1. Introduction
In tropical and mountainous terrains, disasters are commonly caused by landslides triggered by rainfall1,2. Approximately 5% of the total population lives in areas susceptible to landslides3, and about 90% of deaths from landslides have been the result of rainfall-triggering events4, with an annual average of 914 fatalities5.
For the next decades, several authors have alerted about this issue because this global scenario will be more critical as a result of the increase in frequency and intensity of extreme climate events associated with global warming6,7, and the augmented exposure of population and infrastructure to extreme climate events7,8. Recent studies point out the increase in frequency and magnitude of rainfall events because of global warming, due to the rise in temperature and consequently enhanced atmosphere capacity to conserve moisture9. Because of this variation in the frequency and intensity of precipitation, a more frequent occurrence of landslides and changes in their spatial distribution is expected10,11. Although the increment in frequency and intensity of extreme rainstorm events has been observed in many regions around the world9, the Intergovernmental Panel on Climate Change (IPCC) reports have not provided a specific evaluation for landslides. It has only been pointed out, with a high degree of confidence, that changes in heavy rainfall could affect the occurrence of landslides in several regions12.
However, although there is a solid theoretical base that supports the upsurge in the occurrence of landslides as a result of climate change (CC), the specific effect on landslide occurrence is a complex issue due to the high levels of uncertainty in the CC global prediction and regional scenarios10,11,13. In addition, although extreme precipitation events are considered to be increasing because of CC, changes in the amount of precipitation and their local effects are not defined with precision in CC scenarios.
In fact, few studies are found in the literature about the effects of rainfall changes and CC on landslide occurrence10,14,15. The effects of CC on the assessment of landslide susceptibility have been studied at the regional level16-18, including risk conditions19-21, and regional early warning systems22,23. Some of these studies concluded that the rise in mean air temperature and changes in annual precipitation, at the regional and seasonal levels, are the most relevant climatic variations that can affect the occurrence of landslides15. Other authors examined the mechanisms by which CC can affect landslides occurrence, concluding that impacts of CC are considerably less compared to the impacts of anthropic intervention on the territory10. Finally, others studied different approaches to assess the impact of CC on the occurrence of landslides, where they identified 14 different approaches and group them into three broad categories, including (i) long-term monitoring, (ii) retrospective approaches and (iii) forward-looking approaches24. From these approaches, these authors concluded that approximations directly using rainfall as a variable show the highest levels of uncertainty, in contrast to the approach that applies air temperature and annual or seasonal rainfall, because these variables reflect lower levels of uncertainty.
However, even if there is an increase in rainfall intensity, it is actually soil permeability that initially controls soil infiltration, and rainfall excesses result in surface runoff10. For this reason, it is necessary to evaluate the effect of rainfall on landslide occurrence considering the spatial variability of hydraulic and mechanical parameters, as well as slope geometry. As a result, in the last years, some studies have used physically-based models to assess the effect of rainfall on landslide occurrence14,25,26. Physically-based models couple hydrological and geotechnical models to assess variations in hillslope stability conditions as a function of pore pressure and shear strength. As input variables, those authors consider rainfall scenarios generated by global and regional climate models27 or statistical projections of rainfall series. Those physical models are generally applied locally28,31, or in large areas that present homogeneous conditions in the occurrence of landslides23,31-33.
Different physically-based models allow the analysis of the rainfall effect on the stability of infinite slopes. Some of them are based on a saturated stationary flow parallel to the slope, emphasizing the effect of topography (e.g., LISA (Level I Stability Analysis34), SHALSTAB35, and SINMAP (Stability Index MAPping36); while others, such as TRIGRS37 consider partially saturated conditions and transient flows. From these models, SHALSTAB assumes a stationary flow that considers the effect on the cells of the subsurface flow generated in an upstream contributing area. This type of consideration applies to stationary conditions representing prolonged high rainfall intensities. The TRIGRS model, on the other hand, considers a transient flow that can be used to analyse different rainfall intensities and durations. The magnitude of the infiltration is controlled by the initial partially saturated conditions and the hydraulic conductivity of the soil, which allows describing more real conditions of the initial state of soils. Both models are suitable for interpreting the processes of rain infiltration into residual soils, which are characteristic of tropical mountain areas.
This study evaluates the effects of rainfall on landslide hazard mapping under a CC scenario in the north-western Colombian Andes. Two well-known physical-based models were implemented considering steady or transient hydrological conditions: SHALSTAB35 and TRIGRS (Transient Rainfall Infiltration and Grid-Based Regional Slope-Stability Model37, respectively. As input variables for the CC scenarios expected, rainfall changes were obtained from intensity-duration-frequency (IDF) curves, which estimated variations in precipitation intensity and duration associated with different return periods.
2. The problem of landslides in the Colombian Andes
Colombia, located in the north corner of South America, is a tropical country of mountainous topography with a strong hydroclimatic variability, under a complex geology and active tectonic setting. In addition to these complex natural conditions, population growth and increasing urbanization along landslide-prone areas have augmented risk conditions over the past few decades38. The urban population of developing countries grew by 5 times in 40 years and continues to expand rapidly39. By 2018, Colombia's projected population was 48 million, with 84% of the urban population40.
Those complex natural conditions and rapid urban growth show Colombia as one of the world’s most affected countries by landslides41. The World Bank presented a general report on the causes and losses of disasters in Colombia during the period 1970-2011. According to this study, the highest percentages of economic losses and destroyed houses corresponded to landslides and flooding41. For Colombia, a total of 32,022 landslides in the 116-year period between 1900 and 2016 were compiled42. The results showed that landslides are concentrated in the mountainous and most populated areas of Colombia. The 93% of landslides registered are concentrated in 33% of the country, the Andean region, where about 80% of Colombia's population is located in an area of 282,540 km2 and the most important and populated cities of Colombia, Bogotá, and Medellín43. In this study, the precipitation shows a dominant influence on the occurrence of landslides, with 92% of landslides triggered by rainfall, showing an annual bimodal pattern in the Andean region for both rainfall and landslide occurrence42.
One of the areas most affected by landslides in the Colombian Andes is the Aburrá Valley, located in the Central Cordillera of the northern Andes, with an area of 1,326 km2. Its climatic conditions are typical of humid tropical environments, with an average temperature of 22°C and relative humidity of 70%. The average multiannual rainfall varies from 1,400 mm in the central part and 2,700 mm in the north and south of the valley according to CHIRPS satellite data from 1981 to 2019 shown in Figure 1.
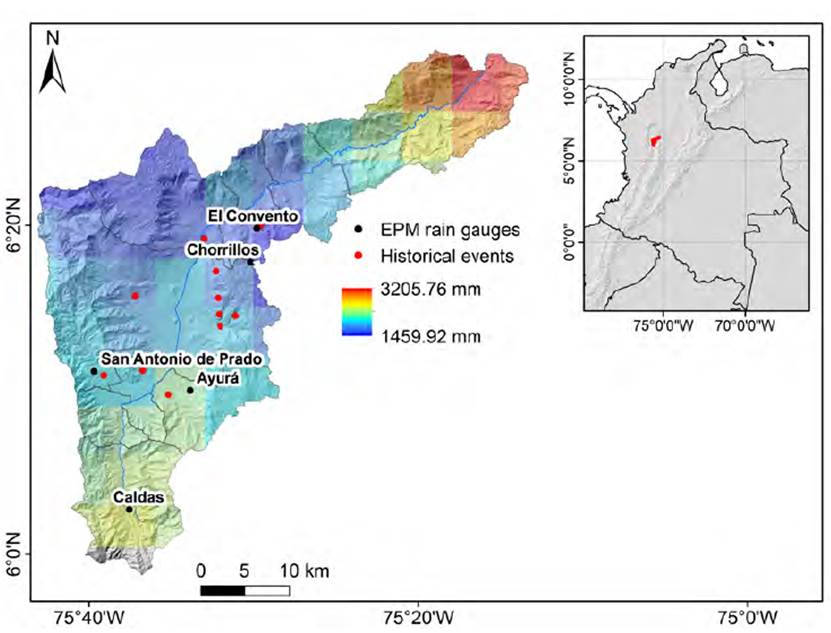
Figure 1 Spatial and temporal rainfall pattern in the Aburrá Valley; the histogram shows the multiannual monthly average, and raster shows multiannual average from satellite data: CHIRPS 1981-2019; black dots represent the location of the EPM rain gauges used and red dots historical landslides from Table 1
In the case of the Aburrá valley, landslides correspond to 3 out of 10 disasters occurring in the region, representing 75% of disaster victims. For the period 1880-2007, landslides have left 1,390 people dead and caused economic losses of around $21,000,000,000 COP44. Some of the most catastrophic landslides are: Rosellón (1927), Media Luna (1954), Santo Domingo Savio (1974), Villatina (1987), La Cruz (2007), El Socorro (2008), El Poblado (2008), and La Gabriela (2010); more information is presented in Table 1, and their location is shown in Figure 1.
Currently, the Aburrá Valley has an estimated population of 3.9 million inhabitants, where 95% is an urban population located in only 26% of the territory (340 km2 of urban area). The most populated municipalities, Medellin (2.5 million), Bello (482 K), and Itagüí (277 K), concentrate much of their population on the valley slopes40. This population growth has been extremely rapid; in a century, the population of the valley has grown by a factor of 30, from 103,305 inhabitants in 1905, to 3,317,166 inhabitants by 2005.
2.1 Rainfall in the Colombian Andes
The Colombian Andes exhibit a complex hydrometeorological behaviour associated with their tropical environment and influenced by different elements such as (i) topographic gradients of the three Andean mountain ranges in Colombia (ii) atmospheric circulation patterns over the Pacific and Atlantic Ocean (iii) strong earth-atmosphere feedback and iv) the combined hydroclimatic and ecological dynamics of the Amazon basin and the Orinoco45. The annual rainfall pattern in Colombia is mainly controlled by the effects of both phases of the ENSO (El Niño-South Oscillation) phenomenon, and intra-annual scale by the 40 to 50-day oscillation phases and the tropical dynamics of waves during the boreal summer affecting precipitation regimes in the different regions of Colombia46.
The ENSO in Colombia is manifested through combined effects of sea surface temperature anomalies of the Pacific coast, relative to those of Ecuador-Peru, and atmospheric teleconnections, combined with feedback from the Earth's atmosphere47,48. During El Niño, there is a decrease in precipitation and mean monthly flows of Colombia's rivers, as well as a decrease in soil moisture49, while La Niña is generally associated with positive precipitation anomalies48,49. The bimodal behaviour of the annual precipitation cycle is mainly observed in central and western Colombia, where rainfall peaks are marked in the months of March-April-May (MAM) and September-October-November (SON). The minimum rainfall peaks are marked in the months of December-January-February (DJF) and June-July-August (JJA), as a result of the double passage of the ITCZ (Intertropical Convergence Zone) over the region46,49,50. In addition to those individually complex processes, there is a coexistence and mixture of different time scales in rainfall between macroclimatic phenomena such as the ENSO with nonlinear interactions with the ITCZ and the Chorro del Chocó, generating a complex pattern of hydroclimatic variability in the Colombian Andes48.
The space-time distribution of rainfall over the tropical Andes has an additional variability due to the strong orographic characteristics of the country with the presence of three mountain ranges with elevations exceeding 4,500 meters above sea level and inter-Andean valleys with north-south directions48,51,52. This topographical configuration induces local atmospheric circulations that enhance deep convection48,53, as a fundamental mechanism in the formation of tropical precipitation52.
Some changes in trends and variability of hydro climatology have been reported by different authors in Colombia54,55. For a conservative CC scenario in the period 2010-2100, Colombia achieved an escalation in the average temperature of 2°C and a rise in precipitation between 10-30% to the central and western country56. Other studies have indicated for the Colombian Andes increasing temperature trends from 0.1 to 0.2°C per decade, and changes in extreme precipitation events between -4% (reduction) and 6% (increase) per decade55,57.
Recent studies analysed CC and variability for the Aburrá Valley, using analysis of long-term precipitation trends at the monthly scale, and Coupled Model Comparison Projects (CMIP) using the model Weather Research & Forecast (WRF)58. Results reported an increasing and significant trend in two stations located in the centre of the valley, while for the other seasons, the annual variation is dominated by long-term trends. Additionally, rainfall changes in extreme precipitation values were studied at the hourly scale, finding that frequency of higher-intensity events tends to have increments of 0.2 mm per decade for the 70th percentile. The dynamic subscale models with the WRF showed changes in precipitation rate. Precipitation rises in July and September and decreases in January, May, and November.
3. Models background
Two physical-based models were used to assess the effects of rainfall changes on landslide hazards. For rural areas, the steady-state hydrologic model, SHALSTAB, was used with a digital elevation model (DEM) with a spatial resolution of 5 m; and for urban areas, a transitory model, TRIGRS, with a DEM of spatial resolution of 2 m. Both models allow incorporating rainfall directly in terms of intensity in their analysis, a key variable in the impact of CC in the landslide occurrence.
The SHALSTAB model employs the hydrological model TOPOG59 in steady-state conditions to establish a moisture pattern map based on the accumulated area, slope, and soil transmissivity of each point35 where soil moisture is calculated as stated in Equation (1).
Where Q is rainfall in stationary conditions (mm/d), a is the drainage area (m2), b is the length of each cell (m), T is the transmissivity of soil under saturated conditions (m2/d), and
is the slope angle. If the soil transmissivity does not vary with depth, it is possible to assume Equation (2).
Where h is the thickness of the saturated soil and z t the total thickness of soil. By combining Equations (1) and (2), it can be estimated the relative saturation of the soil profile as Equation (3).
As for the geotechnical component, the SHALSTAB model is based on limit equilibrium analysis with infinite slope and the Mohr-Coulomb failure criterion, as seen in Equation (4).
Where ρ s is soil density, ρ w is the density of water, g is the acceleration of gravity, C’ is the effective soil cohesion, and φ is the friction angle. This equation can be written in terms of the h/z t ratio as Equation (5).
Finally, by coupling the hydrological model (Equation (3)) with the stability model (Equation (5)) the Equation (6) is obtained.
From this equation, it is possible to determine four stability conditions for each pixel. Pixels where the relationship between the accumulated drainage area and the pixel length (a/b) is greater than the expression on the right side of the equation correspond to unstable cells; otherwise, they are stable cells. The remaining two conditions correspond to stability conditions that do not depend on rainfall. Stable pixels in fully saturated conditions of the entire soil profile (h/z t =1) are called unconditionally stable and meet Equation (7).
Additionally, unstable pixels in dry conditions (h/z t =0) are called unconditionally unstable and meet the Equation (8).
The TRIGRS model couples a one-dimensional vertical infiltration model with an infinite slope stability model to evaluate changes in the factor of safety37.
The infiltration model implemented by TRIGRS is based on the linear solution of Richards' equations37,60. The flow of water in the soil is the result of the sum of a steady-state and a transient component associated with the rainfall event selected. The solution for the case of an impermeable basal at a finite depth is given by Equation (9).
Where
is the pressure head, t is the time,
, z is the coordinate in the vertical direction (positive down), z the coordinate in the normal direction of the slope and δ is the angle of the terrain with the horizontal; d is the initial depth of the level in a vertical direction.
, K
s
is saturated hydraulic conductivity in the direction Z
the steady (pre-storm) infiltration rate into the surface of the ground.
is the rate of infiltration at a given intensity for the nth time interval.
, D
0
is saturated hydraulic diffusivity (
, where S
s
is specific storage). N is the total number of intervals, and
is the Heaviside step function, where t
n
is the time in the nth interval in the rainfall infiltration sequence. The function ierfc has the form
, where
is the complementary error function (37).
To estimate changes in the factor of safety as a function of depth (Z) and time (t), the infinite slope stability model is obtained according to Equation (10).
Where
is the pressure head as a function of depth (Z) and time (t).
4. DATA
4.1 Intensity-Duration-Frequency Curves (IDF)
Although the consequences of CC have local effects, to assess the impact on rainfall and landslide occurrence is complex. This complexity is mainly because output variables of CC scenarios by global numerical models coupling ocean-atmosphere and subscale techniques are at different geographical and temporal scales than those required by landslide occurrence models in special for precipitation, as this is the direct input variable in physical-based hazard models.
In addition to this fact and considering that changes in precipitation occur both in the intensity of rainfall events, as in the duration and frequency, it is recommended to use as input IDF curves. In this way, simulated rainfall events incorporated into models as triggering for landslides can use different scenarios in terms of the intensity, duration, and frequency of rainstorm events.
With these considerations, for this study, the rainfall changes were taken from a study where long-term trend analysis methodologies were used to project 100-year IDF curves, a simplification that implies that the mean and variance of the data change according to the trend of the last 20 years as seen in Table 2 61. IDF curves were built for 5 rain gauges with 5 minutes temporal resolution, located along the Aburrá Valley, 3 in the south (Ayurá, Caldas, and San Antonio de Prado), and 2 in the north (El Convento and Chorrillos). For each rain gauge, the area of influence was defined using the Thiessen polygons, assuming that the rainfall magnitude was the same for the entire area of influence. Figure 1 presents the location of the rain gauges used in this study.
For the SHALSTAB model, rainfall intensities were used for a duration of 1 hour and return periods of 10, 25, and 50 years. For the TRIGRS model, intensities were used for a duration of a 4-hour event and return periods of 10 years. The selection of this duration for the rainfall event, for the TRIGRS model, results from a parametric analysis with variations in duration and rainfall intensity that result in the most critical condition in terms of the highest percentage of failure area.
4.2 Parameterization of models
Physically-based models allow considering the spatial variability of input parameters; however, a proper representation of such variability requires a great deal of effort in estimating the parameters. This study assumes that spatial distribution is controlled by the distribution of geological surface formations, available at 1:10,000 scale for the Aburrá Valley62. For each geological unit, the geotechnical parameters were assigned as reference values according to AMVA63. In addition, parameters of detailed geotechnical studies64,65 were considered and compared. Subsequently, the parameters proposed by those studies were compared with typical values found in the literature; from the typical soil type developed by each geological unit in order to assess the uncertainty of the parameters using the ranges of values collected66. Table 3 presents the selected geotechnical parameters; mean values were used, without considering the variance, since a deterministic approach of the stability was assessed.
4.3 Soil depth
The significant change or reduction of permeability across soil horizons corresponds to a hydraulic border that determines the formation of a perched water table between horizons67. This process, which is essential for establishing stability conditions, requires estimating the thickness of the failure surface, which corresponds to the area where a sudden change in hydraulic properties occurs.
To determine the spatial distribution of sliding soil thickness (h i ) throughout the study area, the model from Equation (11) was used68:
Where
is the slope,
y
correspond to the maximum and minimum slope values, while h
max
and h
min
are values that restrict the thickness (h
i
), established from the weather profiles69. h
max
was selected as the thickness value corresponding to the shallowest horizon according to the profile proposed in each unit70, highlighting changes in granulometry and physical properties that allow estimating the limit of the sliding material. However, for materials transported such as slope deposits, a constant value could not be clearly established due to its heterogeneity and significant changes in thickness, so a maximum thickness was assumed (h
max
) of 3.5 m. The assigned value of h
min
for all units was 0.2 m.
4.4 Hydraulic conductivity (ks)
One of the main variables representing sub-surface flow conditions are the hydraulic characteristics of soil71, where soil hydraulic conductivity controls the generation of pore pressure and its subsequent dissipation through the soil structure, and therefore, dominates the occurrence of landslides72. However, this property is a complex parameter to be determined precisely due to its spatial and temporal variability73-74.
The estimation of hydraulic conductivity was based on the typical soil classification existing on each of the geological units, as well as for the other parameters. For soil classification, the geological description of the most superficial layers is used, according to the weathering profile associated with each unit, in addition to the soil characterization available in a study69. This information was compared and supplemented by the soil classification given by the POMCA75 and the description of the mineral composition of geological units, referred to the explanatory report of the Geological Map76. Subsequently, hydraulic conductivity (ks) values were assigned within the ranges proposed by the Association of Swiss Road and Traffic Engineers77 and by different authors78-80. Table 3 presents the soil type and the value of ks, corresponding to each geological-geotechnical unit. For this study, hydraulic conductivity was considered constant for each geological unit since there are no experimental values to analyse the distribution of this hydraulic characteristic within the same unit.
5. Results
Figure 2 presents the hazard maps using SHALSTAB for current conditions (A) and considering rainfall changes in CC scenarios (B) for seven municipalities in the Aburrá Valley. The model used rainfall events of 1 hour and intensities for three return periods, 10, 25, and 50 years.
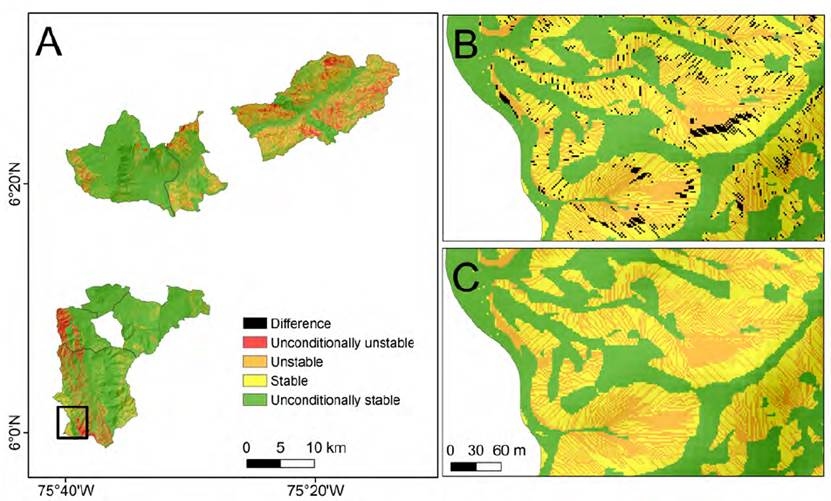
Figure 2 Landslide hazard map for Tr-50 using the SHALSTAB model. (A) Current conditions, (B) Zoom to current conditions with overlapping cells that fail with climate change scenarios and (C) Zoom to climate change scenarios
Table 4 summarizes the results obtained in terms of stable and unstable cells. Unconditionally stable or unstable cells do not vary as they do not depend on rainfall conditions but on the intrinsic soil properties and geometry slopes and therefore are not shown in the table. Meanwhile, unstable cells grow in response to intensified rainfall in the CC scenarios. Results show differences of 11% for 10 years, 13% for 25 years, and 14% for 50 years, showing a reduction in stable cells and an expansion in unstable cells according to the rise of rainfall. Spatially, these areas in which slope stability decrease are concentrated in the southwest of the valley in granitic rocks. This suggests that rises in the rainfall intensities associated will result in a greater number of shallow landslides.
Based on the results obtained in Figures 2, it is observed that the spatial distribution of hazard is mainly controlled by lithology and slope. Granitic units have soils with greater susceptibility to landslides, as well as high slopes that also favour their occurrence. Granitic soils have higher permeability values, so pore pressure responds quickly to rainfall infiltration under intense precipitation events. For the slopes, there is a direct, and non-proportional, relationship between the slope stability and slope angle.
Among the most susceptible areas are those formed by the Antioquia Batholith to the southern valley, the Amagá Stock, and the Quebradagrande Complex in the southwestern region and north-eastern. While the lower slope areas, associated with alluvial and alluvial-torrential deposits, have the lowest susceptibility.
For the analysis of urban areas in the metropolitan area, the TRIGRS model was used, and the factors of safety for the seven municipalities under study were calculated. Figure 3 presents the comparative results of the factors of safety calculated for the municipality of Envigado. Rainfall events of 4 hours duration and intensities no considering CC (A) and considering CC (B) were used for the model for a return period of 10 years.
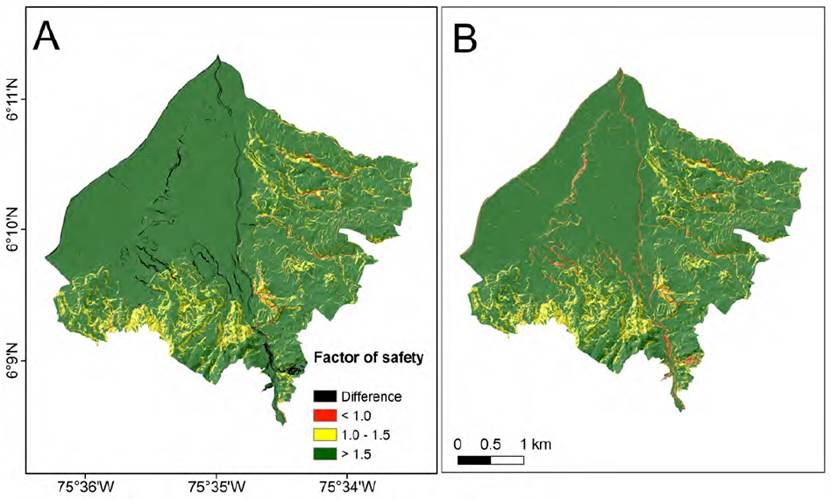
Figure 3 Landslide hazard map for Tr-10 years using the TRIGRS model. (A) Current with overlapping cells that fail with the climate change scenario and (B) climate change scenario
Table 5 presents the results obtained for the seven municipalities of the Aburrá Valley analysed, in terms of unstable cells (cells with FS<1). When comparing cells with factor of safety less than or equal to 1, for scenarios without and with CC, the results indicate a little variation of unstable areas, with the exception of the municipalities of Itagüí and Envigado where unstable areas grow 2.3% and 10.2%, respectively.
Table 5 Results in terms of unstable cells (FS less than or equal to 1) for the Aburrá Valley with the TRIGRS model
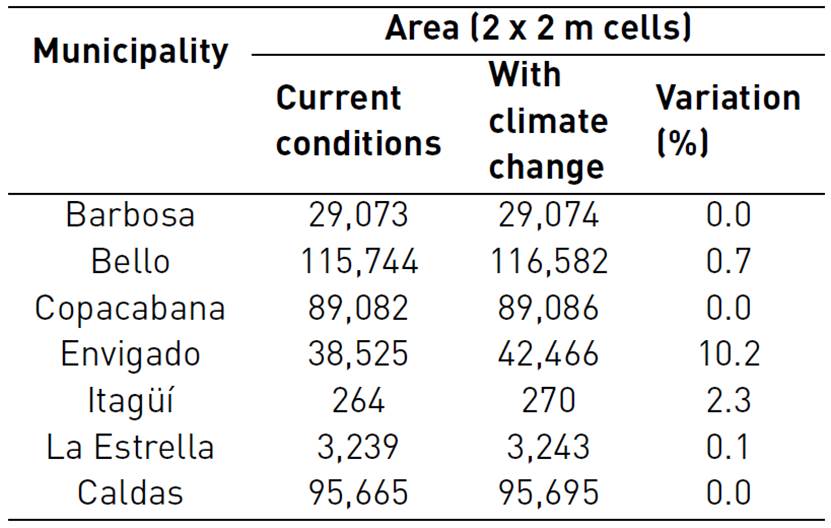
The low increase in areas with FS≤1.0 obtained in most municipalities studied may be explained by the soil infiltration related to rainfall intensity and soil saturated permeability. In soils with low permeability, the rise in rainfall intensity does not contribute significantly to the infiltration of water into the soil but to an augmented surface runoff water. Infiltration into low permeability soils is generated with greater dependence and/or influence of rainfall duration instead of rainfall intensity. In the specific case of the municipality of Envigado, where the greatest growth in the fault area subject to the climate change scenario was identified, these fault areas are concentrated on the north-eastern side of the municipality, predominantly in the slope deposits where permeabilities are high, allowing greater water infiltration and generating a loss of shear strength; this reflected the decrease of the Safety Factor in these sectors.
However, the results are not only the product of geotechnical and hydraulic properties, but all variables involved in the infiltration process, such as geomorphological, hydrological, hydrogeological characteristics, among others, should also be considered. The analysis of slope stability under rainfall conditions involves full knowledge of the territory, in which the variability and complexity of the soil behaviour resulting from the geology, geomorphology, and environment of the Colombian Andes should be contemplated.
6. Discussion
The results obtained in this work indicate that the effects of changes in rainfall intensity, duration, and frequency are not directly related to slope stability. Although raises between 10 and 50% in precipitation intensity for the Aburrá Valley generate an expanded unstable area, such increase is not direct nor proportional. This is because rising pore pressure and reduction in shear strength is also a function of a large number of soil variables71,72,81. Moreover, while soil permeability controls the infiltration process and pore pressure dissipation, hillslope morphometry also plays an important role82, soil cover83,84, and the antecedent moisture of the soil81,85.
Some researchers have shown that shallow landslides are strongly controlled by surface topography35,86, which affects the runoff velocity and the convergence of subsurface flow87,88; not only the slope but the shape of the slope (concavity and convexity) are factors that control the occurrence of shallow landslides89,90. Divergent landforms are generally more stable, followed by flat landforms and concave or convergent landforms, which are less stable91. On divergent or convex slopes, the subsurface flow is dispersed, and pore pressures are typically much lower than on slopes with other landforms15,91. Other authors identified that the curvature of the profile controls the change in velocity of water flow down the hillslope. In contrast, the curvature plane defines topographic convergence, which is an important control for the concentration of subsurface flow90.
For permeability conditions, when comparing the results obtained in Tables 4 and 5, a greater area of unstable areas is observed when modelling a stationary infiltration process (SHALSTAB) compared to the modelling of a transient infiltration process (TRIGRS). Some studies suggest that for conditions of high antecedent soil moisture content, low intensity, and long rainfall duration, the effect of horizontal flow on slope stability tends to be amplified92. For low values of antecedent soil moisture conditions, with short rainfall durations in fine soils, landslide occurrence depends on the redistribution of pore pressures in the vertical profile, where the lateral flow is negligible. However, for long-lasting rainfall cases or more permeable soils, the lateral effect becomes more relevant. Some authors consider that the concept of steady-state subsurface flow is not appropriate to assess the triggering effect of rainfall on landslide occurrence due to the short periods of pore pressure head response in thick soils93,94. Other authors consider that the assumption of a steady state is unrealistic, because these models assume that subsurface flow at any point of the landscape depends on the upstream drainage area, which is valid only if the recharge to the perched water level occurs for the period of time required by each point to achieve the balance of the subsurface drainage and generate drainage from the entire of its upstream contribution area89. Nevertheless, due to the low subsurface flow velocity, this assumption is very difficult to meet; in general, only a small portion of the total drainage area is transmitted. Therefore, steady-state models could be considered as the most critical condition under a conservative scenario. However, it is indisputable that topographic controls of subsurface flow affect long-term moisture patterns within a basin and determine the pore pressure prior to the starting of an extreme rainfall event. Then, the pore pressure should be expressed as the sum of two components, the pressure head produced by long-term infiltration into a steady state with a lateral flow domain, and the response of pressure head in the short term in a transient state dominated by vertical flow60,95. This situation in the field occurs when moisture in soils is high by the presence of antecedent rainfalls, so lower amounts of soil water are required to detonate landslides; on the contrary, when the soil water content is low for a dry period, larger volume of rainfall is required to infiltrate the soil96.
This same behaviour has already been reported by other authors for partially saturated soils who found that the distribution of suction in high permeability soils is more influenced by short, heavy rainfalls, while suction in low permeability soils is governed by rainfall duration97. Studies on soil permeability and hillslope stability indicate that soils with low permeability coefficients are more stable to short rainfall events, while high permeability soils are heavily affected by short-lived, high-intensity rainfall events81,85. The antecedent rainfall is critical for low permeability soils, as these soils require longer periods of time for pore pressure dissipation and recovery of the factor of safety after rainfall events81,98.
This situation also explains that the failure mechanisms for soil slopes with high permeability and low permeability are not similar. Soils with high permeabilities usually show a shallow surface due to a waterfront or a perched water table, while soils with low permeability generate deep landslides by increasing the groundwater table level81. In general, deep landslides respond to antecedent rainfall and not to intense rainfalls as a result of increased water table level that can occur weeks or months after the rainfall events99. Studies find that the difference in the number of preceding rain days depends on the depth of the failure surface, where landslides triggered by high daily precipitation are shallow with depths less than 2 m100. Landslides detonated by antecedent rainfall have deep failure surfaces with more than 6 m.
Therefore, these studies make it possible to point out that the rise in rainfall intensity significantly affects the occurrence of shallow movements (<3 m) in soils of high permeability. Shallow landslides result from a rapid response of the slopes to rainfall conditions, in terms of intensity and duration, as a result of reduced suction or increased positive pore pressures101. These soils usually occur on slopes composed of residual soils of granitic rocks, such as the Antioquia Batholith to the northeast of the Aburrá valley, or smaller geological units, such as the Iguaná Gneiss, or San Diego and Altavista Stocks.
For low-permeability soils, such as the residual soils of the Medellín Dunnite, antecedent rainfall is expected to play an important role in the occurrence of deep landslides, and therefore medium-term changes in precipitation will turn out to have more influence. These changes in CC models have lower uncertainties, so their assessment may be less complex and better predicted24,102. However, as part of the limitations of this study, it was found that both models, SHALSTAB and TRIGRS, use an infinite slope analysis, so they represent only shallow landslides. New studies should incorporate models that allow the simulation of deep failure surfaces, especially considering that disasters generated on this type of soil correspond to the most tragic landslides in our region, as a result of their spatial distribution with respect to the most densely populated areas and the exceptional volumes involving this kind of events (see Table 1).
On the other hand, although economic losses associated with landslides tend to be concentrated in industrialized and developed countries, the number of deaths and people affected is higher in developing and densely populated countries, such as Colombia2,103. The WMO estimated an upsurge of 20% between the 2000s104 compared to the 1990s in the number of fatalities caused by disasters induced by extreme rainfall events, where developing countries have contributed 95% of the victims105. This surge has been the result not only of the rise in the frequency and magnitude of extreme weather events, but also because of occupation and changes in landslide-prone areas. It means that the population size exposed to the occurrence of landslides will suffer an upswing not only by the rise in the occurrence of landslides, but also the occupation of susceptible areas11,106.
Although the implementation of physically-based models for assessing the effects of rainfall changes on the occurrence and distribution of landslides is severely limited, such studies need to be carried out in mountainous terrains such as the Colombian Andes, not only because of the possible increase in high hazard areas by landslides, but also because of the accelerated growth in hillside occupation susceptible to landslides. This is due to the levels of uncertainty at spatial and temporal scales of CC scenarios, especially in precipitation, in addition to the well-known uncertainty in the spatial distribution of soil parameters that control the physical process of hillslope stability.
The above means that it is necessary to develop studies such as the present, in order to evaluate changes in the occurrence of landslides and incorporate in the studies of susceptibility and hazard for land use planning. In general, hazard maps consider stationary climatic conditions, but as discussed in this work, the hydro climatological conditions of the Colombian Andes are not stationary either on short- or long-term time scales. Even without considering CC, there is a strong natural climate variability at different time scales that must be considered in the development of hazard studies.
However, changes in duration, frequency, and intensity of precipitation do not directly affect soil stability conditions; such impact is complex due to multiple variables affecting the process of water infiltration into the soil and increased pore pressure. It is then necessary to make progress both in improving precipitation data at spatial and temporal scales under scenarios of variability and CC, as well as digital terrain models and knowledge of the geomechanical properties of soils and their spatial variability. Additional work must be done to reduce uncertainties in such a way that landslide hazard scenarios under CC conditions provide insightful information and reduce levels of uncertainty for decisions to be taken with higher levels of confidence.
Finally, it is important to consider that increasing precipitation intensity can increase the occurrence of shallow landslides for soils with high permeability that give much more destructive characteristics to such generally flow-like phenomena or torrential flows as a result of the higher water content of the displaced mass, which eventually generates faster movements over greater distances, as well as being deposited over larger areas107. Consequently, it is important to continue to make progress in the implementation of early warning systems supported in rainfall monitoring, but also accompanied by critical rainfall thresholds108,109 and maps of susceptibility and hazard for different rainfall scenarios, in order to generate timely and accurate alerts that reduce human losses, which are usually very high in our territory.
7. Conclusions
There is a general consent with a high degree of confidence on the effects of increased precipitation intensity and frequency on the occurrence of landslides; however, it is necessary to know how such changes will affect hazard conditions on a local scale. This study assessed the effects of rainfall on the landslide occurrence in the northern Colombian Andes through physically-based models, incorporating the intensity and duration of rainfall, as well as the spatial variability of soil parameters and morphometry.
The analysis of rainfall scenarios and landside hazards is needed for land use planning in mountainous terrains. In addition, rainfall scenarios associated with CC permit to estimate the magnitude of unstable areas additionally affected. In this sense, physically-based models become a potential tool to include the complexities of rainfall infiltration processes in saturated and partially saturated soils. Although the models used in this work (SHALSTAB and TRIGRS) consider the topographical aspects and mechanical conditions of the soil, it is also necessary to implement models that simulate deep-seated landslides, which respond to medium-term rainfall conditions, allowing the use of rainfall information under CC scenarios with less uncertainty.
Both SHALSTAB and TRIGRS have proven useful in the determination of unstable areas and landslide hazard zoning in tropical soils110,111 and allow assessing the difference in low- intensity long duration rainfall and high-intensity low duration rainfall impact on triggering landslides112.
The results found using the SHALSTAB model showed that for the CC scenario, an upsurge in landslides for the study area of 11%, 13% and 14% for return periods of 10, 25, and 50 years, respectively. These results show that in the scenario where a steady flow with a high rainfall intensity is considered (the most critical condition under a conservative scenario) result in an increase in the number of landslides; however, the escalation rate in landslides decreases as rainfall intensity rises for greater return periods. In the case of the TRIGRS model, none or lesser raises in the landslides were obtained for the case of transient flows, which can be explained by the relationship between rainfall intensity and soil hydraulic conductivity. Considering that the soils in the studied area have low permeability, the rise in rainfall intensity does not mean greater infiltration of the water into the soil, but an augmentation in surface runoff water. Infiltration into low permeability soils is more sensitive to rainfall duration than to rainfall intensity.