted that nutritional factors may influence biochemical indicators throughout the different periods, as has been found in other works (McGuffey, 2017). It was observed that variables associated with cellular indicators tend to be more stable and showed slower response processes than metabolic adjustments. Therefore, based on these results, a useful tool would be available to predict possible health and production situations in dairy cows. Likewise, regarding the immune protection response, Zecconi et al. (2018) found a better behavior of cellular components when evaluating the immune status in dairy cows during dry period and transition period.
Models allow predicting a behavior or knowing what factors affect it. However, the use of this mathematical tool has not been widely studied on homeorhesis and blood dynamics. Moreover, the absence of reliable data, the uncertainty of biological aspects and the high number of variables affecting hematic dynamics, make the search for interpretation options become a need in order to obtain better field analysis results, as proposed (Tremblay et al., 2018). The analysis of main components and the identification of variation in physiological phases allow the improvement of productive efficiency with low-cost determinations, which benefits the producer by identifying physiological alterations in a timely manner and thus, achieving greater profitability (Grünwaldt et al., 2005).
Among the hematological variables (Table 2), hemoglobin and hematocrit levels are important because they are indicative of animal physiological function. The presence of hematozoa or hemoparasites can significantly affect the productive performance during lactation and the resumption of dairy cows reproductive activity under tropical conditions (Florio-Luis et al., 2012). The hematocrit and hemoglobin levels, show a typical behavior of hematic dynamics during the transition period and the first phase of lactation (Moretti et al., 2017) and similar to reports found by other authors in tropical environments (Krupa et al., 2018).
The accepted value for animal hematocrit levels in similar conditions to those of the study ranges between 27.2 and 53.4% (Florio-Luis et al., 2012). For hemoglobin, the range accepted as normal is between 8 and 15 g/dL. Both hematocrit and hemoglobin presented values at the lower limit of the accepted range. However, these are values without the presence of hemotropics in the systems and are therefore, considered normal within the reference framework proposed for bovines. The observed values coincide with Moretti et al. (2017), who conducted experiments in different periods during prepartum and postpartum stages.
The models established through the Random Forest technique to predict hematocrit and hemoglobin using other hematological variables as predictors, indicated that the models presented a low error rate during the classification of the animals present in the "out of bag" base, which allowed a correct classification of 96 and 90% of the hematocrit and hemoglobin levels, respectively (Table 4). This shows that the hematological variables evaluated in this study allow the identification of normal and abnormal hematocrit and hemoglobin levels. Figure 2 shows the average reduction in the Gini index, a useful measure to identify the importance of hematological variables in predicting hematocrit and hemoglobin levels. In the case of hematocrit, it can be seen that the total protein and hemoglobin variables were the most important, while for hemoglobin the most important variables were NEFA, TP, lymphocytes and hematocrit. Figure 2 shows the main components that explained in each case. TPs participate in the determination of the two components, while NEFA participates in the determination of hemoglobin, which shows the close relationship between blood components and the protein and energy metabolism (Grünwaldt et al., 2005).
CONCLUSIONS
Statistical methods like cluster grouping, multivariate analysis of variance, and random forest were useful to identify hematic dynamics during the transition period of tropical dairy cows. The results of this study evidenced differences in the behavior of hematic variables during the transition period and the first lactation phase in dairy cows managed under tropical conditions in the agrosystems analyzed in the work.
Well defined groups were established during the end of the close-up dry cow, the transition period and the first lactation phase. In the transition period, a different behavior was observed for all cellular components, metabolites and indicators of blood dynamics, compared to the lactation period.
Blood dynamics (hematocrit and hemoglobin) can be estimated from variables associated with metabolic indicators and blood components. In the case of hematocrit, TP and hemoglobin levels were shown to have a high influence on its prediction while for hemoglobin the influence was the NEFA, TP, lymphocytes and hematocrit variables.