Introduction
The diversity of fatty acids (FAs) in ruminant meat and milk is mainly derived from ruminal dietary FA metabolism (Jenkins et al., 2008). Some FA classes, such as unbranched cis and trans isomers of 18:1, 18:2, and 18:3 FAs, are associated with biological activity in humans (Hur et al., 2017). Therefore, a better understanding of unbranched FA metabolism in the rumen may aid in designing nutritional strategies to enhance the quality of beef and milk.
A combination of gas chromatography and multivariate statistics may enable us to infer metabolic relations in the complex ruminal FA profile. Gas chromatography has greatly improved over the last 20 years, and it is now possible to get a detailed ruminal FA profile, including the detection and quantification of several positional and geometrical isomers of the 16:1, 18:1, 18:2, and 18:3 groups of unbranched FAs (Delmonte et al., 2012; Mele et al., 2016). Additionally, multivariate statistics offers different techniques that can capture the covariance structures of complex patterns of variables. One of these techniques is multivariate factor analysis (MFA), which allows to explain the maximum amount of (co) variance among the original variables (Snedecor and Cochran, 1989). This technique divides the total variance of a multivariate system into two components: the variance that all the variables share (i.e., communality) and the particular variance of each variable (i.e., uniqueness). The MFA has been used extensively to analyze the complex milk FA profile (Macciotta et al., 2004; Macciotta et al., 2015; Mele et al., 2016), to model milk composition (Todaro et al., 2005), and to study milk coagulation properties (Macciotta et al., 2012; Dadousis et al., 2018). However, to date, MFA has not been used to analyze the complex ruminal FA profile. Therefore, in this study we investigated the potential of using MFA to obtain information on unbranched FA metabolism in bovine rumen fluid.
Materials and methods
Ethical considerations
All procedures used across studies were approved by the Bioethics Committee of the Facultad de Medicina Veterinaria y de Zootecnia, Universidad Nacional de Colombia (Act 001 of 2010).
Data collection
A dataset containing 107 individual records from two ruminal in vitro incubation studies (Vargas et al., 2012; Vargas et al., 2018) was constructed (Table 1). The studies were conducted at the facilities of Universidad Nacional de Colombia (Bogotá Campus, Colombia). The dataset used was composed of 26 short, medium, and long unbranched saturated and unsaturated FAs (6:0; 8:0; 10:0; 12:0; 14:0; 14:1-t5; 14:1-c9; 15:1-t10; 16:0; 16:1-c9; 16:1-t9; 17:0; 18:0; 18:1-t6; 18:1-t9; 18:1-t11; 18:1-c6; 18:1-c9; 18:1-c11; 19:0; 18:2-c9,c12; 18:2-t11,c15; 18:2-c9,t11; 18:3-c9,c12,c15; 20:0; 23:0),expressed as grams per 100 grams of total FAs.
The concentrations of FAs in the incubation systems were determined using gas chromatography coupled to a flame ionization detector (GC-FID). Briefly, FAs in the incubation systems were extracted and methylated according to Garcés and Mancha (1993). Methylated FAs were quantified using a Shimadzu GC-2014 gas chromatograph (Shimadzu Manufacturing, Inc., Canby, OR, USA). The column was a fused silica capillary (Rt- 2560, 100 m x 0.25 mm i.d. x 0.2 μm film thickness; Restec®, Inc, Belefonte, PA, USA). Helium was used as the carrier gas. Detector and injector temperatures were 260 and 270 °C, respectively, and the split ratio was 30:1. Oven temperature was 140 °C for 5 min, increased by 4°C/min to 220 °C, held for 5 min, increased by 2.0 °C/ min to 240 °C, and held for 10 min. The FAs in samples were identified by comparison of their retention times with those observed in commercial standards as Nu- Chek® Prep (Elysian, MN, USA), and quantified by direct comparison of the peak areas.
Statistical analysis
An MFA was performed using the IBM SPSS (Statistical Package for the Social Sciences; IBM Corp, 2013) Statistics software, version 22 (IBM® Corporation, Armonk, NY, USA), to explore the metabolic relationships between unbranched FAs in bovine rumen fluid (Johnson and Wichern, 2014). The main aim of MFA is to explain the (co) variance of a system defined by n measured variables (Y 1, … ,Y n) by deriving a smaller number p (p <n) of latent unobservable variables (X 1, … ,X p), named common latent factors. The MFA accepts that the variance of each original variable can be decomposed into its common (i.e., communality) and unique (i.e., uniqueness) components. The factor model decomposes the covariance matrix of the measured variables (Q) as follows:
Q = AA’ + φ,
where AA’ and φ are the communality and the uniqueness (co) variance matrices, respectively (Snedecor and Cochran, 1989; Hair et al., 2014).
As stated in the (co) variance model, the measured variables can be represented as a combination of p unobservable common factors (X) plus a unique variable (e):
y1 = a11X1 + … + a1pXp + e1
yn = an1X1+ … + anp Xp + en,
Where a are the loadings that quantify the correlation between the ith latent factor and the measured variable. Loadings are the elements of the A matrix of the theoretical factor variance model.
The MFA was performed on the correlation matrix of 26 individual unbranched FAs. The number of factors to be extracted was based on their eigenvalue (> 1), their readability in terms of the relationship with the original variables, and the amount of variance explained. Factor readability was improved through a VARIMAX rotation. To extract the latent factors, a variable was considered to be associated with a specific latent factor if the absolute value of its correlation with the specific factor was greater than or equal to 0.5 (Mele et al., 2016). The suitability of the dataset used in MFA was further checked by calculating the Kaiser-Meyer-Olkin Measure of Sampling Adequacy (KMO), which determines the difference between Pearson and partial correlations (Hair et al., 2014).
Results
In this study, the KMO was 0.812 (p <0.01), close to the empirical threshold of 0.80 (Macciotta et al., 2012), which allowed to conclude that dataset used was suitable for MFA.
The overall patterns of the composition of ruminal FAs (Table 1) consisted of 11 saturated FAs (SFAs), 11 monounsaturated FAs (MUFAs), and 4 polyunsaturated FAs (PUFAs). The SFAs accounted for almost 42% of total FAs, MUFAs accounted for almost 42%, and PUFAs were a minor part, only accounting for almost 16%. Palmitic acid (16:0) and stearic acid (18:0) were the main SFAs, trans- vaccenic acid (18:1-t11) was the main MUFAs, and linoleic acid (18:2-c9,c12) and alpha-linolenic acid (18:3-c9,c12,c15) were the main PUFAs.
The MFA was able to extract four latent factors from the 26 FAs quantified in the ruminal fluid (Figure 1), accounting for about 86.7% of the total variance. The first latent factor accounted for the 46.3% of the total variance, and it was positively associated with short (6:0), medium (8:0, 10:0, 12:0, 14:0 and 16:0), and long (17:0, 20:0, and 23:0) SFAs, as well as with cis and trans MUFAs from 14 to 16 carbon atoms (14:1-t5, 14:1-c9, 15:1-t10, 16:1-c9, and 16:1-t9). Therefore, this factor was named “de novo FAs and Δ desaturase activity” (Table 2). The second latent factor explained 26.6% of the variance behind the first factor. It was named the “biohydrogenation (18:0 and MUFAs)”, and was positively correlated with 18:0 and the majority of cis and trans MUFAs of 18 carbon atoms (18:1-t9, 18:1-t11, 18:1-c6, 18:1-c9, and 18:1-c11) (Table 2).
The third latent factor was named as “biohydrogenation (PUFAs)”; it accounted for 8.6% of the total variance and was positively related to 18:3-c9,c12,c15 and 18:2-t11,c15, and negatively to 18:2-c9,t11 (Table 2). Finally, the fourth factor was named “novel pathway”; it explained 5.2% of the total variance and was positively correlated with 18:1-t6 and 19:0 (Table 2). However, 19:0 was also negatively associated with the second factor. The 18:2-c9,c12 was negatively correlated with the second and third factors.
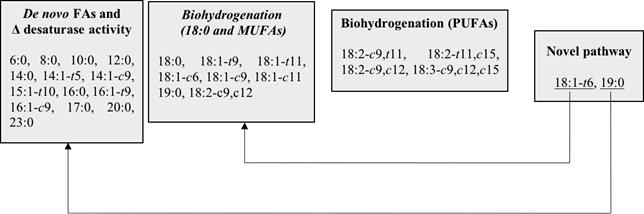
Figure 1 Metabolic groups of FAs in ruminal fluid and their interrelations derived from the multivariate factor analysis. FAs = fatty acids, c = cis, t = trans. Despite 19:0 and 18:1-t6 were mainly associated with the fourth factor, 19:0 and 18:1-t6 had a second significant interrelation with the first and second factors, respectively, which were represented using arrows.
Table 2 Rotated factor (F) pattern and proposed factor name, for F1 through F4.
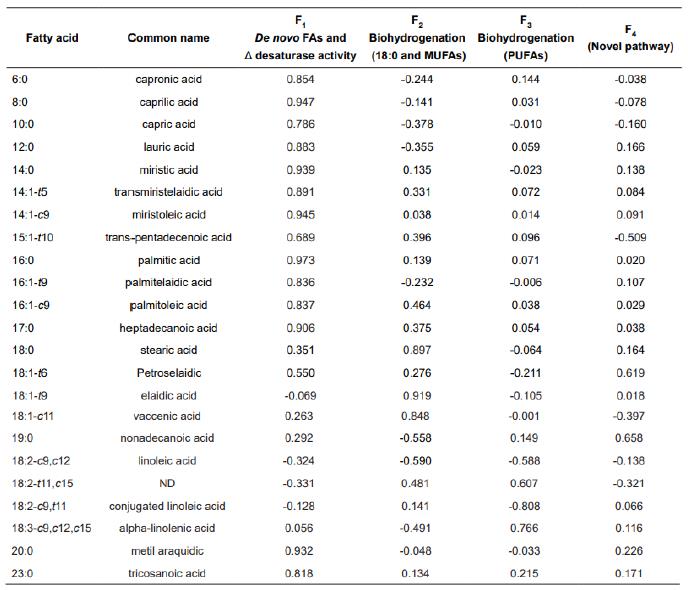
FAs = fatty acids; MUFAs = monounsaturated FAs; PUFAs = polyunsaturated FAs; ND = common name is not defined by the literature; c = cis; t = trans; numbers in bold correspond to main correlations between a variable and a specific latent factor.
Discussion
The aim of this study was to investigate the potential of using MFA to extract information on unbranched FA metabolism in bovine rumen fluid. The results suggested that, unbranched ruminal FAs could be categorized under four latent factors with metabolic significance. This implies that multivariate factor analysis could be applied to studying FA metabolism in the rumen.
The results revealed that 16:0 and 18:0 were the most abundant SFAs in ruminal fluid. These SFAs are the most predominant FAs in ruminal bacteria and protozoa (Jenkins et al., 2008). This is in accordance with Or-Rashid et al. (2007), who revealed that 16:0 content was 74% greater in the protozoal FAs than in the bacterial FAs, whereas bacteria had 2.25-times greater 18:0 content than protozoa. Among MUFAs, 18:1-t11 was the main FA. This was expected because 18:2-c9,c12 and 18:3-c9,c12,c15 tend to be mainly converted into 18:1-t11 during their ruminal biohydrogenation (Jenkins et al., 2008; Ferlay et al., 2017; Vargas et al., 2018). With respect to PUFAs, 18:2-c9,c12 and 18:3-c9,c12,c15 were the main FAs. High concentrations of 18:2-c9,c12 and 18:3-c9,c12,c15 were expected in ruminal fluid because the in vitro studies used to build dataset incubated pure 18:2-c9,c12 and 18:3-c9,c12,c15.
The MFA extracted four latent factors with biological meaning. The factor pattern (i.e., correlations between each factor and original variables) was easy to read. In particular, after examining the patterns across factors, we observed that many variables correlated highly with only one factor and poorly with others, showing correlation greater than or equal to 0.5 with one particular factor. This type of structure was an indicator of the suitability of multivariate factor analysis for examining the dataset used in this study (Snedecor and Cochran, 1989; Hair et al., 2014).
We observed that the first latent factor was positively associated with short, medium, and long- SFAs, as well as with cis and trans MUFAs from 14 to 16 carbon atoms, naming this latent factor as “de novo FAs and Δ desaturase activity”. Short, medium, and long-SFAs are endogenously synthesized by ruminal microorganisms from acetate and butyrate by the acetyl-CoA carboxylase and FA synthase enzyme (Emmanuel, 1974; Or-Rashid et al., 2007). Conversely, short and medium cis and trans MUFAs derive from Δ desaturase activities on the respective SFAs. Also, Ntambi et al. (1999) and Or-Rashid et al. (2007) demonstrated that bacteria and protozoa produce Δ9 and Δ11 desaturase enzymes, which produce MUFAs in the rumen. Therefore, MFA confirmed that these two groups of FAs (i.e., SFAs and short and medium-chain MUFAs) are metabolically linked under lipid ruminal metabolism, demonstrating that this statistical technique may be a valuable tool for analyzing the complex FA metabolism in the rumen.
A better understanding of the metabolic link between SFAs and MUFAs could help to enhance the physicochemical properties of milk. Considering that FA metabolism in the rumen significantly influences the milk FA profile (Jenkins et al., 2008, Hur et al., 2017), and milk fat fluidity is strongly affected by the relative abundance of SFAs (Mele et al., 2016), such understanding could be useful to design nutritional strategies for optimizing milk composition.
The 18:0 is considered as the main final product of 18:2-c9,c12 and 18:3-c9,c12,c15 ruminal biohydrogenation (Jenkins et al., 2008). However, Jouany et al. (2007), Shingfield et al. (2013), and Ferlay et al. (2017) demonstrated that these PUFAs are not completely biohydrogenated to 18:0, being interconverted in a great diversity of cis and trans MUFAs of 18 carbon atoms. Moreover, Or-Rashid et al. (2007) suggested that rumen protozoa have Δ11-desaturase activity, allowing conversion from 18:0 to 18:1-t11, and Kemp et al. (1984) and Ferlay et al. (2017) demonstrated that the ruminal fungus P. communis can dehydrogenate 18:0 to 18:1-c9. Therefore, a metabolic link between 18:0, 18:1 cis and trans FAs, and PUFAs may be expected, as demonstrated by the second latent factor named as “Biohydrogenation (18:0 and MUFAs)”, which was mainly composed by 18:0, a majority of cis and trans MUFAs of 18 carbon atoms, and of 18:2- c9,c12.
The third latent factor was mainly composed by 18:2-c9,c12 (which was also a representative FA of the second latent factor), 18:3-c9,c12,c15, 18:2-t11,c15, and 18:2-c9,t11. This suggests that the 18:2-c9,c12 could be a metabolic link between the second and third factors. This is as expected, considering that 18:2-c9,c12 and 18:3-c9,c12,c15 have common metabolic intermediates (i.e., 18:1-t11 and 18:0) (Jenkins et al., 2008).
The 18:2-t11,c15 and 18:3-c9,c12,c15 presented a positive correlation with the third latent factor. This is because 18:2-t11,c15 is an intermediary key of 18:3-c9,c12,c15 biohydrogenation (Hur et al., 2017). Similarly, 18:2-c9,t11 and 18:2-c9,c12 presented a negative correlation with the third latent factor, due to 18:2-c9,t11 is an intermediary key of 18:2-c9,c12 biohydrogenation (Ferlay et al., 2017).
Unlike 18:0, 18:1, 18:2, and 18:3 FAs are interrelated during lipid metabolism in the rumen (Jenkins et al., 2008), MFA revealed that 18:0 and 18:1 cis and trans FAs need to be described by a factor (i.e., second factor), and 18:2 and 18:3 FAs need to be described by another factor (i.e., third factor). This suggests that these two groups of FAs may also be metabolized by independent biochemical pathways. This is in accordance with Harfoot and Hazlewood (1997), who suggested that despite ruminal FA biohydrogenation occurs by interaction between several microorganism species, there are microorganisms (i.e., Fusocillus) capable to completely biohydrogenate 18:1-c9 and 18:2- c9,c12 to 18:0, as well as 18:3-c9,c12,c15 to 18:1-c15 without interaction with another microbial species. In consequence, with the use of MFA, we could highlight the metabolic differences between these groups of FAs (18:0, MUFAs, and PUFAs) by extracting two different latent factors.
Finally, the fourth latent factor was mainly composed by 18:1-t6 and 19:0. Also, 19:0 was also highly correlated with the second factor. This suggests that the 18:1-t6 may be metabolically linked to 18:1 cis and trans FAs (second factor) using 19:0 as a potential intermediate. A metabolic relation between 18:1-t6 and 19:0 in PUFAs biohydrogenation has not been reported in the literature. However, Kim et al. (2005) demonstrated that 18:1-t11 was strongly associated with odd and branched-chain FAs during lipid ruminal metabolism. Therefore, a potential metabolic relation between 18:1-t6 and 19:0 FAs could be possible as well. Thus, MFA could be used in future studies to find potential novel ruminal biohydrogenation pathways.
We would expect a high correlation of 19:0 and 18:1-t6 to the first and second factors, respectively, considering that the first factor was mainly described by SFAs and the second factor was mainly composed by 18:1 cis and trans FAs. However, they constituted an independent factor. This may suggest that 19:0 and 18:1-t6 have different metabolic origins of their chemical families. This is in accordance with Harfoot and Hazlewood et al. (1997), who suggested that the metabolic origins of FAs during ruminal biohydrogenation may be affected by microbial populations and ruminal conditions. Moreover, the findings of Kemp et al. (1975) and Shingfield et al. (2013) demonstrated that several bacterial species are capable of independently producing specific SFAs, and 18:1 cis and trans FAs, not necessarily involving the general biohydrogenation process.
The data demonstrated that multivariate factor analysis has many positive outcomes when applied to a dataset derived from the analysis of bovine rumen fluid samples. A first positive outcome concerned the reduction of a great number of variables to a few latent factors with biological meaning. Second, the statistical approach classified groups of fatty acids with common metabolic origins or linked to defined metabolic pathways. Considering the recent emerging analytical techniques for determining novel fatty acids involved in ruminal metabolism, we suggest that multivariate factor analysis could be useful to design studies aiming to determine novel fatty acid metabolic pathways. Moreover, this information may aid in designing better nutritional strategies to optimize the nutritional quality of ruminant milk and fat.