1. Introduction
Customer Analytics Capabilities (CAC) have increasingly become a topic of interest among organizational management researchers; however, their conceptual and empirical understanding remains nascent. Evidence of this is seen in the foundational concept of customer analytics, which also lacks consensus regarding its definition and empirical manifestations, further hindering the conceptualization and operationalization of CAC. Various authors refer to customer analytics as “customer analytics” (or “consumer analytics,” “client analytics”, Ediger et al., 2014, Erevelles et al., 2016), while others use seemingly different terms like “customer intelligence” (Dam et al., 2021) or “customer big data analytics” (Hallikainen et al., 2020). Ultimately, these terms describe the use or value of analytics, with or without big data, in the customer’s specific domain. Additionally, some view customer analytics as an action or method (e.g., predicting customer purchase patterns to improve business; Nethravathi, 2020), as a process (e.g., acquiring, storing, processing, and analyzing a vast volume, variety, and velocity of customer-related data; Hallikainen et al., 2020), or as a strategic resource (e.g., integrating the concept of analytics into marketing and strategy disciplines; Louro et al., 2019).
In summary, in the quest for a better understanding of CAC, it is insufficient to rely solely on the concept of customer analytics to theorize from an organizational routines perspective, as its conceptualization and operationalization still lack widespread agreement. Consequently, it is necessary to gather, consolidate, standardize, systematize, and make accessible the common underlying knowledge from the various positions on analytics in customer contexts. This knowledge can then be interpreted from an organizational routines perspective to establish a position on the conceptualization and observable manifestations of CAC based on evidence.
With this in mind, the present study conducts a systematic literature review on customer analytics contexts, aiming to address four questions:
Q1: What bibliometric characterization does the scientific study of analytics present in organizational customer contexts?
Q2: What purposes, interpretations, and uses of analytics in organizational customer contexts prevail in scientific studies?
Q3: What conceptions and roles of CAC can be derived from the purposes, interpretations, and uses of analytics in customer contexts within organizations?
Q4: What taxonomic patterns classify the observable manifestations of CAC, considering the conceptions and roles of analytics in customer contexts within organizations?
By answering these questions, this study provides a consolidated, standardized, synthesized, and interpreted overview of the existing empirical evidence on analytics in customer contexts within organizations. This, in turn, offers elements for a better understanding of CAC regarding its conceptualization and observable manifestations. Such insights enable business leaders aiming to leverage CAC to create competitive advantages, invest their efforts correctly, and allow academics to continue studying CAC from a more precise and consensual conceptual foundation.
This manuscript is organized into five sections. The first section introduces the study. The second section outlines the methods, detailing the protocol for locating, refining, and analyzing the reference works. The third section presents and discusses the results related to the questions of interest. The fourth section focuses on the conclusions, and the fifth section addresses the study’s limitations.
2. Materials and methods
This study follows the macro-processes of the systematic literature review framework proposed by Pérez-Rave (2012, 2019), which includes the identification of the Relevant Literary Space (RLS), description (extraction and narrative synthesis of relevant aspects), deepening (characterization and grouping patterns), and dissemination (report preparation). This framework has been employed in various topics and contexts, including business excellence (La Rotta and Pérez Rave, 2017), project management (Velásquez et al., 2022), resistance to change (González et al., 2022), commitment to social responsibility (Arroyave et al., 2021), and sustainable supply chain (García-Cardona and León-Darder, 2023). The execution of the deployed macro-processes is described below:
2.1. Identification of the Relevant Literary Space
This macro-process considered five inclusion criteria for the studies to be reviewed, some of which have also been employed by González et al. (2022) and Wagman and Håkansson (2019):
Indexed Journals in Scopus: The studies must be sourced from journals indexed in Scopus, the database with the most extensive abstracts and citations from peer-reviewed literature (Vieira and Gomes, 2009; de Moya-Anegón et al., 2007; Cañedo Andalia et al., 2010; Schotten at al., 2017). This implies that these journals adhere to rigorous selection criteria, such as having a scientific committee, originality, and evaluation procedures (Baas et al., 2020). Other authors have also exclusively chosen the Scopus database for their systematic reviews, including Malek and Desai (2020), Mio et al. (2020), Macke and Genari (2019), Parmar and Desai (2019).
Empirical Articles: The studies must be articles that present the structure of the Introduction, Methods, Results, and Discussion (IMRaD) or similar. Therefore, review studies, editorial notes, short articles, books, and chapters are excluded.
Language: The studies must be written in English, the principal language of science.
Recent Publications: Studies published in the last two years (2019 and 2020) ensure the most up-to-date knowledge.
Business and Economics Journals: The studies must be from journals in business and economics, given the focus on organizational management from which the evidence is to be interpreted.
Based on these criteria, the search algorithm was planned and executed (date: 01/04/2021), and the document refinement procedure is summarized in Figure 1.
Figure 1 shows that the initial sample consisted of 74 potential documents. After three refinement stages to ensure compliance with all inclusion criteria, the sample was reduced to a final set of 42 papers, constituting the RLS to be addressed. The refinement occurred in two phases:
First Phase: The abstracts and keywords of the 74 potential documents were reviewed, excluding 16 papers that did not pertain to the topic of interest.
Second Phase: A deeper review of the contents covered the introduction, methods, and conclusions. This resulted in excluding 14 more documents because they were not empirical studies. Additionally, two documents were excluded because they were not available for consultation.
2.2. Description of the Relevant Literary Space
In this stage, the essential elements of each document included in the RLS were extracted after a detailed reading of their content. A synthesis of general fields (e.g., objective, typology, sources of information, and country where the study was conducted) and specific fields (e.g., definitions and uses of analytics in customer-related domains) was conducted. Typical errors made while synthesizing a document were checked and corrected, using the reviewer’s critical sense in writing and following the guidelines of Pérez-Rave (2012, 2019): imprecision, message manipulation, superfluous language, and lack of coherence, among others. This description of the RLS enabled a better understanding of each document and served as theoretical-methodological support for proceeding to the next stage, deepening.
2.3. Deepening in the Relevant Literary Space
In this stage, common study patterns were identified and interpreted using a deductive approach (theory-based) supported by the model proposed by Pérez-Rave et al. (2022). Detailed readings of definitions and empirical manifestations of analytics in customer-related domains, extracted in the description of the RLS, were conducted. In the present work, these manifestations are understood as specific descriptions of observable facts within the organization, confirming the application of analytical methods for any customer-related purpose. For each study reviewed, the meaning of analytics in customer contexts was inferred and classified as per Pérez-Rave et al. (2022): action/method (e.g., methodological purposes guided by methods or data), process (e.g., organizational routines of planning, cleaning, transforming, and modeling data to support decision-making), or strategic resource (e.g., strategic capability that creates competitive advantage).
Additionally, the role of analytics in gaining competitive advantages was inferred and classified according to three functionalities: development of operational capabilities (e.g., development of the organization’s conventional processes, products, and services), development of dynamic capabilities (e.g., renewal of operational capabilities), and adjustment of the firm’s strategy (e.g., global issues that have a significant impact on the organization’s sustainability/progress). The protocol for interpretation, inference, and classification of the evidence was deployed under the “agreement among judges” (consensus achieved among three professionals, one in business administration and two in industrial engineering), and the guidelines outlined in García-Cardona and León-Darder (2023) were considered.
3. Results
3.1. Bibliometric characterization of scientific studies on analytics in customer contexts within organizations
Table 1 presents a sample characterization of the RLS under study, considering four bibliometric variables: journal, classification in the Scimago Journal Rank (SJR), country of journal affiliation, and the areas in which the journal is categorized.
Table 1 Bibliometric Characteristics of the RLS by Publication Source Variables
Study | Journal | Quartile (SJR 2021) | Country of publication | Journal areas | |||||
---|---|---|---|---|---|---|---|---|---|
Marketing | Strategy and management | Technology, innovation or information systems | Decision sciences | Economics, econometrics, and finance | Business and international management | ||||
Mariani & Wamba (2020) | Journal of Business Research | Q1 | United Kingdom | X | |||||
Berggrun et al. (2020) | Finance Research Letters | Q1 | Colombia | X | |||||
Ketzenberg et al. (2020) | Journal of Operations Management | Q1 | United States | X | X | ||||
… | … | … | … | … | … | … | … | … | … |
Source: Authors’ own elaboration.
In Table 1, it can be seen that the 42 located studies are published in 28 different journals, which belong to the four established quartiles to measure their impact, as follows: Q1: 21, Q2: 12, Q3: 2, Q4: 7, with Q1 and Q2 having the highest participation (78.5%). The studies were conducted in the United States (12 studies), India (6), United Kingdom (5), China (2), Iran (2), and other countries (11 studies). Four studies do not record a country. Furthermore, 39 studies (92.8%) use a quantitative methodological design, while the remaining three use a qualitative design (case study: 2, ethnography: 1).
Table 1 also provides the areas of interest covered by the journals that published the studies addressed. In this regard, twelve journals include marketing within their areas of interest (43%), ten include strategy and management (36%), eight encompass technology management, innovation, or information systems (28%), eight include business and international management (28%), six address decision sciences or operations research (21%), and the remaining six cover economics, econometrics, or finance (21%).
Regarding the geographic regions of the publication sources, the United States, India, and the United Kingdom (55%) are the main hubs for knowledge transfer on analytics in customer contexts. Latin American countries’ participation is minimal, with only Colombia, Brazil, and Costa Rica each contributing 2.3% of the studies.
Overall, Table 1 shows that while marketing is the primary domain for analytics in customer contexts within organizations, it also permeates other essential areas of business management. These areas cover organizations’ complex realities (e.g., technology, innovation, or information systems; decision sciences or operations research; economics, econometrics, or finance) and soft realities (including strategy and management; business and international management). This indicates the broad scope of customer analytics and, consequently, the potential for developing organizational capabilities that can be recognized strategically, tactically, or operationally as CAC.
Furthermore, as innovation and technological development issues often occur, emerging countries reflect gaps in attention, generation, and/or pioneering adoption of such problems within their primary action mechanisms.
3.2. Purposes, interpretations, and uses of analytics in customer contexts
Below is a sample of Table 2, which summarizes each study’s purpose, interpretation, and use of analytics in the customer context. The complete table can be requested from the corresponding author.
Table 2 Description of the purposes, interpretations, and uses of analytics in the customer context
Study | Purpose | Interpretations of Analytics in Customer Contexts | Uses of Analytics in Customer Contexts |
---|---|---|---|
Mariani & Wamba (2020) | Analyzing How Consumer Goods Companies are Increasingly Adopting Big Data Analytics and Big Data Analytics Capabilities to Test New Products and Develop Innovation. | It’s a holistic process that involves collecting, analyzing, using, and interpreting data across various functional divisions. The goal is to achieve actionable insights, create business value, and establish a competitive advantage. This process gains even more value when it becomes an organizational capability. | Testing products before they are launched to the market through online customer feedback and machine learning algorithms. Listening to consumers in real-time and adapting offers and marketing communications to customer locations. Designing marketing communications based on location, micro-segmentation of customers, consumer attitudes, pricing, loyalty, optimal sales sequence, and distribution. |
Petrescu et al. (2020) | Evaluating the impact of the Internet of Everything (IoE) on marketing analytics, its benefits and challenges, and its implications for policy and legal frameworks. | It is the ability to transform a large volume of data collected by sensors or other means into valuable business insights, generating higher revenues, lowering costs, and increasing decision-making effectiveness. | Designing personalized offers and advertising based on consumer data collected from all machines and devices they interact with. |
Liu et al. (2021) | Using big data to investigate the effects of luxury brands’ social media marketing on customer commitment. | Leveraging data capture with greater volume, velocity, and variety to generate new capital for companies, as recognized by marketing specialists. | Understanding the influence of focusing on the dimensions of entertainment, interaction, trends, and personalization in a luxury brand’s social media activities on customer engagement with the brand’s social media content. |
… | … | … |
Source: Authors’ own elaboration.
In Table 2, a broad spectrum of purposes can be observed, ranging from limited objectives, such as improving customer routing in a call center (Ilk et al., 2020) or evaluating promotions (Khouja et al., 2020), to more ambitious scopes, such as measuring the impact of marketing strategies on sales (Sohrabi et al., 2019), assessing brand health (Rajan, 2019), or determining the factors that affect customer satisfaction (Lee et al., 2020; Rajendran, 2021). The spectrum is equally broad for the reported uses of analytics in customer contexts.
Table 2 also shows a variety of interpretations that prevail in the studies. For example, analytics in customer contexts can be represented as a holistic process (Mariani and Wamba, 2020), a technology (Boldosova, 2020), an organizational capability (Louro et al., 2019), a practice/art (Lee et al., 2020), or a set of tools (Raeesi-Vanani, 2019), to name a few. The richness represented by this diversity of purposes, uses, and interpretations of analytics in customer contexts is leveraged in the following subsection to discover patterns regarding the conceptions and roles in the organizational routines of CAC.
3.3. Conceptions and roles of CAC
Based on the taxonomy proposed by Pérez-Rave et al. (2022) for analytics in general, Table 3 classifies the reference studies according to three levels of roles and conceptions of CAC. This classification follows the interpretation of analytics’ purposes, interpretations, and uses in customer contexts, as presented in Table 2.
Table 3 Conceptions and roles of analytics in customer contexts
Source: *Taxonomy based on Pérez-Rave et al. (2022)
Concerning the conceptions of CAC exposed in Table 3, the reference works whose contributions were classified in the “action/method” category focus either on the collection, processing, and analysis of data to extract useful information for marketing decision-making (Cao and Tian, 2020) or on observing customer behavior and predicting their purchase patterns to improve business operations (Nethravathi et al., 2020). Manifestations of this conception include, for example, predicting abusive behavior of customers who return products after they have been used (Ketzenberg et al., 2020), generating a model to allocate the marketing budget within the organization (Kolsarici et al., 2020), determining the effectiveness of different types of promotions (Khouja et al., 2020), or properly selecting leads to implement cross-selling strategies (Rakhman et al., 2019).
The “complex process” conception included studies oriented towards the acquisition, storage, processing, and analysis of big data (e.g., routines and technologies in contexts of high volume, variety, and velocity) related to the customer to create meaningful information for decision-making in the company and timely discovery of insights (Lu et al., 2021). Manifestations of this conception include CRM processes based on data collected from user interactions on the internet (Del Vecchio et al., 2020), management routines of customer lifecycle value (Hallikainen et al., 2020), and systematic monitoring of customer perceptions of the company’s brands (Nethravathi et al., 2020).
From the “strategic resource” conception, works focus on the final analytics integration within the marketing and strategy binomial (Louro et al., 2019). Manifestations of this conception include understanding the variables that most affect business sales through classification methods that select the variables that best discriminate sales outcomes (Sohrabi et al., 2019) and measuring brand health through the sentiments of the digital audience (Rajan, 2019).
Concerning the inferred roles for CAC from the analysis of the purposes, interpretations, and uses of analytics in customer contexts within organizations, three categories were used (Pérez-Rave et al., 2022): “develop operational capabilities,” “develop dynamic capabilities,” and “adjust the firm’s strategy.”
Regarding the role of CAC in developing the organization’s dynamic capabilities, reference is made to studies in which analytics represents the firm’s ability to engage in continuous learning from customer and market analysis. This includes using the resulting findings to detect opportunities and absorb information/knowledge to reconfigure organizational resources, favoring sustained competitive advantage (Barrales-Molina et al., 2014). This view is consistent with those of Teece et al. (1997) and Bruni and Verona (2009), which propose that using analytics in customer contexts creates knowledge assets that are difficult to trade, primarily related to the domain of customers and competitors. These assets are part of establishing sensing capability, allowing the firm to obtain valuable information to detect threats and create opportunities.
Studies on the role of CAC in developing operational capabilities focus on how analytics leverage the organization’s collective knowledge and other resources to enhance current business activities related to marketing (Vorhies, 1998). This enables the organization to create customer solutions (Day, 1994), such as pricing and channel management (Prašnikar et al., 2008). This view assumes a role for CAC that does not focus on improving marketing capabilities by consuming new market knowledge (Vorhies et al., 2011).
The role of CAC in adjusting the company’s strategy refers to analytics as a fundamental requirement for generating sustained competitive advantages. This must address strategic issues such as creating an outstanding customer experience and accelerating customer commitment Hossain et al., 2022; Rosado-Pinto and Loureiro, 2020).
As a complement to the previous table, Table 4 provides a two-way frequency analysis, indicating the number of studies in each intersection between conceptions and roles of analytics in customer contexts within organizations.
Table 4 Crosses between conceptions and roles of analytics in the customer context, according to the evidence
Roles in the generation of competitive advantages | ||||
---|---|---|---|---|
Adjusting the firm’s strategy (consequence and not means) | Developing dynamic capabilities | Developing operational capabilities | ||
Conceptions of Analytics in Customer Contexts within Organizations | Action/method (technological) | 5 | 4 | 12 |
Complex process | 3 | 3 | 2 | |
Strategic resource | 4 | 7 | 2 |
Source: Authors’ own elaboration.
According to Table 4, among the studies that conceive analytics in customer contexts as a strategic resource, most reflect an intention to develop dynamic organizational capabilities through their use. Secondly, they reflect the intention to adjust the organization’s strategy, while a minority tends to understand its role in developing operational capabilities. These findings reflect consistency between understanding analytics in customer contexts as a strategic resource and what is expected from the organization. Thus, understanding it as a strategic resource and expecting it only to develop operational capabilities represents a waste and a lack of understanding of the organization’s long-term survival. Similarly, under the same conception, expecting it to contribute to reconfiguring the organization’s strategy results in the best utilization of its potential.
From the studies that conceive it as a complex process, a relative balance is observed among the roles attributed to analytics in customer contexts. This finding is logical, considering that a process-oriented vision is necessary for achieving all three objectives. Among the studies that conceive it as an action or method, most justify the existence of analytics in customer contexts by its role in developing operational capabilities. This fact is consistent with what is expected, as this conception is insufficient to ensure the generation of higher-order capabilities or the reconfiguration of the company’s strategy.
A conceptual representation that describes the underlying associations in the evidence presented in Table 4 is shown in Figure 2.
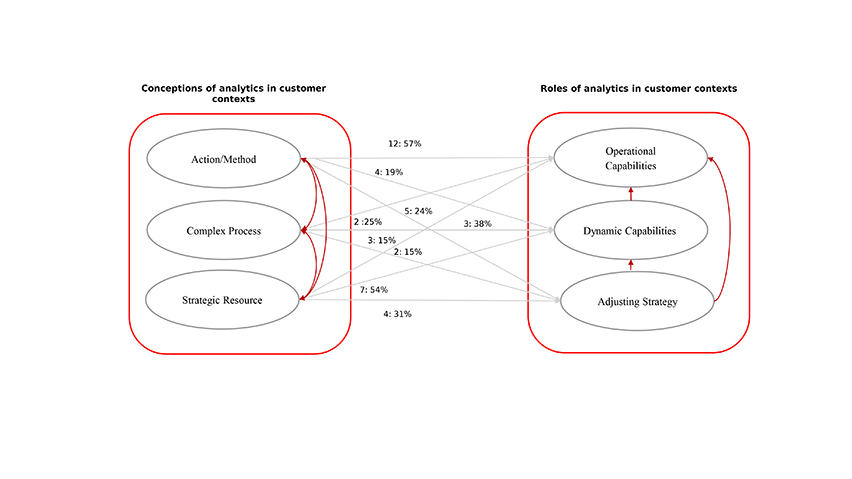
Source: Authors’ own elaboration adapted from Pérez-Rave et al. (2022).
Figure 2 Underlying associations between conceptions and roles of analytics in customer contexts
The conceptual representation in Figure 2 consists of three fundamental elements:
The dimensions of the conceptions of analytics in customer contexts
The dimensions of the roles of analytics in customer contexts for obtaining a competitive advantage for the organization
The associations between conceptions and roles that underlie the evidence presented.
The interpretation of the dimensions of conceptions and roles (elements 1 and 2) is presented at the beginning of this section. The percentages in each oval represent the participation of each dimension within the conceptions and roles, respectively. It is observed that, in almost half of the cases, analytics in customer contexts is assumed as an action or method and that the most sought-after objective is the development of operational capabilities. This reflects that it needs to be sufficiently exploited and has the opportunity to continue gaining maturity in terms of its contribution to the survival of organizations.
Regarding the relationships between conceptions and roles (element 3), in addition to the observations in Table 4, it is worth mentioning that the evidence highlights two significant contradictions: some organizations conceive analytics in customer contexts as a strategic resource but primarily expect it to develop operational capabilities (15% according to the evidence presented in Figure 2), and others conceive it as an action or method and expect it to achieve reconfigurations in their strategy (24% according to the evidence presented in Figure 2). Both contradictions result in unintended consequences: waste and short-sightedness, respectively. Although this study does not aim to generate propositions about these relationships, it does aim to lay the groundwork for future work to explore and confirm them. The relationships found generally show consistency between the conception and the role.
In summary, the conceptions and roles of analytics in customer contexts can be reasonably represented by the reference model’s taxonomy, which generally represents analytics. Additionally, the links between the conceptions and roles of analytics in customer contexts reveal patterns of organizational behavior that show relative consistency with intuition and reports of disconnects between analytics and organizational strategy.
Table 5 describes different maturity levels at which CAC can exist in business organizations. The higher maturity levels (3, 2, and 6) represent organizations with high expectations for using analytics in customer contexts and accordingly understand it as an element of high strategic value. The lower maturity levels (4, 7, and 8) represent organizations with more conservative aspirations regarding the contribution of analytics in customer contexts, viewing it primarily as the operational execution of various analysis methods.
Table 5 Taxonomic analysis of organizational behavior concerning conceptions and roles of analytics in customer contexts
Conception of analytics in customer contexts | ||||
---|---|---|---|---|
Action/Method | Complex Process | Strategic Resource | ||
Role of analytics in customer contexts | Adjustment of organizational strategy | 1. Companies that use CAC to reconfigure organizational strategy (by adopting practices such as understanding mechanisms of performance impact, predicting business behavior, and identifying satisfaction and dissatisfaction factors) still face a disconnect between CAC and strategic issues, limiting its scope to the development of individual skills for applying machine learning algorithms. | 2. Companies that use CAC to reconfigure organizational strategy in all its dimensions (e.g., designing customer relationship strategies, evaluating customer value, determining the best growth and sustainability strategies for the coming years) view CAC as a systematic process for generating actionable insights to support efficient decision-making. However, they have not yet made CAC a strategic issue. | 3. Companies that use CAC to reconfigure organizational strategy and conceive CAC as a creator of sustained competitive advantage undertake initiatives aligned with this aspiration, such as understanding the effect of marketing on the organization’s economic performance and identifying demand determinants. |
Development of dynamic capabilities | 4. Companies that use CAC to continually learn from the market and detect and absorb opportunities from the environment (e.g., adopting practices aimed at understanding the determinants of customer satisfaction, designing business models, understanding the effect of loyalty programs, and understanding brand perceptions) often limit themselves to developing individual skills for applying machine learning algorithms. | 5. Companies that use CAC to continually learn from the market and detect and absorb opportunities from the environment (e.g., seeking to understand customer perceptions of products, services, and the brand) view CAC as a systematic process for generating actionable insights to support efficient decision-making. | 6. Companies that use CAC to learn from the market and detect and absorb opportunities from the environment (e.g., capturing trends and developing new products and services supported by CAC) adopt an abstract view of CAC as a creator of sustained competitive advantage. This approach should contribute to strategic issues like innovation and the company’s competitive position in the market. | |
Development of operational capabilities | 7. Companies that use CAC to develop individual skills necessary to sustain the company’s operation and marketing functions (e.g., measuring promotion effectiveness, preventing returns, increasing incentive use, increasing cross-selling, detecting fraud, routing customers, among others) consistently adopt CAC as a specific action aimed at extracting conclusions from data about customer behavior to apply to decision-making. | 8. Companies that use CAC to develop natural routines of marketing operations in organizations (e.g., personalizing advertising and listening to the customer’s voice based on user-generated online content) view CAC as a systematic process for generating actionable insights to support efficient decision-making. However, they assume it has a greater scope than necessary to achieve this aspiration. | 9. Companies that develop skills to execute the operational routines of the marketing function maintain an abstract view of CAC as a creator of sustained competitive advantage (e.g., aspiring to build a customer engagement strategy based on CAC) but do not materialize the mechanism through which these skills are developed. |
Source: Authors’ own elaboration.
Regardless of the maturity level, profiles 3, 5, and 7 represent organizations that are consistent between their conception of analytics in customer contexts and the contribution they expect from it to the organization. In contrast, organizations of type 1 and 9 lack coherence and are at a higher risk of resource waste or failing to achieve the more strategic objectives attributable to using analytics in the customer domain.
4. Conclusions and future work
This study conducts a systematic literature review to conceptualize CAC through existing evidence on analytics in customer contexts, considering its purpose, nature, and uses reported by the 42 empirical studies that comprised the RLS.
The first research question focuses on the bibliometric characterization of analytics in customer contexts. It is found that 79% of the addressed studies belong to journals classified in Q1 and Q2, demonstrating the increasing relevance of the phenomenon addressed in this study. The prevailing areas of interest among the journals are marketing strategy and management. Most of them have a quantitative approach, and it is noted that the United States, India, and the United Kingdom lead the empirical development of analytics in customer contexts within organizations.
Responding to the second research question, various purposes, interpretations, and analytics uses in customer contexts are condensed. Limited purposes include improving customer routing in a call center (Ilk et al., 2020) or evaluating promotions (Khouja et al., 2020). At the same time, more ambitious scopes involve measuring the impact of marketing strategies on sales (Sohrabi et al., 2019), measuring brand health (Rajan, 2019), or determining the factors that affect customer satisfaction (Lee et al., 2020; Rajendran, 2021). The spectrum is equally broad for the reported uses of analytics in customer contexts. For example, analytics in customer contexts can be understood as a holistic process (Mariani and Wamba, 2020), a technology (Boldosova, 2020), an organizational capability (Louro et al., 2019), a practice/art (Lee et al., 2020), or a set of tools (Raeesi-Vanani, 2019), among others.
The third research question focused on the conceptions and roles of CAC that can be derived from the purposes, interpretations, and uses of analytics in customer contexts. The model presented by Pérez-Rave et al. (2022), which theorizes about analytics in general, serves as the reference framework that allowed the varied evidence found to be standardized and facilitated the identification of grouping patterns for these analytics elements in customer contexts. Such grouping was possible because consistency was found between the perspectives from which the nature (as action or method, complex process, or strategic resource) and the role (develop operational capabilities, dynamic capabilities, or adjust strategy) of analytics, in general, can be understood and the perspectives from which the nature and role of analytics in customer contexts in achieving competitive advantage in organizations can be understood. The evidence highlighted two significant contradictions that can occur: some organizations conceive analytics in customer contexts as a strategic resource but primarily expect it to develop operational capabilities, while others conceive it as an action or method and expect it to achieve organizational strategy reconfigurations.
The fourth research question relates to the taxonomic patterns that allow the classification of the nature of CAC. From the combination of the conceptions and roles mentioned above, nine organizational profiles emerge, reflecting differences in the ability to generate value from analytics routines in customer contexts. Organizations where the mentioned contradictions occur are less capable of generating value from deploying analytics routines in customer-related domains, as they either waste invested effort or fail to exploit its potential. Coherence between the conception of analytics in customer contexts and the roles it is expected to play in achieving competitive advantages favors the proper use of resources allocated to promoting it.
This finding allows for the development of a conceptualization of CAC under which they are conceived as a set of organizational routines specific to organizations that understand analytics in customer contexts as a process or a strategic resource and seek to generate dynamic capabilities and/or adjust the company’s strategy in pursuit of competitive advantages. Such conditions have observable manifestations, such as the implementation of a customer relationship system based on data collected from user interactions on the internet, the management of customer lifecycle value, and systematic monitoring of customer perceptions of the company’s brands (typical of the conception of CAC as a complex process) or understanding the variables that most affect business sales and measuring brand health through the sentiments of the digital audience (typical of the conception of CAC as a strategic resource). Adopting these manifestations, among others, under the named conceptions allows for creating capabilities, i.e., generating routines that contribute to value creation in organizations and thus help achieve sustained competitive advantages.
This taxonomy should become a reference point for developing organizational diagnostics related to CAC, helping to eliminate the inconsistency that the evidence often shows between what is understood and what is expected.
Future work could delve into understanding the mechanism through which CAC impacts organizational performance and explore and confirm the existing relationships between how CAC is conceived and its role in the organization.
5. Limitations
The studies under review were located through Scopus, the world’s most extensive abstract and citation database. Articles published in journals not indexed in Scopus may have been excluded. However, according to the guideline used by Arroyave et al. (2021), the number of excluded articles is expected to be minimal due to the richness of the mentioned database. The language of the included studies was English, so relevant articles published in other languages may have been excluded.