Introduction
It is clear that in developing countries agricultural growth is an instrument of economic growth and poverty reduction (Headey et al., 2005; Byerlee et al., 2009). Long-term agricultural growth has been associated with growth in yields, which is encouraged by investment in research, extension, human capital and infrastructure (Rosegrant and Hazell, 2000; Jayne et al., 2010). The importance of good infrastructure for agricultural development is recognized in its ability to enhance productivity and reduce transactional costs in agricultural marketing (Andersen and Shimokawa, 2007; Munyanyi, 2013; Khapayi and Celliers, 2016). The three types of agricultural infrastructure are road networks, irrigation technology, and post-harvest storage technology (Munyanyi, 2013). Ghosal (2014) provides two categories of infrastructure services in agriculture: soft and hard infrastructure. 'Soft infrastructure' includes transportation services, financial services, animal husbandry, input distribution, and marketing; 'hard infrastructure' includes roads, telecommunications, electrification, and irrigation (Ghosal, 2014). Infrastructure could also be disaggregated as physical (for example transport, storage, and irrigation infrastructure), equipment (tractors, ploughs, and sprayers), institutional (cooperatives and financial institutions), and social infrastructure-consisting of health and education services (Andersen and Shimokawa, 2007).
According to Stilwell and Makhura (2004), infrastructure can also be classified as either economic or physical (for example, roads, storage, bridges, and railways), social or soft (e.g. health and education), or institutional (farmers' cooperatives and agricultural institutions). Economic infrastructure is that component of an economy's capital stock that generates services for enabling economic production or for assisting as input in production (Stilwell and Makhura, 2004). It is important to note that institutional and social infrastructures are equally important as they both play a key role in an economy. In agriculture, institutional infrastructure such as cooperatives, lending, and marketing institutions play a significant role in linking farmers to the markets and thus increasing productivity (Kumar et al., 2015). Social infrastructure impacts the quality of life directly and indirectly since it is the principal foundation that provides services in agriculture, health, education, and recreation (Stilwell and Makhura, 2004). Equipment infrastructure entails all the farm level machinery and small equipment needed to improve labor productivity including tractors, ploughs, and sprayers (Andersen and Shimokawa, 2007; Munyanyi, 2013). Therefore, agricultural infrastructure entails all the basic services, facilities, institutions and equipment that are needed for efficient production and marketing of agricultural commodities (Munyanyi, 2013). This definition was adopted for this study.
Infrastructure has a huge impact on economic growth. According to the Development Bank of Southern Africa (DBSA, 1997), it is important to invest sufficiently in infrastructure as it allows diversification of trade and the country benefits more from globalization. Governments and donors invest heavily in the development of rural roads and transport corridors because investments in rural infrastructure have an important positive effect on agricultural production and trade (Tripathi and Prasad, 2009; Jouanjean, 2013; Manjunath and Kannan, 2017). Adequate and reliable infrastructure provides the physical linkages between different inputs needed for the economy to be functional (Cloete, 2010).
The South African Government introduced the Land Reform to ensure restitution, redistributions and tenure reform of land especially for the previously disadvantaged black South African smallholder farmers from 2002 (Antwi and Oladele, 2013). In order to expedite the development of such farmers into commercialized farmers, the government is investing in infrastructure for smallholder farmers in the North West Province, through programs such as the Comprehensive Agriculture Support Programme (CASP) (DAFF, 2017). Farmers who have received governmental support through the Land Reform and CASP are termed emerging farmers, and they will be referred to as such throughout this paper. The CASP identifies five types of infrastructure as follows: resource management infrastructure, production infrastructure, mechanization (tractors), water storage infrastructure for household food, and marketing infrastructure. This program has continued to fund smallholder farmers since 2005 in terms of agricultural infrastructure and support initiatives for farmers (DAFF, 2017).
There is little formal evidence to show how and under what conditions such infrastructure benefits rural smallholder farming households and improves agricultural income (Jouanjean, 2013). This study used the physical institutional, social, and equipment infrastructure availability and accessibility indices with their components already mentioned to analyze the effect of infrastructure on agricultural income. According to Idiong (2007), other socioeconomic factors such as age, educational level of household head, and access to extension were also included in the analysis as they were expected to have a direct influence on efficiency. Therefore, the objective of the study was to examine whether accessibility and availability of agricultural infrastructure enhance farmers' agricultural income through efficiency gains.
Materials and methods
Data sources and analyses
A structured questionnaire subject to reliability and validity tests was employed to collect data through a survey of150 smallholder farmers in the North-West Province (NWP). The NWP covers an estimated surface area of 116,320 km2, with a population density of approximately 30 people per km2 (Statistics South Africa, 2012). The Province is located in the western part of South Africa (26.6639° S, 25.2838° E), sharing borders with Limpopo Province to the north, Gauteng to the east, the Free State to the east and south, the Northern Cape to the south, and Botswana to the west and north (DAFF, 2017). The NWP was selected as it is mostly rural and agricultural as the main contributor to the provincial gross domestic product (DAFF, 2017).
Data was collected from March to July in 2016 and questionnaires were administered through face to face interviews. The research used stratified random sampling to group farmers receiving infrastructure support and those who did not receive support from the government. Under the CASP of the South African Government, emerging farmers received financial support in form of subsidies to purchase farm infrastructure. However, not all intended beneficiaries of the program received the financial support and the infrastructure (DAFF, 2017). Therefore, the population consisted of 114 smallholder farmers receiving infrastructural support and 72 without support (DAFF, 2017). Using the Raosoft sample size calculator, the sample frame consisted of 89 farmers with infrastructure support and 69 without support. Farmers from each stratum were randomly selected to come up with a total of 150 farmers.
Captured data from completed questionnaires on STATA version 14.0 were analyzed using descriptive statistics and the Stochastic Frontier Model (Battese and Coelli, 1995). The study used the model to assess factors that influence smallholder farmers' agricultural income with respect to availability and accessibility to agricultural infrastructure. Furthermore, factors that influence the agricultural income of the smallholder farmers without access and agricultural infrastructure were analyzed.
Principal component analysis (PCA) was used to compute composite indices of availability and accessibility of infrastructure in terms of physical, institutional, social and equipment infrastructure. The selection of indicators was guided by insights drawn from the literature on agricultural infrastructure (Munyanyi, 2013; Stilwell and Makhura, 2014) as well as data availability. All the major dimensions of agricultural infrastructure were represented by at least one indicator. PCA is a data reduction method used to re-express multivariate data in fewer dimensions. The procedure transforms selected indicators into smaller components that capture most of the information (variation) in the original indicators. A detailed account of the use of PCA for constructing socio-economic status indices has been outlined by Vyas and Kumaranayake (2006). The variables selected for constructing the indices were the agricultural infrastructure categories stated in the questionnaire, which were coded as 1 if the answer was yes and 0 if it was no. These major infrastructure groups were in accordance with the literature on agricultural infrastructure in South Africa, (physical, social, institutional and equipment infrastructure). STATA software was used to provide a simple measure of the aggregation of the agricultural infrastructure indices and PCA, after which the same STATA command was used to predict the availability index, the accessibility index and the satisfaction index used in the study.
A stochastic frontier model for agricultural income for farmers with agricultural infrastructure (availability)
This study follows the modern economic theory based on the assumption of optimizing behavior for producers both technically and economically (Kokkinou, 2010). Agricultural infrastructure and advanced agricultural production methods are synonymous with farmers who can produce efficiently and increase agricultural income. Parametric or non-parametric methods can be used to determine efficiency.
The stochastic frontier production function proposed for this study is a parametric model that makes use of econometric methods to analyze agricultural income for smallholder farmers (Masunda and Chiweshe, 2015). The model was selected due to its extensive use in productivity estimation for smallholder agriculture in previous studies (Binam et al., 2004; Mango et al., 2015; Abdul-Hanan and Abdul-Rahaman, 2017). The Stochastic Frontier Model was originally proposed for panel data analysis by Battese and Coelli (1995). However, the model was adopted for cross sectional data in which the parameters of agricultural income (production function) were specified to be a function of variables associated with a socio-economic variable, such as access to extension services, land tenure, number of years involved in farming, etc. (Mango et al., 2015). The different factors of production involved may affect the responsiveness of the produce (agricultural income). The models are presented in terms of production functions in which the former can only allow a constant return to scale and the latter has more flexibility. As adopted from Abdul-Hanan and Abdul-Rahaman (2017), two functional forms for the stochastic frontier production function that were estimated are described by:
where: Log represents the logarithm base 10; the subscript i represents the i th sample of smallholder farmers; the subscript j represents the number of socio-economic and farm-specific variables;
Y represents the seasonal agricultural income by smallholder farmers in Rands;
X 1 represents the physical infrastructure availability index on the i th farm;
X 2 represents the social infrastructure availability index on the ith farm;
X3 represents the institutional infrastructure availability index on the ith farm;
X 4 represents the equipment infrastructure availability index on the ith farm;
X 5 represents the farmer's level of education on the ith farm;
X6 represents the extension access on the ith farm;
X 7 represents the non-farming activities on the ith farm;
X 8 represents the farmer's membership in an organization on the ith farm;
X9 represents the age of the farmer on the ith farm;
X 10 represents the marital status of the farmer on the ith farm;
X11 represents the land tenure on the ith farm;
X 12 represents the farmer's number of years involved in farming on the ith farm;
X 13 represents assistance of the household member n farming on the ith farm;
βj j = 0, 1, 13 are parameters to be estimated;
Vi's are assumed to be independent and identically distributed N (0, σ2) random variables; and
Ui's are assumed to be independent and identically distributed non-negative truncations of the N (μ, σ 2) distribution.
These models were separately considered for two categories: availability of agricultural infrastructure and non-availability of agricultural infrastructure. In the first case of farmers with the availability of agricultural infrastructure, factors affecting agricultural income were analyzed to understand the efficiency of farmers with agricultural infrastructure on agricultural income. In the latter case, farmers without agricultural infrastructure were taken into consideration. The above models (1 and 2) are production functions, in which the inefficiency effect is subtracted because observed outputs are no larger than their corresponding stochastic frontier, due to the presence of inefficient use in producing the involved outputs. The non-negative random variables Ui in Equation 1 imply that the observed socio-economic (input) variables for a given level of output and quasi-fixed inputs are not as small as possible if the farmers were fully efficient in their use of inputs. Following Abdul-Hanan and Abdul-Rahaman (2017), the translog production function was used in this study. This is specified in Equation 2, so that more general technologies could be accounted for than with the Cobb-Douglas model.
The test of the hypothesis for the parameters of the frontier model was conducted using the generalized likelihood-ratio statistics, λ, defined by:
where L(H 0 ) is the value of the likelihood function for the frontier model in which parameter restrictions are specified by the null hypothesis; H 0 is imposed, and L(H 1 ) is the value of the likelihood function for the general frontier model (Battese and Coelli (1995). If the null hypothesis is true, then λ has approximately a chi-square (or mixed square) distribution with degrees of freedom equal to the difference between the parameters estimated under H 1 and H 0 , respectively.
Stochastic frontier model for agricultural income for farmers with access to agricultural infrastructure
Similarly, two separate models were used for the agricultural farmers with and without access to agricultural infrastructure. The dependent variable was agricultural income and the independent variables used were the same as the ones used for farmers with available infrastructure. However, the infrastructural accessibility indices were used instead of the availability indices.
Results and discussion
Descriptive statistics for the demographic and socio-economic characteristics of emerging farmers
The socio-economic and demographic characteristics of emerging farmers in the North West Province are presented in Table 1. According to Stilwell and Makhura (2004), these characteristics are important because the key household activities are coordinated by the head of the household and decisions of the head are most likely influenced by such demographic characteristics. The results show that the majority (65%) of farmers were males, 61% were married, and 57% had no formal education. The analysis above reveals that participation of women in smallholder farming still remains a challenge in the North West Province. This could be attributed to the fact that most households sampled for the study were made up of more males than females. This finding is consistent with those of Antwi and Oladele (2013) who found that agriculture is mostly reserved for males while women are expected to perform domestic activities in the household. The majority of smallholder farmers in the North West Province are aged between 41 and 60 years, an indication of a paucity of involvement of the youth in smallholder farming agricultural activities in the Province. Smallholder farmers do not have a strong educational background and most of them have no formal education; this could limit their adaptation to new farming agricultural innovations and agricultural infrastructure. This finding is consistent with the results obtained by Montshwe (2006), who found that people with higher levels of education are able to better interpret information. This revelation could be linked to labor costs that have risen in previous years and to the fact that children are being statutorily compelled to be at school during the day. This is also in line with the finding of Harding et al. (2005), who highlighted insufficient family labor as a production constraint of smallholder farming. There is a low number (26%) of people considered as members of farmer groups in the area, posing a threat to organized lending opportunities for farmers.
Factors affecting agricultural income in relation to availability and accessibility of infrastructure following the stochastic models
The results of the stochastic frontier models on factors affecting agricultural income with regards to availability and accessibility of infrastructure are presented in Tables 2 and 3, respectively. The models are appropriate given their significant chi-square (P<0.01) and Log likelihood ratios (for both models in Tabs. 2 and 3).
TABLE 2 Stochastic frontier for factors affecting agricultural income in relation to availability of agricultural infrastructure.
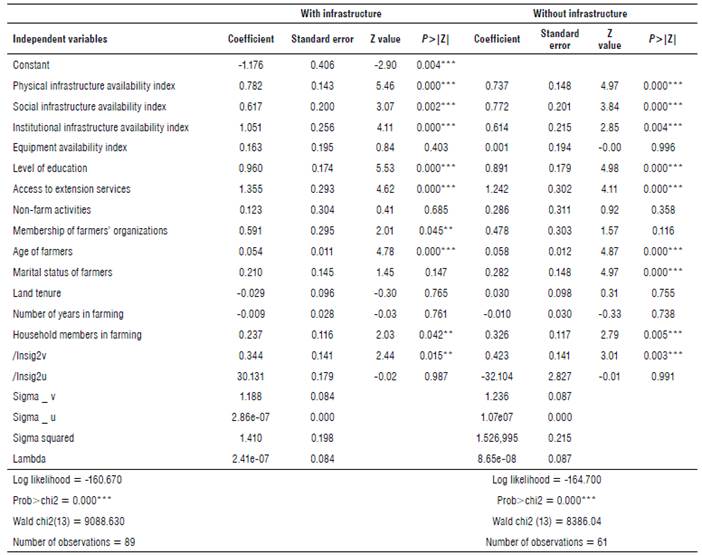
***, ** and * represent significance at 1%, 5% and 10% level, respectively.
Physical infrastructure availability index
The results in Table 2 show that the relationship between the physical infrastructure availability index and agricultural income is positive and statistically significant for farmers who have the available infrastructure and those without infrastructure (P<0.01). Physical infrastructure includes roads, railway lines, fences and irrigation that is infrastructure required for production and marketing of agricultural products. According to Fakayode et al. (2008), Llanto (2012) and Eke and Effiong (2016), provision of efficient infrastructure is widely acknowledged as indispensable to agricultural progress. Increased availability of physical infrastructure would assist farmers to produce efficiently and generate more agricultural income. However, some of the available infrastructure such as railway corridors have become obsolete due to lack of proper maintenance, as people opt for other models of transport. Where irrigation infrastructure is available, lack of government advisory services on the use and maintenance of the infrastructure results in farmers not optimally utilizing the available infrastructure (Khapayi and Celliers, 2016).
Social infrastructure availability index
The influence of the social infrastructure availability index on agricultural income was positive and statistically significant (P<0.01) for both groups of farmers. The positive relationship between the social infrastructure availability index and agricultural income could be attributed to the fact that social infrastructure such as culture, health, and education plays a critical role in terms of maintaining farmers' physical and mental wellbeing, thus, maximizing the labor productivity of the farmers (Nadeem et al., 2011; Kumar et al., 2015). Moreover, the positive relationship between the social infrastructure availability index and the agricultural income of farmers without available infrastructure could be attributed to the fact that farmers could access social infrastructure even though it is not available in their area (for example, health services through mobile clinics and education through distance learning) (Kumar et al., 2015). Social infrastructure plays a strategic role in producing large multiplier effects within the economy, thus, leading to agricultural growth (Bom and Ligthart, 2014). In South Africa, the standards of primary health care facilities are relatively poor and residents in rural areas usually forget their appointments or they miss them due to transportation constraints (Frost et al., 2017). Therefore, low levels of social infrastructure, such as primary health care in rural areas, jeopardize the health of farmers and in turn they impact labor productivity.
Institutional infrastructure availability index
This study revealed a positive and statistically significant relationship between the availability of infrastructure and agricultural income for both groups of farmers (P<0.01). The institutional infrastructure availability index is associated with infrastructure such as financial institutions, farmers' unions, cooperative societies, and agricultural markets. Availability of this infrastructure is most likely to reduce transactional costs of marketing, thus increasing agricultural income. According to Satish (2006), rural infrastructure in the form of farmers' access to markets and availability of institutional finance leads to agricultural expansion and increased technical efficiency. However, in South Africa the collapse of the development corporations in former homelands and the increasing inability of the government to provide agricultural support services have limited productivity of smallholder farmers (Willemse, 2000).
Level of education of smallholder farmers
The study revealed that as the level of education increases, agricultural income was likely to increase significantly for both groups of farmers (P<0.01). This was expected as the higher the farmer's level of educational, the better the agricultural practices of farmers, thus, leading to higher agricultural income for smallholder farmers (farmers with and without agricultural infrastructure). According to Ferreira (2015), educated farmers are more likely to adopt new technologies, and this, in turn, leads to the diffusion of technology to other less educated farmers within the community. The descriptive statistics show that most (57%) of the farmers had no formal education despite its importance in sustainable agriculture.
Access to agricultural extension services
Access to extension services for farmers with available agricultural infrastructure and those without it was found to be statistically significant and positively affecting agricultural income (P<0.01). This implies that any relative increase in access to extension services caused agricultural income to respond positively. Farmers' access to extension services plays a very critical role in the production activities of smallholder farmers, and extension officers are often the ones who share information with smallholder famers on programs to finance infrastructure (Anderson and Masters, 2007).
Membership of farmers' organizations
The study revealed a positive and statistically significant relationship between agricultural income and membership in farmers' organizations (P<0.05) for farmers with infrastructure. The results highlight the importance of gras-sroot farmers' organizations such as Grain South Africa (Grain-SA) and Agri South Africa (Agri-SA). Grain South Africa is a voluntary organization for grain producers in South Africa that allows sharing information on various commodity markets and the adoption of technology. Agri South Africa is a coalition of all smallholder farmers groups with the overall aim of improving farmers' access to information, finance, and markets for sustainable agricultural production. Farmers' organizations provide the platform for smallholder farmers to discuss issues for increasing the availability of infrastructure and engaging governments to fund projects on infrastructure development (DAFF, 2017). Similarly, Kumar et al. (2015) emphasized the role of cooperatives in ensuring efficient delivery of financial support in smallholder farmers.
Age of smallholder farmers
The study revealed a positive statistically significant increase in agricultural income in relation to age for both groups of farmers (P<0.01). Age can be used as a proxy for experience, assuming that farmers are able to utilize infrastructure efficiently and have a better understanding of production practices with experience (Mango et al., 2015). In this study, the small number of young people involved in agriculture is a cause for concern as this jeopardizes the future of smallholder agriculture.
Marital status of emerging farmers in the study area
A positive and statistically significant relationship was observed between marital status and agricultural income (P<0.01) for farmers without infrastructure. This could be attributed to the fact that when one gets married, one's assets may increase and his or her partner could bring some income into the house. Such income could be used to procure more inputs, which could be used in the production activities of farmers, thus increasing agricultural income, even without available infrastructure.
Household members in farming activities
A positive and statistically significant relationship was observed between agricultural income and assistance for household members in farming for farmers with infrastructure (P<0.05) and farmers without infrastructure (P<0.01). The assistance of household members in farming enterprises is very critical for the productivity of smallholder farmers. Labor from household members is relatively affordable since in most cases household members have shares in the business and strive to make them as productive and efficient as possible. This is in line with the findings of Adepoju and Salman (2013), who asserted that household labor is statistically significant and positively influences productivity.
Physical infrastructure access index
The results in Table 3 show that access to physical infrastructure in relation to agricultural income is likely to cause a positive and statistically significant increase in agricultural income for both groups of farmers (P<0.01). This implies that any relative change in access to physical infrastructure in the study area caused agricultural income to respond positively. This is an indication that as farmers in the study area increase their access to physical infrastructure such as roads, fencing and irrigation equipment, they become technically efficient in their agricultural production, thus increasing their income. As stated by Fakayode et al. (2008), access is key to the efficient use of infrastructure; for instance, if there are communal grain silos in the area but the road networks are poor, farmers would not be able to access them, thus resulting in post-harvest losses.
Social infrastructure access index
Regarding access to social infrastructure in relation to agricultural income for farmers with access to infrastructure, any relative change in access to social infrastructure within the study area caused agricultural income to respond positively (P<0.1). This shows that with increased access to recreation, health and educational facilities for smallholder farmers in the study, farmers are likely to be healthier and make economically rational farming decisions, thus, improving their labor productivity. Kumar et al. (2015) asserted that, for social infrastructure, it is the accessibility of the services rather than their availability that is more important.
Equipment infrastructure access index
A positive and statistically significant relationship was observed between agricultural income and access to equipment infrastructure for farmers with (P<0.01) and those without (P<0.05) infrastructure. The reason for the increase in agricultural income is because agricultural equipment constitutes very important input in a farming enterprise. Farmers with access to inputs are likely to produce sufficiently and are able to harvest efficiently and yield more output, thus contributing positively to their farm income.
Level of education of smallholder farmers
Education had a positive significant influence on the agricultural income of smallholder farmers for both groups (P<0.01). As the level of education of smallholder farmers with access to infrastructure increased, the agricultural income of selected farmers also increased. This is due to the fact that educated farmers easily adapt to innovation and understand the fundamentals of production easier, thus implying that educated farmers were able to better utilize infrastructure that is made available to them. With better utilization of infrastructure, farmers in the study area were able to increase their agricultural income. In a similar study, Oduro-Ofori et al. (2014) indicated that a formal secondary education level was adequate for famers to comprehend technology used in agriculture, and extension education had more returns to agricultural productivity in Ghana. This result acknowledges the importance of education for the conception of the principles of basic production.
Access to agricultural extension services
The relationship between access to extension services and agricultural income was positive and statistically significant for both groups of farmers (P<0.01). Farmers with access to extension services received services such as technical support and training from extension officers. These services could assist farmers to expand their production activities, thus contributing to an increase in farm income. To deal with these challenges and to foster agricultural growth, especially in rural areas, agricultural advisory services (AASs) are brought to the center of today's international development discourse (Birner et al., 2009).
Membership of farmers' organizations
The results in Table 2 show a positive statistically significant relationship between agricultural income and membership in farmers' organizations with (P<0.05) and without infrastructure (P<0.01). Farmers who are members of farmers' organizations are able to share information on the utilization of agricultural infrastructure. Organizational training could be arranged to train farmers on the use of infrastructure. This finding concurs with that of Adepoju and Salman (2013) who found that membership in farmers' cooperatives also significantly increased agricultural productivity in a study conducted in Nigeria. Furthermore, Akankwasa et al. (2015) found that farmers who participated more in community-based organizations were likely to engage in social learning about technology, hence raising their likelihood to adopt the technology.
Age of smallholder farmers
This study revealed a positive statistically significant relationship between agricultural income and age for both groups of farmers (P<0.01). This could be attributed to the fact that as farmers become older, the greater their experience and exposure to the utilization of infrastructure, the more this contributes to the efficient utilization of infrastructure by farmers. The positive reaction of agricultural income to farmers without infrastructure is due to the fact that as farmers' age increases, they become more knowledgeable and gain skills in farming efficiently. The experience of farmers also contributes to efficient farming using indigenous knowledge even without sufficient access to infrastructure. Although Saiyut et al. (2018) obtained similar results in Thailand where farmers aged 15-19 were inefficient as compared to farmers over 60, farmers who have aged and subsequently become less productive, should also be considered.
The assistance of household members in farming activities
The study revealed that agricultural income was positively associated with a change in the number of household members assisting the farming enterprises for both groups of farmers (P<0.01). Barro (2001) stated that labor is a major factor of production in traditional farming systems and as such, laborers are key elements in increasing productivity. When household members assist in farming activities, their experience is retained on the farm, thereby increasing labor productivity which ultimately increases technical efficiency of farmers and agricultural income in the long run.
Conclusion
The Government of South Africa has rolled out different agricultural developmental programs including the Comprehensive Agricultural Support that enabled access to infrastructure for smallholder farmers so that they progress towards commercialization. There has been a lack of farm level information on how availability and accessibility of infrastructure affect the productivity of farmers. Therefore, this research was aimed at assessing the effects of availability and accessibility of agricultural infrastructure on emerging farmers' incomes. Stratified sampling was used to identify 89 farmers with infrastructure and 61 without infrastructure in the North West Province of South Africa. Data were collected using semi-structured questionnaires administered through face to face interviews and using a stochastic frontier model for data analysis.
It can be concluded that variables that have a greater influence on agricultural income regarding infrastructure availability include: physical infrastructure availability index, social infrastructure availability index, institutional infrastructure availability index, level of education, access to extension services, membership of farming organizations, age of farmers, and assistance of household members in farming enterprises. Regarding infrastructure accessibility, the variables that statistically significantly influenced agricultural income positively are physical infrastructure accessibility index, social infrastructure accessibility index, equipment accessibility index, level of education, access to extension services, and membership to farming organizations, age and number of household members in farming enterprises. The results show that infrastructure plays a critical role in assisting smallholder farmers to produce more efficiently, thereby improving agricultural income. The majority of smallholder farmers were over 35 years of age, indicating low participation of young people in farming. This is currently the trend for most areas in South Africa, and in most agricultural enterprises. There is a need for government to prioritize programs that will increase the participation of the youth in agricultural activities, particularly in commercial farming since younger farmers may find it easier to utilize infrastructure efficiently and optimally. There is a need to promote greater membership in farmers' organizations among smallholder farmers in the study area. This would assist in sharing information by smallholder farmers in the study area. Farmers who are part of a farmers' organization could also come together to lobby the government, development agencies, and commercial funders to assist with infrastructure funding.