Introduction
The challenges in terms of food security for human societies for the coming decades are enormous. Recent projections of human population growth indicate that global population will reach ca. 9 billion by 2050 (FAO, 2010; UN, 2010). Global food demand will double and at the same time competition for the use of food crops to produce bioenergy will increase (Wheeler and von Braun, 2013; Tilman and Clark, 2014). Such increasing demand for agricultural products will only raise the pressure for natural resources that are vital for agriculture, and this will be compounded by land and water competition of expanding urban centers (Foley et al., 2011; West et al., 2014). Sustainable improvement of crop productivity by closing yield gaps is, thus, a top priority for agriculture across the developing world (Licker et al., 2010; Lipper et al., 2014).
In Colombia, rice is a highly important crop for both food security and farmers' incomes, given its high consumption and acceptance rates. It is, therefore, essential for Colombian daily dietary requirements (Khoury et al., 2014). Rice is the second annual crop in terms of area harvested with a total area of ca. 685,138 ha (30% area in annual crops) in 2018 in Colombia after maize, but in terms of production value it is the first in the nation (DANE, 2016). Colombia is the second largest rice producer in Latin America and the Caribbean (MADR, 2012). In spite of its importance in Colombia, for many developing countries the average on-farm irrigated rice yields are about 50% below their potential (FAO, 2004; Lobell et al., 2009; Licker et al., 2010). In humid tropical conditions, modern rice varieties yield between 10-11 t ha-1, but irrigated rice yields are typically in the range 4-6 t ha-1 for Colombia (FAO, 2004). As in many other countries, sub-optimal management in conjunction with climate variability is the main cause for yield gaps.
In this research, the technical efficiency (a proxy for yield gaps) and its drivers were estimated for a representative sample of rice farmers from different rice growing regions in Colombia using stochastic frontier models (SFMs). In Colombia, only SFMs have been used to quantify technical efficiencies of coffee production (Perdomo and Mendieta, 2007; Perdomo and Hueth, 2011). A large number of applications of stochastic frontier models exist for rice in Asian countries (Xua and Jeffrey, 1998; Mythili and Shanmugam, 2000; Tian and Wan, 2000; Chang and Wen, 2011). The vast majority of these studies focus on estimating production frontiers through stochastic frontier models, typically for panel and cross-sectional datasets. All these studies include variables that are economically directly related to production efficiency functions, such as harvested area, fertilizer applications, and labor and machinery use. However, recent studies also include household socio-economic conditions (Villano and Fleming, 2004) and/or environmental variables, such as temperature and precipitation (Chang and Wen, 2011).
The objectives of this study were to quantify technical efficiencies in rice production across different production environments in Colombia, identify management factors affecting efficiency, and propose realistic changes in management that allow increasing technical efficiency. A database from a survey of 771 representative rice farms from the three main rice producing regions of Colombia (north, center and east) during the period 2007-2015 was used to fit an SFM for a range of environments. The SFMs were built separately for upland (eastern and northern regions) and irrigated systems (central and northern regions) as both systems have very different management regimes and yields. The SFMs were then used to quantify technical efficiencies and their driving factors, and finally to use a sensitivity analysis to assess the management changes required to increase efficiency levels. The findings were discussed in light of potential strategies to enhance farmer incomes and welfare by helping them to reach optimal efficiency levels.
Materials and methods
Stochastic frontier modeling
The overall framework and key concepts used throughout this work were introduced by the following terms. The ability of a given production unit to maximize its output, given a particular set of inputs, is what we term 'technical efficiency'. The overall assumption in our analytic framework is that those units that have the highest productivity form the 'production frontier' that can be estimated from data. Our approach follows that of Kumbhakar and Lovell (2000).
Let q¡ be the amount of rice (kg per harvest) produced by farm i, defined by a production function f (Eq. 1), which is defined by a vector of j variables measured (as fertilize application, man, and machine labor) at the farm (X
l
) with their associated coefficients (
), and a stochastic component n¡.
The component η ¡ is formed by two independent elements (Eq.2).
In summary,
The first element in Equation 2v ¡ is associated with random variations (error) and is symmetrical and independently distributed with mean zero and constantvariance v ¡ ~ N (0, σv) and can take positive or negative values v¡ Є (-∞, +∞). The second element, u ¡ , is the technical inefficiency observed in rice production (q¡) that is asymmetric u ¡ >N (0, σ u ) and g reater than zero and independent from v i .
Given the characteristics of η
¡
and the need to have unbiased and consistent model parameters, the estimators(
) of the stochastic frontier function must be computed us-ing a maximum l ikelihood approach (Aigner et al., 1977). The natural logarithm of the likelihood function (Ln f) is d efined by Equation 4.
where n is the total number of observations (i.e. surveyed farms per system), σs 2 is the variance of the model (Eq. 5), a nd φ(z¡) is the cumulative norm al standard distribution, in which th e parameter ү (Eq. 6) represents the efficiency parameters stemming from both error sources in Eq. 3. When the random effect (v ¡) dominates (i.e. σu 2 → 0 and ү → 0), high efficiency occurs i n a group of farmers(i.e., most farmers adequately u se their inputs to maximize production). Conversely, when the a sym metric component; (u¡) tends towards infinite (i.e. σu 2 → ∞ and ү ≥ 1), the technical inefficiency is the main source of variation in the model (i.e., most farmersuse inputs in a non-optimal way and are hence far from the production frontier).
Finally, the technical efficiency level (TE) can be estimated for each rice farm as the ratio between the actual production (q¡) and the maximum achievable production (q*¡) (Eq. 7).
TE ¡ represents the ratio between the attained and the potential production. Potential production is defined as the production when the rice farmer uses all inputs efficiently. TE ¡ varies in the range 0-1 with high values indicative of high technical efficiency and vice versa. The TE ¡ values can be used to identify farms and municipalities where efficiency is low and, hence, where interventions may be urgently required to close yield gaps.
Study region
Our study region is Colombia, and specifically all provinces -where rice is grown commercially (Fig. 1). The region comprises 11 different provinces distributed across the country and across a range of climatic conditions. Farmers in the Eastern llanos (provinces of Meta and Casanare), the driest region, grow primarily upland rice with relatively low inputs. The central region (provinces of Huila and Tolima) features the largest production quantities. In this region, rice is grow n only under irrigated conditions with relatively high inputs and in a variety of elevations and climatic conditions. The northern Caribbean region is formed by the provinces of Cordoba, Sucre, Bolivar, Magdalena, Guajira, Cesar, and Norte de Santander and features both irrigated and upland systems. This region is the largest in extent and likely the most diverse in inputs and farmer socio- economic conditions.
Input data
Crop data
Crop management and economic data were gathered from a database of the Colombian National Rice Federation (Fedearroz) through the National Rice Survey (ENA, in Spanish). The survey was carried out in a representative sample of 771 rice farms in the main rice producing areas of Colombia (Fig. 1) for the period 2007-2017, for both irrigated and upland production systems. The survey recorded crop yield, seed quantity, cultivar used, farm size, fertilizer use (quantity and frequency), total use of nitrogen and phosphorous, and man and machine labor hours. As an additional socio-economic factor of potential relevance, we calculated access to farms as the distance from each of the 771 farms to the closest primary, secondary or tertiary road.
Table 1 shows the main characteristics of the two systems. As expected from their contributions to national harvested area and production, there are more irrigated farms (509) than upland farms (262) in the database. There is also a difference between their yield, with irrigated rice yielding ca. 30% more than upland rice (about 1.3 t ha-1 more). We note major management differences, with irrigated systems using more fertilizer and more man hours. Due to these differences, we analyzed both systems separately.
TABLE 1 Rice cultivation farm management practices as derived from the National Rice Survey database. Values shown are mean values for each crop system across all observations. Data from Colombia during the period 2007-2015.
Variable / System | Irrigated | Upland | All |
---|---|---|---|
Total number of surveyed farms | 509 | 262 | 771 |
Yield (kg ha-1) | 5,992 | 4,623 | 5,527 |
Quantity of seed used (kg ha-1) | 201.1 | 203.1 | 201.8 |
Average farm size (ha) | 54.3 | 69.1 | 59.3 |
Rate of fertilizer per crop cycle (kg ha-1) | 619.1 | 401.6 | 544.9 |
Total number of fertilizer applications per crop | 5.9 | 3.4 | 5.1 |
cycle | |||
Rate of total nitrogen use (kg ha-1) | 154.3 | 80.9 | 129.3 |
Rate of total phosphorous use (kg ha-1) | 29.9 | 25.9 | 28.6 |
Man labor hours per crop cycle (h) | 102.5 | 77.5 | 92.5 |
Machine labor hours per crop cycle (h) | 48.8 | 77.7 | 60.5 |
Weather data
We gathered daily weather data for the crop cycle (from sowing to harvest) from the weather station network of the Colombian Institute for Hydrology, Meteorology and Environmental Studies (IDEAM). Total precipitation and minimum and maximum temperature data were available for all weather stations. A quality control process was performed on each of the weather stations using the RClimTool software package (Llanos and Arango, 2015) following Esquivel et al. (2018) by detecting and correcting outliers and data gaps. Finally, we assigned the weather information to each farm using geostatistical (autocorrelation) analysis with the geoR library in the R statistical package (R Core Team, 2017). For each farm, we then computed the following variables: total precipitation per crop cycle (P), number of rainy days (i.e. with precipitation above 1 mm, RD), average minimum temperature for the crop cycle (T min ), and average maximum temperature for the crop cycle (T max ).
Soil data
Soil data were gathered from the World Soil Information database (ISRIC, 2014). This database consists of globally interpolated chemical and physical soil properties at a resolution of 30 arc-sec (about 1 km around the equator). Using the package 'raster' of the R software version 3.1, we extracted the organic carbon content and the water holding capacity for each of the rice farms.
Environmental classification
As a preliminary step before fitting the SFMs for each crop system (upland, irrigated), climate and soil data were used to derive homogeneous edapho-climatic groups through a cluster analysis using the FactoClass Method (Pardo and Del Campo, 2007). We used a distance index to determine the optimal number of groups. We grouped farms into environments because, regardless of management or socio-economic conditions, the production frontier and optimal achievable production can shift depending upon the environmental conditions prevalent on the farm (Van Wart et al., 2013; Heinemann et al., 2015). The implication is that the biophysical limit of crop yield varies depending on the prevailing climate (Lobell et al., 2009). We thus deemed it necessary to assess these environmental effects through an environmental group classification.
Quantification of technical efficiencies
Once the farms in each production system had been grouped into different environments, we used the 'frontier' package in the R software version 3.1 (Coelli and Henningsen, 2013) to fit the SFMs for each production system. We transformed all continuous variables as well as crop yields into their log forms and used all crop varieties and environmental groups as binary variables in the models. Technical efficiencies for each farm in each crop system were then derived from the models. Finally, we used the model coefficients and the results of an F-test to determine those variables with strong and statistically significant (P≤ 0.01, ≤ 0.05, and 0.1) effects on yield.
Assessing the potential for reducing yield gaps
To determine the needed improvements in crop management that would lead to increased technical efficiencies and, hence, to closing yield gaps, we conducted a sensitivity analysis using the production functions derived for each system. For each system, we performed SFM model runs by modifying the most relevant inputs (as identified by the F-test, above), both separately and in combination, making sure input changes did not fall considerably outside their observed ranges. Specifically, for those inputs having a significant positive impact on yield, we increased their observed values by 5%, 10% and 15%, and for those having a negative impact, we decreased their observed values by 5%, 10% and 15%. All sensitivity runs were performed for each of the rice varieties that had a strong effect on yield and for each environment, so that our results would provide sensible environmental-specific management recommendations for improved rice farming.
Results and discussion
Environmental groups
Clustering results indicate that for upland rice, there are four groups of environments, with largely varying edaphoclimatic characteristics per environment (Tab. 2). We note large differences in total precipitation and in the number of rainy days. Environmental group (EG) 2 received the most precipitation with a relatively poor distribution (53 rainy days), suggesting the occurrence of extremes. This environment also showed the largest differences between maximum and minimum temperatures and soils with high organic carbon contents. Conversely, EG 3 showed the lowest precipitation (1,012 mm per crop cycle), with a more even distribution as compared to EG 2. EG 1 and 4 showed the best temporal distribution of precipitation that may result in yield advantages, although their organic carbon contents were about half those of EG 2.
TABLE 2 Environmental groups and their average climatic and soil characteristics for both irrigated and upland rice production. Data from Colombia during the period 2007-2015.
System | EG1 | P (mm)1 | Rd (d)1 | T min CC) 1 | T max CC) 1 | ASW | OC |
---|---|---|---|---|---|---|---|
1 | 1,425 | 68 | 18 | 35 | 12 | 13 | |
Upland | 2 3 | 2,097 1,012 | 53 41 | 12 10 | 34 35 | 12 12 | 22 20 |
4 | 1,507 | 69 | 20 | 35 | 10 | 11 | |
1 | 904 | 52 | 17 | 36 | 12 | 13 | |
Irrigated | 2 | 710 | 47 | 17 | 36 | 11 | 29 |
3 | 450 | 31 | 16 | 37 | 10 | 10 |
1EG: environmental group, P: total precipitation per crop cycle, RD: number of rainy days (i.e. with precipitation above 1 mm), Tmin: average minimum temperature for the crop cycle, Tmax: average maximum temperature for the crop cycle, ASW: available soil water, OC: soil organic carbon content.
For irrigated systems, only three EGs were found (Tab. 2). As with the upland systems we found a large variation in the total seasonal precipitation across irrigated EGs. EG 1 had the largest rainfall amounts (904 mm) followed by EG 2 (710 mm), whereas EG 3 showed the lowest seasonal precipitation (450 mm). Large variations were also found for organic carbon, with EG 2 showing the highest content. We noted only small differences between average seasonal maximum and minimum temperatures, suggesting that temperature might not be a limiting factor for irrigated rice yields. The lower (2-3°C below) maximum temperatures in upland systems compared to irrigated systems highlights the importance of irrigation in allowing evaporative cooling in warm conditions (Peng et al., 2004; Julia and Dingkuhn, 2013).
Upland rice
For upland rice, a statistically significant variable was the nitrogen application rate, with a yield impact of ca. 0.18% for each 1% increase in this rate (Tab. 3). This result is expected (Nagai and Makino, 2009; Mueller et al., 2012), and is also consistent with previously reported nitrogen yield impacts of 0.08% in a study for Taiwan (Chang and Wen, 2011). The amount of seed was also found to have a significant impact on yield, with a regression coefficient of 0.08 ± 0.04. This result can be explained by the fact that Colombian rice systems are directly seeded and large amounts of seed are often required to ensure appropriate germination rates and sufficiently high yields.
TABLE 3 SFA Coefficient estimates for upland rice systems. Data from Colombia during the period 2007-2015.
Variable | Coefficient | Standard Error | Z Statistic | P Value |
---|---|---|---|---|
(Intercept) | 7.39 | 1.89 | 3.91 | 0.00 *** |
log(nitrogen) | 0.18 | 0.10 | 1.88 | 0.06 * |
log(phosphorous) | -0.03 | 0.04 | -0.73 | 0.46 |
log(quantity of seed) | 0.08 | 0.04 | 1.82 | 0.07 * |
log(crop area) | 0.01 | 0.02 | 0.46 | 0.64 |
log(fertilizer use rate) | 0.04 | 0.07 | 0.60 | 0.55 |
log(fertilizer application) | -0.12 | 0.08 | -1.49 | 0.14 |
log(pesticide use rate) | -0.06 | 0.05 | -1.13 | 0.26 |
log(pesticide application) | -0.01 | 0.07 | -0.15 | 0.88 |
log(access) | 0.08 | 0.16 | 0.51 | 0.61 |
log(man hours of labour) | -0.10 | 0.04 | -2.57 | 0.01 ** |
log(machine hours of labour) | 0.00 | 0.03 | -0.02 | 0.99 |
Variety Fedearroz _ 2000 | 0.01 | 0.08 | 0.08 | 0.94 |
Variety Fedearroz 473 | -0.16 | 0.17 | -0.97 | 0.33 |
Variety Fedearroz _733 | -0.23 | 0.07 | -3.33 | 0.00 *** |
Variety Fortaleza | -0.27 | 0.05 | -5.34 | 0.00 *** |
Variety Improarroz _1550 | -0.18 | 0.06 | -2.81 | 0.00 *** |
Environment 2 | -0.10 | 0.17 | -0.58 | 0.56 |
Environment 3 | -0.13 | 0.09 | -1.49 | 0.14 |
Environment 4 | 0.06 | 0.07 | 0.79 | 0.43 |
0.13 | 0.03 | 5.25 | 0.00 *** | |
0.95 | 0.04 | 21.93 | 0.00 *** |
Contrary to what would be expected, we found a negative association (regression coefficient of -0.1 ± 0.04) between man labor hours and yield. While this is somewhat counterintuitive, further analysis of the database indicated that most man labor hours are spent on harvesting activities that would reduce the overall harvesting time but would not increase productivity. It is thus important that increases in man labor time are targeted towards activities that have a greater impact on yield such as fertilizer applications, sowing, and pests, diseases, and weed control.
The survey included seven different varieties, with Fedearroz 733 (F733) being the most common in irrigated systems and overall in the country and F473 being the most used in upland systems, but also to some extent in irrigated systems (Fig. 2). Other varieties such as F2000, F174 and F60 are less often used, although some of them (particularly F2000) have been commercially released only recently. We find that most terms associated with varietal choices were also negatively associated with yields. Our models indicate that F2000 and F473 have no significant difference from the base category (F174), although F473 tends to yield less and F2000 tends to yield slightly more than F174 (Fig. 3B). The other varieties (F733, Fortaleza and IA550) all show statistically significant and negative effects on yields (-0.23, -0.27, and -0.18, respectively, all at P< 0.01). Since coefficients associated with these varieties were the largest in magnitude across all variables, we conclude that varietal choice is the most important management factor in upland rice systems, with optimal choices being F174 and F2000.
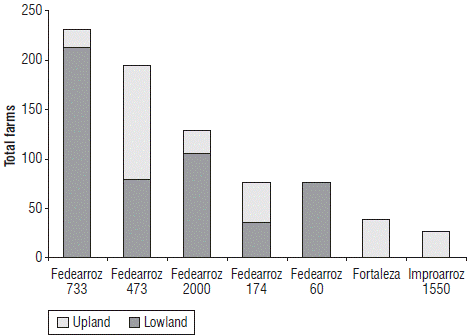
FIGURE 2 Frequency of use of different rice varieties for both rice production systems, as derived from the database used in this study. Data from Colombia during the period 2007-2015.
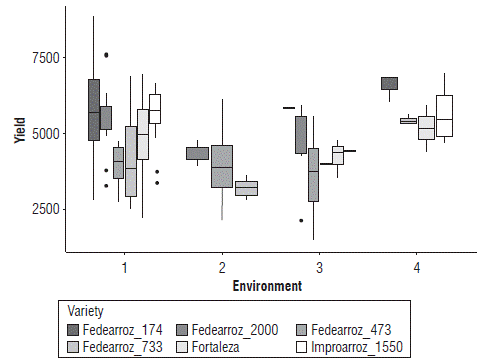
FIGURE 3 Effects of management variables and environmental groups on rice yields for upland rice systems. (A) Model coefficients and (B) yield of all rice varieties for each environment. Horizontal lines show the median, boxes show the interquartile range, whiskers extend to 5-95% of the data, and dots are outliers. Data from Colombia during the period 2007-2015.
The rest of the variables showed no significant impact, but this does not necessarily mean that they do not have impact under specific conditions or farms. For instance, pesticide doses are not important if the variety used is pest-resistant, but it would be important if the selected variety were sensitive to pest attacks. High noise in the recorded data or low variability across farms could also have precluded the identification of a relevant variable for the yield response. However, for this farm population there are no statistically significant responses for these variables.
Finally, we estimated technical efficiencies for each farmer and found an average technical efficiency level of 77% across all upland rice farms. Despite this relatively high value, we observed some farmers with relatively low efficiencies (below 60%), particularly in the municipalities of Villavicencio, Cumaral, Puerto Lopez and Fuente de Oro, all of which are located in eastern Colombia (Fig. 4).
Irrigated rice
Relevant variables in irrigated systems are different to those in upland systems. This is confirmed by developing system-specific models (Tab. 4). The phosphorous application rate was amongst the most important variables for irrigated rice (coefficient of 0.04). Its positive effect on yield as well as the fact that it had a smaller effect than the nitrogen application rate is in broad agreement with theory (Longs-treth and Nobel, 1980; Fujisaka et al., 1994). The number of fertilizer applications was also found to be statistically significant, suggesting that a larger number of better temporally distributed yet lower-dosage applications ensures such applications are more efficiently used by the crop in contrast to a reduced number of higher-rate applications.
TABLE 4 Stochastic Frontier Analysis coefficients estimate for irrigated rice system. Data from Colombia during the period 2007-2015.
Variable | Coefficients | Standard Error | Z Statistics | P Value |
---|---|---|---|---|
(Intercept) | 9.14 | 0.55 | 16.66 | 0.00*** |
log(nitrogen) | 0.05 | 0.04 | 1.18 | 0.24 |
log(phosphorous) | 0.04 | 0.01 | 3.18 | 0.00*** |
log(quantity of seeds) | 0.03 | 0.04 | 0.63 | 0.53 |
log(crop area) | -0.01 | 0.01 | -1.33 | 0.18 |
log(fertilizer use rate) | 0.07 | 0.06 | 1.18 | 0.24 |
log(fertilizer aplication) | 0.07 | 0.04 | 1.66 | 0.09* |
log(pesticide use rate) | -0.02 | 0.03 | -0.60 | 0.55 |
log(pestiside aplication) | -0.04 | 0.03 | -1.23 | 0.22 |
log(access) | -0.12 | 0.03 | -3.56 | 0.00*** |
log(man hours of labour) | 0.02 | 0.04 | 0.41 | 0.68 |
log(machine hours of labour) | 0.00 | 0.02 | 0.11 | 0.91 |
Variety Fedearroz2000 | -0.06 | 0.05 | -1.13 | 0.26 |
Variety Fedearroz2000473 | 0.10 | 0.06 | 1.63 | 0.10* |
Variety Fedearroz200060 | -0.02 | 0.06 | -0.31 | 0.76 |
Variety Fedearroz2000733 | -0.06 | 0.05 | -1.09 | 0.28 |
Environment 2 | 0.08 | 0.04 | 2.23 | 0.03** |
Environment 3 | 0.15 | 0.03 | 4.57 | 0.00*** |
0.11 | 0.01 | 8.23 | 0.00*** | |
0.97 | 0.02 | 50.14 | 0.00*** |
*** Significant at 1% ** Significant at 5% * Significant at 10%
Access to farms, calculated as the distance from each farm to primary, secondary and tertiary roads was found to affect yields significantly and negatively (coefficient of -0.12). This negative effect indicates that farms that are further away from roads have lower yields. Large distances from farm to roads can limit the timely access of fertilizers and machinery. This could negatively impact farm activity schedules and cause fertilizer, pesticide, or herbicide applications, or machinery-related activities to happen at non-optimal times, thus reducing yields.
Finally, both environments and varieties had a significant impact on yield. In particular, we found that both EG2 and EG3 had a yield advantage as compared to EG1 and that the variety F473 had a significantly positive impact (coefficient of 0.1) compared to F174. The other varieties had negative albeit not statistically significant yield impacts (Fig. 5). Our analysis suggests that adequately selecting varieties per environment is critical to increasing yields in irrigated systems (Fig. 5B). The analyses generally highlight the importance of including site-specific variations in yield response models so as to allow the identification of both constraints and opportunities at the scales that are relevant for farmers and extension agents (Jiménez et al., 2009, 2011; Delerce et al., 2016).
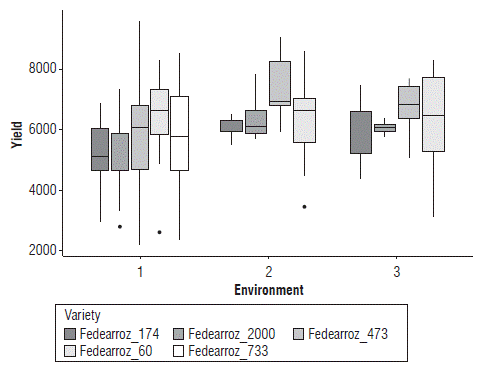
FIGURE 5 Effects of management variables and environmental groups on rice yields for irrigated rice systems. (A) Model coefficients; and (B) yield of all rice varieties for each environment. Horizontal lines show the median, boxes show the interquartile range, whiskers extend to 5-95% of the data, and dots are outliers. Data from Colombia during the period 2007-2015.
Technical efficiencies for irrigated rice are shown in Figure 6. Notably, the average technical efficiency was 78%, which is very similar to the average efficiency of the upland rice system. However, there are large variations across farmers and environments. Municipalities such as Lorica, La Jagua and Cucuta show low efficiency levels and hence large yield gaps (>40%) for some farmers, whereas the municipalities of Espinal, San Martin, Valledupar and Purificacion show consistently high efficiency levels across farmers. This is consistent with evidence that farmers in northern Colombia are less technologically developed (DANE, 2004).
Conclusions
The stochastic production frontier methodology is a useful alternative to more classical yield gap assessments based on detailed process-based modeling (Bhatia et al., 2008; van Bussel et al., 2015), and has two clear advantages. First, the method integrates microeconomic theory and empirical analysis, thus allowing the empirical validation of both biophysical and economic hypotheses regarding the cropping systems under study. And secondly, since the method is based on commercial farming information, it has the potential to provide recommendations that are tailored to the farms and farm systems analyzed. The use of georeferenced information at the farm level from a geographically widespread sample of rice farms allowed us to integrate soil and climate data. This not only helped to reduce noise in the responses to environment factors but it also enhanced the capabilities of the methodology beyond only identifying management factors, identifying management x environment interactions, and allowing a more comprehensive assessment of the rice crop systems.
For the upland rice system, our analysis suggests that increasing nitrogen fertilization and seed quantity in upland systems is needed for yield gap closure. Conversely, the amount of man labor hours was found to have a negative effect on productivity, which we attribute to non-optimal use of an excessively large number of man labor hours on harvesting activities. For irrigated systems, we identified the phosphorous application rate as well as the number of fertilizer applications as having a significant and positive effect on yield. For irrigated rice, we also report that accessibility is a limiting factor for a number of farms.
For both rice production systems, we identify two critical aspects that need to be well-managed so as to ensure high levels of production. Most critical is the correct matching of varieties to the prevailing climates of the farms. Our analysis indicates significantly different effects from varieties for the different environments for both production systems. For municipalities with low average efficiency levels, we suggest that improved technical assistance through extension services will be needed to ensure optimal management. For upland systems, we identified Villavicencio, Cumaral, Puerto Lopez and Fuente de Oro, whereas for irrigated systems it would be Lorica, La Jagua and Cucuta. Such technical assistance should be targeted at providing site-specific recommendations and could be based on both our results and the experience of the local extension agents and farmers. Finally, we note that, as this study is based on commercial farming practices and yields across all rice growing areas of Colombia, these recommendations have the potential to directly benefit rice farmers.