Introduction
Concerns regarding the scientific literacy of students and the need to reduce the gender gap in science have been raised globally (Carrasquilla et al., 2022). This is a particularly pressing issue in the Brazilian context. According to the latest report of the Programme for International Student Assessment (OECD, 2019), only 1% of students achieved the highest level of proficiency in science, with overall scores far below the OECD average. Similarly, the science achievement of Brazilian students lags far behind that of other Latin American countries. Given that the affective variable, such as attitudes and motivation improves performance and has a positive impact on career decisions (Bidegain & Lukas Mujika, 2020; Lupión-Cobos et al., 2019; Wigfield & Eccles, 2020), its promotion is, therefore, an important goal for the science literacy agenda in Brazil. However, research in the Brazilian context has largely neglected such a construct, and the development of a valid and reliable motivation instrument has yet to be undertaken. Therefore, this study describes the cross-cultural adaptation of the Science Motivation Questionnaire II (SMQ-II) (Glynn et al., 2011) to Brazilian Portuguese and provides baseline data for Brazilian high school students, who are going through a moment where science career aspirations are established (Maltese & Tai, 2011). The rationale for choosing the SMQ-II and its theoretical underpinnings follows below.
Defining motivation
Motivation is an umbrella term used to refer to the internal state that arouses, directs, and sustains goal-oriented behaviour (Glynn et al., 2009; Schumm & Bogner, 2016). It is regarded as a multidimensional construct that interacts with cognition to influence learning (Potvin & Hasni, 2014; Taasoobshirazi et al., 2016). Important components of motivation include, but are not restricted to, intrinsic and extrinsic motivation, self-determination, self-efficacy, and task-values orientation (Anderman, 2020; Glynn et al., 2011; Ryan & Deci, 2000; Velayutham et al., 2011; Wigfield & Eccles, 2020). The study of students’ science motivation is a longstanding effort (Pintrich, 2003; Potvin & Hasni, 2014; Wigfield & Eccles, 2000, 2020). Motivation is regarded as an important trait influencing science academic success and career aspirations (Aeschlimann et al., 2016; Guo et al., 2015; Wang, 2013). Hence, the search for appropriate instruments to measure science motivation has received increased attention in recent years (Appianing & Van Eck, 2018; Fortus & Vedder-Weiss, 2014; Glynn et al., 2011; Kosovich et al., 2015; Tuan et al., 2005; Velayutham et al., 2011, Toma & Meneses-Villagrá, 2020; for reviews, see Potvin & Hasni, 2014; Toma & Lederman, 2022).
The Science Motivation Questionnaire
The Science Motivation Questionnaire (SMQ) is widely regarded as one of the highest-quality instruments of its kind, and it is arguably one of the most commonly used instrument in science education research to measure motivation (Ardura & Pérez-Bitrián, 2018; Salta & Koulougliotis, 2015, 2020; Schumm & Bogner, 2016). It was designed to examine the science motivation of undergraduates by elucidating why students strive to learn science, how intensively they strive, and the feelings and emotions aroused in this process (Glynn et al., 2007, 2009). The pursuit of a psychometrically valid and reliable instrument resulted in the development of a second version (SMQ-II, Glynn et al., 2011). According to Glynn et al. (2007, 2009, 2011) the SMQ-II was developed within the theoretical framework of social-cognitive theory and refined based on exploratory factor analysis results, yielding five central constructs: (i) intrinsic motivation, which refers to inherent satisfaction in learning science for the sake of learning science; (ii) career motivation; (iii) grade motivation, which involves learning science as means to an end, thus representing extrinsic motivation; (iv) self-determination, which represents students’ beliefs that they control their learning of science; and (v) self-efficacy, which denotes students’ belief that they can succeed in science.
The framework makes use of intrinsic and extrinsic motivation, self-determination theory, and self-efficacy, with reference to Bandura (2001) and Ryan and Deci (2000). As motivation is a latent construct that cannot be directly observed, such theories could be used as interpretive frameworks for the motivational measures in the SMQ-II.
Glynn et al. (2007, 2009, 2011) did not, however, explain the deeper theoretical rationale behind the items included. As a result, the wording of the self-determination construct is inconsistent with Ryan and Deci’s (2000) conceptualisation of intrinsic motivation, which is unrelated to the concept of effort (e.g., Item 22. I study hard to learn science) or spending time on a task (e.g., Item 11. I spend a lot of time learning science). It should be noted in this regard that self-determination items were originally designed to assess responsibility for learning science (Glynn et al., 2009). This may explain why some studies failed to reproduce the instrument’s original factor structure (Komperda et al., 2020; Yamamura & Takehira, 2017), which will be explored as follows below.
Cultural adaptations of the Science Motivation Questionnaire II
The SMQ-II has been extensively used and adapted to many countries and languages, including Turkey (Tosun, 2013), Greece (Salta & Koulougliotis, 2015), South Korea (Ha et al., 2016), Germany (Schumm & Bogner, 2016), Japan (Yamamura & Takehira, 2017), Spain (Ardura & Pérez-Bitrián, 2018), and Indonesia (Prasetya & Ridlo, 2018). Overall, the research gathered evidence that the SMQ-II can be used with secondary school and undergraduate students. Yet, the factor structure and length of the questionnaire varied across contexts. For example, the Japanese version only consists of four dimensions and 13 of the original 25 items. Among the adaptations that retained the original five-factor structure, several items were dropped from the Turkish, German, and Indonesian versions (Prasetya & Ridlo, 2018; Schumm & Bogner, 2016; Tosun, 2013). Similarly, when the SMQ-II was adapted to specific science-related disciplines, such as chemistry and biology, several items were problematic and thus removed (Komperda et al., 2020). Despite such differences in questionnaire length, the widespread use of the SMQ-II proved useful, revealing important trends in students’ science motivation. On the one hand, girls have higher self-determination than boys, but the results for self-efficacy and intrinsic motivation in science are the opposite (Ardura & Pérez-Bitrián, 2018; Glynn et al., 2011; Salta & Koulougliotis, 2015; Schumm & Bogner, 2016). On the other hand, as the school grade level increases, there is an overall decrease in motivation for science (Salta & Koulougliotis, 2015).
The present study
The Standards for educational and psychological testing conclude that adequate measurement properties of instruments are critical for achieving high-quality results and research trustworthiness (AERA et al., 2014). Reviews of the literature on measurement instruments for science education fell short of identifying motivational instruments for the Brazilian context (Blalock et al., 2008; Potvin & Hasni, 2014; Toma & Lederman, 2022). As a result, this study aims to cross-culturally adapt the SMQ-II to Brazilian Portuguese and assess the motivation of high school students. The SMQ-II was chosen for several reasons: (i) it measures core motivational constructs rooted in longstanding theories such as self-determination theory or social-cognitive theory; (ii) its short length and easy administration and scoring procedure reduces data-collection burden and respondent fatigue; and (iii) it is a widely used instrument and has been adapted to more than eight different languages, hence allowing for international comparisons. This endeavour is significant because it fills a gap in the Brazilian literature for high-quality measurement tools. It also contributes to a better understanding of Brazilian students’ motivation, allowing for the identification of aspects that require educational interventions if scientific literacy is to be pursued.
Method
This is an instrumental type of research, which refers to studies analysing the psychometric properties of measurement instruments (Ato et al., 2013). It adopts a cross-sectional design and uses convenience sampling techniques (Cohen et al., 2018).
Participants
Heuristics for determining the sample size for factor analysis, such as a 10-to-1 participant-to-variable ratio, are discouraged (Ferrando et al., 2022; Lloret-Segura et al., 2014; Mundform et al., 2005). Current recommendations consider the number of variables/items per factor and the magnitudes of item communalities. The SMQ-II is composed of five items per factor and has a five-factor solution, and previous research reported high communalities ranging between .60 and .80 (Schumm & Bogner, 2016). Therefore, a sample size of approximately 260 participants for each exploratory and confirmatory factor analysis would produce accurate estimates (Gaskin & Happell, 2014; Mundform et al., 2005).
The sample was drawn from the Federal Institute of Education, Science and Technology of Rio Grande do Norte (IFRN), located in the city of Pau dos Ferros, Brazil. IFRN’s are institutions promoted by the Federal Government of Brazil since 2008 to offer specialised vocational and technological education. It consisted of 646 students enrolled in the 1st (31%), 2nd (28.9%), 3rd (27.1%), and 4th (13%) grades of secondary school, with a mean age of 16.15 years (SD = 1.29). They were equally representative of the different study modalities: nutrition (34.5%), apiculture (31.6%), and computer science (33.9%). Half of the students declared being girls (50%), and several of them (9%) did not indicate their gender.
Translation of the questionnaire
The SMQ-II was translated to Brazilian Portuguese using cultural validation methods (Beaton et al., 2000). Two bilingual university professors with Brazilian Portuguese as their native language and a high level of English proficiency worked independently. The first one forward translated the items from English to Portuguese. The second professor, blinded to the original instrument, back-translated the questionnaire into English. Then, the four authors of thisstudy and the two professors jointly reviewed the equivalence between the two versions, ensuring that the Brazilian Portuguese version is an adequate reflection of the original.
Prior to large-scale administration, a group of eight Brazilian secondary school teachers reviewed the translated questionnaire and a pilot study was conducted with 30 students enrolled in 1st grade of secondary school. This process led to minor changes in vocabulary to accommodate items within the Brazilian context. The word “relevant” was replaced with “important” (item 1); “coloco bastante esforço” was adapted to “me esforço bastante” (item 5); “con hecer ciência” was changed to “ter conhecimento em ciência” (item 10); and “uma nota alta” was specified as “uma nota 10” (items 8 and 18).
During this process, the final instrument was obtained and administered in paper-and-pencil format to a large sample using Glynn et al.’s (2011) five-option Likert-type response (Never; Almost never; Sometimes; Almost always; Always). Informed consent was secured from the parents or legal custodians of all students. Participants were informed that participation was voluntary, anonymous, and would not affect school grades.
Data analysis plan
Psychometric properties
The Standards for educational and psychological testing define validity as the “degree to which evidence and theory support the interpretation of test scores for proposed uses of tests” and is regarded as the most fundamental consideration in developing tests (AERA et al., 2014, p. 11). It is considered a unitary concept with several types of evidence based on the test content, internal structure, or relations to other variables (convergent and concurrent evidence). This study focuses on the internal structure of the test scores, traditionally referred to as structural validity, which determines whether the various components of the questionnaire are homogeneous and distinct from each other (p. 16).
Since previous studies adapting the SMQ-II reported a different factorial structure than the original version, the internal structure of the Brazilian Portuguese version was assessed in two stages by randomly splitting the sample into two groups (Ferrando et al., 2022; Lloret-Segura et al., 2014). Responses from the first subgroup (n = 327) were subjected to robust exploratory factor analysis (EFA). Extant research suggests that factors are moderately correlated; hence, Maximum Likelihood with Direct Oblimin rotation was used (Ferrando et al., 2022; Gaskin & Happell, 2014; Lloret-Segura et al., 2014), which is also a procedure best suited for ordinal, Likert type of data. Factor retention was determined based on Cattell’s scree test, Horn’s parallel analysis, and Velicer’s MAP test, which are the most recommended criteria (Gaskin & Happell, 2014; O’Connor, 2000). Items with factor loadings below .32 or with cross-loadings between factors above .32 were removed (Tabachnick & Fidell, 2007). Informed by Ferrando and Lorenzo-Seva (2018), the quality and appropriateness of the factor solution were assessed based on the strength and replicability of the factorial solution (generalised H index > .80), the determinacy and accuracy of the individual score estimates (FDI > .90), and the closeness to unidimensionality index (UniCo < .95; ECV < .85). Inter-factor correlation matrices with correlations below .80 would suggest discriminant validity for each factor, while factor intercorrelations above .80 imply poor discriminant validity (Watkins, 2018). FACTOR (v.10.10.03) software was used (Lorenzo-Seva & Ferrando, 2006).
Successively, responses from the second subgroup (n = 319) were subjected to confirmatory factor analysis (CFA) to assess the model fit of the factorial structure indicated by EFA results. Mardia’s coefficient of 716.67, p < .01 indicated a violation of multivariate normality. Thus, the unweighted least squares estimation method was used and model fit was assessed against consensual goodness-of-fit indices for non-normality data (Harrington, 2009): normed fit index (NFI ≥ .95); adjusted goodness-of-fit index (AGFI > .90); and root mean square residual (RMR ≤ .08). Amos (v.23) software was used (Arbuckle, 2021). Configural, metric, and scalar invariance testing was conducted to examine whether the latent structure and factor loadings are equivalent and whether they measure the same aspect across gender (girls and boys), grade level (1st to 4th grades), and study modality (nutrition, apiculture, and computer science). Based on common recommendations (Byrne, 2016), invariance was assessed against the cutoffs described above, a nonsignificant change in Δ2, and a change in CFI ≤ .01, since the former is very sensitive to sample size.
Finally, responses from both subgroups (n = 646) were subjected to reliability analysis. Cronbach’s Alpha (a) and McDonald’s Omega (w) were calculated for each unidimensional factor as an index of internal consistency reliability, the latter being much more appropriate for ordinal items (Hayes & Coutts, 2020). Values above .70 were considered acceptable.
Brazilian students’ science motivation
Baseline scores for secondary school Brazilian students’ motivation in science were established using a 2 (gender) x 4 (grade levels) x 3 (study modality) multivariate analysis of variance (MANOVA) on sum scores (Widaman & Revelle, 2023) since the SMQ-II factors are conceptually related (Glynn et al., 2011; Salta & Koulougliotis, 2015; Schmid & Bogner, 2017).
Results
Validity based on internal structure evidence.
The mean values for each item ranged from 2.91 to 4.47, with eight items (32%) having a mean value greater than four. Given a Likert scale with five response options, there are no floor or ceiling effects.
The Kaiser-Meyer-Olkin (KMO) measure of sampling adequacy was marvelous (.91) and Bartlett’s test of sphericity was significant (x2 = 6479.02, p < .01), indicating an adequate correlation between the items for factor analysis. Cattell’s scree test revealed a significant drop at the fifth factor, suggesting that a four-factor structure accounts for most of the variance. Likewise, Horn’s parallel analysis and Velicer’s MAP test suggested that four factors were present in the data. Hence, four factors were extracted.
Direct oblimin rotation indicated that item 1 (The science I learn is relevant to my life) had a weak loading and low communality (< .32). Removal of item 1 yielded a parsimonious structure explaining 53.91% of the variance (Table 1). Inter-factor correlation matrix showed correlations below .80, indicating discriminant validity between factors (Table 2). Quality of factor solution indices met established criteria: (i) H index was .83, .79, .86, and .90 for each construct, respectively, suggesting well-defined latent variables which are more likely to be stable across studies; (ii) FDI was .91, .89, .94, and .95 for each construct, respectively, indicating that factor scores can be used for individual assessment; and (iii) UniCo was .94 and ECV was .82, indicating that the questionnaire should not be treated as unidimensional.
Table 1 Pattern matrix for exploratory factor analysis
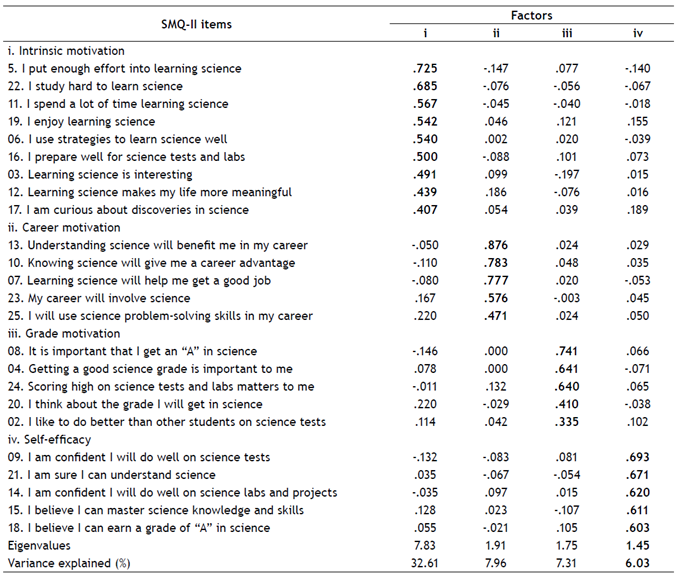
Notes: the wording of the original items is shown; loadings above 0.32 are shown in bold for readability reasons.
The four-factor model was further supported by the results of CFA, indicating good model fit (NFI = .97, AGFI = .98, RMR = .05) and strong standardised factor loadings (Figure 1). Since the self-determination construct was not salient, with such items loading into the intrinsic motivation factor, a three-factor model (Figure 2) was further submitted to CFA, also indicating a good model fit (NFI = .97, AGFI = .97, RMR = .6). These results suggest that a shorter, 3-factor model of the questionnaire without the self-determination and intrinsic motivation items could also be used to measure students’ science motivation. In the remaining analysis, we use the 4-factor model since it is more comprehensive and displayed slightly better model fit than the 3-factor one.
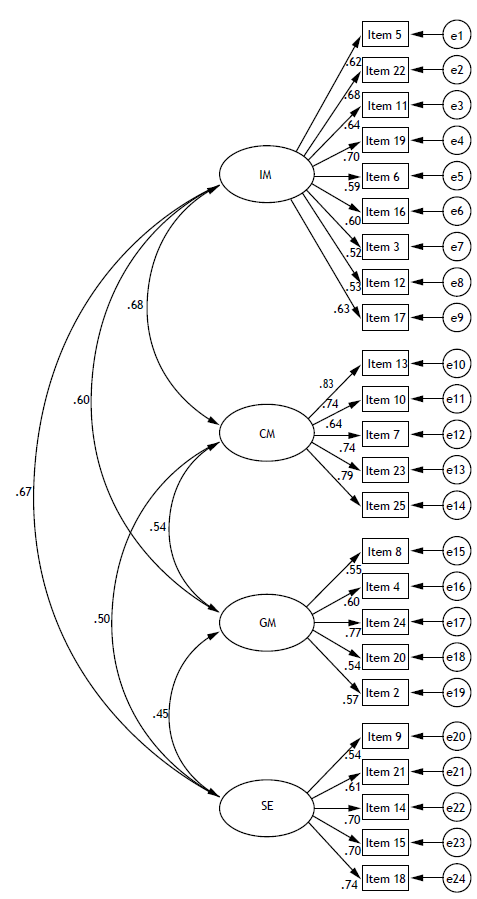
Note: Intrinsic motivation (IM); Career motivation (CM); Grade motivation (GM); Self-efficacy (SE).
Figure 1 Standardised estimates for the four-factor model
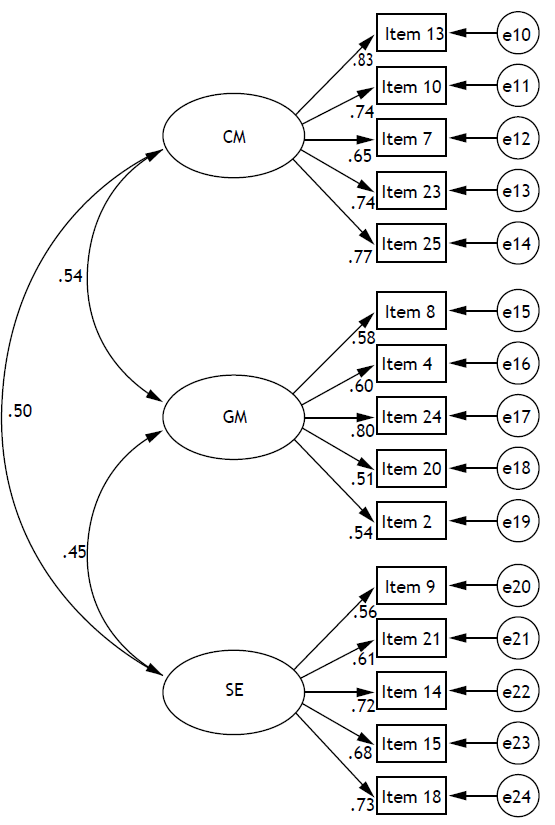
Note: Career motivation (CM); Grade motivation (GM); Self-efficacy (SE).
Figure 2 Standardised estimates for the three-factor model
Next, since sum scores will be used to analyse students’ motivations, justification for the unidimensional nature of each scale was provided (Widaman & Revelle, 2023). Hence, confirmatory factor analysis was conducted for each of the unidimensional models, with adequate model fit (Table 3). Likewise, the standardised factor loadings for the unidimensional models were strong (Figure 3), supporting that each scale is unidimensional and only measures one construct.
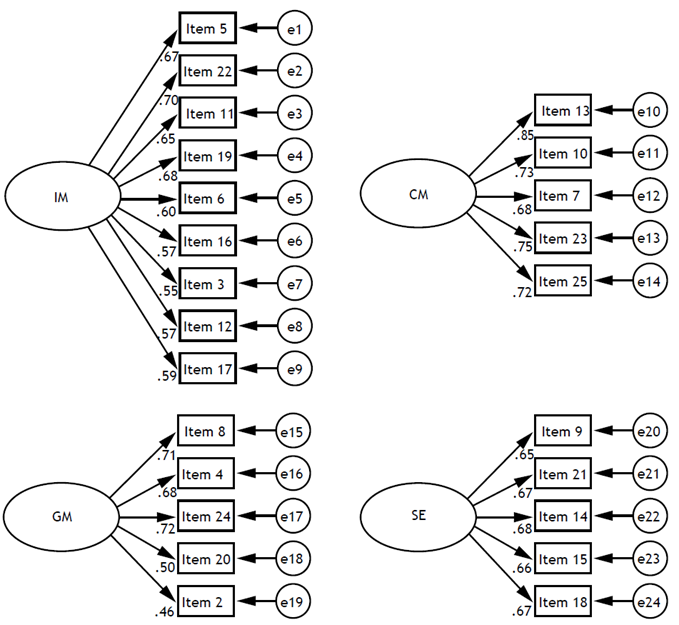
Note: Intrinsic motivation (IM); Career motivation (CM); Grade motivation (GM); Self-efficacy (SE).
Figure 3 Unidimensional-model for each construct
The results of configural invariance yielded a good model fi t a cross g ender ( NFI = .95, A GFI = .96, R MR = . 07), grade level (NFI = .94, AGFI = .95, RMR = .08), and study modality (NFI = .94, AGFI = .95, RMR = .08). These findings indicate equal factor structures across groups.
Findings of metric invariance yielded a non-significant change in ∆χ2 and a CFI ≤ .01 across gender (∆df = 20, ∆χ2 = 16.44, p = .69; ∆CFI = .002), grade level (∆df = 60, ∆χ2 = 79.97, p = .04; ∆CFI = .006), and study modality (∆df = 40, ∆χ2 = 38.86, p = .52; ∆CFI = .001), indicating that factor loadings are equal across groups.
Finally, scalar invariance was also established for gender (∆df = 20, ∆χ2 = 43.96, p = .002; ΔCFI = .009), and study modality (∆df = 44, Δχ2 = 5.74, p > .05; ΔCFI = .02), but not for grade level (∆df = 68, ∆χ2 = 122,992, p < .001; ∆CFI = .08); hence, findings of comparison in motivation between grade level should be interpreted with caution.
Reliability
Students’ responses to each factor were found to produce reliable results. Cronbach’s a were .85 (intrinsic motivation), .86 (career motivation), .75 (grade motivation), and .80 (self-efficacy). McDonalds’ ω were similar, with values of .86, .81, .72, and .82, respectively.
Students’ science motivation
Students in this sample reported moderate levels of motivation: intrinsic motivation (M = 3.61; SD = .65); career motivation (M = 3.89; SD = .89); grade motivation (M = 3.88; SD = .74), and self-efficacy (M = 3.54; SD = .71). MANOVA revealed no tertiary or secondary interaction effect between gender, grade level, and study modality (p > .05). Yet, there was a statistically significant main effect in the combined SMQ-II dependent variables for gender, F (4, 561) = 15.34, p < .01; Wilks’ Lambda = .90; ηp2 = .10, and grade level F (12, 1484.56) = 5.05, p < .01; Wilks’ Lambda = .90; ηp2 = .04.
Post-hoc univariate analysis revealed that girls reported significantly higher levels of intrinsic motivation (F (1, 564) = 5.65, p = .02, ηp2 = .01) and career motivation (F (1, 564) = 11.98, p < .01, ηp2 = .02) than boys. Opposite results for the self-efficacy variable were identified (F (1, 564) = 16.38, p < .01, ηp2 = .03). A small effect size was detected for all differences (Table 4).
Regarding the grade level variable, statistically significant differences were found for the intrinsic motivation (F (3, 564) = 3.35, p = .02, ηp2 = .02) and grade motivation (F (3, 564) = 14.69, p < .01, ηp2 = .07) variables. A post-hoc univariate analysis with Bonferroni correction revealed that first year students reported significantly higher intrinsic and grade motivation than third and fourth year students, with small and medium effect sizes, respectively (Figure 4).
Discussion
In recent years, research has paid increasing attention to the motivation construct. Yet, there is no valid and accurate instrument for assessing the science motivationthis study was to examine the psychometric properties of the SMQ-II (Glynn et al., 2011) regarding secondary-school students speaking Brazilian Portuguese, and to provide baseline data for their science motivation. Overall, students’ responses to the Brazilian Portuguese version of the instrument exhibited evidence of structural validity and internal consistency reliability and thus serves as a promising instrument that measures four constructs related to science motivation in this population. Examining students’ responses, on the other hand, revealed a moderate level of motivation that tends to decline in the upper grades, as well as gender disparities worth mentioning.
In terms of translation, the use of cross-cultural adaptation procedures and a pilot study with the target population indicated that the items of the SMQ-II in Brazilian Portuguese were accurately understood by secondary school students while sustaining an adequate representation of the original version. Next, exploratory and confirmatory factor analysis provided evidence of validity and supported the existence of a simple structure composed of four, well-defined and parsimonious underlying factors measuring science intrinsic motivation, career motivation, grade motivation, and self-efficacy. Likewise, reliability indices reached satisfactory results (Hayes & Coutts, 2020). The measurement invariance for gender and study modality was established. For the grade-level variable, only configural and metric invariance were supported, resulting in unsatisfactory results for scalar invariance. These findings imply that the factor structure is consistent across grade levels; however, students from different grade levels may respond differently to the Likert scale used for the SMQ-II, so comparisons across grade levels should be interpreted with caution.
In addition, some structural differences between the Brazilian Portuguese SMQ-II and the original version should be noted. Whereas Glynn et al.’s (2011) instrument contained 25 items measuring five motivational constructs, the Brazilian Portuguese version retained 24 items divided into four constructs. In the exploratory factor analysis, the self-determination construct was not salient, with such items loading into the intrinsic motivation factor. These findings were not unexpected. According to Komperda et al.’s (2020) theoretical analysis of the SMQ-II, such items do not align well with self-determination theory because they are unrelated to the psychological needs of autonomy, competence, and relatedness traits. Similarly, Ryan and Deci (2000) described self-determination theory within a continuum of actions ranging from extrinsic to intrinsic motivations; hence, considering self-determination as a different construct of intrinsic motivations is inconsistent with this theoretical framework.
Another explanation for these findings could be methodological variations among the research. Based on exploratory factor analysis, Glynn et al. (2011) initially retained the self-determination items as a separate construct. However, such a decision appears to be the outcome of erroneous EFA decisions. Indeed, neither the original study nor any translations of the instrument used valid construct extraction criteria, such as parallel analysis or the MAP test. Instead, the eigenvalue greater than one criterion was applied (Glynn et al., 2011; Schumm & Bogner, 2016; Tosun, 2013). However, such a procedure has been strongly criticised and is not advised because it results in the over-extraction of factors (Patil et al., 2008). Similarly, in several of the studies retaining five constructs, items were extracted using the Varimax-orthogonal rotation (Ardura & Pérez-Bitrián, 2018; Tosun, 2013). Varimax rotation is problematic since it rests on the assumption that factors are not correlated (Gaskin & Happell, 2014; O’Connor, 2000), which is not the case for the SMQ-II, nor the motivational traits investigated in social science research in general (Lloret-Segura et al., 2014).
In any case, the SMQ-II adaptation exposed the theoretical issues that the instrument encountered when attempting to adapt it to other languages (Komperda et al., 2020; Schumm & Bogner, 2016; Yamamura & Takehira, 2017). As previously stated, there is a conceptual problem with the constructs measuring intrinsic motivation and self-determination, as well as the items that represent the latter, in that they do not represent how these constructs have been defined in the motivational literature. As a result, while the SMQ-II is promising, especially given the scarcity of instruments for Portuguese-speaking students, and given that it is a widely used instrument in the international literature, it is certain that the SMQ-II would benefit from a theoretical revision, which has yet to be successfully conducted (Komperda et al., 2020).
Finally, the gender and school-level differences in students’ motivation are worth highlighting. Brazilian girls reported higher levels of intrinsic and career motivation, yet boys reported greater levels of self-efficacy. These results are consistent with extant literature regarding research conducted in Spain, the United States, Greece, and Germany (Ardura & Pérez-Bitrián, 2018; Glynn et al., 2011; Salta & Koulougliotis, 2015; Schumm & Bogner, 2016). On the other hand, science motivation decreases in the upper levels of secondary education, findings that are consistent with studies conducted in Greece (Salta & Koulougliotis, 2015).
The adaptation of the SMQ-II addresses the gap in sound measurement instruments for science education research (Potvin & Hasni, 2014; Toma, 2020). In their influential review study on the psychometric properties of existing measurement instruments, Blalock et al., (2008) advocated continuing to adapt and validate promising instruments, rather than designing new ones. Eventually, a cohesive set of instruments will emerge, allowing international comparisons to be made. As a result, the current study sought to accomplish this goal by focusing on the SMQ-II, which has been widely adapted to different countries, thus contributing to the literature with a promising instrument for the assessment of Brazilian students’ science motivation. Therefore, the proposed instrument can be considered a first step in the measurement of Brazilian secondary school students’ science motivation.
These findings, however, must be interpreted in light of the sample’s characteristics. As a result, the participants were drawn from a vocational and technological school. This sampling strategy may have resulted in a lack of participation from students interested in other fields, such as the humanities or social sciences. Following the Standards for educational and psychological testing (AERA et al., 2014), which state that validity is an ongoing process, future studies with a more diverse sample are required to determine whether the psychometric properties advanced here are generalisable. Evidence is specifically required for the instrument’s temporal validity (test-retest), which would support its use in studies with pretest-posttest designs or longitudinal studies with more than one data collection phase (Toma & Lederman, 2022). Similarly, if the instrument is to be used in predictive studies, evidence of the instrument’s predictive validity, i.e., the extent to which it predicts what it is theoretically supposed to predict, such as performance or achievement in science, is required first. This additional validity evidence would be extremely helpful in establishing the SMQ-II as a robust instrument for measuring science motivation in secondary education.
Despite these limitations, the Brazilian SMQ-II can be used to better understand how scientific motivation is shaped. Baseline data from this study suggests moderate levels of motivation in high school. Future studies should be undertaken to determine what variables may be causing or inhibiting science motivation, as well as what educational initiatives could be implemented to address the problem. Future research into the validity and reliability of the SMQ-II in late elementary/middle school students is also highly recommended. This would give a measurement instrument for longitudinal studies assessing the development of science motivation during compulsory schooling 1 2 3 4 5.