Services on Demand
Journal
Article
Indicators
-
Cited by SciELO
-
Access statistics
Related links
-
Cited by Google
-
Similars in SciELO
-
Similars in Google
Share
Ingeniería e Investigación
Print version ISSN 0120-5609
Ing. Investig. vol.33 no.3 Bogotá Sept./Dec. 2013
H. M. Usgame Sandoval1, C. A. Pedraza Ramirez2 and J. E. Quiroga Méndez3
1Hector M. Usgame Sandoval. Mechanical Engineer, Universidad Industrial de Santander, Colombia. E-mail: hemaus2@hotmail.com
2Camilo A. Pedraza Ramírez. Mechanical Engineer, Universidad Industrial de Santander, Colombia. E-mail: camilopedraza@hotmail.com
3Jabid Quiroga. Mechanical Engineer, Universidad Industrial de Santander, Colombia. MSc in Mechanical Engineering, Florida State University, USA. Affiliation: Universidad Industrial de Santander, Colombia. E-mail: jabib@uis.edu.co
How to cite: Usgame, H. M.., Pedraza, C. A., Quiroga, J., Acoustic emission-based early fault detection in tapered roller bearings., Ingeniería e Investigación, Vol. 33, No. 3, December 2013, pp. 5 - 10.
ABSTRACT
This paper proposes an acoustic emissions-based method for monitoring tapered roller bearings. This method monitors tracking time-based fault indicators (i.e. RMS, peak value, ring-down count and kurtosis) obtained using the AE signal. Experiments were carried out on a dedicated test bench for different levels of tapered roller bearing outer and inner race defect severity. Although the fault indicators studied could not discriminate between outer and inner raceway defects, the experimental results highlighted the proposed indicators' tapered roller bearing fault detection and fault severity assessment ability (i.e. peak value, RMS and ring-down count).
Keywords: acoustic emission, bearing fault detection, bearing monitoring.RESUMEN
En este artículo se propone el uso de las emisiones acústicas (EA) para el monitoreo de rodamientos cónicos. Este monitoreo se realiza a través del seguimiento de los indicadores de falla: RMS, valor pico, ring-downcount y curtosis, los cuales son obtenidos a partir de la caracterización de la señal de EA en dominio del tiempo. La experimentación se realiza en un banco dedicado para este tipo de pruebas, en el cual, se experimenta con los rodamientos cónicos bajo distintos niveles de severidad de falla en la pista externa e interna. Aunque no es posible distinguir claramente las fallas en la pista interna y externa usando los indicadores propuestos, algunos resultados experimentales mostraron que el valor pico, el RMS y el conteo de picos de la señal de emisiones acústicas, permiten detectar y estimar la severidad de la falla en rodamientos cónicos.
Palabras clave: emisiones acústicas, detección de fallas en rodamientos, monitoreo de rodamientos.
Received: February 29th 2012 Accepted: October 2th 2013
Introduction
High operational reliability is expected in productive systems to satisfy production requirements. Ideally, any machine's abnormal functioning must be detected during its early stages to successfully avoid disturbances, failures, breakdowns and unplanned shutdowns. Well-developed condition monitoring techniques enable monitoring any system's actual condition; they allow maintenance resources to be prioritised and optimised for obtaining the maximum useful life for each physical asset before taking it out of service. A condition monitoring system is usually able to determine a system's current state by using information processed online regarding vibration, stator currents, temperature, etc.
The trend towards increasing power, complexity and efficiency regarding rotating machinery has limited rolling element design and early fault identification as requirements for ensuring reliable operation. Mass imbalance, misalignment and overload are common problems concerning rotating machinery, thereby reducing bearing service life. Different methods have been used for detecting and diagnosing bearing defects; current techniques can be classified as vibration or acoustic measurements. Vibration monitoring of bearings is probably the most established diagnostic technique for rotating machinery. Vibration is predominantly analysed in the frequency domain; this provides enough information for estimating fault location (i.e. inner race, outer race, rolling element and retainer) but is not suitable for detecting incipient faults. Acoustic emission (AE) is increasingly being used as a complementary technique in the time domain for bearing diagnosis; however, limitations in processing, interpreting and classifying the acquired data have limited its successful application and extensive use. The advantage of AE monitoring over vibration monitoring is that the former can detect the growth of subsurface cracks, whereas the latter can only detect defects when they appear on the surface. Eftekharnejad et al., (2011) demonstrated that AE was more sensitive in detecting incipient damage than vibration. It should be noted that the energy released to the bearings by neighbouring components is below 50 kHz vibrational frequency. This frequency range often masks the vibrational energy released by a defective rolling element bearing. Such frequency range does not affect AE monitoring because the AE frequency emitted by a faulty bearing is in a higher frequency range (Tandon and Choudhury, 1999).
Tandon and Nakra (1996) presented a detailed review of vibration and acoustic methods, such as vibration measurement in time and frequency domains, sound measurements, the shock pulse method and the AE technique for monitoring rolling bearing condition. Several studies have investigated defective bearings' AE response. Bagnoli et al., (1988) and McFadden and Smith (1984) dealt with AE monitoring of rolling bearing defects. Yoshioka and Fujiwara (1982, 1984) have shown that AE parameters can detect defects before they appear in the vibration acceleration range and can also detect possible sources of AE generation during a thrust ball bearing fatigue life test. Experiments have shown that analysis of acoustic emissions captured in bearings was able to detect defects in the form of a fine scratch on the inner race of angular contact ball bearings axially loaded at low speeds (McFadden and Smith, 1984). Tandon and Nakra (1990) have demonstrated the usefulness of some AE parameters (such as peak amplitude and count) for detecting defects in radially loaded ball bearings at low and normal speeds. Abdullah M et al., (2006) have reported using AE for identifying the presence and size of a defect on a radially loaded bearing. This paper showed that the fundamental source of AE in seeded defect tests was due to material protruding above mean surface roughness.
The present work used peak value, root mean square (RMS), kurtosis, ring-down count for detecting and evaluating the level of defective bearing severity via AE signals. Experimental results showed that some of the aforementioned indicators provided information for the early detection of the onset of faults. The results also indicated a direct relationship between signal features and defect size, thereby providing a diagnostic tool which can be used for effectively quantifying a detected defect.
Using AE for monitoring bearing condition
AE involves external stimuli (such as mechanical loading, defect formation and irreversible failure) in structural materials under mechanical or thermal stress producing transient elastic waves. These waves are generated by the rapid release of energy within a solid material, impacts and/or friction. Crack generation and propagation are the main source of AE. AE sources act as focal sources and irradiate high frequency energy in spherical waves. Most AE emitted energy is observed at 100 kHz to 1 MHz frequency. AE instrumentation consists of a transducer (usually piezoelectric type), a preamplifier and a signal processing unit. The most commonly measured AE parameters in the time domain are ring-down count, events, energy and peak amplitude. Ring-down count is the number of times a burst signal crosses the detection threshold within a fixed period of time. An event consists of a group of ring-down counts and represents a transient wave. Figure 1 shows AE signal time domain parameters.
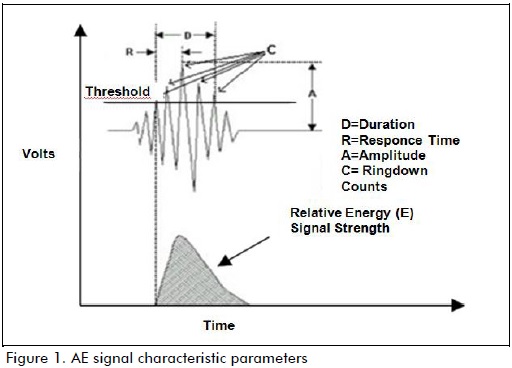
Some statistical quantities can be used as AE parameters, such as the standard deviation, kurtosis, variance and RMS. Kurtosis (K) is the degree of distribution peakedness, representing the fourth moment of a data set or population (1):

where is the mean, is signal variance, E expectation and N is the signal number of samples. Peak value is found using (2):

where is AE signal. RMS is determined using (3).
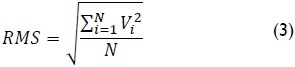
Bearing defects may arise during operation by surface crack formation attributed to induced stress at the rolling contacts, races and particles. There are two main sources of particles in bearings, particles produced by wear and those introduced from outside due to a faulty seal.
Experimental
This section describes the test bench used for the experiments and validating the proposed fault detection method. The test bench was specifically designed for fault detection and diagnosis, especially for tapered roller bearing faults. Figure 2 gives an illustration of this test bench; its components are illustrated in Table 1. The bearings were affected to emulate fault conditions; different levels of severity were produced by eroding the internal and external bearing races. The AE signal was captured by piezoelectric transducer having a low-noise FET input and an oscilloscope.
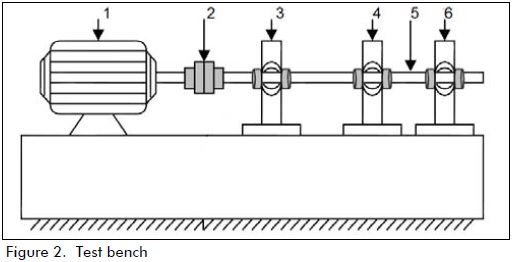
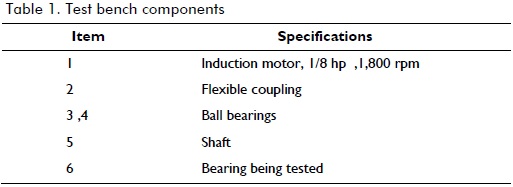
The sensor was attached close to the bearing to avoid losses in energy transmission. Intimate required mechanical contact was achieved by using films of grease between the transducer and the bearing. Figure 3 shows the test bench. AE sensor specifications were: 40 ± 1 dB gain, 30 dB peak sensibility (ref 1 V / mBar) and > 80 dB dynamic range.
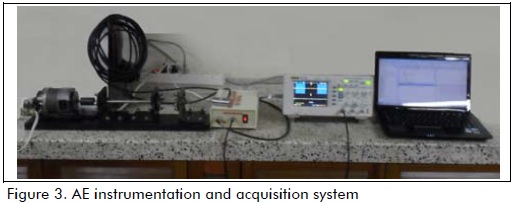
The acquisition system involved using an oscilloscope (RIGOL DS1102E). The AE signal was sampled at 2.5 MHz. The data was processed using Matlab environment (as shown in Figure 4).
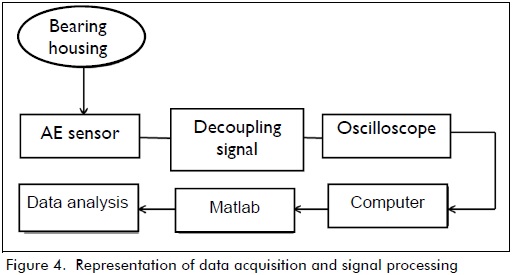
A 30203 tapered roller bearing was used in the experiments; this type of bearing was chosen because it is simple to assemble or disassemble and the races are thus available for producing the fault condition. Experimentation in this study involved using unloaded bearings in normal conditions (N0) and unloaded bearings having spot defects and different levels of outer and inner race defect severity (N1, N2 and N3). The different levels of severity are described in Table 2 and Figure 5 a) and b).
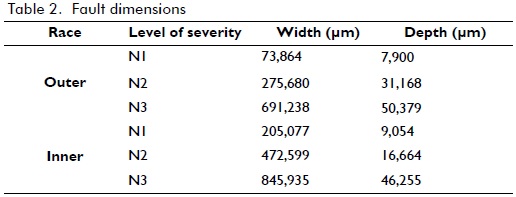
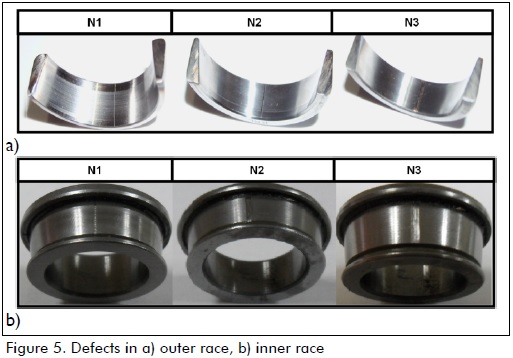
A digital microscope (HIROX KH-7700) was used for measuring each fault's depth and width (Table 2); Figures 6 and 7 show the images acquired for each bearing. The baseline was determined using a bearing in normal condition. The values of the parameters used in this study for each fault condition were compared with the baseline to determine AE characteristics associated with the three fault scenarios (i.e. outer and inner race faults); time domain data was captured and averaged for each fault scenario. Figures 8-14 show the AE signal for each scenario studied here.
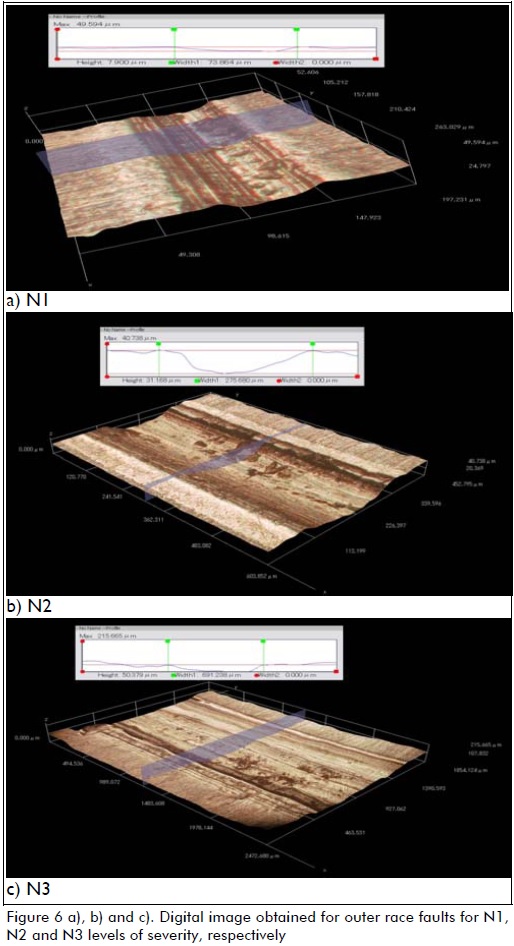
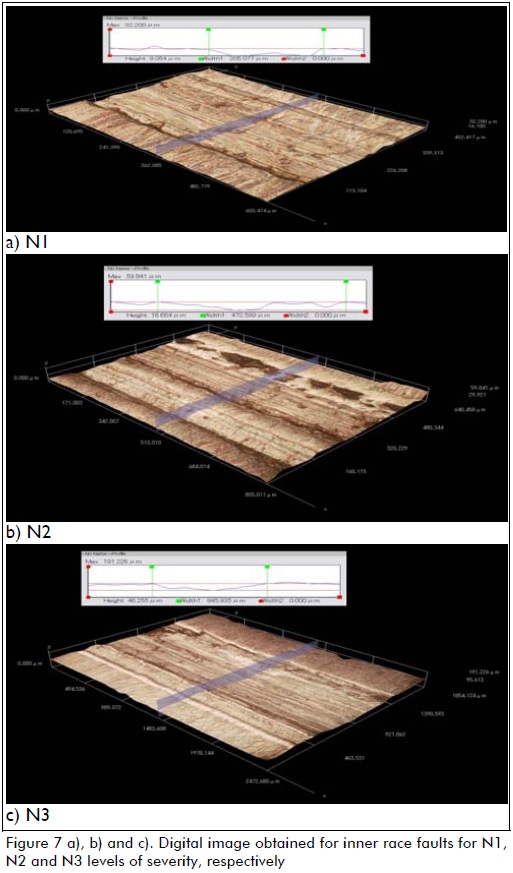
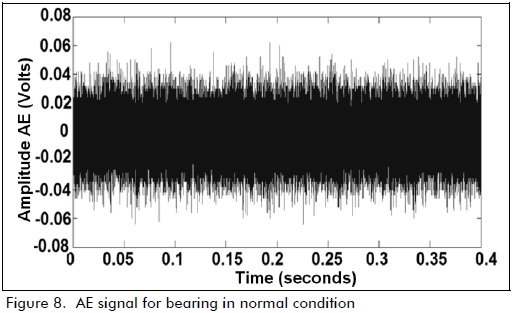
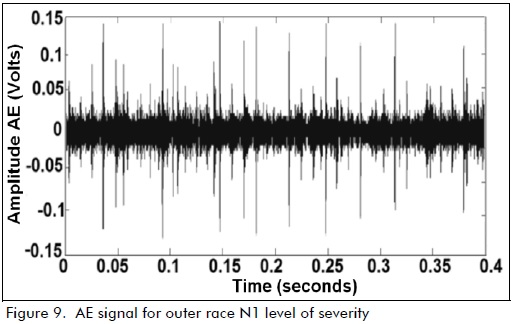
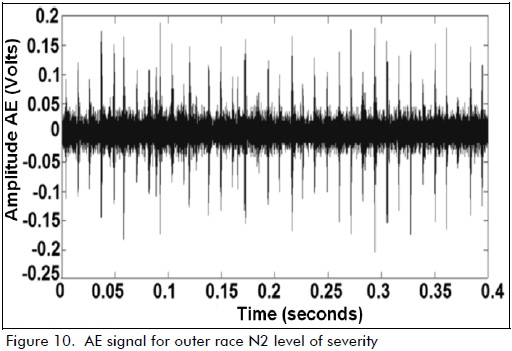
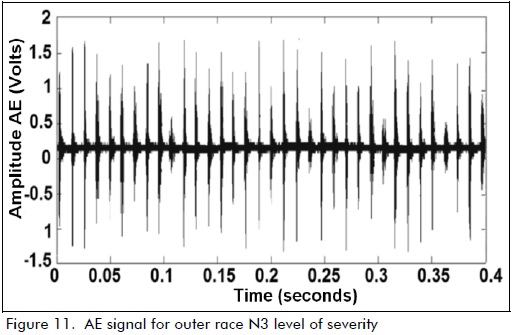
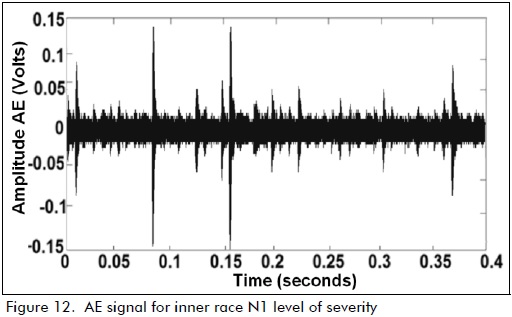
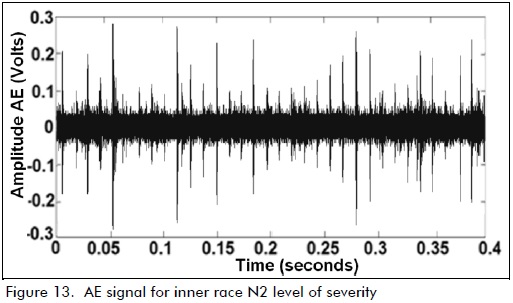
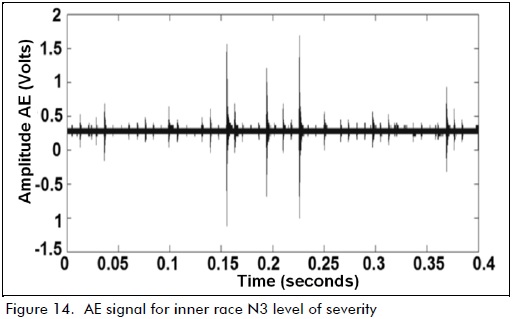
Results
The fault indicators obtained from the AE signal in this study (i.e. peak value, RMS, kurtosis and ring-down count) in each outer and inner race fault scenario are shown in Figures 15-18 and Tables 3-4.
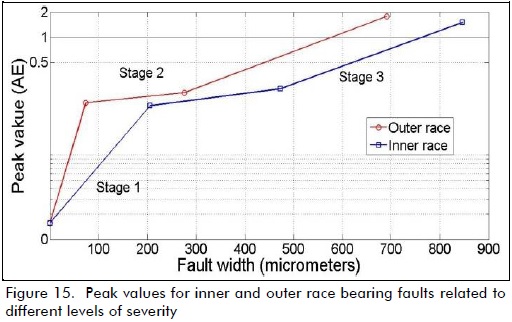
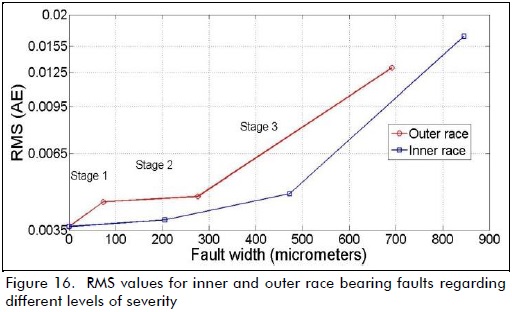
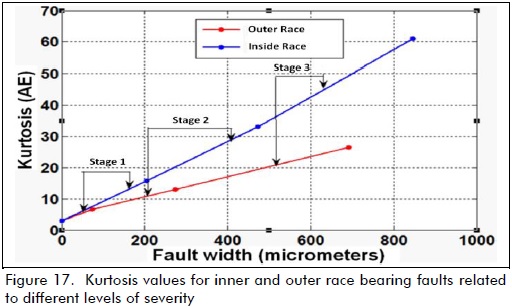
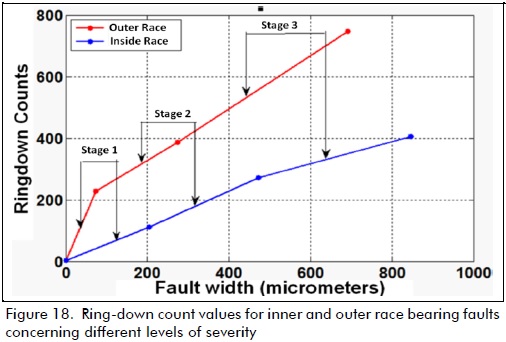
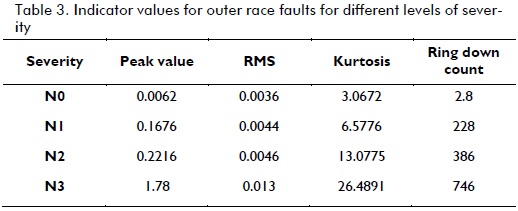
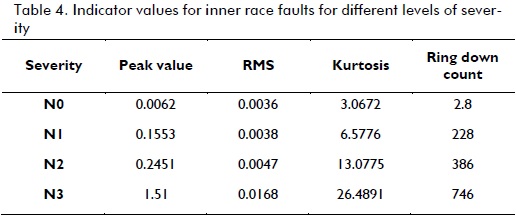
Figures 15-16 show AE signal peak value and RMS insensitivity for fault severity below 500µ; the indicator therefore produced undetectable changes compared to the baseline values in normal condition regarding the onset of a fault. However, peak value and RMS amplitude changed meaningfully regarding a severer fault condition. Figures 17-18 show a proportional increase in fault indicator amplitude with the growth of fault severity in outer and inner races. The criterion established for determining the ring-down count threshold in the experiments was based on the signal-to-noise ratio. Figure 17 shows a lineal increase in the kurtosis indicator regarding inner and outer race fault severity.
Conclusions
The experimental results led to concluding that AE signal time domain analysis provided information for distinguishing between a normal and a faulty bearing. The experiments showed that an increase in fault indicator magnitude (i.e. peak, RMS and ring-down count values) was produced by an increase in inner and outer race fault severity. AE signal amplitude regarding inner race defect was lower compared to that for outer race defect. This was attributed to variation in the transmission path from the AE source (inner race) to the AE sensor on the bearing casing (Saad Al-Dossary et al., 2009). Poor peak value and RMS performance was found regarding the detection of incipient faults because they had low sensitivity in the first stages of the fault being studied. Furthermore, kurtosis and ring-down count performed satisfactorily by increasing in magnitude in the presence of the fault condition, even during the onset of a fault. It was thus concluded that kurtosis and ring-down count could be suitable candidates for monitoring bearings when using AE signals.
Although experimentation showed the proposed indicator's potential for tracking faults in bearings, it was still not clear how inner and outer race faults and locating defects could be discriminated. AE sensor position plays a fundamental role in the appropriate capture of an AE signal; a monitoring scheme thus needs a prior study to ensure that a sensor is suitably positioned.
References
Abdullah, M., Al-Ghamd, D., Mba, A comparative experimental study on the use of acoustic emission and vibration analysis for bearing defect identification and estimation of defect size., Mechanical Systems and Signal Processing, Vol. 20, No. 7, Oct., 2006, pp. 1537-1571. [ Links ]
Bagnoli, S., Capitani, R., Citti, P., Comparison of Accelerometer and acoustic emission signals as diagnostic tools in assessing bearing damage., In: Proceedings of 2nd International Conference on Condition Monitoring, London, May, 1988, pp. 117-125. [ Links ]
Eftekharnejad, B., Carrasco, M. R. B., Charnley, D., Mba, The application of spectral kurtosis on Acoustic Emission and vibrations from a defective bearing., Mechanical Systems and Signal Processing, Vol. 25, No. 1, Jan., 2011, pp. 266-284. [ Links ]
McFadden, P. D., Smith, J. D., Acoustic emission transducer for the vibration monitoring of bearings at low speeds., Proc IMeche, Vol. 198, 1984, pp. 127-130. [ Links ]
Saad Al-Dossary, R. I., Raja Hamzah, D., Mba, Observations of changes in acoustic emission waveform for varying seeded defect sizes in a rolling element bearing., Applied Acoustics, Vol. 70, No. 1, Jan., 2009, pp. 58-81. [ Links ]
Tandon, N., Choudhury, A., Review of vibration and acoustic measurement methods for the detection of defects in rolling element bearings., Tribology International, Vol. 32, No. 8, 1999, pp. 469-480. [ Links ]
Tandon, N., Nakra, B. C., Vibration and acoustic monitoring techniques for the detection of defects in rolling element bearings a review., Shock Vibr Digest, Vol. 24, No. 3, 1992, pp. 3-11. [ Links ]
Yoshioka, T., Fujiwara, T., Application of acoustic emission technique to detection of rolling bearing failure., In: Dornfield, D. A., (ed.), Acoustic emission monitoring and analysis in manufacturing., New York, ASME, 1984, pp. 55-75. [ Links ]
Yoshioka, T., Fujiwara, T., A new acoustic emission source locating system for the study of rolling contact fatigue., Wear, Vol. 81, 1982, pp. 183-186. [ Links ]