Introduction
The Andean blueberry (Vaccinium meridionale Swartz) (Ericaceae) is a woody shrub that grows spontaneously in the mountainous areas of Colombia, Ecuador, Peru, Venezuela, and Jamaica (Muñoz et al., 2009; Torres et al., 2009; Maldonado Celis et al., 2017). The Andean blueberry plants have a high domestication potential for fruit consumption in fresh and industrial processing due to the fruit's antioxidant activity and high content of phenolic compounds including anthocyanins, which can exceed those found in commercial species such as blueberries (Bernal et al., 2014; Maldonado Celis et al., 2017; Garzón et al., 2020).
The nursery material corresponds to plants cultivated for subsequent transplanting to the field (Singh et al., 2017); this is decisive in the quality and productivity of crops (Sánchez-Aguilar et al., 2016), so multiple studies focus on this phase. In Andean blueberry propagated from seeds, the nursery stage of growth ends after approximately 26 months (Torres et al., 2012). For asexually propagated Andean blueberry, few reports indicate the duration of the nursery period. According to González et al. (2018), a duration of 5 months is an adequate period for a rooted cutting of Andean blueberry to reach a size of around 20 cm in height and be suitable for transplanting to the field. Medina-Cano et al. (2015) report a period between 15 and 18 months from preparing the cuttings to their transplanting to the final site. Due to the taxonomic relationship of Andean blueberry with blueberry (V. corymbosum), reports for early growth of blueberry may be adjusted to Andean blueberry. Generally, in blueberries propagated from cuttings or by tissue culture, the nursery stage lasts from 18 to 24 months; after this period the plants are considered ready to be transplanted to the final site (Bryla & Machado, 2011; Bryla & Strik, 2015). In young plants of Andean blueberry, initial growth is characterized by extensive leaf formation. Vegetative growth progresses through formation and differentiation of axillar buds, followed by expansion and development of leaf blades. Finally, young leafy shoots are developed, characterized by the presence of leaves, prominent elongation of branches and leaf expansion, and formation of new axillary buds that can be differentiated into vegetative or reproductive organs (Medina-Cano et al., 2019).
The leaf area (LA) quantifies the total leaf surface that a plant possesses; this variable is of high importance in research in biology, plant physiology, agronomy, and ecology (Cabezas-Gutiérrez et al., 2009; Weraduwage et al., 2015). The LA is used as a response or predictor variable in processes such as light interception for photosynthesis and transpiration for efficient water use, and as an indicator of growth and productivity (Kucharik et al., 1998; Montoya-Restrepo et al., 2017). At the plant community level, LA is the basis for estimating light interception indicators, such as the leaf area index (LAI) used in function-adjusted growth analysis (Cabezas-Gutiérrez et al., 2009; Benedetto & Tognetti, 2016; Hunt, 2017).
The methods to estimate LA in plants can be classified into direct and indirect groups (Yan et al., 2019). The direct or destructive methods limit the possibility of measuring the same individual as a function of time in growth studies and require intensive labor, as they involve removing the leaves of a plant to measure them with different techniques. Direct LA measurement can be done with LI-3000C portable leaf area meter (LI-COR, Lincoln, NE, USA) or the Portable Laser Leaf Area Meter (CID Bio-Science, Camas, WA, USA). The use of these meters is limited because of their cost. As an alternative, the use of images is a strategy that allows estimating values from the use of image processing software such as ImageJ (Rasband, 1997) used in various studies (Schneider et al., 2012; Easlon & Bloom, 2014; Milic et al., 2018; Suarez Salazar et al., 2018; Cosmulescu et al., 2020; Wakui & Kudo, 2021), Adobe Photoshop (Adobe Systems Incorporated, 1990), and AutoCAD (Autodesk, 1982), which are based on the use of a scale and the manual segmentation of images to differentiate the background of leaves.
The LA estimation is useful when the number of experimental units or observations is small or the same experimental unit is monitored, such as in perennial or timber crops, or where direct measurement of LA is costly (Montoya et al., 2017). Indirect methods obtain an approximation to LA from mathematical models based on non-destructive morphometric measurements and indicators of growth including: i) the ratio of length and width of leaves, number of leaves, and combination of these variables (NeSmith, 1991; Igathinathane et al., 2006; Fallovo et al., 2008; Spann & Heerema, 2010; Celik et al., 2011; Cabezas-Gutiérrez & Peña-Baracaldo, 2012; Suárez Salazar et al., 2018; León-Burgos et al., 2022), and ii) models or relationships with other variables such as leaf dry weight (Akram-Ghaderi & Soltani, 2007; Huang et al., 2019) among other variables. One of the limitations in using mathematical models is their specificity at the level ofvariety, cultivar, phenological stages (Pire & Venezuela, 1995) and stratum in the canopy (Brito et al., 2007). This generally requires direct methodologies for the model validation (Yan et al., 2019), ignoring, in some cases, the use of other growth variables related to LA that could increase the precision of the LA estimation.
The Andean blueberry has small, simple, alternate, coriaceous, lanceolate or oblong-lanceolate leaves, with a serrated margin, cuneate leaf base and acute apex (Ehlenfeldt et al., 2022). In blueberries, structural traits of leaves and stems can correlate with yield parameters (Barai et al., 2022), while, for the Andean blueberry, no studies have been carried out in this regard. Additionally, leaves can be one of the by-products of the Andean blueberry cultivation (Zapata-Vahos et al., 2015) since they have a high content of polyphenols and other substances of nutraceutical and medicinal importance (Borda-Yepes et al., 2019). Direct measurement of LA in Andean blueberry plants is difficult due to the high number of leaves and their small size (Ehlenfeldt et al., 2022), resulting in a slow and meticulous procedure. In other Vaccinium species (V corymbosum, V. virgatum, and others), models have been developed for the measurement of leaf area. However, these species are deciduous, while the Andean blueberry is an evergreen perennial plant; therefore, it is important to develop specific models for the Andean blueberry that allow a better estimation of this variable given the difficulty of direct LA measurement and the scarcity of alternative methods for estimating its LA. The purpose of this research was to establish five models to estimate LA in young Andean blueberry plants. These models employed various growth variables and used experimental and statistical validation.
Materials and methods
Plant material and experiment establishment
The experiment was carried out between 2019 and 2021 under greenhouse conditions at the Faculty of Agricultural Sciences of the Universidad Nacional de Colombia, Bogotá, at an altitude of 2,550 m a.s.l., with average air temperature 18.6±10°C, average relative humidity 66±26% (ELMA DT-171 datalogger, Elma instruments, Ryttermarken, Denmark). In total, 436 Andean blueberry plants propagated asexually were used in the vegetative stage of growth, with ages from 10 to 26 months (Tab. 1). The leaf area (LA) of 54,492 leaves was measured. The first group of plants was obtained by rooting of apical cuttings of wild plants from the municipalities of Guachetá, Cundinamarca (2800 m a.s.l., 5°2671.8" N and 73°42'09.2" W) and San Miguel de Sema, Boyacá (2572 m a.s.l., 5°36'09.3" N and 73°45'23.8" W); rooted cuttings were planted in 2.0 L plastic pots with a substrate (3:1:0.3 v/v/v): sand + perlite + Klasmann® peat without nutrients. The second and third groups were plants propagated in vitro by proliferation of axillary buds in a commercial nursery in the municipality of Rionegro, Antioquia (24°C, 80% relative humidity, 2800 m a.s.l.); the plants were planted in 2.6 L plastic pots with sand + Klasmann® peat without nutrients (5:1 v/v). Phytosanitary management was carried out preventively, with monthly application of a garlic-chili based pest repellent (70 g L-1) (Alisín® - Sáfer Agrobioló gicos S.A.S), and a chlorothalonil-based protective fungicide (720 g L-1), both at a dosage of 1 ml L-1.
Nitrogen and phosphorous fertilizations were carried out with doses between 0 to 2.7 and 0 to 1.3 g/plant per 5 months, respectively. The range of fertilization with N and P varied because these plants were the object of another fertilization trial with these mineral nutrients. The rest of the fertilization plan was carried out in the same proportion for all plants (1.25 K2O, 0.73 CaO, 1.08 MgO g/plant per 5 months and 18.15 Fe, 12.45 Mn, 6.21 H3B2O3, 0.70 Zn, and 0.70 Cu mg/plant per 5 months). The total fertilizer dose was divided into 4 applications realized at 3, 8, 12, and 16 weeks after transplanting the plants into plastic pots. Fertilizer sources were: urea (CO(NH2)2), calcium nitrate (Ca(NO3)2), di-ammonium phosphate ((NH4)2HPO4), triple super phosphate (Ca(H2PO4)2xH2O, potassium sulfate (K2SO4), calcium sulfate (CaSO4), magnesium sulfate heptahydrate (MgSO4x7H2O), iron sulfate (FeSO4), boric acid (H3BO3), zinc sulfate (ZnSO4), copper sulfate (CuSO4), and manganese sulfate (MnSO4). Fertilization doses were established in accordance with the research by González et al. (2018) and studies conducted on plants of the genus Vaccinium (Percival & Privé, 2002; Jeliazkova & Percival, 2003; Percival et al., 2003; Nestby et al., 2014; Maqbool et al., 2017) and a pre-assay of this research.
Evaluated variables
A descriptive analysis of the average leaf area (ALA) calculated from the total LA of each plant divided by the number of leaves was carried out to compare the groups of plants. Regressions were made from non-destructive variables such as height (H), measured from the base of the stem to the last apical vegetative shoot, number of vegetative shoots (NS), which, in the case of V. meridionale, are reddish in color due to the presence of anthocyanin pigments, and number of leaves (NL) of each plant (Medina-Cano et al., 2015; González et al., 2018). Similarly, regressions were performed for destructive variables such as dry weight per organ (DW) and LA, the latter as an explanatory variable.
To determine the total DW per plant, the plants were separated into roots, stems, and leaves and dried in a muffle oven at 75°C until constant weight. Subsequently, the following were determined: the root dry weight (RDW), leaf dry weight (LDW), stem dry weight (SDW) of plants propagated by cuttings, dry weight of the branches originating from stems in plants propagated in vitro (BSDW) and the total plant dry weight (TPDW) by adding the weight of all organs (Becerra et al., 2022). The leaf area (LA) of each plant was determined from digital image processing using the software ImageJ® v. 1.52 (Schneider et al., 2012). The photographs were taken with a mobile phone 25 MP camera at a height of 60 cm.
Statistical analysis
Statistical analysis was performed using the statistical software R (version 3.5.1.) and the respective libraries according to the statistical procedures described below. An exploratory analysis was carried out from the average LA of a single leaf to compare the groups of plants according to age. A descriptive analysis was performed from scatter diagrams to identify patterns in the relationships of variables. Pearson correlations were used to identify and quantify the linear relationship between variables with respect to LA. The plots were made using the "ggplot2" library (Wickham, 2016) and the correlation matrix was obtained with the "GGally" library extension (Schloerke et al., 2021).
From the variables with the highest correlation, various simple or multiple linear regression models were explored. In order to establish or propose a model that involves only non-destructive variables, models were tested with the predictor variables NL, H and NS, and LA as a response. Compliance with the regression assumptions was evaluated for all models. As morphometric variables and performance indicators are usually highly correlated, multicollinearity was evaluated from the variance inflation factor (VIF) with the "DescTools" library (Signorell, 2018). Similarly, linearity was studied using Pearson's correlation and residual normality using the Kolmogorov-Smirnov normality test, both from the "stats" library (R Core Team, 2022). Heterosce-dasticity was evaluated using the "Non-constant Variance Score Test" from the "car" library (Fox & Weisberg, 2019) and the presence of influential data was evaluated using the Cook distance criterion. In cases where some of the regression assumptions were not met, the weighted least squares technique was used (PennState, 2021). Finally, the models that met the assumptions were compared based on their predictions using the root mean square error (Root Mean Square Error, RMSE).
Results and discussion
Determination of the average leaf area (ALA) has been used to estimate the total leaf area in commercial blueberry plants and wild blueberries (Fallovo et al., 2008; Celik et al., 2011; Lancheros, 2012; Becerra et al., 2022) and perennial crops such as cherry (Prunus avium) and coffee (Coffea arabica) (Cittadini & Peri, 2006; Taugourdeau et al., 2014). For the consolidated database of the Andean blueberry, ALA varied between 0.03 cm2 (first quartile) and 1.85 cm2 (fourth quartile), with a median of 0.94 cm2 and a mean of 0.93 cm2 (Fig. 1). However, the mean ALA presented differences between the study groups, being 0.44 cm2 for group 1 in which all plants were propagated by cuttings, 0.92 cm2 for group 2 and 1.07 cm2 for group 3; plants in these last two groups were propagated in vitro.
Between the groups, differences were observed in the ALA related to the type of propagation (Fig. 1). The plants propagated by cuttings presented lower ALA (group 1: 0.45 ± 0.27 cm2) compared to plants propagated in vitro (group 2: 0.92 ± 0.26 cm2, group 3: 1.03 ± 0.22 cm2). The ALA for the group of plants propagated by cuttings coincides with the range of 0.1 to 0.3 cm2 described by Lancheros (2012) for plants propagated by seeds.
Variations in ALA were reported in wild and domesticated blueberries depending on the method of propagation, with tall and medium-tall blueberry plants obtained in vitro growing faster and having a greater number of stems, more lateral branches, and a greater number of shoots as well as higher canopy area and plant dry weight compared to plants obtained by stem cuttings (Marino et al., 2014). Blueberry (V. corymbosum L.) plants micropropagated in vitro produced more and longer shoots than plants obtained from semi-woody cuttings (Litwinczuk et al., 2005). This coincides with the present research, which observed shorter primary branches and shorter internodes in plants propagated by cuttings as compared to longer primary branches of in vitro propagated plants. Additionally, in vitro propagated plants of Andean blueberry had a planophyllous, almost runner-type branch formation, which promotes higher leaf production (Fig. 2). The higher number of shoots per plant in the in vitro propagated plants could also be due to the differences in plant age or size between the two propagation methods during this study.
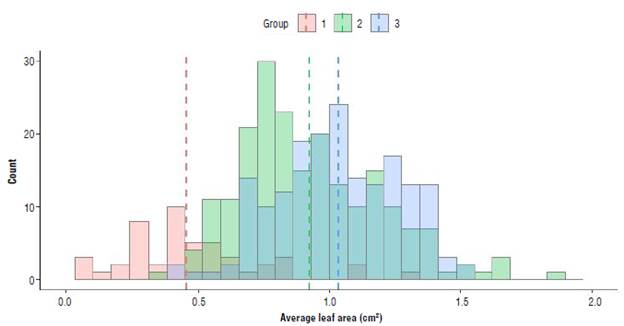
FIGURE 1 Histogram of the average leaf area for each of the Andean blueberry (Vaccinium meridionale Swartz) plant groups. Group 1: Young plants 10 months old (red) propagated by cuttings. Group 2: young plants 14 to 18 months old (blue) and group 3: young plants 22 to 26 months old (green) propagated in vitro. Dotted lines represent mean of each group. n=436.
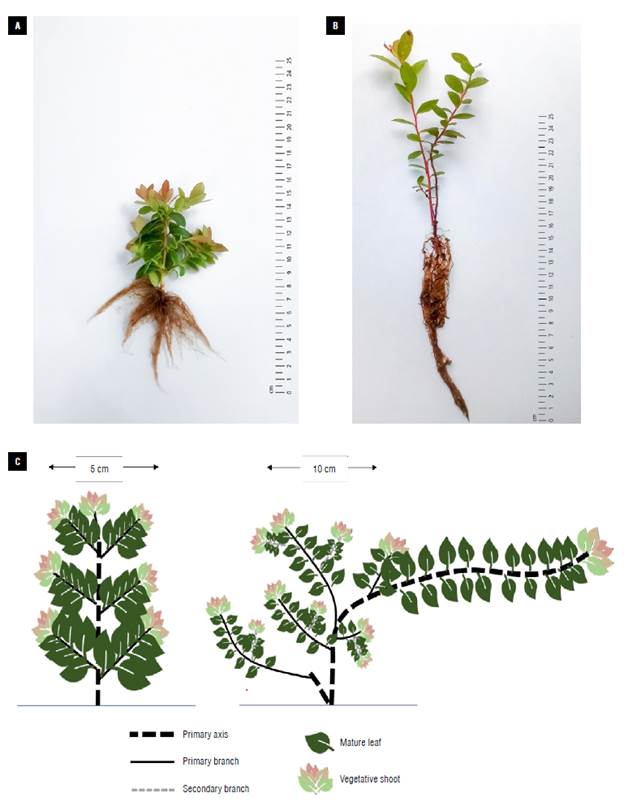
FIGURE 2 Photographs (A and B) and schematic representation (C) of the architecture of young Andean blueberry [Vaccinium meridionale Swartz) propagated from cuttings (A) and in vitro (B). The boxes in Fig. 2C indicate the scale in cm.
The ALA differed according to age; plants 10 (0.45 ± 0.27 cm2) and 14 (0.75 ± 0.18 cm2) month-old had lower ALA values, while plants 16 (0.99 ± 0.24 cm2), 18 (1.03 ± 0.27 cm2), 22 (0.99 ± 0.23 cm2), 24 (1.01 ± 0.22 cm2), and 26 (1.13 ± 0.20 cm2) month-old had a similar average ALA value (Fig. 3).
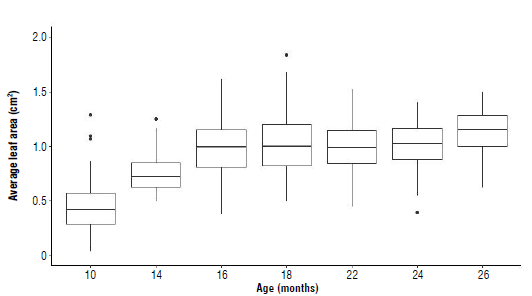
FIGURE 3 Box-and-whisker plot for average leaf area (ALA) in young Andean blueberry (Vaccinium meridionale Swartz) plants according to their age. Plants 10 months old propagated from cuttings and plants 14 to 26 months old propagated in vitro. n=436.
Most of the variables evaluated presented linear relationships with LA, except for plant height (H), number of vegetative shoots (NS), and root dry weight (RDW) (Fig. 4). Although in different plant species a linear relationship between LA and H has been reported (Calvo-Alvarado et al., 2008; Fanourakis et al., 2014), in Andean blueberry this relationship was not observed, apparently, due to the relatively low rate of growth of this species.
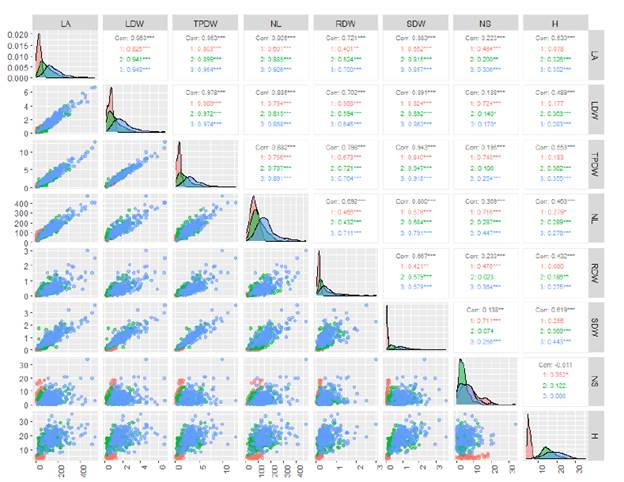
FIGURE 4 Dispersion, distribution, and Pearson correlation by pairs for the variables leaf area (LA), leaf dry weight (LDW), total plant dry weight (TPDW), number of leaves (NL), root dry weight (RDW), stem dry weight (SDW), number of shoots (NS), and height (H) of young plants of Andean blueberry (Vaccinium meridionale Swartz). Group 1: young plants 10 months old (red) propagated by cuttings. Group 2: young plants 14 to 18 months old (blue) and group 3: young plants 22 to 26 months old (green) propagated in vitro and the consolidation of these groups (black). n=436.
For the consolidated data, LA presented the highest Pearson correlation values with leaf dry weight (LDW) (0.95), total plant dry weight (TPDW) (0.95) and number of leaves (NL) (0.92) variables. The NL relationship with LA differed by groups, being similar between the groups of in vitro propagated plants (group 2: 0.88, group 3: 0.92) and higher than the young plants propagated by cuttings (group 1: 0.60), which suggests that the relationship between LA and NL differs according to the type of propagation (Fig. 2). Considering that the variables LDW, TPDW and NL presented the highest correlation values according to the methodology proposed by Mukaka (2012) and that the correlation between the groups was similar, models for leaf area prediction were proposed based on LDW, TPDW and NL, while the other variables were discarded.
The linear and multiple models initially proposed failed to meet some of the assumptions, but two of the multiple and three of the linear models (Tab. 2) from their weighting with the errors, using the weighted least squares technique, did meet the assumption. This technique is useful for observations that present non-constant variances in the errors (PennState, 2021) and was employed by Panigrahi and Sankar Das (2020), where they used this method to improve the remote estimation of the leaf area index and canopy water content in rice plants under water stress.
TABLE 2 Regression models with the best fit for estimating leaf area in young plants of the Andean blueberry.
N° | Model | R2 | R2 adjusted | RMSE |
---|---|---|---|---|
1 | LA = (28.392 x TPDW) + (0.465 x NL) -12.752 | 0.9182 | 0.9178 | 21.740 |
2 | LA = (51.119 x LDW) + (0.457 x NL) -10.766 | 0.8104 | 0.8100 | 21.753 |
3 | LA = (77.870 x LDW) + 7.191 | 0.8735 | 0.8732 | 26.579 |
4 | LA = (43.474 x TPDW) + 5.229 | 0.8733 | 0.8730 | 26.791 |
5 | LA = (1.117 x NL) - 18.730 | 0.8104 | 0.8100 | 33.702 |
LA: Leaf area per plant, cm2; LDW: Leaf dry weight of an individual plant, mg; TPDW: Total plant dry weight, mg; NL: Number of leaves per plant. R2: Coefficient of determination; R2 adj: Coefficient of determination adjusted for multiple variables; RMSE: Root Mean Square Error.
The multiple models (1 and 2) described in Table 3 had higher determination coefficients and a lower error, which shows their high capacity to estimate LA; however, their use implies the measurement of two variables, which depending on the case were LDW and NL or TPDW and NL. Considering the parsimony criterion, the use of linear models can be an interesting alternative, since this requires a single variable. Although models 3 and 4 use a destructive variable, it is experimentally easier and more accessible to weigh the plant organ than to make a direct measurement of LA. The Andean blueberry has numerous small-sized leaves (Ehlenfeldt et al., 2022), which makes it difficult to directly measure the LA or to perform other types of non-destructive measurements such as leaf length or width (Lancheros, 2012).
The leaf dry weight (LDW) is considered the most appropriate criterion to estimate plant photosynthetic assimilation, as it is closely related to the LA (Taiz et al., 2018). This is in agreement with Akram-Ghanderi and Soltani (2007) who showed that, for cotton, the power equation best described the relationship between LA and LDW. Finally, model 5 is an LA estimation alternative that uses a non-destructive variable, such as the number of leaves (NL). Similar studies in perennial crops, such as grapevines (Lopes & Pinto, 2005) and coffee (Montoya et al., 2017), show that models based on the NL result in an easy-to-measure and economical alternative to estimate LA. This has also been reported in semi-annual crops such as corn by Dwyer and Stewart (1986) and Elings (2000); the latter author highlighting that this relationship is true if the average LA of a single leaf is known and the plant has leaves of similar size, as is the case of the Andean blueberry (Ehlenfeldt et al, 2022).
Conclusions
The differences found in plant architecture and the average leaf area in young Andean blueberry plants depending on the propagation method (by cuttings or in vitro) must be considered for the planning of agronomic practices, such as pruning, studying the canopy architecture (plant density) and frequency of agronomic labors. This research proposes five models for an adequate estimation of leaf area that used destructive and non-destructive variables, and their combination. The adjustment of the models using weighted least squares fulfilled the assumptions for some of the proposed models. The use of the models developed in this research, especially the one that uses number of leaves (a non-destructive variable), could facilitate future studies for the determination of leaf area in this species.