INTRODUCTION
The enormous literature about the effects of natural resource abundance on the economic performance and welfare, suggests the existence of a sort of “curse of natural resources”, this phenomena often takes a specific form, usually called “Dutch disease”. This disease occurs when a natural-resource boom causes over-appreciation of the local currency and deindustrialization. Corden (1984) consolidates the first survey on booming sector economics and Dutch disease. More recently, Sachs and Warner (1999, 2001), Bravo-Ortega and De Gregorio (2005), Maloney, Manzano and Warner (2002) ,Lederman and Maloney (2007), Michaels (2010), Van der Ploeg (2011) and Rajan and Subramanian (2011) among others, show that long-run economic growth based on natural resources (oil, coal, cooper, etc.) is slower than the growth based on manufacturing production, and that Dutch disease increases the relative prices of non-tradable goods, affecting capital goods prices, investment rates and economic growth. This combination of facts could push the labor force from the manufacturing sector to mining and/or the agricultural sector, and therefore reducing labor productivity.
Many cross-country studies based on cross-section data frequently find a nega tive association between natural resource abundance and economic growth, see for instance Sachs and Warner (1995, 2001); while other papers, taking advantage of both cross-section and time-series variations, do not find any effect, for example, Manzano and Rigobon, (2001), and Raddatz (2007). Institutional differences and the period of time analyzed may explain these differences, such as in Mehlum, Moene and Torvik (2006); Collier and Goderis (2007), and finally, in Van der Ploeg (2011). In spite of their contributions, these papers present methodological problems and uneven data quality, as well as problems due to the non-treatment of omitted variables, which usually could be correlated with natural resource abundance.
Recent studies have tried to address some of these problems by taking advantage of commodity production information among national borderlines. These studies have focused mainly on oil extraction. A partially consistent pattern among cross-country studies has begun to emerge, for instance, Caselli and Michaels (2013) investigates the impact of a positive shock of the oil production across similar Brazilian municipalities. They found no impact of this shock on the gross domestic product (GDP) and in spite of an increasing public spending (supported by the oil income) over a wide range of budget items, its impact on the governmental transfers, public goods provision, infrastructure and household income has been too weak. Michaels (2010) studies the impact of petroleum abundance in southern U.S. on its long-run development. He finds evidence that oil abundance tends to increase local jobs, population, per capita income and quality of infrastructure.
Of course, what we expect from any type of underperforming economic growth, especially if it is due to commodities boom and Dutch disease, is a decreasing value of the quality of life indicators. However, the empirical literature does not display strong evidence about this relationship. Hajkowicz, Heyenga and Moffat (2001) find no evidence of systematic negative associations between quality of life and the gross value of minerals production. Instead, mining activity has a positive impact on incomes, housing affordability, communication access, education and employ ment across regional and remote Australia. In the same way, Rolfe et al. (2007), find that the coal industry boom in Central Queensland’s Bowen Basin has generated a number of positive economic and social impacts, including increased employment, income and expenditure levels. Loayza, Mier y Teran and Rigolini (2013) estimate the impact of the coal boom on the Peruvian economy. They conclude that this boom has brought huge benefits that have impacted many social variables such as poverty, by improving some needs indicators related to UBN.
In this paper, we are interested in investigating the local impact of gold mining activity on several socio-economic indicators in the Colombian municipalities, as the unmet basic needs (hereafter UBN), some poverty measures such as the Multidimensional Poverty Index (hereafter MPI), and others related to number of people with access to public utilities (as% of the population). To achieve this goal, we use information from a large dataset that was built appending the 1993 and 2005 national censuses, provided by the National Administrative Department of Statistics DANE (as its acronym in Spanish).
The main motivation to carry out this investigation is that, after an exhaustible searching in the literature on the impact of mining boom on social indicators, we barely find interesting papers, which currently is a topic that have captured the attention of the researchers. Moreover, the current literature that investigates de impacts of the natural resources boom on welfare and development in many countries have provided inconclusive results, even if we focus on Latin-American countries, where the boom has had important repercussions since 2001, see for instance Manzano and Rigobon (2001) and Raddatz (2007).
It is worth noting that the identification of the local impacts of gold mining boom requires dealing with some methodological issues that are crucial for both a correct empirical specification and the selection of variables that describe the characteristics from municipalities. It is even more important to point out two features of the data we are dealing with: first, the regional scale of the analysis imposes the treatment of the exact location of the natural resources (whether it be at the municipal or at the departmental level), since it is very likely that when two or more municipalities share the whole of these natural resources, determining the effect of any mining boom brings along more complexity.
Secondly, distinguishing in the sample between producing municipalities and their non-producing neighbors, as Loayza, Mier y Teran and Rigolini (2013) has shown, allows us to treat the endogeneity problem that underlies the decision of localizing productive activity; thirdly, we must consider that the sample should be restricted to municipalities that effectively have mining activity, with the aim of reducing specification problems due to bias by omitted-variable, among other problems that must be specified in the section of describing the methodology.
In this paper, we only consider mining activity related to gold extraction, not only because gold mining is the oldest type of mining activity in Colombia but also because gold is the commodity of exist more reliable available statistical information exists. But moreover, the price of gold has had a dramatic increase since 2001, exacerbated by the 2008 global economic crisis when gold became the investors’ shelter.
Figure 1 shows the evolution of the international gold price. Note the sustained growth in gold prices since September of 1999 up to September of 2014, with a nominal variation of almost 360%, jumping from 264.7 US$/Oz in September to 1999 up to 1234 US$/Oz in September of 2014. Because of the increase in the international prices and due to the crisis in the European Union and the United States, gold production has also increased notoriously in Colombia, above other commodities such as silver and platinum.
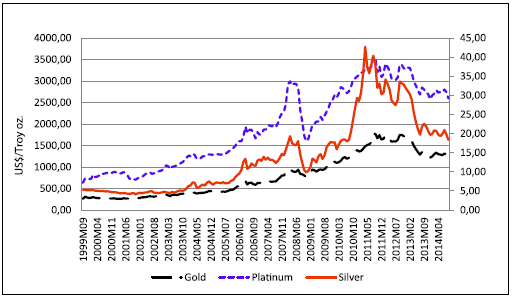
Source: World Bank Commodity Price Data (The Pink Sheet)
Figure 1 Gold monthly price evolution 1999-2014
The problems associated to gold mining, make Colombia an illustrative case of study about the tension between taking advantage of the mining boom versus economic development. As could be observed in map in Annex, the location of mining zones and mining municipalities is distributed among the Western and Central Mountains and covering one part of the Eastern Mountains. In fact, what we are describing from a geographical point of view is part of the periphery of the country. This situation suggests that the distribution of the benefits of mining activity may not be so egalitarian, in principle, and that the economic and social benefits are not expanding to the rest of the population.
In order to estimate the impact of mining gold boom we chose two types of municipalities: gold producing municipalities (treatment group) and gold non-producing municipalities (control group) that should be spatially close to each other and should have similar observable characteristics. We take some social indicators as outcome variables from the DANE censuses, and then our strategy is to estimate the difference in those outcomes by controlling the regressions for observables characteristics. So, if the mining boom has impact, we should find, among treated and control municipalities, statistically significant differences in their respective outcomes.
We consider that the fact of being or not gold producing is (in most of the cases) an accident of the nature. In doing so, then the observed sample (municipalities) is almost a random experiment. This means that it can be seen as an almost natural experiment. Therefore, the estimation of the impact of the mining boom is straightforward, because it is enough to perform an ordinary least squares (hereafter OLS) regression of the outcomes variables on a set of explanatory variables -observables characteristic- in particular, a dummy variable specifying if the municipality is gold producing or if it is a neighbor (non-producer) of a producing municipality.
Our main hypothesis is that payoffs of the mining boom have not spread to the population of the municipalities whose main economic activity is precisely mining activity. This hypothesis is confirmed by the empirical result that suggests that the gold boom experimented in Colombia, has not had a significant positive effect on living standards for the municipalities in our sample.
This paper is divided in the following sections. In the first section, we show the domestic context in which gold prices have moved. Section II describes the performed empirical methodology and how we merge the data. Section III shows us the empirical results. In section IV, we make some conclusions and suggest future research line.
1. COLOMBIAN IN THE CONTEXT OF INCREASING GOLD PRICES
At least for the last three decades, commodity royalties (coming from the production of different natural resources, such as, gold, silver, coal, and oil, among others) have become an important source of financing for the country and its regions. According to studies from different offices, such as the National Planning Department (DNP) (as its acronym in Spanish), and the Colombian Central Bank, from 1995 to 2009, the income from royalties amount to 42 billion pesos (almost 16.800 million US$ considering an exchange rate of 2.500 COP/US$).
Encouraged by high international gold prices, Colombia has experienced an important increase in gold production. In contrast to other minerals such as silver and platinum, the volume of gold production has had a sustained increase from 2007 to 2012, and it coincides temporarily with the movement of the prices, see Figure 2. This growth has been caused mainly by a growing demand from emerging economies like India and China, which has led to rising prices.
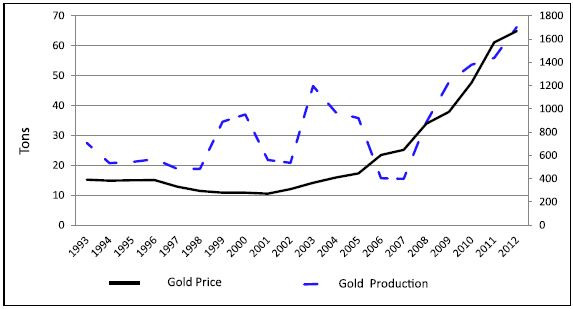
Source: Authors´ own elaboration based on Mining Information System -SIMCO
Figure 2 Production and gold price
Of course, it is evident the importance of royalties from mining production for municipalities’ budget (royalties are transferred by the national government to the municipalities according to Act 1606 of 2013, which creates the National Royalties System). Unfortunately, by the time of the gold boom, the country did not have any Act on mining Royalties, and the allocation of mining surpluses was guided only by a kind of handbook of royalties. The Act came out five years after the beginning in the falling of commodity prices related to precious metals.
It is worth noting that the observed dynamics of commodities prices has been significant for the Colombian economy, and this is because the mining sector has become a significant part of Colombian exportations. Most recent statistics indicates that 21,3% of total exportations correspond to mining activity. Moreover, in the Behre Dolbear Group Survey (a survey about attractiveness for investing in mining) Colombia is at 7th place among 25 countries.
Antioquia is the main gold producer in Colombia, with almost 68% of national production as of 2006, and it is also the region that has the highest level of mod ernization in gold extraction. After Antioquia, we have other important producers as Chocó and Bolivar, and to a lower degree we have Cauca, Tolima, Santander, Nariño, Valle del Cauca and Risaralda. Thus, most of the municipalities involved in this research are placed in those departments (see map in annex).
2. EMPIRICAL STRATEGY
2.1 The source of the Dataset
In order to analyze the possible effect of mining boom on the standard of living of municipalities we consider the following outcomes variables: UBN, MPI (headcount) percentage of illiterate population (%), sewerage coverage (%), electricity coverage (%) and aqueduct coverage (%). These variables are available for 2005. We have the UBN also available for 1993, thus, we can evaluate if there were pre-treatment differences (before the boom period) between treated and control groups.
The dataset used in this paper is the result of merging three large surveys: 1993 and 2005 censuses, provided by the DANE, gold mining production taken from the Mining Information System (SIMCO, as its acronym in Spanish) and some socioeconomic characteristics from municipalities collected from The Center for Economic Development Studies (CEDE, as its acronym in Spanish) from University of the Andes. Our cross-section sample is at the municipal level, the country’s most disaggregated political and administrative division.
For econometric analysis purposes, the set of municipalities was split into three categories. First one: the municipalities that we call mining districts, denoted by DM, which are municipalities that reported gold production in the period 2001-2004. Second, we have the non-producing municipalities which are the closest neighbor to the mining districts, we call them first-order neighbors and they are denoted by FON. In order to classify any municipality as FON we used the Queen Contiguity Matrix, which considers as first-order neighbor any municipality that shares boundaries (edges or vertices) with the mining districts. Finally, we have municipalities that are not producers, neither are they closest neighbor to mining districts, they are placed far from any mining zone or its influence, and therefore, we refer to them as second-order neighbor or SON.
Our total sample consists of 1119 municipalities, 201 municipalities classified as DM, 380 municipalities classified as FON and 538 municipalities classified as SON. We also take into account time-invariant variables taken from 1993 DANE census, in order to control for different socio-economic characteristics of municipalities before the boom.
2.2 Descriptive of the variables
The econometric strategy is based on comparing outcome variables for gold producing municipalities and non-producing municipalities that are similar in their observable characteristics. We mean by outcome variable (or variable of interest) the variable we expect to be modified because of the treatment. In our case, we expect that the gold mining boom has a measurable impact on some welfare indicators. The outcomes variables and the observable characteristics (some of them time-invariant) are listed in Table 1.
Table 1 Impact Variables at Municipal Level
Outcome variables |
---|
UBN 1993 and 2005 |
Illiterate population (%) in 2005 |
MPI for 2005 (Incidence) |
Sanitation coverage in 2005 (%) |
Electricity coverage in 2005 (%) |
Aqueduct coverage in 2005 (%) |
Control variables |
Log 1993 population |
Municipal land Gini in 1984 |
Dummy if the municipality produced coca leaf in 1994 |
Dummy if the municipality produced opium in 1994 |
Altitude (km) |
Terrain roughness level index |
Precipitation |
Distance to the capital of the department (km) |
Area (km2) |
Square log 1993 population |
Rurality Index (%) |
Source: Authors´ own elaboration
Table 2 shows the descriptive statistics for the observable characteristics of the different municipalities belong to DM, FON and SON. The descriptive statistics show that simple averages compared among types of municipalities do not provide much evidence of the impact of the mining boom. However, you can see that when making a comparison of means over 1993, the UBN index has decreased.
Table 2 Descriptive Statistics
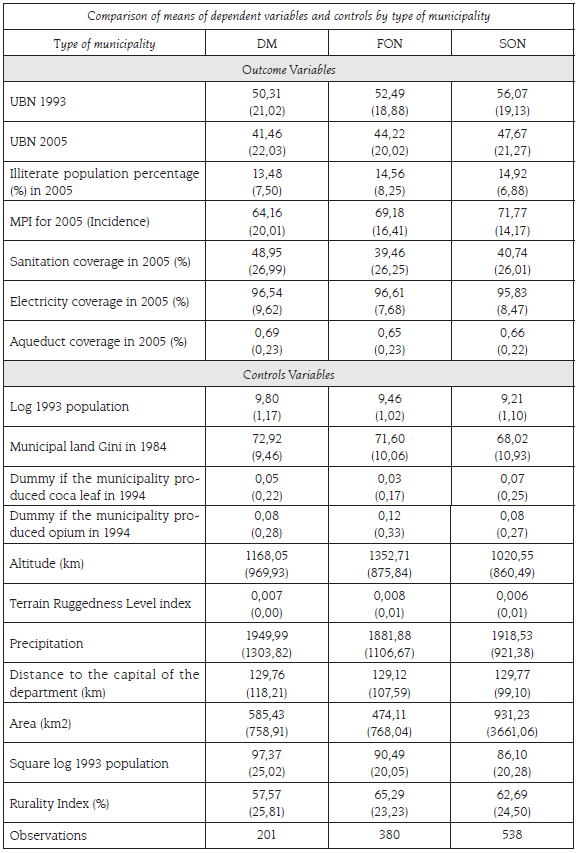
Note: Standard deviation in parentheses
Source: Authors´ own elaboration based on DANE, SIMCO and CEDE.
According to Table 2, it is worth noting that the main difference on averages of the observables variables between the mining districts, the first-order and second-order neighbors is in sewerage coverage and MPI, This would indicate that there are certain differences in the values of the dependent variables for each of the categories before the boom, but it is necessary to verify this by controlling for the observable characteristics of every municipality, On the other hand, it is clear that there are municipalities with differences in the time-invariant observables because of spatial distribution of municipalities and its wide variety of climate.
3. METHODOLOGY1
The impact evaluation is carried by constructing two variables; the first one is a binary variable that takes the value of 1 for producing municipalities and 0 in other case, The second one is another binary variable that takes the value of 1 if the municipality is a FON and 0 in other case, The motivation to define this last variable, as we mentioned before, is to check if there is any spillover effect of the mining activities, The 1993 DANE census data is used to control for municipal characteristics in 1993, prior to the mining boom. This means to control for the municipalities’ initial conditions, so, the underlying regression can be specified as follows:
Where Y i denotes the variable of interest at municipality i,II i [DM] and II i [FON] are the aforementioned binary variables, Xi is a set of observables characteristics in 1993, VR is a specific regional fixed effect and ϵi, is a classic zero-mean error term, It is worth pointing out that under equation (1), we are considering two types of treatment groups: producing municipalities and first-order neighbors, Therefore, the estimates of β 0 and β 1 can be interpreted as the respective impacts on the two types of treatment municipalities, In particular, to have gold (underground or in rivers) is a natural phenomenon, and later, in most of the cases towns and municipalities settled down.
Equation (1) is the base for a diagnostic analysis in order to verify the possibility of pre-treatment differences, then, by taking the UBN index from the 1993 census, we can test if the two sets of municipalities under treatment and control had different UBN levels before the mining boom, later, as an alternative to correct for differences in observed characteristics between DM, FON and SON, we can perform a matching procedure based on observable time-invariant characteristics and the characteristics of 1993, specifically, we match municipalities belonging to DM and FON with non-producing municipalities of similar characteristics. This could be done by constructing a propensity score (PS) that is built upon a Probit regression, the most popular estimators using matching PS are the average treatment effect (ATE) and the average treatment on the treated (ATT).
Our motivation to estimate ATE and ATT is because we consider that the set of observable variables is not determined by what in this context is considered the “treatment” (gold mining boom). Likewise, we assume that potential outcomes on the variables of interest (on which we will measure the impact) are independent of the treatment, in particular (again) because the condition of being a mining municipality is (almost) an accident of nature.
This implies that the conditional independence assumption (CIA) is satisfied. Such assumption says that the expected value of the outcome variable for municipalities treated in the absence of treatment equals the expected value of the outcome variable for municipalities non-treated and without treatment, all expected values conditioned on observable variables X, then:
and therefore the selection bias equals zero. Note that if we estimate the impact of the boom-mining by comparing the outcome variables in the treatment group and the control group we get:
If we add and subtract the counterfactual E (Yi (0) | Di = 1, X) then:
So we have the ATT plus the selection bias, which disappears assuming the CIA is satisfied. So we get,
As stated, the matching is done by using PS, since the matrix X contains many variables, So instead of using X, we match municipalities classified as DM, FON and SON by their estimated probability of being gold producers, or being treated, given that they are geographically very close each to other and that they are very similar in their observables; in other words, we are matching municipalities based on the following conditional probability p(X) = p(D = 1 | X), which can be estimated based upon a Probit estimation. In doing so, we avoid the well-known curse of the dimensionality.
Therefore, the ATT estimator based on PS -by assuming that the CIA assumption is fulfilled- is given by:
And it is reported in tables 5 and 6. We estimate the ATE and the ATT using an Epanechnikov kernel.
Finally, we extend the analysis to explore whether or not the amount of gold production affects the outcomes variables. For this aim, we replace the binary variable IIi [DM] in equation [1] with the logarithm of 1+ f * 1 where f * 1 is defined as the aggregated value of mineral production in each municipality in the period 2001-2004. Thus, the empirical specification can be written as:
The results of the estimation based on (3) are reported in table 3.
4. ECONOMETRIC RESULTS
To start, we consider analyzing the possible existence of pre-treatment dif ferences in UBN index, the outcome variables available for 1993, Then, we compare the UBN for municipalities belonging to DM, the FON and SON by performing ordinary least squares in equation (1); thus, given that we have an almost random sample, we get consistent estimators of β 0 and β 1 , and thus obtain evidence of whether or not there were pre-treatment differences.
The empirical results of the regression (1) are reported in table 3; note that each column shows the different specifications depending on the number of explanatory variables we include. So, the estimated parameters and
for 1993, which tells us if there existed pre-treatment differences between municipalities, show that in terms of UBN there are no significant differences before the boom, In fact, the more observables we include in the regression the less impact we observe, which means that when we take into account all controls any pre-treatment difference disappear.
The next step is estimating the same regression model with data for 2005, the year after the boom, by performing OLS. The, estimated parameters, and
, capture the effect of the mining-boom, which started around 2001, in this case, aside from the UBN index, we include other variables of interest like illiteracy (%), MPI (%), sanitation, electricity and aqueduct coverage. Thus, including the same regressors as in table 3, we conclude that there is no statistically significant difference for both producers and FON with respect to their control group. Table 4 shows us the results of the estimation of equation (1).
Table 4 OLS Regression 2005
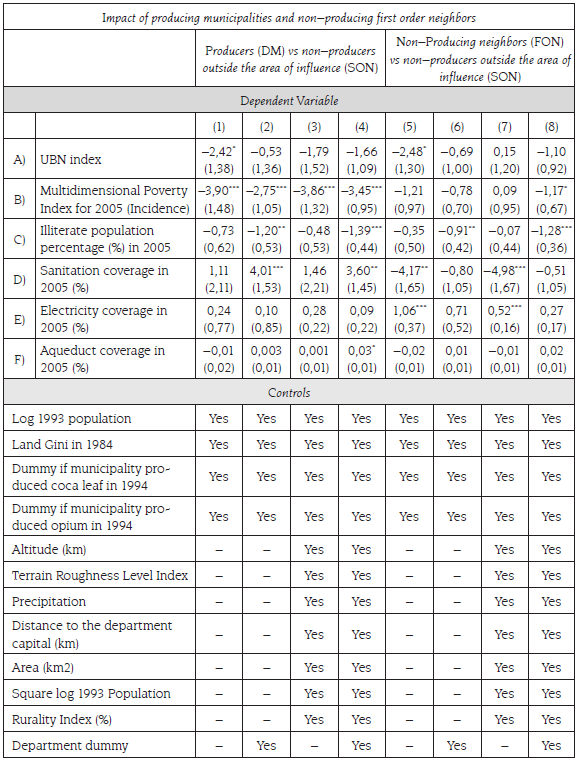
Note: Standard errors in parentheses; *; **; *** indicate significance levels at 10%; 5% and 1% respectively
Source: Own Design using Stata Software
However, there are aspects to be considered. First, the MPI is the only one showing a significant negative difference between producing municipalities and the FON for all regressions, it is not the case when we compare the results for FON and SON groups, This can be explained in part because, in contrast to the other dependent variables which are more specific by definition, the MPI reflects many unobservable characteristics that are captured in the regression through error term.
Second, the results indicate a significant negative difference in the percent age of illiterate population when we take into account the department fixed effects and, in particular, when we include all regressors (columns (4) and (8)). Therefore, the evidence suggests that maybe the boom could positively impact the poverty and illiteracy condition of the population, Third, note that estimated parameter in columns (5)-(8) show evidence of spillover effects just in few cases such as UBN, sanitation and electricity coverage.
In order to confirm the results above, we perform propensity score match ing analysis, tables 5 and 6 show the average treatment effect -equation [6]- and the average treatment effect on the treated estimators. This, estimation is carried out firstly for 1993, and as can be seen, there is no evidence of pre-treatment differences; however, the results for 2005 are not as good as we expected. The ATE and ATT show acceptable results for MPI, which show a negative and significant estimated parameter.
Table 5 Average Treatment Effect
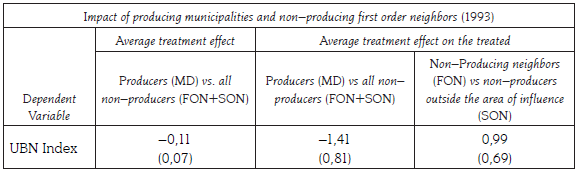
Note: Standard errors in parentheses
Source: Own Design using Stata Software
Table 6 Average Treatment Effect
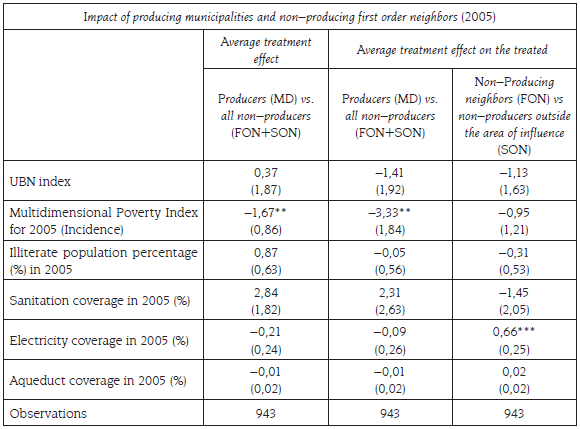
Note: Standard errors in parentheses.
Source: Own Design using Stata Software
Finally, we calculated the effect of a change in the production of gold on each of the living condition variables, The results presented in table 7 show the cumulative effect of (up to 2005) -equation [7]- gold production in the mining districts, thus the variable under consideration has an effect on multidimensional poverty index and coverage of water supply, but only when it is not conditioned by all control variables considered, however the sign is opposite when we include all regressors, so it can be evidence that perhaps cumulative production has no correlation with the geographical conditions of the municipalities.
5. CONCLUSIONS
As disclosed throughout the various proposed econometrics exercises, there are no conclusive results about the effects of the mining boom on the standard of living of Colombian mining municipalities, while the trend is that the effect is not statistically significant in most of the outcomes variables considered in the study, the multidimensional poverty index displayed a greater negative and statistically significant impact most of the times, compared to the other dependent variables.
This is important, because it has been mentioned that the gold and mining boom in general does not have a great effect on employment, at least on qualified employment, and employment is the main source of livelihood of families in the municipalities. What the results show is that in the mining municipalities, compared with first-order neighbor, the boom brought a decline in MPI, perhaps associated with higher (barely observable) income; and however, it is not easy to predict what would happened in the next three years after the boom took place, in particular with the generalized decline in commodity prices, particularly from the gold according to Figure 1.
In some cases there has been a positive post-boom effect on UBN; in other cases, illiteracy has decreased and the percentage of municipalities with access to water and sanitation has increased, not being the same situation with the electricity coverage, in particular because of the expansion the electric system is not associated with the volatility of the prices of precious metals.
Of course one of the weaknesses of this study has to do with the fact that no information concerned to royalty Act was available by the time of the gold price boom. So, we do not have evidence of the impact of royalty Act on population welfare. And we are sure that this Act had enormous impact on UBN indicator.
One of the utility of this paper is related to the reliability of the official statistical information. More precisely, the data set is not telling us the whole story about the gold production and the socioeconomic situation of the mining districts. We mean that by considering the dramatically increasing of the gold price and the over production of gold it would necessary to observe a proportional increasing of the welfare indicators, but is not the case. Maybe the illegality and informality of the production is responsible of the low performance of these social welfare measures.