Introduction
According to Sterlacchini (2008), higher education levels of a population are directly related to the regional economic development. Moreover, the academic activities of teaching and research have a positive influence on regional indicators of economic development (Drucker & Goldstein, 2007; Goldstein & Drucker, 2006). In the higher education context, Engineering education performs a key element as it is an activity that may foster innovation in the industry and in all other economic sectors (Borges & Almeida, 2013). In Brazil, there was an increase of 265% from 2001 to 2011 in the number of vacancies offered in Engineering degrees, which have a minimal duration of 5 years and 3,600 effective hours. Nevertheless, the country exhibits the lowest percentage of Engineering students in higher education when compared to other BRICS countries (Brazil, Russia, India, China and South Africa) (Oliveira, Almeida, Carvalho, & Pereira, 2013).
In recent years, there is a call for transformation in Engineering education not only in Brazil, but all over the world. Novel curricula would support learning through more integrated and experiential activities and Engineering students would develop competencies such as problem-solving, innovation, communication, teamwork, leadership, ethics, sustainability, among others. Besides, this transformation should be informed by educational research and supported by institutions and Engineering faculties (Aizpun, Sandino, & Merideno, 2015; Fink, Ambrose, & Wheeler, 2005; Passow & Passow, 2017). Still, not only the future engineers' competencies need transformation, but also Engineering educators' competencies as well. However, most research focuses on future engineers' competencies or novel teaching methodologies (Gómez et al., 2014; Ramírez-Echeverry, Olarte Dussán, & García-Carillo, 2016; Verano-Tacoronte, Bolívar-Cruz, & González-Betancor, 2015), whilst research on educators' competencies is still underdeveloped.
This research is inserted within this context as it aims to analyze the influence of Engineering professors' competencies on their performance, as perceived by Engineering students and graduates from a Brazilian federal public university. In order to accomplish this aim, a set of Engineering educators' competencies are proposed, identified, and validated by exploratory factor analysis (EFA), parallel analysis, internal consistency (Cronbach's alpha), and partial confirmatory factor analysis (PCFA). Afterwards, their influence on Engineering educators' performance is analyzed by means of logistic regression. This paper is structured as follows. This first section covers the introduction. The second section comprises the theoretical framework, which is mainly about educators' competencies. The third section presents the methodology employed, and the fourth section presents the results and the analyses carried out. Finally, the fifth section contains the final considerations of the paper.
Professors' Competencies
A wide range of studies show that aspects beyond knowledge, such as the satisfaction of students, who started being viewed as customers, may be important for establishing professors' success in the classroom (Grebennikov & Shah, 2013; Kunter et al., 2013; Sarrico & Rosa, 2014; Vuori, 2013). Skills and attitudes have started being demanded in the academic environment. The more diverse the students in a class, the bigger the competence demanded from professors and from the institutions' structure as a whole (Veiga, Leite, & Duarte, 2005). Being professionally competent implies retaining knowledge, skills, and attitudes for mastering specific situations. These elements may be acquired through a learning process, through experimentation, and especially through practice (Klieme et al., 2008; Tardif, 2012).
Knowledge is a result of man's constant search for learning. Skill employs previous knowledge to solve problems and promote innovation, and it is also known as "know-how". Attitude, in turn, combines knowledge and skill in order to make things happen (Mascarenhas, 2010). The two first elements are known as essential competencies, whereas the last is known as an individual one (Bitencourt, 2002). For many years, attitude was an individual competence that was disregarded, especially by organizations (Ruthes & Cunha, 2007). Nevertheless, the importance of professionals' attitude and behavior is emphasized nowadays, since they encompass individual competencies that may contribute to superior performance at work or in any other situation (Jaques, 1998).
According to Sánchez & Leicea (2007), the competent professor or professional possesses skills related to being, doing and knowing. With regard to "being", it is observed that a competent professional follows a behavior delimited by codes of conduct, values, and ethics related to the profession. The "knowing" is related to significant and updated knowledge around the area of professional action. Finally, the "doing" is closely related to the ability to plan a task, integrate and lead groups, think creatively and critically, act autonomously, learn through practice and master the technological tools of the area.
Rojo, Jiménez, & Flores (1999) concluded that the teaching competence, despite being hard to define and measure, would be closely related to a professor's professional aspects (mastering the subject, dedication, class preparation, assuming roles, teaching innovation), knowledge about students' needs and characteristics (motivation and deficiency in education), and teaching skills (simplicity, clear evaluation criteria, mastering of the argumentation process and communication skills with the students).
Menges & Austin (2001) draw attention to the fact that the teaching competence is also connected to a professor's ability to encourage students' active participation in the classroom. Onwuegbuzie et al. (2007) stress that students believe that competent professors are able to draw attention to the subject taught during classes. Heller, Beil, Dam, & Haerum (2010) show that Engineering students view engagement in terms of professors' enthusiasm, involvement, and interaction with students. Recent studies have stressed the importance of developing professors' emotional competence as a way of enhancing both professors and students' performance (Palomera, Fernández-Berrocal, & Brackett, 2008; Sutton & Wheatley, 2003).
Masetto (2012) contends three competencies as fundamentals for higher education teaching: deep understanding of a knowledge area; mastering the pedagogical area; and exercising the political dimension of higher education teaching, i.e. the professor is an actor who interacts with and transforms society, even when trying to be as neutral as possible. Similar competencies are shared by Engineering education research.
While discussing the novel role of Engineering educators, Fink et al., (2005) posit that expert teachers develop knowledge in three areas: content (disciplinary expertise), pedagogical (types of pedagogy) and pedagogical-content (demonstrate procedures and explain particular concepts within the content area). Furthermore, Engineering educators develop their competence in mainly three stages: first, by enhancing common teaching techniques; second, by understanding the science and principles of learning and teaching; finally, by exploring the humanistic dimension of education.
In a study involving Electrical Engineering postgraduate students about to enter the educational career, Cargnin-Stieler, Teixeira, & Assuncao (2014) in engineering education, and also technical and scientific competencies. There is a growing demand for engineering teachers and this raises concern with regards to the formation of these professionals in Brazil. This paper begins with the information collected by electronic mail sent to professors of all Electrical Engineering postgraduate programs in Brazil. The questions/suggestions requested included the advice that could be given to postgraduate students about to enter the teaching profession. This research, grounded in the studies of Braslavsk, Perrenoud, Masetto and Zabalza, among other studies, indicated a convergence towards three engineering professor competencies: in-depth knowledge about the subject to be taught (technical and scientificidentified three main Engineering professors' competencies: in-depth knowledge of the subject, knowing the teaching content (which is less in-depth), and knowing how to teach this content (pedagogical-didactic competence). Complementarily, Kaw (2005) lists seven techniques employed by highly effective Engineering educators, such as organization, respect for students, teaching tools, rapid feedback, among others.
There has been a discussion of what should be the competencies or ideal qualification profile of business and information systems Engineering (BISE) professors. Several researchers advocate a balance between theory and practice, whereas others contend research excellence as the primary competence (Loos, 2013). In a more practical research, Soler-González, Onate-Andino, Andrade-Merino, & Álvarez-Calderón (2016) developed a fuzzy model to assess financial Engineering professors competencies level, which included eight out of sixteen competencies from Ecuador's Council responsible for higher education. Interestingly, the competence of Aizpun et al. (2015) of stimulating students to participate in university-industry collaboration practical projects in order to develop creativity, innovation, problem-solving and leadership was reckoned by students as important, but it is still neglected by Engineering education literature. Nevertheless, a discussion considering students' views with regard to Engineering educators' competencies is still a gap in the literature, which is addressed in this research.
Methodology
This research is predominantly quantitative as it collected in 2014-15 data regarding Engineering professors' competencies and performance based on a sample of 139 students and graduates in Electronic and Computer Engineering from a federal public university in Brazil. Particularly, the survey focused on students enrolled in the last year of the Engineering course (the total duration is five years) and graduates that received their degree within a 2-year period. The data were collected through online structured questionnaires made available to students/ graduates by email. Regarding sample characteristics, the majority of participants (79%) were students. Besides, about 90% of participants were men, which is a percentage in accordance with the reality of these courses in the surveyed university.
The analyses were performed in the IBM SPSS software, which included exploratory factor analysis and binomial logistic regression. The initial questionnaire was derived from the literature and contained 35 questions that asked participants to provide an estimate of the percentage of their professors that presented certain competencies. The answer options for each question were: 0 (none of them), 25 (few of them), 50 (considerable portion), 75 (most of them) and 100 (all of them).
The main constructs related to professors' competencies were identified through exploratory factor analysis, specifically, principal components analysis, based on robust statistical literature (Field, 2009; Hair, Anderson, Babin, & Black, 2010). Criteria such as scree plot, Kaiser criterion, and parallel analysis were considered in order to determine the number of factors, i.e. the competencies dimensions in this research. First, the Kaiser criterion retains factors with eigenvalues higher than 1. Second, the scree plot suggests that the number of factors for extraction is indicated by the inflection point in the eigenvalues plot, specifically, the last point before the graph straightens to the horizontal axis. In other words, when the difference between the current and next components eigenvalues become marginal in comparison to previous differences. Finally, the parallel analysis calculates, for a random sample, the eigenvalues for the 95th percentile and only the factors with eigenvalues higher than those of the percentile are extracted (Hayton, Allen, & Scarpello, 2004). It is worth mentioning the application of the programming code developed by O'connor (2000) to calculate the eigenvalues of parallel analysis in SPSS.
In order to check the sampling adequacy, the following criteria were applied: minimum general KMO (measure of Kaiser-Meyer-Olkim) of 0,5; minimum individual KMO (by variable, that is, each item in the questionnaire) of 0,5; significant Bartlett's test of sphericity (p < 0,05); minimum commonality of 0,5; and loading of each variable in a single factor. Finally, the orthogonal Varimax rotation was employed with Kaiser normalization. Additionally, internal consistency (Cronbach's alpha) and partial confirmatory factor analysis (Gignac, 2009) were also performed in SPSS in order to confirm factors. Regarding internal consistency, the minimum critical value to Cronbach's alpha is usually 0,70 (Field, 2009; Hair et al., 2010). Regarding confirmatory factor analysis, an acceptable model fit is reached when fit indexes such as the normed-fit index (NFI), comparative fit index (CFI), and Tucker Lewis Index (TLI) are superior to 0,90. The root mean square error of approximation (RMSEA) is inferior to 0,08; and the standardized root mean square residual (SRMR) is inferior to 0,10. Similarly, good model fit is reached when NFI> 0,95; CFI > 0,95; TLI > 0,95; RMSEA < 0,05; and SRMR < 0,05 (Baki, 2017; Hair et al., 2010; Maroco, 2010; Shelby, 2011)
After identifying the main competencies dimensions present in the questionnaire through exploratory factor analysis, a logistic regression was carried out in order to verify which of these influence professors' performance. The logistic regression has a dependent variable that is dichotomical (either one category or other) and independent variables (predictors) that may be continuous, ordinal or even categorical. The logistic regression was preferred to multiple regression, since it overcomes statistical assumptions constraints such as residuals' normality and homoscedasticity (Field, 2009; Hair et al., 2010).
The dependent variable of the study, "percentage of professors who are role models", was dichotomized as follows: the values equal or higher than 50 were transformed into 1 and values lower than 50 into 0. Thus, the value 1 represents that most professors were considered role models by respondents, whereas the value 0 represents that the minority of professors were considered role models.
The independent variables (predictors) included the three competencies dimensions identified in the EFA, namely pedagogical-content knowledge, emotional skills and innovation-inspiring attitudes, along with a categorical dichotomous variable related to the interviewed situation - whether an Engineering student or graduate. The value 0 (zero) was assigned to Engineering students and the value 1 (one) was assigned to Engineering graduates for the variable "graduate".
Results and Analysis
Exploratory Factor Analysis
Facing the expressive number of variables observed in the research questionnaire of this study, the first technique applied was the Exploratory Factor Analysis (EFA) in order to reduce and simplify its structure. Through this technique, it was also possible to identify the lowest possible number of hypothetical variables (factors) that explain the highest possible percentage of covariance between the variables. The number of hypothetical variables of the study was supported by 3 outputs inherent to the analysis in question: the scree plot, the parallel analysis, and the Kaiser criterion. On the one hand, the first output (scree plot) revealed the existence of a strong inflection point on the third factor as the difference between the current eigenvalue (1,99) and the next (1,63) became marginal in comparison to previous differences (11,42; 3,71; 1,99). This recommended the extraction of only 3 factors, which was confirmed by the parallel analysis. On the other hand, the Kaiser criterion, exhibited in Table 1, recommended the extraction of 7 factors. Due to the convergence of the criteria and considering the strength of the parallel analysis method, the option of extracting 3 hypothetical variables was initially selected.
Afterwards, the adequacy measures were analyzed. Both the general and individual KMOs criteria were met, as well as Bartlett's test of sphericity. However, the minimum value of commonality of 0,5 was not met by several variables. Therefore, the variable with the lowest commonality was excluded and the exploratory factor analysis was restarted. Eighteen rounds were carried out until all criteria were met. The number of factors for extraction was also reassessed in each stage.
Table 2 presents the results of the parallel analysis and Kaiser criterion for the last round of the EFA. The scree plot of the last round of the exploratory factor analysis suggests retaining 4 factors. The parallel analysis pointed to retaining 2 factors, whereas the Kaiser criterion indicated 3 factors. Due chiefly to the proximity of the third factor eigenvalue (1,47) to the limit of the 95th percentile (1,52), in addition to meeting the Kaiser criterion, the option of retaining 3 factors in the exploratory factor analysis was selected. The total variance explained by the retention of 3 factors was 64,2%, the general KMO was 0,891 and Bartlett's test of sphericity was significant to the level of 0,001.
Table 3 presents the results of the commonalities and factor loadings of the variables after the Varimax rotation with Kaiser normalization. Values under 0,4 were omitted from the table. It is possible to observe that each variable loaded on only one factor, in accordance with the criterion presented in the methodology section.
Considering the initial 35 items in the questionnaire, in the beginning of the EFA, five items did not load on a single factor, thirteen items loaded on the first factor, six on the second one, and eleven on the third one. In the end of the EFA (Table 3), ten items loaded on the first factor, four on the second, and other four items loaded on the third factor.
Finally, in order to validate the factors obtained, internal consistency (Cronbach's alpha) and partial confirmatory factor analysis were carried out. The 3 factors identified presented Cronbach's alpha values superior to the standard limit of 0,7 (Field, 2009; Hair et al., 2010), thus corroborating their internal consistency. As shown in Table 4, the results of the partial confirmatory factor analysis (NFI = 0,90; CFI = 0,96; TLI = 0,94; RMSEA = 0,06; and SRMR = 0,04) indicate acceptable to good fit indexes (Baki, 2017; Hair et al., 2010; Maroco, 2010; Shelby, 2011), thus corroborating the identified factors.
Each factor was then interpreted and named according to the nature of the included questions and to the theoretical basis related to professors' competencies Thus, the first factor is related to professor's content-pedagogical knowledge, since it includes questions related to professors' competencies of lecturing clearly to facilitate students learning, of employing practical activities, of connecting theory with practice, of using real companies examples and practical activities, besides inspiring environmental and social responsibility in their students. Contributing further to other studies that separated knowledge in content, pedagogical and even the combination "content-pedagogical" (Cargnin-Stieler et al., 2014; Fink et al., 2005; Masetto, 2012), this result suggests that students do not perceive content and pedagogy separately, but the combination of them as a single knowledge.
The second factor is professors' innovation-inspiring attitude, wherein professors motivate students to participate in innovation activities such as the junior business, the pre-incubator, and the technological incubator, as well as to the research field by publishing articles. This result is somehow revealing that not only Engineering students need to develop innovation related competencies (Aizpun et al., 2015; Fink et al., 2005; Passow & Passow, 2017), but Engineering educators need to develop the attitudes that inspire students to innovate as well. Similarly, research excellence is considered crucial by Engineering educators (Loos, 2013), but motivating students to engage in research is also an innovation-inspiring attitude perceived by students.
Finally, the third factor is professors' emotional skill, as it includes questions related to respecting students, keeping calm in conflict situations, willingness to answer students' questions and clarifying doubts concerning assessments. This result corroborates literature that also considered such aspects to some extent (Kaw, 2005; Palomera et al., 2008; Sutton & Wheatley, 2003). Binomial Logistic Regression
After identifying the main variables through the exploratory factor analysis (pedagogical-content knowledge, emotional skills, and innovation-inspiring attitudes), the logistic regression was carried out in order to identify which variables influence students' perception of professors' competence. The independent (predictor) variables included the 3 identified in the Exploratory Factor Analysis and a categorical variable related to the student's situation - whether they had already graduated or not.
Eight models were tested in order to verify the influence of the variables. Table 5 presents detailed results for each variable, such as significance (p-value) and odds-ratio (Exp B), whilst Table 6 presents the models general assessment. The first model is the simplest and it contains only the constant as predictor. It is employed as reference for comparison to the next models, which are expected to have better explanatory power than the basic model. Finally, it is stressed that the basic model has a global percentage of accuracy of 56%.
Table 5 Results of the Binomial Logistic Regression
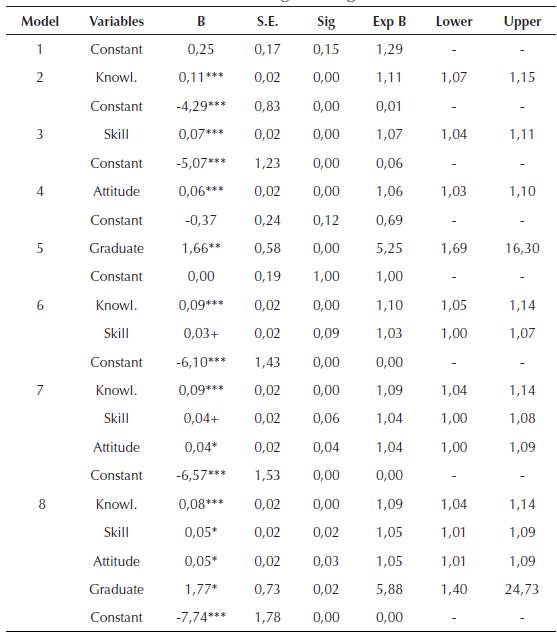
Note: + p < 0,10; * p < 0,05; ** p < 0,01; *** p < 0,001
Source: Authors (2015).
The models from 2 to 5 comprised only one variable and the constant. Model 2 contained the variable knowledge and the constant, which were both significant to the level of 0,001. Moreover, the model correctly classified 76% of the cases and presented a high value of explained variance: 45% by the Nagelkerke R2. Model 3 contained the variable skill and the constant, which were also significant to the level of 0,001. However, the model correctly classified 69% of the cases and presented 26% of variance explained by Nagelkerke R2. Both values were inferior to model 2 (knowledge and constant as predictors), but superior to model 1 (only the constant as predictor).
Model 4 contained the variable attitude, significant to the level of 0,001, and the constant, which was not significant. This model correctly predicted 66% of the cases and presented 16% of explained variance (Nagelkerke R2). Thus, this model was superior to model 1, but inferior to models 2 and 3. Model 5 contained the variable graduate, significant to the level of 0,01, and the constant, which was not significant. This model presented 10% of explained variance (Nagelkerke R2), but it correctly predicted only 56% of the cases, like model 1.
Based on the results of models 2 and 4, models 6, 7 and 8 were developed. Each one adds one variable consecutively. Considering the explanation power of the models with only one variable, the variable knowledge was selected to be the first one added, since it had the highest explained variance (45%), followed by the variables skill (26%), attitude (16%) and graduated (10%).
Thus, model 6 contained the variables knowledge and skill and the constant. The variable knowledge and the constant were significant to the level of 0,001, whereas the variable skill was partially significant (p < 0,10). That points to a larger contribution of the variable knowledge in relation to the variable skill for the prediction of the model. Finally, the explained variance of the model was 47% and the accuracy was 75%, which are close to those of model 2. Model 7 contained 3 variables (knowledge, skill, and attitude) and the constant. The variable knowledge and the constant were significant to the level of 0,001, the variable attitude to the level of 0,05 and the variable skill partially to the level of 0,10. The model explained variance was 50% and the accuracy was 76%, which are values superior to those of model 6. Finally, model 8 contained all 4 variables (knowledge, skill, attitude and graduated) and the constant. The variable knowledge and the constant were significant to the level of 0,001, whereas the other variables were significant to the level of 0,05. Including the variable graduate had a positive influence on the variable skill, which went from a partial significance level of 0,10 to 0,05. Additionally, the last model presented the highest percentage of explained variance (54%) and the highest accuracy (79%).
Essentially, the last model indicated that all variables are important to explain students' perception of their professors' competencies. Specifically, an increase of one percentage point in the content-pedagogical knowledge increases the competence of professors in 9%. Similarly, both emotional skills and innovation-inspiring attitudes account for a 5% increase. Therefore, these results contribute to the literature about Engineering educators competencies and performance (Cargnin-Stieler et al., 2014; Fink et al., 2005; Kaw, 2005; Klieme et al., 2008; Mascarenhas, 2010; Masetto, 2012; Sánchez & Leicea, 2007; Tardif, 2012; Veiga et al., 2005) by highlighting the impact of somehow singular competencies (especially innovation-inspiring attitude) on performance.
Another result worthy of notice was the statistical significance of the variable graduate, which indicated that graduates are almost six times more likely to consider professors as role models than undergraduate students. This result suggests that after leaving college, entering the job market and starting to experience real situations, graduates have a different understanding of the competencies developed by their professors during college education. In this sense, further research could delve into these differences, for instance, by applying structural equation modeling confirmatory factor analysis considering such segmentation (Shelby, 2011).
Conclusions
This study aimed to analyze the influence of Engineering professors' competencies on their performance, as perceived by Engineering students and graduates from a Brazilian federal public university. As a theoretical contribution, a set of Engineering educators' competencies were proposed, identified, and validated by exploratory factor analysis (EFA), internal consistency (Cronbach's alpha) and by partial confirmatory factor analysis (PCFA). Regarding results, first, content-pedagogical knowledge is perceived by students as a single merged competence. Second, not only Engineering students need to develop innovation competencies, but Engineering educators also have to develop innovation-inspiring attitudes to motivate students' innovativeness. Third, emotional skills play an important role as well. Finally, the impact of these competencies on Engineering educators' performance was confirmed and analyzed by means of logistic regression.
As a practical implication, identifying the elements that influence professors' performance may contribute to improvements in Engineering education.
Concerning the methodology, the sample size was considered adequate for the statistical analysis applied (exploratory factor analysis and logistic regression). Retaining three factors could be seen as a methodology limitation, even though several methods were considered conjointly to decide this number of factors (parallel analysis, Kaiser criterion and scree plot). Other limitations may include the definition of the factors, which are bounded by authors' conjointly interpretation of statistic results and the literature, as well as the professors' performance measure, which is related to students/graduates' perceptions of professors as role models. Even though these results cannot be generalized for all fields in all universities, a theoretical consequence was verifying the importance of the professor's knowledge, skills, and attitudes in the perception of competence by the students and graduates, which was still an approach neglected by Engineering education studies. Among the suggestions for future research, the replication of this study in other fields, universities, or countries can be made, in addition to the identification of novel factors that may influence the competencies and performance of Engineering professors.