Research on the academic performance of students in secondary education has shown the influence of student variables (e.g., academic self-concept, self-efficacy, etc.) (Marsh et al., 2018; Rossi et al., 2020) and the socio-familial variables (e.g., family economic status, parental expectations, family support, etc.) (Cheng & Kaplowitz, 2016; Zamudio et al., 2019). The socio-family environment in which the student develops can endanger his good academic performance, reducing his academic outcomes and even leading him to fail in secondary school due to the influence of socio-familial risk variables such as low family income, poor family expectations in the child’s academic development, intrafamily conflict, lack of parental support, etc. (Acacio-Claro et al., 2018; Renta et al., 2019).
The socio-familial risk variables considered in this research are parents’ low educational level and parents’ low educational expectations of their child’s education. Parents with a lower educational level are less able to properly advise their children on their educational trajectories (Chaparro et al., 2016; Tan, 2017). Parents who have low expectations in regards to their children’s educational successes are usually from disadvantaged backgrounds, and had few positive experiences in their educational life, feel less able to support their children’s learning - factors that probably contribute to a child’s expectations of academic success (Diemer & Li, 2012; Froiland & Davison, 2014; Mitchall & Jaeger, 2018).
Non-cognitive variables are factors related to attitudes and beliefs, social and emotional qualities, learning processes, and personality (Lipnevich & Roberts, 2012; Rodríguez- Rodríguez, 2020), with self-eficacy and academic self-concept being some important examples given their influence on academic performance, so they must be included in the predictor models (Cárcamo et al., 2020; Mello & Hernández, 2019; Talsma et al., 2018; Willems et al., 2019).
Academic self-concept is understood as a student’s perception of their academic ability, which is formed through their own experience in learning contexts and from relevant educational achievements (Brunner et al., 2010). Self-efficacy is described as beliefs in one’s capabilities to organize and execute the courses of action required to produce given attainments (Bandura, 1997), and has been shown to be one of the variables with the best results when it comes to predicting and explaining academic performance. Therefore, it has been a key factor in the consideration of the influence of non-cognitive variables on academic performance (Fonteyne et al., 2017). Despite the fact that perceived non-cognitive family support has not counted on the same amount of research compared to the previous non-cognitive variables described, different authors have indicated their contribution to academic performance (Wilder, 2014). A student’s perception that he or she will be supported by his or her parents in the face of any academic adversity is essential for academic adjustment, as it promotes the psychological well-being of the student and allows him or her to confront stressful situations (Dorrance et al., 2017; Jiménez et al., 2020).
Recent evidence suggests that in students who grew up in socio-familial risk settings, the incidence of non-cognitive and socio-familial variables in academic performance may be different (Benner et al., 2016; Gordon, 2016). Therefore, more studies about the influence of non-cognitive and socio- familial variables on the academic performance of atrisk students are needed.
Secondary school students who grew up in familial risk backgrounds are more vulnerable to face academic difficulties and even academic failure (Hancock et al., 2018). This puts some students who are in a crucial educational stage at a disadvantaged, since finishing secondary education successfully predicts, to a large extent, their social and professional future (Palomar-Lever & Victorio-Estrada, 2017).
The accumulation of risk factors is one of the approaches with the greatest empirical evidence when considering the socio-familial risk factors that students may present (Rutter, 1979, 1987). According to this approach, additive effects must be considered, given that the exposure to several socio-familial risk factors is even more detrimental than the exposure to each of them individually. Research from this perspective confirms the negative influence of the accumulation of socio-familial risk factors on academic performance (Prelow & Loukas, 2003; Roy & Raver, 2014).
Current study
The empirical evidence has been consistent in supporting the effect caused by both socio-familial (Gordon, 2016; Whitney et al., 2017) and non-cognitive variables (Froiland & Worrel, 2016; Rocchino et al., 2017) on the academic performance of secondary school students. However, research regarding determinants of academic performance has largely been focused on the identification of individual determinants, while the interaction of these factors has been less investigated (De Clercq et al., 2013).
Therefore, the purpose of this research was to develop a model of academic performance of at-risk students from secondary education based on non-cognitive and socio-familial variables, and to verify similarities and differences with not-at-risk students.
The hypotheses proposed for this research are, (1) the accumulation of socio-familial risks negatively affects academic performance, (2) the weight on the academic performance of the socio-familial and non-cognitive variables will be different for at-risk and not-at-risk students, and (3) non-cognitive variables mediate the effect of socio-familial variables.
Method
Participants
Conglomerate random sampling was used to select the sample, using the class as the sampling unit. The clusters were selected from all secondary education classes in state secondary schools in Tenerife. 52 classes from 32 state secondary schools in Tenerife (Spain) were selected. A total of 1296 students (640 girls and 656 boys) aged between 12-18 years (M = 14.22; SD = 1.26) participated in this study. 25.9% were in the first grade, 26.9% in the second grade, 22.1% in the third grade, and 25.1% in the fourth grade. Each course was checked using a Chi Square to see if there were differences in the distribution of girls and boys. The results showed a homogeneous distribution, c2 (3) = 6.38; p = .09. The total sample was divided into two groups, at-risk students (n = 305) and not-at-risk students (n = 991).
The selected sample of students with socio-familial risk factors was made by calculating a cumulative risk index (CRI), according to the perspective of accumulated risks (Rutter, 1979, 1987). In order to do this, the procedure used in previous studies was followed (Ragnarsdottir et al., 2017; Roy & Raver, 2014). The socio-familial variables used to calculate the CRI were the following: educational level of father and mother, and expectations of father and mother regarding the academic future of the student. In each variable the lower-range response was considered as a risk factor: without studies, and null expectations that his/her child will finish secondary education. All socio-familial risk factors correlated with each other (p < .05), so the creation of the CRI was justified (Evans et al., 2013). The answers were dichotomized, assigning a value of 0 to the alternatives without risk and 1 to the previous alternatives considered to have a risk. The sum was calculated in order to obtain the CRI, with a range of 0-4.
Once the CRI was calculated, a receiver operating characteristic (ROC) curve analyser was performed. A ROC curve was used to determine the optimum number of socio familial risk factors that can be used to classify at-risk students versus not-at-risk students. Table 1 provides a summary of the ROC curve analysis for the CRI. The CRI had an accuracy of 81% correct classification, with a score of 3 or higher resulting in a sensitivity of .808 and a specificity of .659 This means that using 3 as a cut-off point would correctly identify those students who are at-risk to fail academically (GPA < 5) 80.1% of the time, while incorrectly identifying the not-at-risk students 34.1% of the time.
Instruments
Academic self-concept. The content and the questions of the secondary education level, “What do you think about yourself?” Scale was adjusted (Rodríguez-Espinar, 1982), maintaining the number of items, the format of the presentation, and the alternative answers. The instrument consists of seven statements with five different responses, 1 being the worst option and 5 the best option. In order to know the validity of the adaptation made, an exploratory factorial analysis was carried out with the total sample of this study. The KMO test was from .882 and Bartlett’s sphericity test was significant (p < .001). The Principal Component Analysis showed a single factor that explained the 60.05% of the total variance. The reliability of Cronbach’s a was .88.
Self-efficacy. The General Self-Efficacy Scale (Sanjuán et al., 2000), formed by 10 items on a four-point rating scale, ranging from 1 (wrong) to 4 (true). Cronbach’s for the total sample of this study was .83.
Perceived affective family support. To gather information about the perceptions of affective family support, the adaptation made by Santana et al. (2016) for secondary school students, from the original scale elaborated by Figuera et al. (2003), was used. The scale contains four items with 4 response alternatives, with 1 totally disagreeing, 2 disagreeing, 3 agreeing and 4 totally agreeing. The KMO test gave a value of .748 and Bartlett’s sphericity test was significant (p < .001). The Principal Components Analysis generated a single factor that explained 68.32% of the total variance. Cronbach’s for this sample was .85.
Socio-familial variables. A sociodemographic questionnaire was designed ad hoc. This questionnaire included the following socio-familial variables with their respective categories: educational level of father and mother (1 = No studies, 2 = Primary, 3 = Secondary, 4 = High school or equivalent, 5 = University studies) and expectations of father and mother regarding the academic future of the student (1 = null expectations that the child will finish secondary education, 2 = expectations that child will finish secondary education, 3 = expectations that child will finish high school or equivalent, 4 = expectations that child will finish university studies).
Academic performance. This was obtained from school records, using the grade point average (GPA) of all the subjects in the final evaluation. Grades in the Spanish education system range from 1 to 10, with a score of 5 or more being considered a “pass”. Therefore, ≥5 GPA was considered a good performance and <5 GPA a low performance.
Procedure
Parental informed consent was obtained prior to the beginning of the study as well as from both the teachers and students. In all three cases it was reported that the participants’ data would be treated confidentially and would only be used for research. The measures of non-cognitive variables were group administered to the students by the study researchers during normal scheduled class time. The students were encouraged to respond to each item according to their own beliefs and knowledge. The measures of the socio-familial variables were administrated to parents of students by letter, later to be returned by their children to the school. All participants were aware of the voluntary, anonymous, and confidential nature of the investigation.
Statistical analysis
Descriptive statics with t-tests were performed and Cohen’s d was used to establish the effect size, while correlations and demographical data were analysed using SPSS (Vers. 24). SEMs were made with Amos (Vers. 24). After verifying compliance with the normal distribution of the variables in both groups of students (at-risk and not-at-risk), the Maximum Likelihood estimator was used for each group. The fit of the models was checked with the Chi-Square Test of Model Fit (c2), the comparative fit index (CFI), the Tucker-Lewis Index (TLI), the root mean square error of approximation (RMSEA), and the standardized root mean square residual (SRMR).
Results
Descriptive statistics disaggregated by risk status are presented in Table 2 for academic performance, perceived affective family support, academic self-concept, and self-efficacy. Group means were compared using t-tests and, as expected, students identified as at-risk scored significantly lower on all measures.
Table 2 Descriptive statistics of variables by risk status and t-tests comparing groups
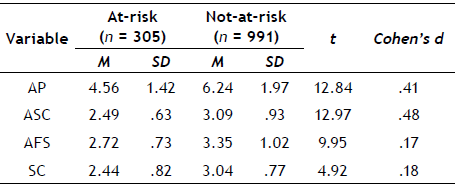
All t-tests significant at p < .001. AP = Academic Performance; ASC = Academic Self-Concept; AFS = Perceived affective family support; SC = Self-Efficacy.
Pearson’s correlation was performed for each group among all the variables considered for the realization of the SEM. Table 3 shows the results for at-risk students and Table 4 for not-at-risk students.
Table 3 Correlations, kurtosis and skewness of variables at-risk group
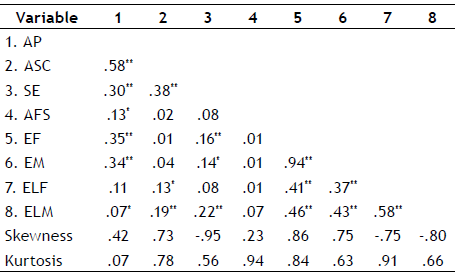
* p < .05. ** p < .001. AP = Academic Performance; ASC = Academic Self-Concept; SE = Self-Efficacy; AFS = Perceived affective family support; EF = Expectations of the father; EM = Expectations of the mother; ELF = Educational level of the father; ELM = Educational level of the mother.
Table 4 Correlations, kurtosis and skewness of variables not-at-risk group
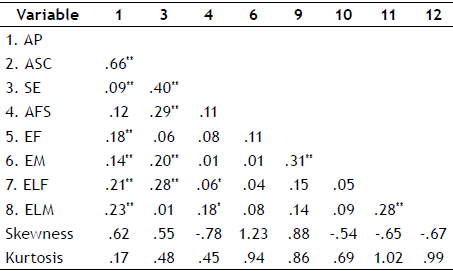
* p < .05. ** p < .001. AP = Academic Performance; ASC = Academic Self-Concept; SE = Self-Efficacy; AFS = Perceived affective family support; EF = Expectations of the father; EM = Expectations of the mother; ELF = Educational level of the father; ELM = educational level of the mother
In at-risk students the academic self-concept, the self-efficacy, the perceived affective family support, the expectations of the father and the mother, and the educational level of the mother were positively associated with academic performance.
In not-at-risk students the academic self-concept, the self-efficacy, the expectations of father and mother, and the educational level of father and mother were positively associated with academic performance. The main differences between both groups were that in the at-risk students the perceived affective family support was positively associated with the academic performance, while in the not-at-risk students this association was not found, and in not-at-risk students the educational level of the father mother were positively associated with academic performance.
Structural models
Two separate path models were run, one for at-risk students and one for not-at-risk students.
The first SEM was developed with at-risk students. The observed fit statistics showed that the measurement model was a good fit to the data (χ2 = 3.66, p <. 05; CFI = .97; TLI = .96; RMSEA = .04; SRMR = .03 The standardized path coefficients for the research model are presented in Figure 1. This model accounted for 66.5% of the variance in academic performance (R 2 = .665; p < .001).
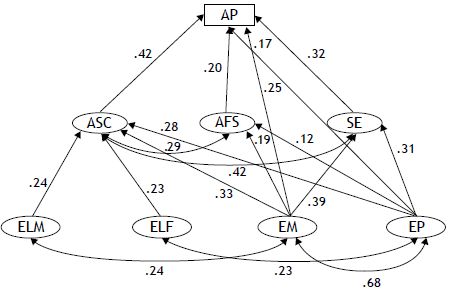
AP = academic performance; SE = self-efficacy; ASC = academic selfconcept; AFS = perceived affective family support; EF = expectations of the father; EM = expectations of the mother; ELF = educational level of the father; ELM = educational level of the mother.
Figure 1 At-risk students model
The results obtained showed that for the at-risk group the predictors of academic performance in order of importance were: the academic self-concept (β = .42; p <.001), self-efficacy (β= .32; p <.001), expectations of the mother (β = .25; p < .001), perceived affective family support (β = .20; p < .01) and the expectations of the father (β = .17; p < .05).
The second model (see Figure 2) was developed with not-at-risk students. In this case, the good fit of the model was also confirmed (χ2 = 13.90, p > .05; CFI = .98; TLI = .96; RMSEA = .04; SRMR = .04). The p ercentage of v ariance in the academic performance of the not-at-risk students was 64.2% (R 2 = .642; p < .001).
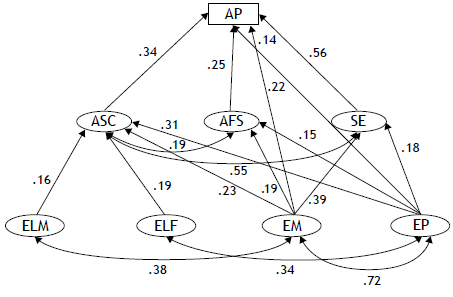
AP = academic performance; SE = self-efficacy; ASC = academic selfconcept; AFS = perceived affective family support; EF = expectations of the father; EM = expectations of the mother; ELF = educational level of the father; ELM = educational level of the mother.
Figure 2 Not-at-risk students model
For the not-at-risk students, it was observed that, although the same variables were related to academic performance, their weights were different from the at-risk student model. From greater to lesser importance according to their impact on performance were: first, and with a lot of difference from the others, self-efficacy (β = .56; p <.001), followed by academic self-concept (β = .34; p <.001), perceived affective family support (β = .25; p <.001), expectations of the mother (β = .22; p <.001), and lastly the expectations of the father (β= .14; p <.01).
Discussion
The overall objective of this work was to study the relationship of non-cognitive variables (academic self-concept, self-efficacy and perceived affective family support) and socio-familial variables (educational level and expectations of each parent) and their impact on the academic performance of secondary education at-risk students, and more specifically, on the similarities and differences that may exist between at-risk and not-at-risk students. Regarding the three hypotheses proposed, the first and the second were confirmed and the third was partially confirmed. It was shown that the accumulation of socio-familial risks negatively affects academic performance, that the weight of socio-familial and non-cognitive variables on the academic performance is different for at-risk and not-at-risk students, and that non-cognitive variables mediate the influence of socio-familial variables in the academic performance of students, except in the expectations of parents, which directly influenced academic performance.
The study confirmed that, whenever the risk index based on socio familial factors was three or more, a significant decrease in students’ academic performance was obtained. These results are partially consistent with previous research, which, using the cumulative risk pproach to examine students’ school progress, have found that the specific use of cumulative risk index provides an effective and efficient way to explain poor academic results (Prelow & Loukas, 2003; Ragnarsdottir et al., 2017).
In this study, the results obtained with both groups of students indicated that the relationship of non-cognitive and socio-familial variables with academic performance were different depending on which group they belonged to.
In the case of at-risk students, academic self-concept and self-efficacy were found to be the non-cognitive variables that best predicted performance. This was reaffirmed with the high correlation found between these same variables. Other studies with students at risk due to socio-familial circumstances have also shown the importance of these factors for academic performance. Rocchino et al. (2017) found that self-efficacy was a determining factor for these students since it encourages more adaptive coping styles, which in turn results in performance improvements. Regarding academic self-concept, authors of reference in this field (Marsh et al., 2018) have argued the importance of this variable in educational intervention with students in situations of socio-family disadvantages.
Among the socio-familial variables, the expectations of the mother were the variable that had the greatest impact on performance. This result is consistent with those obtained in previous studies in which it has been shown that the influence of parental expectations on academic performance was greater than other family variables such as supervision or participation in school, and even those related to SES familiar. Thus, for example, the longitudinal study conducted by Diemer and Li (2012), with a sample of students evaluated from 13 to 18 years old, showed the relevance of maternal expectations in students of low SES in obtaining good educational results. In the same direction, Benner et al. (2016) found that the effect of the expectations of the mother was especially beneficial for the academic performance of low-SES high school students. The results of the importance of parents’ expectations are important to mitigate the effects of socioeconomic origin of academic performance, since schools can influence parents’ expectations, but they cannot change their socioeconomic status. Schools could involve parents more directly by encouraging parental involvement in their children’s learning, and communicating the importance of raising parents’ expectations for student learning (Tan, 2017). Therefore, parental expectations are crucial in order to improve the academic performance of these students and should be taken into account for the educational improvement of at-risk students (Froiland & Davison, 2014).
With regard to not-at-risk students, the non-cognitive variable that stood out over the others when considering their impact on performance was self-efficacy; however, the results also confirmed the relationship of the academic self-concept and the perceived affective family support on the performance of not-at-risk students. These results are in line with what the most important reviews elaborated regarding the influence of self-efficacy on the performance of students without socio-familial risks affirm. The meta-analysis carried out by Talsma et al. (2018) confirmed the fundamental role of self-efficacy in academic performance, but the authors propose that the study of this variable should be deepened according to the socioeconomic, family and cultural origin of the students.
Among the family variables analysed, as found with the at-risk students, the expectations of the mother was the variable with the highest incidence in performance. This result showed the relevance of parents’ expectations for the academic success of their children, even when other socio-familial factors such as SES were taken into account (Froiland & Davison, 2014). Tan (2017), in a study conducted with data from eight countries from the Program for International Student Assessment (PISA) 2012, found that parents’ expectations had a great effect on their children’s school results, above other socio-familial variables such as educational level or occupational status of parents.
Research on the academic performance of secondary school students normally uses normative and widely available samples, but this study found that variables associated with performance differences in the general population do not influence similarly depending on whether or not students are at-risk. In the group of at-risk students, the most important variable was the academic self-concept, followed by self-efficacy. In the not-at-risk students group, the weight of the most relevant variable was self-efficacy, which had a considerably higher weight than the rest. Finally, the perceived affective family support was more important in the not-at-risk students.
Regarding the similarities between the two groups, it was found that they were associated with the socio-familial variables. Thus, both, in not-at-risk and at-risk students, it was obtained that the parents’ expectations was the variable with the highest impact on performance; although in the group of at-risk students, the values were higher in the expectations of the mother than in the expectations of the father.
This research has some limitations. It was cross-sectional and did not investigate whether the relationships between the variables change over time in regards to the prediction of academic performance. This study was also limited in terms of the variables that were included in the model. Future studies should include additional variables that have demonstrated a significant impact on academic performance, such as learning strategies, self-regulation of effort, and variables related to teachers.
Conclusions
The findings have important practical implications. Given the academic vulnerability presented by at-risk students, teachers and educational institutions should intervene supporting the development of academic self-concept and self-efficacy to achieve significant improvements in their school results. It is especially noteworthy to take into account the academic self-concept for adolescents who face considerable family, social and economic disadvantages in such interventions. The development of a positive academic self-concept can be especially important to counteract the negative academic self-beliefs generated by negative stereotypes and the socioeconomic disadvantages suffered by at-risk students. Therefore, it must be considered as a central objective of education to address inequalities experienced by students with social and familial risks. Specific interventions that focus on reinforcing tutoring with these students to explicitly focus on academic self-concept may be important for the educational success of these teenagers.
The results of the study also suggest that the most important socio-familial variables for both at-risk or not-atrisk students’ performance, are the parents’ expectations (especially the expectations of the mother) and the affective family support. Interventions that are carried out by schools in the relationship with families should take into account these two variables. Schools could engage parents more directly, foster parental involvement in their children’s learning and communicate the importance of raising parental expectations for student learning.
There are few investigations, which have combined multiple non-cognitive and socio-familial variables when explaining academic performance, especially with at-risk students. The proposed model explained a considerable percentage of the variance of the academic performance of at-risk students, so it can lead to the development of educational strategies for the academic improvement of these students, which are those in a situation of greater academic vulnerability and, therefore, most likely to fail in their schooling.
The identification of variables of the students themselves that improve the academic performance of students at risk due to their family context is necessary to develop and implement projects for preventive intervention of school failure during secondary education. If the educational institutions can identify the socio-familial risk factors directly affecting students’ academic performance at an earlier stage, the chances of applying preventive strategies are highly increased. These strategies will be focused on counteracting the negative influence of their social and familial environments.