Introduction
According to the International Labor Organization, around 152 million children were victims of child labor in 2019 (ILO, 2019). Child labor affects different spheres of development and the capacity of societies to create sustainable economies. For instance, recent literature highlights the short and long-term adverse effects of child labor on children’s well-being, as it reduces school participation, and is detrimental to children’s learning, and health (Dinku, 2019; Edmonds & Pavcnik, 2005; Emerson, Ponczek & Souza, 2017).
Household budget constraints and living in poverty are some of the reasons that may explain child labor (Edmonds & Schady, 2012). However, the potential of international remittances to ease the budgetary restriction of migrant households in their country of origin has led to an increase in the analysis of the impact of remittances on child labor (Cuadros-Menaca & Gaduh, 2019) and the channeling of these resources on human capital investments (Ambler, Aycinena & Yang, 2015). In this article, we follow this stream of the literature and analyze whether international remittances may reduce child labor and enhance school attendance in Santiago de Cali.
Eliminating child labor is recognized as a priority in the sustainability and development agenda. SDG-8 includes the immediate banning of the worst forms of child labor, and by 2025, it expects the prohibition of child labor in all its forms. In this document, we define child labor as any market work activity and follow the idea that going to work represents an opportunity cost for children in terms of their learning process. On the other hand, the SDG-10 recognizes international remittances as an effective way to improve the living standards of migrants and their communities back home.
In recent literature, different studies also highlight the role played by remittances in schooling. Hanson & Woodruff (2003) relate the migratory behavior of Mexican households and the educational level of children; their results show that emigration helps to relax household credit restrictions to financing education. In the same vein, Edwards & Ureta (2003) examine the effect of international remittances on household schooling decisions in El Salvador and find that remittances have a significant impact on school retention. Recent evidence also highlights the role of remittances in deciding where to study and the quality of the educational institution, finding that families who receive remittances are more likely to send children to private schools (Salas, 2014).
To analyze the role of international remittances in child labor and schooling, we use data from the household survey Encuesta de Empleo y Calidad de Vida (EECV) conducted by the Colombian Ministry of Labor. The survey is representative of the 22 districts of Santiago de Cali and its rural area and serves to identify the population’s labor and quality of life conditions. It includes information on the characteristics of the households involved and their members, such as schooling, whether they work, and whether they live in a remittances-receiving household.
We use the Propensity Score Matching (PSM) technique introduced by Rosenbaum & Rubin (1983). This methodology uses the conditional probability of receiving treatment given the characteristics of pre-treatment; that is, the technique matches those cases that are subject to intervention (whether they are international remittance recipients) with one or more cases that do not receive the intervention. As long as selection comes from observable characteristics, this method consistently estimates treatment effects in a non-experimental setting.
We follow Acosta (2011) in using the Nearest Neighbor algorithm. We find that children living in remittance-receiving households are less likely to go to work, but we find no evidence that living in a recipient household affects attendance. The average treatment effect on the treated (ATT) indicates that living in a remittance-receiving household reduces the probability of working by 10.6 percentage points. The estimates are significant at the 1 percent level. Moreover, we show that our estimates are robust to different matching algorithms; Stratification Matching, Kernel Matching, and Radius Matching.
We also explore whether the effect of remittances varies with socioeconomic status. We exploit information in the survey that indicates the socioeconomic level of the households, finding that the share of working children is larger for those at the lower levels and decreases as we move to higher socioeconomic levels. Based on this, we create three groups: low, middle, and high. Although we do not find that remittances decrease child labor at the lower levels, the estimates indicate that those in the middle level benefit the most from remittance income.
The results in this document are consistent with recent findings in the literature. Acosta (2011) explores the way in which remittances influence education and work activities carried out by children in El Salvador. As in this paper, the author finds that living in a remittance-receiving household reduces the likelihood of child labor. For Colombia, Cuadros-Menaca & Gaduh (2019), using the instrumental variables methodology, find that an increase in the amount of remittances received reduces the probability of sending children to work. Our results coincide with these two studies, as they did not find a significant effect on the probability of children attending school. Moreover, the results in this paper add new evidence that child labor and schooling are far from being substitutes (Ravallion & Wodon, 2000), particularly for Colombia (Attanasio et al., 2010). Finally, this paper complements recent findings in the literature on remittances in Colombia (Arango, de la Mata & Obando 2015; Bonilla-Mejía, 2016; Cuadros-Menaca, 2020; Mora, 2013; Tovar & Vélez, 2007).
Overall, the results shown in this paper suggest that international remittances may complement government efforts to reduce exposure to labor for children in cities, such as Santiago de Cali, that have experienced episodes of migration in the past. It highlights the importance of creating policies aimed at making it easier for migrants to send money back. It also emphasizes the need for strategies that can help to channel these resources in a way that promotes economic development, such as investments in schooling.
The rest of the paper is organized as follows. Section 2 describes the background related to migration, child labor, and schooling for Colombia and Santiago de Cali. In Section 3, we describe the data obtained from the survey, the construction of the sample, and the variables. Section 4 describes the empirical strategy. In Section 5, we present our main results, and in Section 6, the heterogeneous effects by socioeconomic level. Finally, we present our conclusion in Section 7.
2. Background: International Migration, Child Labor and School Attendance
This section describes the historical and institutional context for the analysis of remittances, school attendance, and child labor. First, we present the background of international migration in Colombia and Santiago de Cali, and the implication on current remittance flows. Second, we describe the institutional and legal framework for child labor in Colombia and Santiago de Cali. Finally, this section presents the institutional context of the educational system.
2.1. International Migration and Remittances
Migration provides a safety net against adverse shocks or market failures in transnational households (de Haas, 2010). For example, The New Theory of Migration groups the causes of migration processes into three hypotheses. First, families seek to improve their socio-economic conditions. Second, migration may be the product of individuals’ desire to conduct income-generation activities (e.g., investments in education, agriculture, among others). Finally, migration can be caused by adverse shocks experienced by a household (e.g., social violence, natural disasters, among others).
The United Nations Department of Economic and Social Affairs (DAES) reports that by 2017, the number of migrants reached 258 million, compared to 173 million in 2000. One of the main economic results of these migratory flows has been the money transfers made by people to their families or friends in their places of origin. According to World Bank data, these flows reached a record high in 2018, with Latin America and the Caribbean, reaching a total of US$88 billion.
Colombia is no stranger to the impact of international migration and remittances. The Colombian Ministry of Foreign Affairs, estimates that by 2019 around 4.7 million Colombians were outside the country. The main destination countries include the United States, Spain, Chile, Ecuador, and Venezuela. This migratory flow has translated into a gradual increase in international remittances to Colombia (See Figure 1).
Nowadays, remittances represent one of the primary foreign exchange inflows for Colombia, even above some of the leading traditional export products, such as coffee, flowers, bananas, and textiles (Ramírez & Mendoza, 2013). By 2012, Colombia’s central bank, Banco de la República, the institution in charge of monitoring international remittances, reports the United States (35 percent), Spain (29 percent), and the Bolivarian Republic of Venezuela (10 percent) as the leading countries in which remittance income originates. Meanwhile, the main destinations for remittances in Colombia are the regions of Valle del Cauca (28 percent), Antioquia (15 percent), and Cundinamarca (13 percent) (Asobancaria, 2013).
According to the EECV for Santiago de Cali, the capital city of Valle del Cauca, remittances are mainly destined to recurring household expenses. Households report using this income primarily for household expenses (82 percent), which includes rent payments, utilities, food, health and clothing; home purchase (6 percent); education (5 percent); home improvement, vehicle purchase, or establishing a business (2.52 percent); other expenses (4.48 percent).
2.2. Child Labor
The Colombian Institute of Family Welfare, Instituto Colombiano de Bienestar Familiar (ICBF), is the state institution in charge of the protection of early childhood, adolescence, and the well-being of families in Colombia. This institution defines child labor as any activity, paid or unpaid, at the service of another person, by a child or adolescent under the age of 18, which, consequently, prevents their education and full development. According to data from the National Department of Statistics (DANE), by 2012, 10.2 percent of children/adolescents in Colombia under the age of 18 reported to be working.
It is essential to highlight that the Childhood and Adolescence Code states that children and adolescents must be protected against the worst forms of child labor and that the minimum age for admission to work is 15 years old. Children must be authorized by the Labor Inspection, or in its absence, the Local Territorial Entity (Law 1098 of 2006). However, boys and girls under the age of 15 may receive authorization to perform remunerated artistic, cultural, recreational, and sports activities. The permission will establish the maximum number of hours and prescribe the conditions in which this activity must be carried out. In no case will the permit exceed fourteen (14) hours per week. Nevertheless, (Cuadros-Menaca & Gaduh, 2019) report that these laws are not perfectly enforced, and a significant number of children start working in income-generating activities at the age of 12.
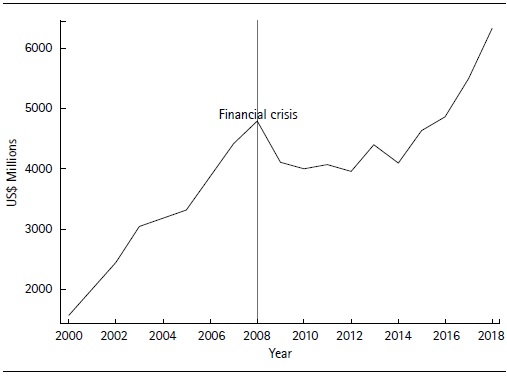
Figure 1. Remittance Flows to Colombia 2000-2018Notes: Remittance data obtained from Banco de la República de Colombia.
To prevent and eradicate child labor, the local authorities in Santiago de Cali have created a strategy called “Cali, a territory free of child labor.” This program implements actions that aim not only to prevent child labor but also to commit society and the participation of private and public institutions to fighting this problem. According to the EECV, around 6.13 percent of children aged 12 to 18 reported to be working.
2.3. Schooling in Colombia and Santiago de Cali
In Colombia, the General Education Law (Law 115 of 1994) organizes the educational system. In 2012, this law enforced compulsory schooling for every child aged between 5 and 15. Children must attend one year of preschool between the ages of 3 and 5, basic primary school between 6 and 10, and basic secondary school between 11 and 15. Finally, to access higher education, children must complete two years of upper secondary school, but these levels were non-compulsory (See Espitia, Rodriguez and Meñaca (2014) for details).
Figure 2 shows the evolution of school participation for those at basic secondary level in Santiago de Cali. The figure shows that for 2012 the schooling rate was 74.1 percent, the lowest since 2006. According to the EECV, the main reason behind children not studying has to do with high educational costs and lack of money (23 percent). Other options include that the child is not interested (17 percent) or considers that it is over (13 percent). To a lesser extent, children report needing to work or looking for work (11 percent), doing household chores or looking after the other children in the household (5 percent), lack of alternatives (3 percent), and the need for special education (2 percent). The remaining percentage reports other options. To tackle this problem, the government has carried out plans that promote school attendance, such as the Municipal Ten-Year Plan for Education, aimed at improving quality and guaranteeing access and permanence.
3. Data
In this section, we describe the data used to analyze whether remittances may reduce child labor and enhance school attendance. We also describe the sample construction process, the creation of the dependent and independent variables, and we present the descriptive statistics of the variables used by type of household.
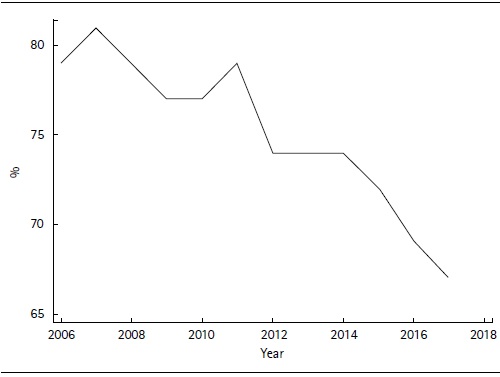
Figure 2. School Participation: Basic Secondary in Santiago de Cali 2006-2017Notes: Data on school attendance obtained from Cali en Cifras.
3.1. The Survey
We used the Encuesta de Empleo y Calidad de Vida (EECV-2012) applied in Santiago de Cali, and representative of the 22 districts of the city and its rural area. The purpose of the survey is to identify the labor situation of individuals in the city and design statistics regarding the population’s quality of life. The survey collects demographic characteristics for each household member and household characteristics, including housing assets and migration information.
3.2. The Sample
The total sample in the EECV consists of 30,458 individuals. Given that we are interested in child labor participation, we use the labor participation module that collects information for individuals over 12 years of age. We consider the definition of child labor and the General Law of Education described in the previous section. As such, we include children under 18 years of age, according to the ICBF definition, which, at the same time, captures grade repetition and late starters in the educational system. Our final sample includes 3,560 children/adolescents aged between 12 and 18. The total number of households is 2,752.
3.3. Child Labor, School Attendance, and Remittances
We create two dummy variables to measure child labor and school attendance. The latter takes the value of one if the child reports to be participating in some market activity, which involves paid and unpaid work, domestic service, and family work. We construct our second dependent variable, attendance, with a dummy that takes the value of one if the child reports to be attending school.
As we described in Section 2, international remittances are a valuable source of income for households in the Valle del Cauca region. The EECV collects information about whether each member of the household receives remittances. We follow the unitary household model to build the main independent variable as a binary variable that takes the value of one if the child lives in a household where one of the members received remittances during the last twelve months.
The statistics from the EECV report that in terms of child labor, around 2 percent of the children in recipient households report to be working, while this statistic in non-recipient households is 6 percent. On the other hand, 86 percent of children in recipient households report to be attending school compared to the 84 percent in non-recipient households. These descriptive statistics suggest that remittances may improve children’s welfare in the remittances-receiving household, decreasing their exposure to child labor and increasing schooling.
3.4. Other Independent Variables
Other explanatory variables correspond to characteristics of the child, the head of household, and the household. In the child characteristics group, we include the age and gender of the child. For the set of characteristics relating to the head of the household, we include the years of education and two dummy variables: gender and employment status. For the set of household characteristics, we include the number of adults (members over 18 years of age) and children (members under 12 years of age) in the household and an asset index. For the latter, we use assets and access to certain services to measure the household standard of living.
We use the following variable to construct the index: the availability of electricity, access to water services, sanitation installation, the type of material used for the floor, and the possession of certain items such as telephone and a television. Table 1 shows the list of variables used to construct the index. According to McKenzie (2005), the main advantage of constructing an asset index instead of using income level to measure the standard of living lies in the quality of the measurement. This variable is likely to help reduce bias in the respondent’s memory by asking questions related to the assets in the household rather than about how much income is spent and on what precisely.
Table 2 presents the descriptive statistics for our sample of children by type of household. Regarding individual characteristics, there are more girls in both recipient and non-recipient households. Besides, the average age is around 15. For the household-head characteristics, Table 2 indicates a higher proportion of women heads of household in recipient households. This may be related to the migration scenario of spouses. Moreover, the share of the employed household head is larger for non-recipient households. This may be explained by household members in the country of origin using remittances to buy time away from the labor market. Lastly, we also observe that the household heads’ level of education in both types of households is around eight years, and slightly higher in recipient households.
Concerning household characteristics, we find that the number of younger members and the number of adults is similar in both types of households. At the bottom of the table, we report the share of children by the asset index. The results suggest that most of the children are in the second quartile, with a larger share of children in the lowest quartile in non-recipient households. Finally, around 6 percent of the children live in recipient households.
4. Empirical Strategy
We use the Propensity Score Matching (PSM) methodology (Rosenbaum & Rubin, 1983) to analyze the influence of remittances on child labor and school attendance. To do this, we explore which individuals are more likely to live in a remittance-receiving household and calculate the average treatment effect for the treated (ATT). We are interested in comparing the incidence of child labor and school attendance for those children in households that receive remittances (T = 1) and those not exposed to treatment (T = 0). Thus, we calculate the difference in participation between those treated and those with the same probability of being treated.
The variables used for PSM include characteristics of the child, characteristics of the head of the household, and characteristics of the dwelling. These variables cover the most crucial migration determinants identified in the literature (Sasin & McKenzie, 2007). In particular, we include the variables mentioned in Section 3.4. Table 3 reports diagnostics of covariate balancing after matching for each variable and, at the bottom, reports the likelihood ratio test after matching. The statistics indicate that there were no systematic differences in the covariates between children in remittance-receiving and non-remittance receiving households, suggesting that those households which do not receive remittances can be considered a good control group.
PSM addresses the selection problem of living in a remittance-receiving household according to observable characteristics. We also perform sensitivity analyses after matching using the methodology in Rosenbaum (2002). Thus, we explore to what extent unobservable heterogeneity (hidden bias) between children in remittance-receiving and non-receiving households affects our estimates.
5. Results
5.1. Remittances, Child Labor and Schooling
This section presents the baseline results for this paper. Table 4 reports the ATT of living in a remittance-receiving household on the probability of working and attending school for children aged between 12 and 18. Panel A and B report the effect on child labor and school attendance, respectively. We follow Acosta (2011) in using the single nearest neighbor as our chosen algorithm. This matching method seems to perform better when the non-treated observations are more common than the treated.4 For the analysis, the standard errors are calculated following Abadie & Imbens (2011).
Before reporting the main results, we analyze whether the model is correctly balanced, given the independent variables used. In Figure 3, we present box plots of the propensity score over treatment levels for the raw data and the matched sample. The plot reveals that the matched sample box is the same over the treatment level, suggesting that the matched sample is balanced.
Table 4. ATT of Remittances on Child Labor and School Attendance (PSM)
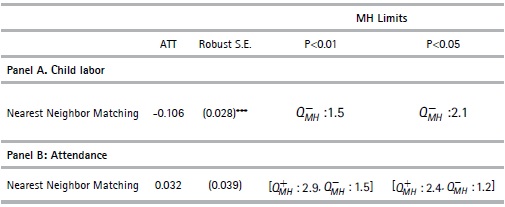
Notes:ATT are obtained using data from EECV-2012. The last two columns present the results of the statistical test (Mantel & Haenszel, 1959), suggested by Becker & Caliendo (2007). indicates the value of
under the assumption that we have overestimated the true effects.
indicates the value of
under the assumption that we have underestimated the true effects. We report robust standard errors in parenthesis following Abadie & Imbens (2011). */**/*** denotes significance at the 10/5/1 percent level.
We also check whether the overlap assumption is satisfied. Violating the overlap assumption implies that we cannot account for the unobserved outcomes for some individuals. We follow Busso, DiNardo & McCrary (2014) to check whether the estimated density has too much mass around 0 or 1. Figure 4 plots the density of the predicted probabilities that a child in a non-recipient household is, in fact, in a non-recipient household, and the estimated density that a child in a recipient household is in a non-recipient household. Neither graph indicates too much probability mass near zero and one suggesting that the overlap assumption is not violated.
Table 4 presents the baseline results of this paper. Under the PSM Nearest Neighbor Matching (NN) algorithm, each individual in the treatment group is paired with an individual in the closest control group, regardless of distance. ATT results indicate that living in a remittance-receiving household reduces the probability of working by 10.6 percentage points. This effect is significant at the 1 percent level. Unlike child labor, we find no evidence that living in a remittance-receiving household has any effect on the likelihood of attending school.
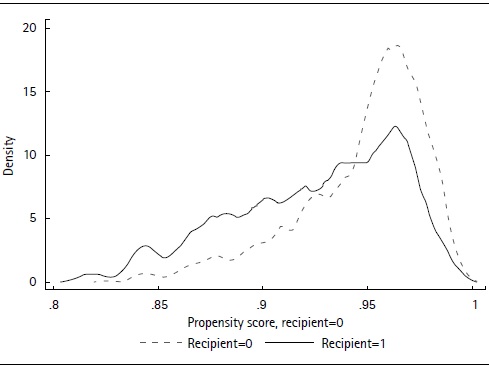
Figure 4. Estimated Densities and Overlap AssumptionNotes: The figure is obtained using data from EECV-2012.
We assess the magnitude of our estimates by comparing our results with two studies in Latin America, analyzing the recipient of remittances (at the extensive margin) and child labor. The study by Acosta (2011) uses propensity score matching to evaluate the effect of remittances on child labor in El Salvador. The author finds that with an incidence of child labor of around 10 percent in non-recipient households and 7 percent in recipient households, receiving remittances decreases the probability of working by 3.6 percentage points. In turn, Alcaraz, Chiquiar & Salcedo (2012), using a difference-in-difference strategy and data from Mexico, find that with a child labor incidence of 14.6 percent, increased child labor as a response to a decrease in remittances is of 11.4 percentage points. With an incidence of child labor of 6 percent in our sample, our results are large in magnitude.
The last two columns of Table 4 report the results of the statistic proposedby Mantel & Haenszel (1959) to determine the critical levels of sensitivity ofthe bias generated by possible differences in unobservable characteristics. Inthe case of child labor, the columns report the maximum value that rejectsthe null hypothesis that the treatment effect is zero at a significance level of1 and 5 percent under the assumption that we have underestimated the trueeffect. We do this because, under the assumption of no hidden bias, the QMH
statistic indicates a significant effect. Besides, the statistic becomes even moresignificant for increasing values of
under the assumption that we have overestimated(more positive) the true effect (See Table A1 in the Appendix). Underthe assumption that we have underestimated the treatment effect, which inour case is more relevant given the negative coefficient in our estimate, theresult becomes insignificant at the 1 percent level with a value of
= 1.6. Thisresult means that for an unobserved covariate, this variable should affect theodds of receiving remittances by a factor of 60 percent to alter the estimates.
In the case of school attendance, the p-values under the assumption that we have over(under)-estimated the true effect decrease as increases (See Table A1 in the Appendix). Moreover, even when the critical value of
does not mean that there is no effect of the treatment in the outcome variable, the lack of significance under the assumption of no bias
= 1 constitutes consistent evidence that receiving remittances does not affect school attendance. Based on these results, we report the first value of
, where the effect becomes significant at the 1 and 5 percent significance level under both assumptions of the statistic. Under the assumption that we have over(under)-estimated the treatment effect, the results state that the confidence interval would not include zero at the significance level of 5 and 1 percent if an unobserved variable caused the odds ratio of treatment assignment to differ between those at recipient households and their comparison group by 2.4(1.2) and 2.9(1.5) respectively. Overall the estimates for child labor and school attendance are unlikely to suffer from hidden biases.
One of the negative impacts that child labor may have in Colombia is the potential adverse effects on schooling (Ministerio de Educación Nacional, 2012). However, reducing child labor does not necessarily equal increased school attendance and vice versa. For instance, Ravallion & Wodon (2000) show that if schooling and leisure are perfect substitutes, increases in school attendance may come particularly by the reduction of leisure time and not a reduction in labor participation. Colombia is a good example of this. Attanasio et al. (2010) find that school and work time are not perfect substitutes, reporting that even when the government program Familias in Acción increased school participation by reducing the cost of schooling, there was no reduction in income-generating work activities, suggesting that some of the increased time in school comes from reducing children’s leisure time. With the absence of children’s time allocation data in our case, our results can only be taken as new evidence from Colombia that child labor and schooling are far from being substitutes. Nevertheless, the reduction in child labor underlines the importance of international remittances as a source of private transfers that may complement government programs such as Familias en Acción.
5.2. Robustness Checks
We now check the sensitivity of our results to different algorithmic methods. Estimates of the average treatment effect on the treated are reported in Table 5, with the baseline estimate in Column 1 for comparison. In Column 2, we report the results using the stratification method proposed by Dehejia & Wahba (2002). Column 3 contains the results matching the treated units with a weighted average of all controls (Kernel Matching). This methodology gives greater weight to controls with smaller distances. In Column 4, we use a distance-weighted radius matching methodology introduced by Lechner, Miquel & Wunsch (2011). One of the advantages of this methodology is that we do not need to decide the radius ad hoc, as it uses a partially data-driven choice of the radius size as a function of the distances in pair matching.
Panel A and B in Table 5 report the results on child labor and school attendance. Overall, we find that receiving remittances leads to a decrease in child labor. As in the case of nearest-neighbor matching, we find no evidence that remittances affect school attendance. In our baseline result, the coefficients are lower in magnitude but still within range of the estimates in the literature (see the previous section). In the stratification procedure, we find that with five blocks, the mean propensity score is no different from treated and control in each block. Moreover, we test the balancing property for each variable in each block and find that our covariates are balanced (See Table A2 in the Appendix). It is worth noting that the latter result is consistent with the covariates balancing test in our baseline specification (See Table 3). The results in the stratification procedure and the kernel matching indicate that living in a remittance-receiving household reduces the probability of working by 4.2 percentage points. Although there may be some concerns about the reliability of the standard errors under these two specifications (Abadie & Imbens, 2008), the results are still robust to our nearest neighbor matching. Finally, the results in Column 4 indicate that receiving remittances decreases child labor by 8.5 percentage points. Overall, the negative effect of remittances on child labor is robust to the different chosen algorithms.
Table 5. Robustness checks. The impact of remittances on Child Labor and School Attendance
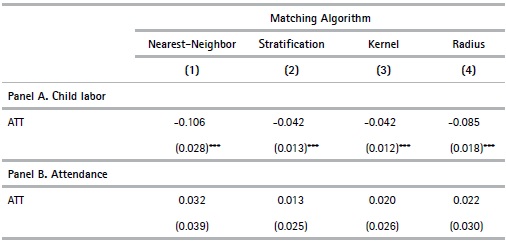
Notes:TT are obtained using data from EECV-2012. The Propensity Score in Columns 2-4 is computed using the variables in Table 3. The Kernel matching algorithm uses a bwidth (0.06). We report standard errors in parenthesis. Standard errors in Column 1 are computed using Abadie & Imbens (2011). Standard errors in Columns 2 and 3 are calculated using Bootstrapping. The radius matching estimator and standard error in Column 4 are computed based on Huber, Lechner & Steinmayr (2012). */**/*** denotes significance at the 10/5/1 percent level.
6. Heterogeneous Effects by Socioeconomic Level
In this section, we explore whether remittances may affect children differently according to their socioeconomic status. The literature on child labor considers poverty as one of the primary causes of using child labor to guarantee a household’s survival (Basu & Van, 1998). The literature also states that child labor may create poverty traps and lead future children to work at younger ages (Emerson & Souza, 2011). Accordingly, we are particularly interested in those with lower socioeconomic status.
To define the poverty condition of the household, we exploit information on the socioeconomic level of the household reported in the electricity bill. Households in Colombia are classified by socioeconomic levels on a scale of one to six. We build three socioeconomic levels following DANE classification. The lowest socioeconomic group is made up of households at levels one and two, which DANE considers low-low and low. The second group is formed of those children in households at levels three and four, which DANE classifies as middle-low and middle. The last group includes those households classified by DANE as middle-high and high. We exclude households classified as business properties or those that report not to be classified in any of the six levels.
Table 6 presents the ATT of living in a recipient household on child labor and school attendance. Panel A presents the results of child labor using the nearest neighbor algorithm as in the baseline results. The results indicate that living in a recipient household decreases the probability of working only for children in the middle class. Living in a remittance-receiving household reduces the probability of working by 8.2 percentage points. Regarding school attendance, the results in Panel B follow the baseline results. We find no evidence that living in a recipient household may promote school attendance.
In Figure 5, we present box plots of the propensity score over the treatment levels and the estimated density of the predicted probabilities by socioeconomic level. The plots suggest that the matched sample is balanced, and the overlap assumption is not violated. Moreover, diagnostics of covariate balancing after matching for each variable in each group in Table 7 show that the covariates are balanced.
Finally, Tables A3 and A4 in the Appendix report the results of the QMH
statistic to determine the critical levels of sensitivity of the bias. Regarding child labor, the statistic states that the confidence intervals would include the zero even under the assumption of no hidden bias for those children in the low and high socioeconomic groups. In the case of those in the middle socioeconomic level, since our estimate is negative and the p-values decrease as increases under the hypothesis that we have overestimated the effect, we focus on the case where we have underestimated the true effect. Only with a value of
= 1.4 and
= 2.0, does the statistic stop being significant at the 5 and 10 percent, respectively. Thus, the estimate is unlikely to suffer from hidden biases. On the other hand, in the case of school attendance, in the absence of hidden bias and under several values of
, the results reveal that the confidence interval would include zero which is consistent with our baseline results.
Table 6. ATT of Remittances by Socio-Economic Level (PSM)
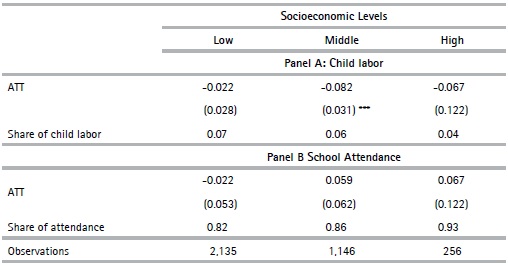
Notes:The sample includes children aged between 12-18 included in EECV 2012. The individual and household controls included are the same as in Table 3. Robust standard errors are computed following Abadie & Imbens (2011) and reported in parentheses. */**/*** denotes significance at the 10/5/1 percent level.
Table 7. Diagnostic of Covariate Balancing
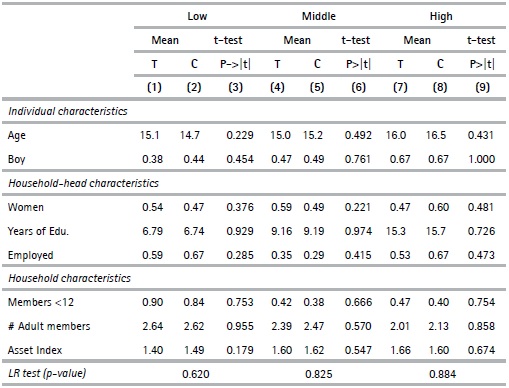
Note:Data is obtained from the EECV-2012 for children aged 12 to 18. LR denotes Likelihood Ratio Test, Tdenotes the treated sample and C the control sample. Columns 3, 6, and 9 report p-values form the t-teston the equality of means.
7. Conclusions
In this paper, we provide new evidence on the effect of international remittances on child labor and school attendance. Using a household survey representative for the city of Santiago de Cali, and a Propensity Score Matching strategy to correct the selection problem of living in a remittances-receiving household, we find that international remittances have a positive effect on the well-being of children/adolescents. In particular, we find that those children in recipient households are less likely to work.
The positive effect that remittances have on reducing child labor is relevant if we consider the negative effects of child labor on outcomes related to development such as poverty traps, as found in the literature. The sustainability and development agenda has ambitious goals of ending poverty (SDG-1) and eradicating any type of child labor (SDG-8), and the results in this document suggest that remittances may complement government efforts to achieve these goals.
Although we do not find that remittances have a positive impact on attendance, this result emphasizes the need for government programs that help channel remittances to human capital investments. It also highlights the need for other public programs that, again as found in the literature, promote human capital accumulation such as Familias in Acción (Attanasio et al., 2010) and Ser Pilo Paga (Londoño-Vélez, Rodríguez & Sánchez, 2020).
Finally, despite the fact that our results tackle only the problem of selection according to observable characteristics, the results in this paper complement and are consistent with other literature on remittances in Latin America and Colombia. These other studies use different strategies, such as instrumental variables, to solve the potential selection and the endogeneity problem of analyzing remittances. Further work in this direction may help to explore other heterogeneous effects of remittances.
Overall, the results in this paper are consistent with the New Migration Economics literature and they highlight the ability of international remittances to relax the budget constraints of households in the migrant’s country of origin and promote sustainable development (Taylor, 1999). With that, it also emphasizes the need for policies that make it easier for migrants to send money back home, and that reduce the cost of sending money (SDG-10).