INTRODUCTION
Quorum sensing (QS) is a mechanism allows to bacteria regulating the specific genes expression in response to changes in their population density, coordinating their activities as a multicellular entity 1,2. This mechanism is based on the production, by the bacteria, of small molecules signals called autoinductors (AIs) 3, which are released into the extracellular environment and detected by other bacteria through specific receptors 3. In Gram negative bacteria, at low bacterial density, small amounts of the autoinducer (AI) are produced by the presence of a LuxI-type enzyme, the Als passively diffuse into the extracellular medium in favor of a concentration gradient and immediately diluted on the surroundings of the environment. An increase in bacterial population density results in a gradual accumulation of AIs into and surrounding the cells 4,5,6. The Als specifically activate a transcriptional receptor (proteins type LuxR) by union to this one. The activated receptors then interact with white DNA sequences improving or locking the transcription of the genes regulated by QS, resulting in the synchronized activation of some phenotypes in the bacterial population such as the production of virulence factors, formation of biofilm, bioluminescence, sporulation and other behaviors, which have a significant impact on health, agriculture and the environment 7,9.
Knowing the nature of this phenomenon has generated great interest in the development of methods to control this signaling mechanism. In the last 25 years, significant advances have been achieved through both theoretical and experimental studies, focusing the efforts on design and synthesis of ligands able of modulating the different circuits from QS present at the negative Gram bacteria Pseudomonas aeruginosa, due to the potential fatal effects of their infections, causing death to miles of immune-engaged persons each year 10,11,12. Pseudomonas aeruginosa presents at least three well characterized QS circuits, being LasI-AI-LasR the most studied of all 13. Until present days, the QS synthetic agonist and antagonist are very rare and mainly structurally analogous to native N-acil homoserina lactonas (AHL), as can be observed at Figure 1. Recently, a set of 200000 compounds was tasted against a P. aeruginosa strain, unable of producing AHLs, being identifies powerful LasR14,15 activators composed by nucleus with base 2-(benzamido methyl)phenyl benzoate, which possess poor or null chemical similitude with AIs, AHLs of P. aeruginosa (Figure 2). These compounds, designed as TP-1 and its derivative TP-2, TP-3, y TP-4, have probed union affinities by LasR receptor, which are several orders of magnitude lower than any other type of activator and they don´t show crossed reactivity with the related signal receptors QScR, LuxR, and RhlR15).
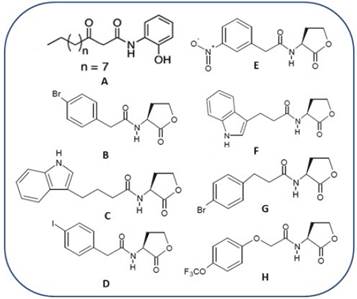
Figure 1 LasR antagonist synthetic reported until present days. Compound A, reported by Smith et al., 2003. Compounds B-H reported by Blackwell et al., 2007.
More than 50 species of Gram negative bacteria use the QS communication systems and more specifically use the AHLs as signal molecules, and therefore to conduct research in this area of micro-biology is of vital importance due to the resistance that bacteria have developed to the antibiotics.
Nowadays, computational chemistry tools are being used to support the development of new antibiotics with restriction each time major allow performing tests with specific receptors and later inferring about the rest of the receptors of this proteins family. The application of the virtual screening has become in a procedure much more simple of understanding and also faster of developing. It consists of computational analysis of databases of compounds, focused to identifying and selecting a limited number of candidates possess the whish bio-logical activity over a specific therapeutic goal 16.
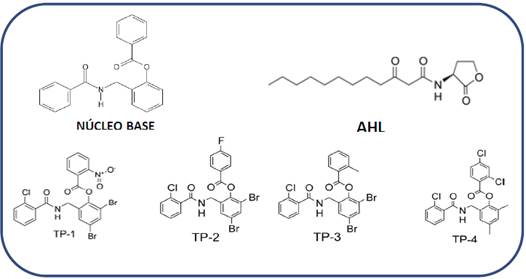
Figure 2 Structure of the molecules with nucleus with base 2-(benzamidomethyl) phenyl benzoate, TP-1, TP-2, TP-3 y TP-4. The structure of the native ligand (signal molecule) for LasR independent highlighting process, AHL is included as reference.
There are two approaches: virtual screening based on ligand and virtual screening based on the receptor structure. Among the ligand-based methods, pharmacophore search is one of the methods used for this propose, when a compound or a set of active compounds is available (which have in common certain steric and electronic features necessaries to guarantee an optimal supramolecular interaction with a biological structure and for activating or locking its biological answer) a pharmacophoric model can be generated, which can be used as a filter to suppress those compounds do not comply with the conditions of the necessary activities 17.
By the other hand, the molecular docking is a computational strategy based on the receptor structure, which has as last goal predicting (or usually reproducing in retrospective) the best way for one compound can couple into the binding site of one macromolecular object, evaluating the intermolecular interactions present in each explored shaping 18. Therefore, for a study of molecular docking requires two essential components: the search algorithm and the evaluation function.
In the present research, there were applied two virtual screening strategies in a sequential way, first the generation of pharmacophoric models later used as filters to screen a database of compounds for finally obtain a list of possible QS agonist, which were categorized by evaluation functions of molecular couple programs (docking). After developing and analyzing the outcomes of docking, those ligands with the best evaluation performance are possible candidates to be synthetized or they can be compared for being used during the experimental researches.
MATERIALS AND METHODS
For the identification of the possible compounds with agonist activity of the QS the following methodology was followed.
Analysis and comparison of interactions
Given the availability of crystalline structures from LasR-AHL, LasR-TP-1, LasR-TP-2, LasR-TP-3 and LasR-TP-419, there was performed an analysis of different interactions of each one of these molecules, obtaining biochemical and structural information allowed understanding the microenvironment the ligands are exposed into the active receptor 19.
Database
The base structure 2-(benzamidomethyl) phenyl benzoate present in the four compounds reported as agonists 14LasR-LasI and RhlR-RhlI. LasI catalyzes the synthesis of N-3-oxododecanoyl homoserine lactone (3OC12-HSL (Figure 2), was used to develop a search based on the substructure (only 2D structure or topology of the molecule) over the PubChem database20, the search result generated 32 compounds with structures similar to the nucleus base.
There was obtained information related with the molecular shape, ramification degree, molecular size and structural flexibility of the compounds resulting from the search. These compounds were used to generate a new focused database includes the 32 compounds obtained from the search over PubChem, the four compounds reported as agonist 14,21 and 5 compound belonging to a group of possible active reported 22. On this database, there is a totality of 9 real active and the 32 compound with possible activity, those last one based on the supposing of compounds with similar structure also possess similar activity; summarizing 41 compounds. Once created the database, a multi-conformational database was generated using the program Moe23.
Generation of pharmacophoric models
Taking into account the structural and chemical features of the compounds TP-1, TP-2, TP-3 y TP-4, two pharmacophoric models were developed using Moe program23. There were manually generated due to the common features into the active ligands set can be easily identified and also there exists experimental evidence of the functional groups must be present in the ligand to get a good activity 24.
Pharmacophoric model I
To the bioactive conformation of the most active compound (TP-1), hydrogens were added and optimized using the MMFF94 force field. This pharmacophoric model was manually generated setting the position, rates and other set of restrictions related to location and pharmacophoric features of the ligand all in an interactive way. For getting that goal there was used Pharmacophore Query Editor de Moe, which automatically detects the pharmacophoric features generating the pharmacophoric hypothesis 23. Pharmacophoric features used were donor and acceptor capacity of hydrogen, hydrophobic and aromatic hydrophobic bridges.
Pharmacophoric model II
The generation of the second pharmacophoric model was performed based on the alignment of the most active compounds TP-1, TP-3, TP-4, using Pharmacophore Consensus de Moe23, there was applied the same procedure that at model I except by the hydrophobic features.
Obtaining and hits addition
Once the pharmacophoric models were generated, the search was performed over a multiconformational database (database of conformations of each of the considered compounds) previously calculated and not generated during the search because each one of the database entries is straight superposed over the generated pharmacophoric hypothesis (using Pharmacophore Search de Moe) generating a list of compounds, which represent possible hits present in the screened database. The result obtained is a list with the compounds considered as active (Hit list), which is ordered takin into account the average squared distances, expressed as RMSD among each pharmacophoric feature from the model and its corresponding point in the conformation evaluated.
To evaluate the hits list and the precision of the applied methods the following metrics were used, typically applied in searches with pharmacophoric models 25.
For a database D of n compounds, A represents the number of active compounds in database D, Ht is the number of compounds in the list of hits, and Ha is the number of active in the list of hits.
Molecular Docking
The receptor structure was obtained from Protein Data Bank26. The program used for molecular docking was AutoDock 4.227. Validation of the modeling system was previously performed. The 3D structure of the TP-1 ligand was extracted from the co-crystallized complex with PDB code 3IX319, using the program Sybyl 7.3 28 and was subjected to a geometric optimization using the PM3 semiempiric method 29 implemented on Gaussian 0330. The structure protein was visualized and modified (all water molecules remoting) with Sybyl 7.3. TP-1 ligand was prepared with AutoDock tools31 to check the polar hydrogens, Gasteiger charges were added 32 and routable bonds were specified. On the other hand, only the polar hydrogens were added to the protein and the Gasteiger type loads were calculated. For the grid preparation a box was taken centered at the ligand binding site with a size 50 × 50 × 50 with a spacing between the points of 0.375 Å. For the generation of the poses was selected the genetic algorithm lamarckian 33, 100 runs were calculated. The other parameters were selected by default.
After coupling, the co-crystallized structure of the TP-1 ligand was compared versus its coupled structure into the active site of LasR receptor, using the standard mean square deviation (RMSD) criterion. The 22 ligands obtained from the pharma-cophoric search were also optimized and coupled following the same protocol previously describe du-ring the validation. The best conformation of each ligand was selected taking into account the docking free energy and its position inside the binding site.
RESULTS
Interactions analysis
In order to identify the pharmacophore and structural characteristics of the LasR agonists, previous docking calculations between the active site of LasR and its native ligand and between the active site of LasR and the ligand TP-1 (Figure 3) were performed. A great similarity in the orientation was taken by each ligand in the active site. The hydrogen bonding interactions present between LasR and its native ligand are also present between this receptor and TP-1 ligand. In addition, the latter ligand has three aromatic rings in its structure that intervene in other similar interactions with the receptor (Figure 3). Ring 1 is stacked on the indole group of Trp-88 by a “face-to-face” interaction and interacts with the aromatic ring of Phe-101 as reported by Zou et al (19.
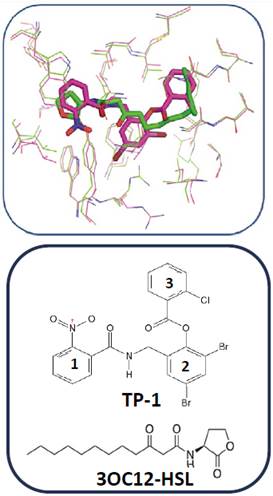
Figure 3 Alignment between the active site of LasR co-crystallized with its native ligand (in green, 3OC12-HSL) and the active site of LasR co-crystallized with the TP-1 ligand (in pink pocket).
Ring 2, on the other hand, has two bromine atoms, which participate in halogen bridges with the carbonyl oxygen of Tyr-47 (3.52 Å) and the Nε1 of Trp-60 (3.69 Å) and participates in a “Face to face” (ᴫ -ᴫ interaction) with the aromatic ring of Tyr-64. Finally, ring 3 mimics the orientation taken by the hydrocarbon chain of the native ligand in the hydrophobic LasR pocket, establishing Van der Waals interactions with the residues present in this pocket.
Pharmacophoric model I: Based on the bioactive conformation of TP-1
The database created is composed of 41 molecules, 9 real active and 32 obtained from the PubChem database (possible active). The pharmacophoric model I (Figure 4) generated a hit list of 31 molecules, 25 of them are possible actives and 6 are real actives. This model presents an actives return of 19.35% and an actives ratio of 66.66% (Table 1). In general, this model recognizes as hits to the four real agonists 14 and also two of the compounds with agonistic activity reported by Blackwell et al, 22, which is an evidence of its selectivity, only classifies 3 real active molecules as false negatives, as can be seen in Table 1. By the other hand, it identifies 25 compounds (false positives) that comply with the pharmacophoric characteristics, such as a hydrophobic region and a region with donor and electron acceptor groups.
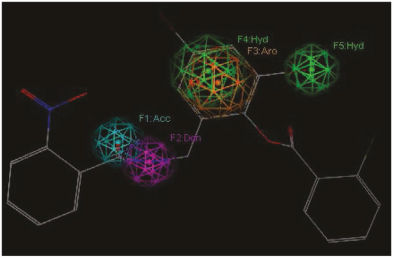
Figure 4 Representation of the pharmacophoric model I. features are represented with colored spheres on the TP-1 compounds (the most active): hydrophobic (Green), Donor (lilac), acceptor (cyan).
Pharmaphoric Model II: based on the alignment
The pharmaphoric model II (Figure 5) generated a hits list of 26 molecules, 22 of them are possible actives and 4 are real actives. This model presents an asset yield of 15.3% and an actives ratio of 44.4% (Table 2). In general, this model recognizes four real agonists, the compounds with nucleus base 2-(benzamidomethyl)phenyl benzoate 13, being an indication of its selectivity, classifies five real active molecules as false negatives, as can be seen in Table 2. By tradition, the model with major actives performance value (%Y) is the best, and more selective will be its pharmacophore over the database domain, therefore, the first model is more selective, being its more logical selection to follow with the research
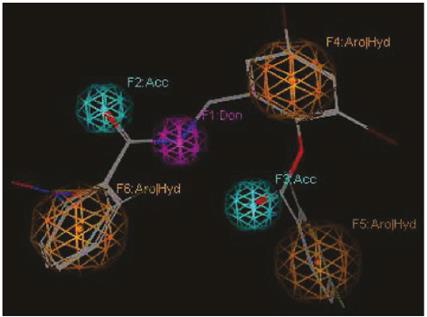
Figure 5 Representation of the pharmacophoric model II. Features are represented with colored spheres over the alignment compounds 2: Aro (orange), Donnor (lilac), acceptor (cian).
Analyzing the pharmacophoric features of model II and comparing with the interaction type presents into the co-crystallized complex from TP-1, TP-2, TP-3, TP-4, there was found a high relation among them. Therefore, the 22 false positives identified by this model (Figure 6), were submitted to a molecular docking calculation with the studied receptor (LasR).
Molecular Docking
In the validation of the docking methodology applied in this work the co-crystallized structure of the TP-1 ligand was compared to its structure coupled to the active site of the LasR receptor, using in addition to the visual criterion, the criterion of mean square deviation (RMSD). Figure 7 shows the alignment between the conformation of the TP-1 ligand corresponding to the co-crystallized complex in green color and the conformation result of the docking is shown in yellow color. As can be observed, the coupled structure is located at the LasR binding site taking a very similar conformation to that presented by the TP-1 ligand in its crystallized structure, superimposing the three aromatic rings almost identically to the ligand Presented in the co-crystallized structure. The only difference is found in the nitro substituent in ring number 1 of TP-1.
The qualitative analysis described above was confirmed by the RMSD value, calculated for the conformation coupled to the conformation of the TP-1 ligand present in the co-crystallized complex, for this calculation a value of 0.62 Å was obtained, this value is an indication that our Docking program (AutoDock 4.2) correctly reproduces the binding form and the interactions present between the LasR receptor and the TP-1 ligand. This value is an indication of our Docking (AutoDock 4.2) program effectively reproduces the union shape and the interactions present between the LasR receptor and the TP-1 ligand.
An analysis starting with the hydrogen bon-ding interactions between TP-1 and the active site of LasR (Figure 7) was observed that the ligand regions involved in this type of interactions are likely to recognize and need the nascent polypeptide to begin their folding, for such reason, these polar groups present in the ligands are given great importance at the time of synthesizing compounds capable of modulating the receptor-ligand circuit present in the QS, in particular the adjacent carbonyl and amine groups.
There have been demonstrated by multiple alignment between different transcriptional receptors type LuxRs (34) that the aminoacids implicated into hydrogen bridge interactions are conserved in a wide variety of these proteins(LuxR type) from Gram negative bacteria 34-36) some docking calculations were carried out for analogs of the acyl homoserin lactones (AHLs). It is thus that the chemical characteristics of the functional groups present in the residues that participate in this type of interactions are considered as key points for the search and rational design of modulators of the QS circuits present in Gram negative bacteria.
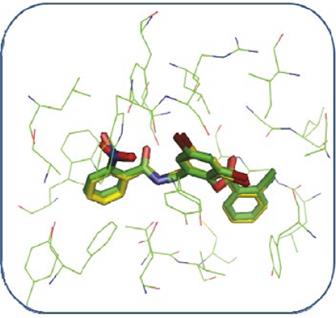
Figure 7 Alignment between the TP-1 ligand present in the co-crystallized complex (green) and the coupled ligand (yellow) using the AutoDock 4.2 program.
On the other hand, when analyzing possible interactions between the aromatic rings of the TP-1 ligand and the aromatic side chains of some amino-acids at the active site of the LasR receptor (Figure 8) between the ring number 2 of the TP-1 ligand and the aromatic ring of Tyr-64 there is a “face to face” arrangement allowing, in this way, a favor-able interaction between the rings. Ring number 1 again has a face-to-face arrangement with Trp-88 and an edge-to-face arrangement with Phe-101. The presence of the nitro group allows a favorable interaction with the indol ring of Trp-88, as well as the aromatic ring from Phe-101. Ring number 3, presents a T-shaped arrangement with Tyr-47, as can be observed in Figure 9. The interactions present in this type of stacks are of type CH-π, very similar to the interactions that are present between a weak base and a weak acid.
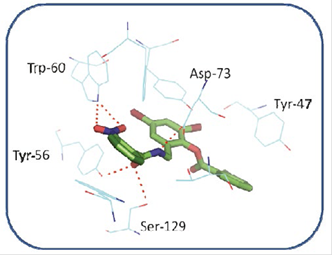
Figure 8 Interactions between some aminoacids of the active site of LasR and TP-1. The amino acid Tyr-47, has a small interaction with the bromine atom in the meta position of ring number 2
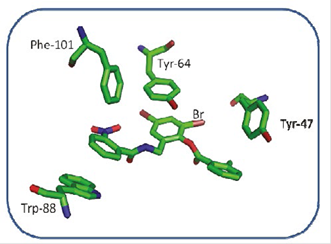
Figure 9 Interactions between the aromatic rings of the residues Tyr-47, TYR-64, Phe-101, Trp-88 and the aromatic rings of the TP-1 ligand.
The interactions mentioned above are those that allow nucleoside 2-(benzamido(methyl) phenyl benzoate compounds to stabilize the folding of the transcriptional receptor LasR. For this reason, when analyzing the results of our docking calculations, we will take into account the compounds having the polar groups mentioned above and the aromatic groups capable of reproducing all the π-π type interactions.
Docking between LasR and the possible agonists
In general, the coupled compounds took a conformation very similar to that taken by the TP-1 ligand; in some cases it is evident that the conformation allows the ligands to form all the existing interactions in TP-1, the aromatic rings of all coupled compounds oriented correctly towards the amino acids with which they form the π-π interactions (Figure 10). The compounds shown in Figures 11a and 11c, having a base similar to 2- (benzamido(methyl) phenyl benzoate, are considered potential candidates for synthesis and in vitro agonist activity tests.
However, for other cases, marked differences between the conformation of the ligands under study and the conformation of the TP-1 ligand (Figure 11b and 11d) were observed. In Figure 11b it is observed that the chemical structure of the coupled ligand differs from the base structure of the TP-1 ligand by the presence of a bicyclic at the position of ring number 2 of these compounds.
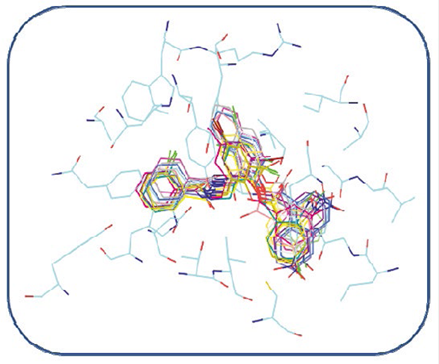
Figure 10 Alignment of the best conformations of all compounds identified as hit in our pharmacophoric model within the LasR binding site
This fact can have consequences from the steric point of view, for which this characteristic must be taken into account in a possible synthesis. In the case of Figure 11d also a structural difference was observed with the base structure of 2- (benzamido-methyl) phenyl benzoate. There is a bicyclic at the position of ring number 3, unlike the steric effect that would possibly cause the bicyclic of Figure 11b to the complex. The effect in this case is different because the bicyclic is located in a hydrophobic pocket, a much larger volume so it would be ideal to establish interactions of the Van der Waals type, which would possibly increase the stability of the receiver.
DISCUSSION
The alignment shown at Figure 3 evidences the tridimensional orientation taken by TP-1 ligand into the active site from LasR, which allows knowing the structural features and the interactions between this compound and LasR, stablishing conditions to attend a focused search about new possible QS antagonists, using pharmacophoric models in our case.
The Figure 5 shows the pharmacophoric features present into the model II, which are more than those proposed in the model I, for that reason the compounds pass thru this filter should be chemical and structurally very similar to the compounds used for performing the alignment, being the possibly identified as Hit. The final goal to develop these pharmacophoric model was to use them filters to identify significant features in the molecular acknowledge. This research that procedure has a binary character type because answer to requests about the pharmacophoric features or not.
In the validation of the docking protocol the calculated RMSD value was 0.62 Å, an RMSD value less than 2 Å is considered a reliable result 37 and in addition the structural similarity between the hits and the four core compound 2-(benzamidomethyl) phenyl benzoate can give some certainty about the results that AutoDock 4.2 shows. The interactions between each ligand and the conserved residues of this family of proteins are those that allow molecular recognition, whereas other interactions, such as π-π type interactions, are those that allow the folding of the transcriptional receptor type LasR. For this reason, when analyzing the results of our docking, we took into account the compounds that have the polar groups mentioned above and the aromatic groups capable of reproducing all the interactions type π- π. These interactions are reproduced in each of the 22 compounds shown in Figure 6, this may be an indication of the possibilities possessed by this group of compounds to be considered for a rational synthesis.
In general the compounds found can be classified into three groups which possess the bicyclo at the position corresponding to the ring number two of the 2-(benzamido(methyl) phenyl benzoate derivatives, which have the bicyclo at the position corresponding to ring number three of The 2-(benzamido(methyl) phenyl benzoate derivatives and those having the same base structure as the 2-(benzamidomethyl) phenyl benzoate derivatives. Finally, it is important to mention that for further studies it is of vital importance to use compound with biological activities, to be able to relate to the data obtained from the theoretical calculations, in order to move from classification models to predictive models.
CONCLUSIONS
Among the two pharmacophoric models designed in this study, the number 2 (model based on the alignment of the most active compounds) showed greater ability to select or identify compounds that had important structural and chemical characteristics to be considered possible hits. With this model, 22 compounds were identified, which were subsequently subjected to docking calculations.
In general, the docking protocol used is adequate, since in validating the conformation of the co-crystallized ligand, the program reproduces it adequately, in addition to the visual criterion an RMSD value of 0.62 Å was obtained in validation processes A computed conformation is considered correct if its RMSD value is less than 2 Å. The above is a valid reason to use AutoDock 4.2 as a program to work the existing links between this type of ligand and the transcriptional receptors belonging to the LuxR family of proteins.
The antagonist capacity of TP-1, TP-2, TP-3, TP-4 compounds is mainly due to their chemical structure and the pharmacophoric groups present, which allow them to be recognized by the LasR receptor to start their folding while supply structural requirements and interactions necessary for the correct LasR folding
The results of the docking were supported to affirm that the identified compounds can be considered possible candidates for computational analysis more expensive and also to evaluate the possibility of routes of synthesis, taking into account the structural similarity that these compounds present with the derivatives of 2-benzamido(methyl) phenyl benzoate, thus providing an alternative to find new compounds with QS agonist activity.