Introduction
Today, pollution and climate change are recognized realities all over the world. The introduction of alternative vehicle technologies such as electric vehicles (EVs) is an efficient effort to reduce carbon and nitrogen oxides emissions (Akbari et al., 2018). Moreover, electric vehicles are regarded as sustainable transport solutions, unlike those with conventional combustion engines (Usman et al., 2020). Electric vehicles are therefore considered to be a clean, affordable means of transportation that will most likely replace conventional petrol/diesel vehicles (H. Wang et al., 2019).
The advantages of electric vehicles, which include reductions in greenhouse gases and other emissions, energy security, and fuel savings (Faridimehr et al., 2019), have led governments to pro-mote EVs as a key measure to try to reduce greenhouse gas emissions (K. Huang et al., 2016). In 2017, the Chilean Energy Ministry set the target that 40% private vehicles should be electric by 2050 (Ministerio de Energía, 2017).
The demand for EVs has grown rapidly since 2010, influenced by renewable energy and socio-economic factors (X. Li et al., 2017), but also by government support, changes in the industry and private consumer demand (Cao et al., 2019), the price of EVs, their range, and the charging process and its associated infrastructure (Domínguez-Navarro et al., 2019).
Nevertheless, the social benefits of adopting EVs on a large scale cannot be achieved without the large-scale deployment of public charging stations (Faridimehr et al., 2019), since the existing network is insufficient. In other words, the small number of existing stations and their poorly chosen locations constitute a significant barrier for the propagation of EVs in many countries (Csiszár et al., 2019). Thus, the first step towards a wider adoption of electric vehicles is to establish the necessary charging infrastructure (H. Wang et al., 2019). However, this is not the only consideration, since the extensive penetration of EVs will threaten the stability of the electrical grid (Abdulaal et al., 2017). The generalized introduction of EVs and the deployment of renewable distributed generation on a large scale pose a major challenge to modern distribution systems (Ehsan and Yang, 2020). Furthermore, EVs introduce new charging demands that change the traditional patterns, while renewable energy sources (RES) are characterized by their highly variable generation patterns (de Quevedo et al., 2019).
All these factors imply that anticipating the use of future rapid charging stations, as well as the energy that they will demand, is critical information for organizations involved in planning the deployment of charging infrastructure for EVs (Bryden et al., 2018). At the same time, it is hard to decide on locations for charging stations due to the uncertainty of candidate sites and unidentified charging demands, which are subject to many variables (Ahn and Yeo, 2015).
It is therefore of great importance to obtain up-to-date information on charging demands in terms of both power and infra-structure, in order to support planning for the incorporation of EVs by governments, the private sector, and any other types of organization. The existing literature contains a number of scientific articles on this issue, but there is a lack of publications that systematize important knowledge of the mathematical models used to support decisions on charging requirements, which are also cross-linked with other important elements like vehicle types, study objects, etc. This research offers some answers to the questions raised above, together with a systematized presentation of the information currently available in articles and other documents.
Our methodology is one used mainly in the medical field, which has however been adapted to other areas: a systematic mapping study (Kitchenham et al., 2004).
The document is organized in sections as follows: Related works; Methodology; Results and discussion; Conclusions; and finally References.
Related works
The literature reports no works containing systematic mapping applied to the use of demand models associated with electric vehicles. Thanks to the versatility of the tool, however, and the broad scope of the field of EVs, some related works were found.
Systematic mapping study
The aim of a systematic mapping study is to organize and synthesize scattered information on a subject and present the relationship between two study variables in such a way that it can be quickly and easily understood. This tool can therefore be applied in a great variety of disciplines. One example from the healthcare area is a study by Saleemi et al. (2020) which uses systematic mapping to thoroughly analyze the available evidence on ubiquitous medical attention, in order to understand and evaluate the progress made in the field and identify the challenges that hinder further progress. Another example is a study by Drissi et al. (2020) which aims to provide a general description and structured comprehension of the literature on connected mental health (CMH). In a similar context, the research by Behmanesh et al. (2020) reports on the identification and classification of tele-orthopedic applications and services, together with a general description of trends in this field. Strictly in the field of technology and information, the following studies use systematic mapping: Khan et al. (2021), who try to identify, categorize, and present a complete general description of the approaches, techniques, and tools used in election predictions on Twitter; Rachad and Idri (2020), who aim to provide an overview of the use of machine learning (ML) techniques in the design and development of mobile applications; Jafari and Rasoolzadegan (2020), who explore security patterns as a means of encapsulating and communicating tested security solutions and introduce security into the development process. Moreover, Belmonte et al. (2019) present an overview of automatic tasks based on the concept of unmanned aerial vehicles (UAV); Zakari et al. (2019) present a study of software fault localization (SFL) in order to determine the general productivity of investigation, demography, and trends, as well as to classify existing techniques; Cravero et al. (2018) offer an overview of how IoT (Internet of Things) technologies and BigData can help oenologists to manage and optimize wine production, and how the components of the computer architectures used can be determined; Haghighatkhah et al. (2017) propose a classification and analysis of the literature related with software engineering in the motor car industry; and Gabriel et al. (2016) analyze the existing digital tools to support creativity in organizations. Other disciplines report the use of systematic mapping studies, such as geoconservation (Németh et al., 2021), legal ontologies (Rodrigues et al., 2019), musical intervention carried out by nurses as part of research (Cigerci et al., 2019), socio-technical congruence (Sierra et al., 2018), smart tourism (Celdrán-Bernabéu et al., 2018) , and gamification in education (Dicheva et al., 2015), to name but a few.
When conducting a systematic mapping study, the relationship between two study variables is presented by a graph with circles, where circle size is proportional to the simultaneous occurrence frequency of both variables. Presenting the data in a graph makes an easy way to know and visually evaluate these relationships. Furthermore, conducting a systematic mapping study involves the creation of starting research questions that can be answered by the information obtained directly from observing the graph.
Reviews
With regard to the literature reviews about EVs, there are studies on: electric vehicle routing problems and their variants and algorithms (Qin et al., 2021); EV converters highlighting topology, features, components, operation, strengths, and weaknesses (Lipu et al., 2021); energy storage systems and balancing circuits (Habib et al., 2020); charging technologies and methods (Brenna et al., 2020); charge-discharge coordination between EVs and the power grid (Solanke et al., 2020); prospects of EVs in developing countries (Rajper and Albrecht, 2020); the performance of battery management and control systems for lithium ion batteries (Dileepan and Jayakumar, 2020); the technical development of EVs and emerging technologies (X. Sun et al., 2020); the integration of solar photovoltaic energy in various types of electric and hybrid vehicles (Waseem et al., 2019) ; the characterization of pure EVs, their energy sources, environmental impacts, energy management strategies, and the challenges they face (Z. Li et al., 2019); forms of charge planning, applying dynamic pricing (Limmer, 2019); analysis of the experiences of plug-in EV users (Meisel and Merfeld, 2018); the operating process of different types of EVs, batteries, and supercapacitor technologies (Ding et al., 2017); and theories, network modeling, solution algorithms, and applications (Jing et al., 2016), etc.
Methodology
This section presents some general conceptual elements, followed by the activities carried out in the systematic mapping study.
Use of mathematical models
The use of mathematical models is fundamental for generating information for decision-making in the context of EV incorporation planning. Specifically, these models are useful for designing charging stations (Mehrjerdi and Hemmati, 2019), determining the number of charging stations (Kim and Kim, 2021), determining the charging time and/or the amount of charge involved (Cheon and Kang, 2017), and determining the flow that charging requires from transmission and distribution systems (Bhat et al., 2017), as well as for the robust operation of distribution networks (Wei et al., 2017), etc.
Depending on the purpose for which the model is used, it may include spatial and location elements (de Quevedo et al., 2019); uncertainty in demand, consumption, or any other variable (Hafez and Bhattacharya, 2018b); the number of charging stations and population densities (X. Li et al., 2017); space-time parameters (Arias et al., 2017); the spatial distribution of traffic flows (Xiang et al., 2016); economic parameters such as operating costs, investment, connection costs, the total cost of losses, etc. (Liao and Lu, 2015; Simorgh et al., 2018); and the state of a vehicle, the state of battery charge (SOC), and distance to the destination (Kim and Kim, 2021 ), to name only a few.
Activities carried out in the systematic mapping
Research Questions. The following research questions (RQ) were defined in accordance with the proposed aim of this study and the recommendations made by Kitchenham and Charters (2007).
RQ-1: What is the use given to demand projection in the study (consumable, focus)? RQ-2: What are the requirements to enable the demand to be constructed (physical elements, electrical elements, mixed)? RQ-3: What type of vehicle use is considered in studying the demand (public, private, mixed)? RQ-4: What is the context of the research (applied or theoretical)? RQ-5: How is the type of (mathematical) model used in the demand projection (or study) defined?
Data sources and search. To answer the research questions, data were collected from the Web of Science (WoS) and Scopus digital databases, which are available online. The search for articles in these databases used Boolean expressions such as "Or" and "And", with the following key words (in English): "Electric car", "Electric vehicle", "Demand", "Charging station" (as well as their plurals, as appropriate), which were defined from the research object. The advanced search option was used in order to obtain articles which contained the search terms in the title and/ or keywords. The search was limited to the period between 2015 and May 2021. The result was a total of 1 245 articles, 472 in WoS and 773 in Scopus.
Inclusion and exclusion criteria. A preliminary selection was made of the 1 245 articles found, which was based on the following set of criteria:
First, all articles were included which were written in Spanish or English and came from journals, conferences, and congresses in which mathematical demand models were developed, describing physical and/or electrical requirements associated with the different types of electric cars.
Articles with fewer than three citations (Agostino et al., 2020) and referring to electric vehicles other than cars or buses (e.g. drones or electric bicycles) were excluded. Articles which did not present a mathematical demand model were excluded, as well as those that contained only mean data or took probability distributions to obtain the median value and its variance, which were used as final data in order to reflect demand without using an explicit mathematical model. These criteria were applied to the abstracts of all articles. If the abstract did not provide sufficient information to make a decision, the conclusions and general content of the document were reviewed in greater detail. After applying the inclusion and exclusion criteria to the 1 245 articles, only 50 articles remained, in which the particular demand models were studied, and answers to the research questions were sought.
Classification elements. Once the relevant publications had been selected, a set of classifications was defined based on the study object, namely (i) the use of the demand, or the identification of the purpose for which the demand model was constructed and used. The following potential uses were included: a) consumable, where information on the demand was used as information for a subsequent purpose within the study; and b) focus, where the construction of the demand model was the final object of the study. (ii) Requirements of the demand: The elements for which the demand was developed were classified into: a) physical elements associated with the number of stations, charging points, infrastructure conditions, etc.; b) electrical elements, considering the amount of energy, its power, voltage, supply characteristics, etc.; and c) mixed, i.e., whether the model allowed information to be derived from both the physical and electrical elements. (iii) Type of vehicle: This element classified the type of vehicle included in the study, namely
a) public, when the vehicle was available for use by the general public, including taxis, buses, van services, etc.;
b) private, when the vehicle was available for private use, including private vehicles and Uber, limousines, and similar services; c) mixed, for both public and private use; and d) unreported, when the article provided no information for classification. (iv) Research area: The purpose of this definition was to establish the context in which the demand model was applied. The options for this classification were: a) application, b) theoretical, or c) unreported.
Systematic mapping. Based on the above classifications and the study of the articles, the relationship between different elements was graphically depicted on the XY plane. The size of each circle in the graph is proportional to the frequency of the occurrence in conjunction of the X and Y variables defined on the axes. Thus, Figure 1 shows the interaction between the research context (Y axis) and the use of the demand and is requirements (X axis).
Figure 1 shows that the highest frequencies are associated with: (i) the theoretical content, when the demand information is used as a consumable, and (ii) with the theoretical content, when the demand requirements are electrical.
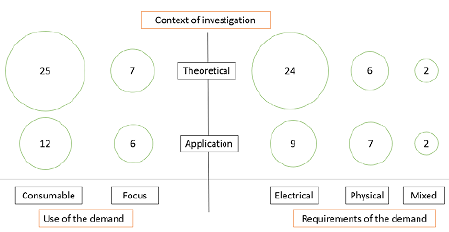
Source: Authors
Figure 1 Systematic mapping according to the research context, the use of the demand, and the demand requirements
Figure 2 shows the link between the use of the demand (Y axis) and the type of vehicle and the demand requirements (X axis).
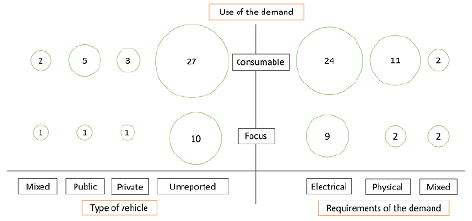
Source: Authors
Figure 2 Systematic mapping according to the use of the demand, the type of vehicle, and the demand requirements
Figure 2 clearly shows that the combinations occurring with the greatest frequency are (i) a consumable use of the demand with a lack of information on the type of vehicle, and (ii) a consumable use of the demand identified for electrical demand requirements.
Results and discussion
The answers to the research questions obtained by analyzing the articles are given below:
For RQ-1, it was found that 74% of the reviewed articles used the demand model as a consumable for the construction of further information. For RQ-2, the main requirements for the construction of the demand were associated with electrical elements, (66%). However, it should be noted that 8% of the articles used a model that provided data on both electrical and physical requirements. The type of use of the vehicle in the demand study, i.e., the subject of RQ-3, was not clearly identified in most cases (74%). Some articles explicitly mentioned cars in shared use, fleets of taxis, and applications for buses. The results for RQ-4, i.e., the research area, show a clear tendency towards the theoretical field (64%). However, when the research area is compared to the different demand requirements, it is found that, for articles addressing physical demand requirements, the application field (7 cases) predominates over the theoretical one (6 cases). Finally, as for the results for RQ-5 (i.e., the type of (mathematical) model used for demand projection) were very diverse. Table 1 is a summarized list of authors, articles, and the various models applied.
This Table shows the remarkable and wide variety of methodologies designed to establish the demands associated with electric vehicles. Mathematical modeling and operations research tools play a leading role. An interesting aspect is the use of queueing theory as a mathematical theory of the stochastic service system (L. Li et al., 2016), which refers to queue analysis when a client comes to a service station and has to queue before being served (Hamdan et al., 2017). The tool is applied to provide an answer regarding the efficient number of parameters or charging points needed to supply energy to EVs. Different stochastic systems may be used to calculate this number, such as the one that treats the arrival of the vehicles as a non-homogeneous Poisson process while modeling the serving time based on the detailed characteristics of the battery (Hafez and Bhattacharya, 2018b). Other cases are defined with a compound M/G/oo queueing model (Ou et al., 2015). In parallel, and in the same field of operations research, Markov models are also used in several cases, e.g., to determine the forecast power demand for charging EVs at fast charging stations located in urban areas (Arias et al., 2017), or to estimate the energy states among a group of charging stations (Chen et al., 2018).
An interesting finding outside the parameters indicated for this study emerged while reviewing the abstracts: the object of this investigation was to study the demand for EVs, particularly physical or electrical requirements; however, many publications do not mention these elements, but refer to the concept of the response to demand and charging patterns. These are signs of progress being made in the most recent needs regarding EVs, where it is important to have data and models with which to describe how vehicle users follow their charging programs, both as a function of the time they devote to charging and the time of day in which they do so. It is also relevant to study how users' charging behavior influences supply systems and the power grid. Some examples of this situation are found in the works by Kamruzzaman and Benidris (2020), A. A. S. Mohamed et al. (2020), P. Wang et al. (2020)), Yan et al. (2020), M. Zhang et al. (2020), and Zhou et al. (2020).
Conclusions
The results of the systematic mapping study show a wide variety of demand models for electric vehicles., among which mathematical programming and operations research tools such as Markov chains and queueing models are the most frequently used.
Demand models are frequently used as a research consumable in the reviewed articles to establish electrical over physical ones. Most articles did not provide information on the type of vehicle, suggesting that this was not relevant for their research purposes. Finally, most of the reviewed articles were associated with the theoretical field rather than the practical one.