Services on Demand
Journal
Article
Indicators
-
Cited by SciELO
-
Access statistics
Related links
-
Cited by Google
-
Similars in SciELO
-
Similars in Google
Share
Ingeniería e Investigación
Print version ISSN 0120-5609
Ing. Investig. vol.28 no.2 Bogotá May/Aug. 2008
Fredy Andrés Olarte Dussan1, Carlos Arturo Clavijo Ramírez2 and Hernando Díaz Morales3
1 Ingeniero electrónico, Universidad Distrital Francisco José de Caldas, Colombia. M.Sc., en Automatización Industrial, Universidad Nacional de Colombia. Estudiante Doctorado, Ingeniería Eléctrica, Universidad Nacional de Colombia. faolarted@unal.edu.co
2 Biologo. M.Sc., en Bioquímica, Universidad Nacional de Colombia. Ph.D., en Farmacología y Toxicología Moleculares, University of Southern California, Estados Unidos. Profesor Asistente, Departamento de Biología, Facultad de Ciencias, Universidad Nacional de Colombia. caclavijor@unal.edu.co
3 Ingeniero electricista, Universidad Nacional de Colombia. M.Sc., Electric Power Engineering, Rensselaer Polytechnic Institute, Estados Unidos. Ph.D., Electrical Engineering, Rensselaer Polytechnic Institute, Estados Unidos. Profesor Titular, Departamento de Ingeniería Eléctrica y Electrónica, Universidad Nacional de Colombia, sede Bogotá. hdiazmo@unal.edu.co
ABSTRACT
Designing a robust control-based treatment for human immunodeficiency virus (HIV)-infected patients was studied. The dynamics of the immune systems response to infection was modelled using a 5th order nonlinear model with separate efficacy coefficients for protease inhibitor (PIs) and reverse transcriptase inhibitors (RTIs). The immune response has been represented as an uncertain system due to errors in parameter estimation and the existence of unmodelled dynamics. A polytopic system was constructed incorporating all possible system parameter values. A control system was designed using robust pole location techniques stabilising the polytopic system around an equilibrium point having a low viral load. Numerical simulation results (including the organisms pharmacokinetical response to anti-retroviral drugs) showed that the control law could lead to long-term stable conditions, even in extreme cases.
Keywords: robust control, polytopic system, HIV, AIDS, mathematical model, immune system dynamics.
RESUMEN
Este artículo describe el diseño de tratamientos para pacientes contagiados con virus de inmunodeficiencia humana (VIH), usando técnicas de control robusto. La dinámica de la respuesta del sistema inmune ante la infección se modela por medio de un sistema no lineal de quinto orden, el cual incluye diferentes coeficientes de eficacia para el inhibidor de proteasa (PI) y el inhibidor de transcriptasa reversa (RTI). Debido a los inevitables errores en la estimación de parámetros y a la existencia de dinámicas no modeladas, la respuesta inmune se representa por medio de un sistema con incertidumbre: se construye un sistema politópico que incorpora todos los valores posibles de los parámetros del sistema. Se diseña un controlador para este sistema, usando técnicas robustas de localización de polos, para estabilizar el sistema politópico alrededor de un punto de equilibrio con carga viral muy pequeña. Los resultados simulados, incluyendo la respuesta farmacodinámica del organismo, muestran que la ley de control así diseñada conduce a una condición estable en el largo plazo, aun en casos extremos de variación de parámetros.
Palabras clave: control robusto, sistema politópico, VIH, sida, modelos matemáticos, dinámica del sistema inmune.
Recibido: marzo 7 de 2008
Aceptado: junio 23 de 2008
Introduction
The HIV-AIDS epidemic is sweeping the world. A report by UNAIDS (United Nations Agency for the control of AIDS) pointed out that by the end of the year 2007 more than 33 million people were affected by acquired immune deficiency syndrome (AIDS) around the world, 2.5 million were new caes and almost 2.1 million people had died as a consequence of this pandemic (UNAIDS 2006). Even though currently there are no means to completely eradicate HIV from an infected person, progress has been made in terms of increasing these patients life expectancy. This has been mainly due to using drugs having antiretroviral activity. Drugs approved by the US Food and Drug Administration include 11 reverse transcriptase inhibitors (RTIs) and 9 protease inhibitors (PIs). RTIs block the activity of the viral enzyme necessary for virus replication; PIs block enzymes whose activity is necessary for virion maturation, thus resulting in non-infective viral particle production (Simon et al., 2006).
HIV has a remarkable ability to generate genetic variants called quasi-species amongst the viral population present in an infected individual (Heeney et al., 2006). It has been postulated that this ability lies in three main characteristics: high viral replication rate, low reverse transcriptase accuracy and genetic recombination ability. A large amount of quasi-species in an infected individual increases the risk of drug resistance developing, thereby implying that (when possible) a low viral load should always be maintained through therapeutic treatment. This premise has been implemented by the combined use of several potent antiretroviral drugs in socalled highly active antiretroviral therapy (HAART), leading to very positive results in developed countries in terms of reduced morbidity and mortality (Schwartlander et al., 2006).
Whereas it is clear that a patient in the diseases chronic phase should start HAART as soon as possible, there is no consensus regarding such timing in asymptomatic HIV-infected patients, considering the complications arising from long-term use of anti-retroviral drugs. Therapeutic schemes having a programmed schedule of periods of drug treatment separated by periods without it have been proposed to reduce HAARTs side-effects (structured treatment interruptions STIs) (Montaner et al., 2006).
A further evolution of this approach has predicted that STI use induces the gradual and sustained recovery of an infected individuals immune system in a process mimicking the long-term non-progression (LTNP) status of some (5-10%) infected patients (Sankaran et al., 2005). LTNP status in an HIV-infected patient is defined as the lack of AIDS symptoms for more that seven years in the absence of treatment (Sankaran et al., 2005).
The experimental conditions necessary for inducing a patients immune system to reach LTNP status are not easy to determine and a proper modelling system would therefore be extremely valuable. This paper reports developing a dynamic HIV treatment based on robust control principles.
Numerous mathematical HIV infection models have been developed to account for different aspects of the process. Some well-known examples are presented in (Wodarz and Nowak 1999, Perelson and Nelson 1999, Kubiak et al., 2001, Wein et al., 1997). It has been recognised since the late 1990s that the problem of designing treatment strategies is basically a control problem (Wein et al., 1998). The first approaches were directed at finding optimal controls allowing the system to reach LTNP status while minimising some objective functions which might have been viral load or some quadratic (LQR-type) performance function (Kirschner 1996). It soon became evident that the processs inherent uncertainty required a robust control design. Hence, robust controllers were developed using different approaches such as model predictive control (MPC) (Zurakowski and Tee 2006). A method has been recently proposed which uses an observer-based feedback scheme to achieve robust stability (Alvarez-Ramirez et al., 2000). Some works have considered constant dose treatments from a bifurcation point of view (Kim et al., 2005).
Others have considered system controllability to derive useful results about HIV treatments (Jeffrey et al., 2003, Ko et al., 2006). This paper considers the problem of designing a robust treatment schedule from the point of view of stabilising differential inclusions defined as polytopic linear systems (Boyd et al., 1994).
The immune system
The human body has several lines of defence against potential invaders including tissue layers such as the skin or specialised tissue surfaces such as the mucosa. Those pathogens managing to cross those primary layers are the subject of attack by an army of specialised cells forming the so-called innate immune system and include granulocytes and macro-phages. These cells constitute our immune systems first line of defence and can normally neutralise several microorganism-induced infections in a complex series of events leading to the inflammation associated with infection. When a pathogen or foreign agents presence persists, a second group of specialised cells develop an adaptive immune response which is characterised by the ability to specifically recognise the pathogen or foreign agent (now called antigen) and provide enhanced protection against reinfection. The types of cells responsible for the adaptive immune response include B- and T-lymphocytes which require antigen-presenting cells (such as dendritic cells) for their function. Both innate and adaptive immune responses work in an integrated way to protect our organism from potential threats (Zinkernagel 2005). The mechanism by which innate response can neutralise a pathogen depends upon the cell involved. B-lym-phocytes specialise in secreting proteins called antibodies which can specifically bind to pathogens or toxins and block their entry to susceptible cells. Those pathogens covered by antibodies are more easily ingested and destroyed by phagocytic cells such as macrophages. A T-lymphocyte subclass (cytotoxic T-lymphocytes or CTLs) operates via direct contact with specific antigens on the surface of virally infected cells. CTLs induce programmed cell death in such infected cells and, by so doing, prevent further expansion of the attacking virus. CTLs typically express the CD8 molecule on their surfaces and they are thus named CD8+lymphocytes. Yet another type of T-lymphocytes (T-helper cells), characterised by expressing the CD4 molecule on their surfaces (CD4+), have the role of activating other cells such as B-lymphocytes for antibody secretion or macrophages for active destruction of intracellular bacteria (Janeway et al., 2006).
HIV and the immune system
The HIV-1 virus is the causative agent of AIDS, a disease characterised by three distinguishable stages. The virus can invade different kinds of cells during acute infection (macro-phages and dendritic cells, in addition to their more conventional host, the T-lymphocyte). This stage is characterised by high levels of circulating virions (viremia) and is followed by an asymptomatic phase which can last for years or even decades. CD4+ lymphocytes are gradually destroyed by the acute infection phase reaching critical levels during the third infection phase (chronic) when the syndrome symptoms become manifest (Heeney et al., 2006).
HIV-1 is a retrovirus (i.e., its genetic material is RNA which is transcribed into DNA by enzyme reverse transcriptase (RT) once inside the host cell). HIV-1 infects CD4+ T lymphocytes causing a loss of this important cell population, correlating with acute viremia. Infected CD4+ T-lymphocytes are eventually killed by CTLs after antigen recognition; however, HIV infection is not eliminated by this immune response. HIV sets up a persistent process of replication in newly-infected cells inducing a progressive reduction of circulating CD4+ T cells correlating to the adaptive immune systems reduced ability to withstand opportunistic pathogens. A minor population of HIV-infected individuals develops LTNP status, although the mechanism responsible for this behaviour has not been made completely clear.
Mathematical model of HIV response
The Wodarz-Nowak model (Wodarz and Nowak 1999), adapted by Kubiak (Kubiak et al., 2001) was used here, with the addition of separate control signals for RTIs and PIs actions (Ko et al., 2006, Kim et al., 2005). The complete model can be described as follows: Let x, y, v, w, z represent uninfected CD4+cell, infected CD4+ cell, free virus, precursor cytotoxic cell and effector cytotoxic cell concentrations, respectively. Free viruses are produced at rate k (1-ηP)y and eliminated at rate µv. β is an infection rate. Precursor cells are produced at rate cqyw. Effectors kill infected cells at rate py z. ηR and ηP are the efficacy coefficients for RTIs and PIs (antiretroviral drugs), respectively. The dynamic model is thus:
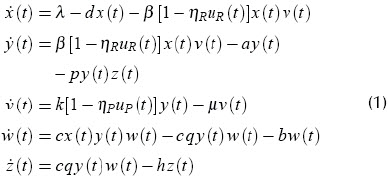
Table 1 shows parameter values suggested by (Kubiak et al., 2001), where λ is the uninfected CD4+ cell production rate, β(1-ηR)xv gives the rate of infection. d, a, b and h are the natural death rates of x, y, w and z, respectively.
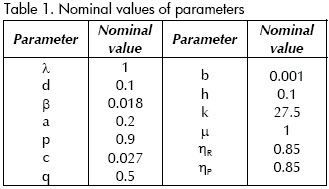
The nonlinear system (1) has four equilibrium points, when control inputs are constant. The first one is at the origin, an uninfected status. The second equilibrium is an unstable one and, hence, of no interest
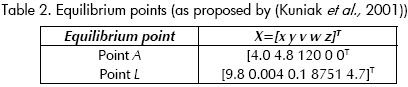
here. The other points represent clinically meaningful conditions:
Point A,
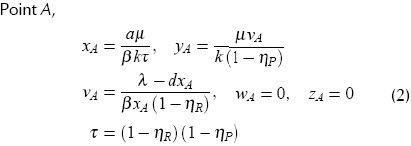
This is a stable point with high viral load (v) and immune system failure. It represents a patients AIDS condition.
Point L
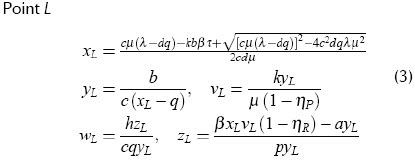
This point corresponds to an LTNP status; it is stable without medication. The concentration of infected cells (x) and viral load (v) are low, while precursor cytotoxic cells (w) are abundant.
Linearisation
Equation (1) is linearised around equilibrium point L. The resulting linear system is given by

where X = [x y v w z]T is a state vector, U = [uP uR]T is a control input vector, y is a vector of measured (output) variables. Matrices A, B, C and D were obtained by differentiation.
Uncertainty
The nonlinear model (1) depends on a number of unknown parameters which must be estimated from clinical experiments. These values may vary from one patient to another and are never known exactly.
The linear model (4), whose matrices depend on nonlinear model parameters, therefore becomes an uncertain system.
This uncertain system will be modelled as a parameterised system:

where p is a vector of all parameters which are not exactly known.
Polytopic models
If unknown parameter values are assumed to vary independently and if variation ranges are estimated for each one, then the uncertain system can be represented as a polytopic model (5), where the system matrices
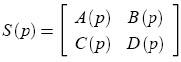
vary within a convex polytope:
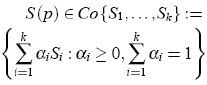
where S1, . . . ,Sk are vertex system matrices, given by extreme values of the parameter set. S(p) is a convex combination of the extreme matrices S1, . . . ,Sk. Figure 1 illustrates the concept of a polytopic model.
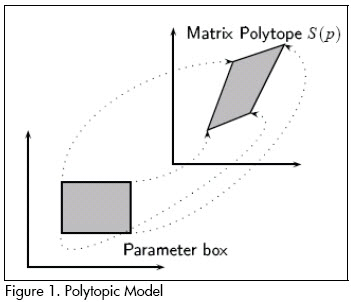
Polytopic HIV model
A polytopic model for HIV dynamics was constructed as follows:
1). Five of the most significant HIV model (1) parameters were selected and their extreme values were estimated. The parameters variation range is shown in Table 3. The table contains one parameter (τpk) which is not a part of the model. It will be used to represent (un-modelled) drug pharmacokinetics time constant in our simulations. A full parameter set polytope is not feasible (it has 213 = 8,192 vertices!).
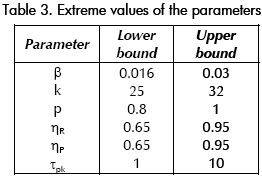
2). A linearisation of 1) was calculated for each of the parameter sets 32(= 25) vertices.
3). A polytopic system was constructed from the 32 vertex systems.
Designing the H ∞ controller
Several feedback controllers were designed for linearisation of form (4) system (1) around equilibrium point L. They included state variable feedback and output feedback. A controller having particularly good results was a state variable feedback law, u = Kx designed in such a way that:
-It guaranteed that the infinity norm of T(s) (closed loop transfer matrix) was kept below a specified limit γ > 0, i.e., llT(s)ll∞ < γ.
-Closed loop poles were in some region .
Poles were placed in a generalised stability region of the complex plane: a convex subset of C defined by linear matrix inequality (LMI) (Boyd et al., 1994):

where M and L = LT were fixed real matrices and the bar indicates complex conjugation. Very general regions can be described this way.
The controller design problem can be formulated as an LMI problem. Nominal performance (llTll∞>γ) and pole placement in D can be guaranteed (see Chilali and Gahinet 1996) if there are matrices K and X XT 0 (i.e., X is a positive definite matrix) satisfying the LMI:
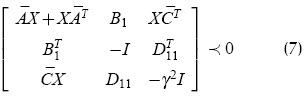
where sign indicates that the matrix on the left-hand side is a negative definite matrix.
Robust controller design
It is desirable that the control law leading to a patients treatment strategy be effective regardless of actual parameter values and the diseases initial state when therapy were initiated. Such robustness requirement was pursued in this work by considering an uncertain system described by a polytopic linear system of the form (5). A polytope, including all values for the parameters considered plausible, was produced, constituting the uncertainty set for the HIV-model.
Given the polytopic system defined by a k-vertex polytope of linear systems, a state feedback gain was found stabilising all systems in the polytope (Boyd et al., 1994). This became a system of LMIs including one inequality of the form (7) for each polytope vertex; it could be shown that this was a convex problem and could thus be solved quite efficiently by existing optimisation algorithms. In the uncertainty model used here, k = 25 = 32.
Simulation results
A nonlinear system (1) was simulated which included dynamic modelling of drug pharma-cokinetics. The model was a linear first-order differential equation with time constant τpk. A controller was obtained satisfying all requirements descrybed above; it will be referred to as SF-MO. Another controller (named SF) was designed using the nominal model to achieve pole placement for the nominal closed loop system. This controllers performance was similar to the robust controller when all parameters were at their nominal value.
The objective of closed-loop controller-based treatment design was to drive the state of an HIV-infected person, not yet in the AIDS condition (equilibrium point A), to an LTNP status (point L). A successful therapy design must consider the following:
1). Low concentrations of uninfected CD4+ cells accompanied by high concentrations of free viruses are highly undesirable. Opportunistic infections and/or drug resistance may appear;
2). Treatment objective is to induce an LTNP condition. Medication is therefore applied for a limited time; the immune system is expected to take command and maintain LTNP status from there;
3). Cytotoxic cell concentration determines how the immune system responds. A successful treatment must therefore keep their concentration high; and
4). Clinical implementation of treatment recommendation uses discrete drug dose values. A continuously varying dose, corresponding to a continuous control signal, represents a slow variation of anti-retroviral. This strategy can lead to the appearance of drug-resistant strains due to viral mutation (Stengel et al., 2002, Kim et al., 2005, Bonhoeffer et al., 2000). A full dose (binary signal) is thus preferred; such discontinuous control action was simulated using a threshold-comparator mechanism. Other discretisation schemes have been tried, but the results are not noticeably affected.
The simulation results presented here used an initial state very close to the AIDS condition (equilibrium point A): Cin = [x0, y0, v0, w0, z0] = [0.4 3.5 95 0.001 0.001]. This initial condition was found to be a very severe one and most controllers had difficulty driving the system to point L from there. Control action was discontinued after a year of treatment in all simulations.
Nominal system
The first analysis concerned linear approximation relevance to (1). All simulations were done at this stage with parameters at their nominal values. Figures 2 and 3 show nominal system response to the SF-MO control law. CD4+ concentration became high after a few days whilst viral load became reduced. A slight T-lymphocyte reduction followed but the immune system was able to sustain stable conditions near point L. Figure 5 shows control input. Notice that the input followed an STI-like pattern. Cytotoxic cell evolution is displayed in Figure 4.
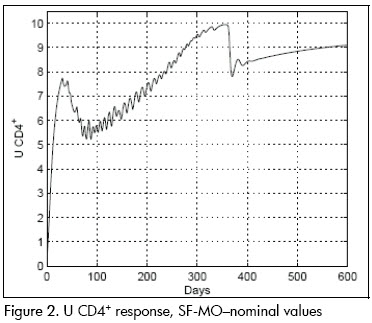
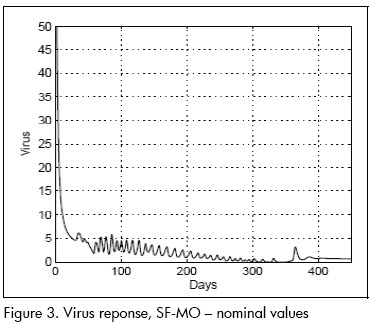
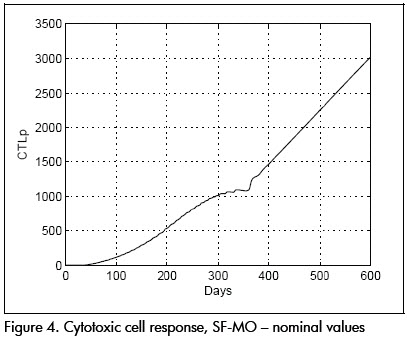
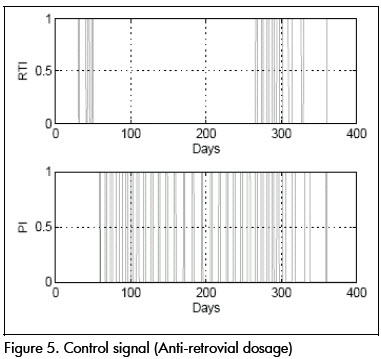
As a comparison, closed loop system response using SF controller is shown in Figures 6 and 7. Notice that therapeutic success was achieved in this case also. Both controllers produced similar results for the nominal system.
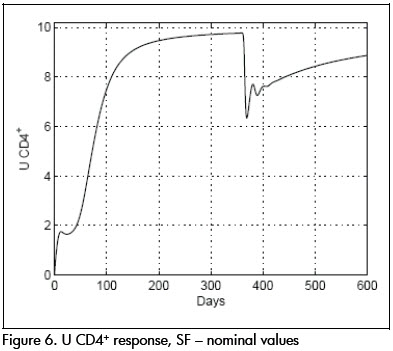
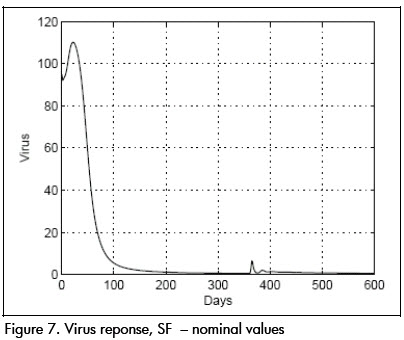
Robustness
Parameters were modified from their nominal values and controller effects on the nonlinear system were compared for analysing design robustness.
Parameters were then changed to condition Π1 given by: β= 0.022, p = 0.8, k = 32, ηR = 0.65, ηP = 0.65, τpk = 5 and the SF-MO controller was applied. Viral and CD4+ cell concentrations dynamic responses are shown in Figure 8. The response was slower than in the nominal case, achieving target state after about 70 days, approaching point L. CTLp concentration remained high, as can be seen in Figure 9.
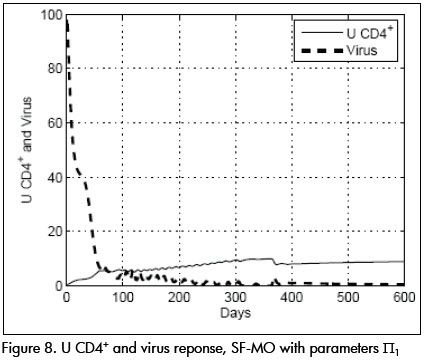
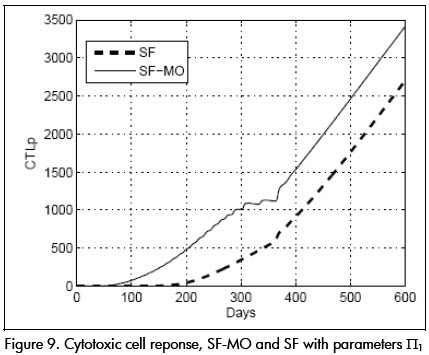
Controller SF, on the other hand, produced a very different response for these parameter values. Viral load grew rapidly to a peak value of 150 units, as seen in Figure 10. The time necessary for achieving condition (I) was around 200 days, a period of more than six months during which the patient was at risk of attack by opportunist infection.
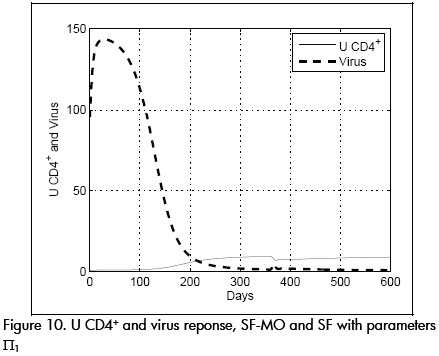
CTLp concentration remained dangerously low during this time (see Figure 9).
In another experiment, parameter values were fixed at a value at the limit of the uncertainty model Π2 given by: β= 0.03, p = 1, k = 33, ηR = 0.65, ηP = 0.75, τpk = 8. System performance using controller SF-MO is presented in Figure 11. This controllers ability to drive the system to state L is once again shown. Controller SF, on the other hand, could not stabilise the system around the desired point L (Figure 12.)
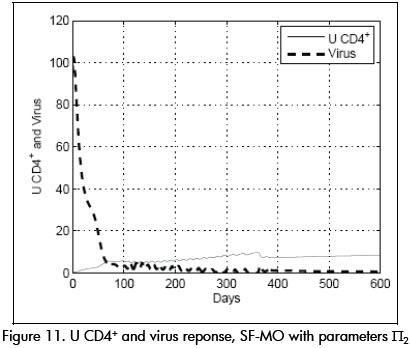
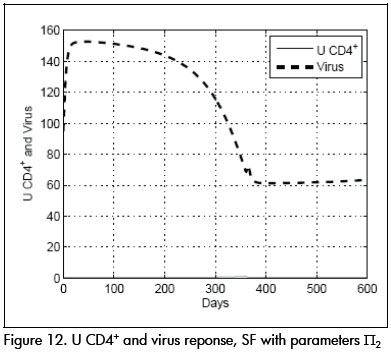
A very interesting and illustrative case was produced when pharmacokinetic time constant was substantially reduced; parameter values (Π3) were: β=0.016, p=1, k=30, ηP= 0.65, ηR =0.98, τpk =0.7.
System response to controller SF was very good as long as drugs were being administered and a reduced viral population was reached after a few days. However, when the drug was discontinued, the immune system practically crashed and was unable to maintain condition L. The response is shown in Figure 14.
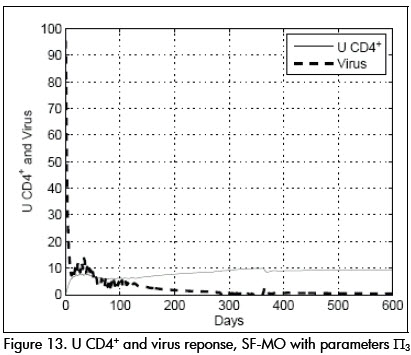
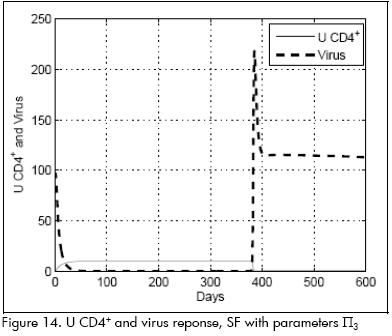
Instead, controller SF-MO produced a very good stable response and condition L was stable (Figure 13).
Conclusions and future work
A robust control-based treatment strategy was proposed stabilising nonlinear HIV dynamics around a long-term non-progressive state for a wide range of parameter variations. The uncertain system was modelled as a family of linear systems having varying parameters (i.e. a polytopic model). To the best of our knowledge this approach to HIV infection dynamics uncertainty modelling is new.
The problem of control system design leading to the treatment law was formulated as a linear matrix inequality problem. A solution was obtained using standard numerical tools. The results were tested on a full nonlinear simulation of the system including the effects of drug pharmacokinetics; the discretisation accounting for the drug dosages binary nature was also modelled in the simulation.
The simulations showed that the robust control law was very effective at stabilising the nonlinear system around point L, identified by a long-term non-progressive condition (stable immune system condition).
The control law was compared to alternative designs and found to be robust in the presence of parameter variation and un-modelled pharmacokinetic dynamics. The resulting drug dosage control signals took the form of structured treatment interruption therapy, a desirable characteristic from a clinical point of view.
The results of many simulations suggested that LTNP status could be induced, even in patients suffering from a very severe condition (Olarte et al., 2007). It was observed that many cases where STI treatment failed could be stabilised and returned to equilibrium point L, even when initial conditions were very close to the AIDS condition with a properly-designed treatment.
Of course, all results will have to be validated by clinical experiments before any definitive conclusion can be applied to therapy design. The control law is a closed-loop one, requiring measurement of patients state. The systems observability is currently being analysed from the available measurements to develop an observer allowing implementation. The observer-based controller robustness is also being studied. O-her un-modelled effects may also be considered in the simulations, such as time delays in effector cell activation.
Bibliografía
Alvarez-Ramirez, J., Meraz, M., Velasco-Hermandez, J., Feedback control of the chemotherapy of HIV., Int. J. of Bifurc. Chaos, 10, 2000, pp. 22072219. [ Links ]
Bonhoeffer, S., Rembiszewski, M., Ortiz, G. M., Nixon, D. F., Risks and benefits of structured antiretroviral drug the-rapy interruptionsin HIV-1 infection., AIDS, 14, 2000, pp. 23132322. [ Links ]
Boyd, S. P., El Ghaoui, L., Feron, E., Balakrishnan, V., Linear Matrix inequalities in system and control theory., SIAM, Philadelphia, 1994. [ Links ]
Chilali, M., Gahinet, P., H? design with pole placement constraints: an LMI approach. IEEE Trans., Automat. Contr, 41:358367, 1996. [ Links ]
Heeney, J. L., Dalgleish, A. G., Weiss, R. A., Origins of HIV and the evolution of resistance to AIDS., Science, 313, 2006, pp. 462466. [ Links ]
Janeway, C. A., Travers, P., Walport, M., Shlomchik, M., Immunobiology., Garland Publishing, 5th edition,2006. [ Links ]
Jeffrey, A. M., Xia, X. , Craig, I. K., When to initiate HIV therapy: a control theoretic approach., IEEE Trans. Biomed. Eng., Vol. 50, No. 11, Nov. 2003, pp. 1213 1220. [ Links ]
Kim, J., Kim, W. H., Chung, H. B., Chung, C. C., Constant drug dose leading long-term non-progressor for HIV-infected patients with RTIs and PIs., In Proc. 44th IEEE Conference on Decision and Control, and the European Control Conference, Dec. 2005. [ Links ]
Kirschner, D., Using mathematics to understand HIV-immune dynamics., Notices Am. Math. Soc., 43, 1996, pp. 191202. [ Links ]
Ko, J. H., Kim, W. H., Chung, C. C., Optimized structured treatment interruption for HIV therapy and its performance analysis on controllability., IEEE Trans. Biomed. Eng., Vol. 53, No. 3, 2006, pp. 380 386. [ Links ]
Kubiak, S., Lehr, H., Levy, R., Moeller, T., Parker, A., Swin, E., Modeling control of HIV infection through structured treatment interruptions with recommendations for experimental protocol., Technical Report Tech. Rep. (CRSC-TR01-27), CRSC, http://www.math.montana.edu/~parker, 2001. [ Links ]
Montaner, J. S., Hogg, R., Wood, E., Kerr, T., Tyndall, M., Levy, A. R., Harrigan, P.R., The case for expanding access to highly active antiretroviral therapy to curb the growth of the HIV epidemic., Lancet, 368, 2006, pp. 531 536. [ Links ]
Olarte, F. A., Clavijo, C. A., Diaz, H., Robust control of HIV-treatments using a polytopic model., In Proceedings, European Control Conference - ECC-07, 2007. [ Links ]
Perelson, A. S., Nelson, P. W., Mathematical analysis of HIV-1 dynamics in vivo., SIAM Review, Vol. 41, No. 1, 1999, pp. 344. URL citeseer.ist.psu.edu/perelson98mathematical.html. [ Links ]
Sankaran, S., Guadalupe M., Reay, E., George, M. D., Flamm, J., Prindiville, T., Dandekar, S., Gut mucosal T-cell responses and gene expression correlate with protection against disease in long-term HIV-1-infected nonprogressors., PNAS, Vol. 102, 2005, pp. 9860 9865. [ Links ]
Schwartlander, B., Grubb, I., Perriens, J., The 10-year struggle to provide antiretroviral treatment to people with HIV in the developing world., Lancet, 368, 2006, pp. 541546. [ Links ]
Simon, V., Ho, D. D., Abdool-Karim, Q., HIV/AIDS epidemiology, pathogenesis, prevention, and treatment., Lancet, 368, 2006, pp. 489504. [ Links ]
Stengel, R., Ghigliazza R., Kulkarni, N., Optimal enhancement of immune response. Bioinformatics., Vol. 18, No. 9, Mar. 2002, pp. 1227 1235. [ Links ]
UNAIDS., 2006 report on the global AIDS epidemic. Technical report, UNAIDS, http://www.unaids.org/-en/HIV_data/2006GlobalReport/default.asp, 2006. [ Links ]
Wein, L. M., Zenios, S. A., Nowak, M. A., Dynamic multidrug therapies for HIV: A control theoretic approach., J. Theor. Biol., Vol. 185, 1997, pp. 1519. [ Links ]
Wein, L. M., DAmato, R., Perelson, A., Mathematical analysis of antiretroviral therapy aimed at HIV-1 eradication or maintenance of low viral loads., J. Theor. Biol., 192, 1998. pp. 8198. [ Links ]
Wodarz, D., Nowak, N. A., Specific therapy regimes could lead to long-term immunological control of HIV., PNAS, Vol. 96, No. 25, 1999, pp. 1446414469. [ Links ]
Zinkernagel, R. M., Immunology and immunity against infection: General rules. Journal of Computer Applications of Mathematics., Vol. 184, 2005, pp. 49. [ Links ]
Zurakowski, R., Tee, A. R., A model predictive control based scheduling method for HIV therapy., Journal of Theoretical Biology, 238, 2006, pp. 368382. [ Links ]