INTRODUCTION1
On August 29, 2019, two former leaders and peace negotiators of the demobilized Revolutionary Armed Forces of Colombia (FARC) -Iván Márquez and Jesús Santrich- announced their return to war and the creation of the dissident group Segunda Marquetalia (@pinzonoob, 2019). This came three years after signing a peace agreement that sought to end South America’s most protracted conflict. However, the dissidents do not have the military strength of the former FARC, as most ex-combatants are highly committed to the reintegration process (Pérez & Castrillón, 2021). Nevertheless, political opponents of the peace deal have used social media platforms to highlight the formation of dissident groups as a failure of the peace deal and to fuel controversy about Colombian politics (@MariaFdaCabal, 2019).
The inclusion of former guerrillas in politics was a controversial concession for many Colombians. Although overall the reintegration of former rebels into civil society is perceived as a notable achievement of the peace process, research suggests that the negative connotations of granting political concessions were a significant factor in Colombians voting against the peace referendum2 (Matanock & Garbiras-Díaz, 2018). Given that interactions on social media platforms can reflect and provoke emotions, and are key elements in the adoption of conflict management strategies (Duncombe, 2019), it could be argued that the political participation of ex-combatants remains a polarizing issue that hinders the reconciliation process in Colombian society, even in cyberspace. The Latin American Public Opinion Project survey, conducted in Colombia in 2018, showed high citizen support for several parts of the 2016 peace agreement related to rural development, especially agrarian reform. However, while citizen support for the political participation of ex-combatants remained low, only three out of ten respondents (29.1%) believed that political parties of former FARC combatants should have access to the same conditions of security and media coverage as other political parties (LAPOP, 2018). Moreover, this sentiment towards the political participation of ex-combatants did not change between 2016 and 2018, demonstrating the deep challenges of trying to get citizens to question their opinions on this issue.
Social media platforms have adopted soft moderation policies, also known as labeling, to reduce the polarizing effect of misleading, controversial, and unverified claims about content shared in cyberspace. Misinformation and related concerns exacerbate the tension between government agencies seeking to regulate a company, such as Twitter, and corporate policies implemented directly by social media platforms to address these issues. Several studies have examined the usefulness of employing soft moderation interventions when analyzing various topics, such as the Covid-19 pandemic (Roth & Pickles, 2020), the Russia-Ukraine war (Fischer, 2022), and the impact of misinformation in the US presidential election (Twitter Help Center, 2020). However, existing research on content labeling shows mixed results and omits post-conflict cases where we know of intense online polarization beyond conventionally peaceful democratic contexts. It remains unclear how effective tagging is in a post-conflict scenario. Therefore, this study aims to answer the question: How does the introduction of soft moderation labels on social media posts affect political polarization in peacebuilding contexts? We argue that in post-conflict societies, labeling controversial or unverified claims on Twitter has limited effects on changing users’ opinions about the political participation of former rebels.
We use an experimental research design analysis to test our argument in this exploratory study. We collected survey data in Colombia using convenience sampling and received 625 responses. Our aim is to understand the impact of common labels used by Twitter in the context of cyber peacebuilding, which allows us to capture the nuanced conditions that mediate interactions between users and political leaders when discussing the political participation of ex-rebels.
Furthermore, the type of content subject that Twitter labels can be divided into three main categories: misleading information—understood as claims that have been proven by experts or are misleading by experts; disputed claims—statements or opinions where the accuracy, truthfulness or credibility of the claims is disputed or unknown; and unverified claims - unconfirmed information that could be true or false (Roth & Pickles, 2020). When soft moderation interventions were first introduced, labels were included through an internal process in which the social media platform curated the lists and sources of the additional information that was provided to users (Matthews, 2020). More recently, with the adoption of the Civic Integrity Policy, Twitter is working with web checkers to train the platform’s algorithms to catch topics of widespread interest that may generate misleading information (@Twitter, 2022). Our study contributes to existing research by examining the potentially counterproductive effects of labeling in the context of cyber peacebuilding.
In the following section, we describe the gap in the literature regarding the impact of corporate policies adopted by Social Media platforms such as Twitter in cyber peacebuilding contexts. We then present our theory, highlighting the relevance of cyberpolitics as a tool for understanding the interaction between social media users and political leaders, which in turn can shape political attitudes in cyber peacebuilding contexts. In addition, we present our dataset and the experimental model used to measure the effects of labels on misinformation, disputed and unverified claims. Finally, we propose other explanations for why users reject the labels suggested by the algorithm, as well as other topics for further research.
LITERATURE REVIEW
The use of labeling strategies on Twitter has been tested in various political scenarios, but the academic literature on user interactions within a cyber peacebuilding framework is limited. Cyberdemocracy encompasses a range of theoretical approaches to the application of computer technology to democratic regimes (Ferdinand, 2003), and aims to understand how cyberspace can potentially disrupt political, economic, and social transformations. A key component of these transformations is the potential to avoid centralism and foster communities based on shared interests, and digital network communication enables this potential (Barth & Schlegelmilch, 2014; Kaiser et al., 2017). In sum, cyberdemocracy offers a new lens for understanding the relationship between the rulers and the ruled.
Social media interactions can both deepen democracies and exacerbate social conflict. On the one hand, social media interactions have facilitated collective action through micro-actions such as ‘likes’ small donations, and the joining of social causes through e-signatures that enable conditional cooperation (Margetts et al., 2015). On the other hand, social media interactions create cyberchallenges to state control (Choucri, 2012), reflecting the conflict processes and political representation issues inherent in democratic societies. In particular, interactions on social media platforms erode the power of the state by short-circuiting government control (Castells, 1999). Therefore labeling on social media platforms plays an important role in moderating potential sources of conflict and violence.
Labeling strategies as a soft moderation intervention on Twitter have primarily focused on clarifying misinformation related to the Covid-19 pandemic and the 2020 US presidential election. Research suggest that labeling has a limited effect on changing opinions or attitudes. Applying labels to controversial content appears to have little effect on typical readers of memes and news articles, most likely because many readers do not pay close enough attention to absorb the information on the label (Oeldorf-Hirsch et al., 2020). Furthermore, according to Kim and Walker (2020), labeling measures are not well suited to identifying emerging misinformation because they rely on intensive manual labeling or known sources of misinformation (i.e., domains, URLs, or accounts). A recent study by Sharevski et al. (2022) showed that the use of labels does not have a strong effect on changing the opinion of social media users on topics related to serious health issues such as the Covid-19 pandemic. The use of misinformation labels on Twitter related to the Covid-19 pandemic proved to reinforce the perceptions of pro-vaccine participants and backfire on vaccine sceptic participants.
In the context of American politics, soft moderation interventions such as warning labels have a limited effect on users. Studies show that there is a small effect of labels in mitigating misinformation, especially when social media platforms assign warning labels that are noticed (Nassetta & Gross, 2020). One study using a mixed methods analysis found that tweets with warning labels received more attention than tweets without them (Zannettou, 2021). The author offers two possible explanations for this phenomenon. One is that users are more influenced by their own political ideologies/biases than by Twitter’s warning labels in political discussions. On the other hand, perhaps the tweets that ended up with warnings were potentially harmful and received a lot of attention before the warning label was added. In addition, Green et al. (2020) conducted a study of elite cue polarization on Covid-19 using a dataset of tweets from members of the U.S. Congress between January 17, 2020, and March 31, 2020. The study reveals how such polarization can impede effective responses to public health crises, and highlights the important role of political elites in providing consistent and accurate cues. Finally, Papakyriakopoulos and Goodman (2022) show that warning labels do not affect how users interact with tweets, but they do reduce users’ tendency to create harmful content and increase the externalization of stances.
In the context of cyber peacebuilding, actions that delegitimize online violence, build capacity within society to peacefully manage online communication, and reduce vulnerability that can trigger online violence are critical (Chenou & Bonilla-Aranzales, 2022). Colombia is an appropriate case to test the effect of labels in a cyber peacebuilding context due to the ongoing peace process with the FARC, the existence of numerous accounts of political leaders from both ends of the political spectrum (DataReportal, 2022), and the popularity of Twitter among them. There is also an opportunity to fill a gap where existing research on the effect of social media interactions in Colombia omits the effect of soft moderation interventions on users’ attitudes.
In this experimental study, we argue that there is a lack of empirical research exploring the use of soft moderation interventions by social media platforms in Colombia. Initially, research on the Colombian cyberspace focused on the analysis of programs implemented by the Colombian government using Information and Communication technologies (ICT) to provide services to the general population. These programs considered issues related to the lack of access to the Internet and the psychological conditions at play in the relationship between the Colombian state and its citizens (Massal & Sandoval, 2010). Recently, there have been some studies on cyberpolitics that analyze the behavior of Colombian political parties and leaders on Twitter, with a specific focus on the 2018 presidential elections (Alvarado-Vivas, López, & Pedro-Carañana, 2020; Carreazo, 2020; Espinel & Rodríguez, 2019; Galvis et al., 2021; Manfredi & González-Sánchez, 2019; Ruano et al., 2018). However, most of the recent research has been framed within the context of the transitional period in Colombia.
In this regard, some studies fit into the cyberpeacebuilding framework, analyzing interactions between political leaders and regular social media users during key milestones. These milestones include the peacebuilding mechanism chosen to ratify the Colombian peace process in 2016 (Gallego et al., 2019; Nigam et al., 2017), the reactions and interactions around the emergence of a far-left dissident group in 2019 (Tabares Higuita, 2022), and the analysis of contentious politics reported on Twitter in 2019 (Rodríguez Rojas, 2020). Despite this recent research, none of these contributions has considered the effect of labeling in social media interactions through an experimental design approach, which is a relevant component for a comprehensive analysis of the political reintegration of former rebels in Colombian society.
THEORETICAL EXPECTATIONS
As discussed above, the existing literature suggests an effect, albeit limited, of soft moderation on online polarization. While there are few case studies on the effect of soft moderation in general and even fewer in highly polarized post-conflict societies, we expect to see a similar pattern in the Colombian case. Soft moderation interventions can provide users with information that may encourage them to think before commenting, sharing, or liking. As a result, the polarizing tone of social media discussions could be reduced, leading to improved deliberation. Furthermore, improved deliberation increases mutual understanding and trust, and strengthens democratic practices (Bächtiger et al., 2018).
Moreover, we acknowledge that social media interactions reflect specific elite cues that may influence the adoption of political actions in peacebuilding contexts. It can be argued that citizens’ views and opinions play a crucial role during the implementation phase of peace agreements, influencing how these actions are interpreted and implemented in political practice (Haass et al., 2022). These cues, which are clear signals or messages from trusted sources such as politicians, policy experts, interest groups, and journalists, can shape citizens’ opinions and guide their decision-making processes (Gilens & Murakawa, 2002). In the Colombian context, citizens would rely on signals from political elites to support specific provisions outlined in peace agreements (Garbiras-Díaz et al., 2021). Given the significant role of emotions in the spread of information on platforms such as Twitter (Duncombe, 2019), it is reasonable to expect that cues from political elites would be particularly important in the context of cyber peacebuilding.
This study examines the complex relationship between polarization, soft moderation, and democracy in the context of cyber peacebuilding. Deliberative democracy is the ideal definition of democracy that emphasizes the process of political debate, leading to the common interest through the exchange of arguments (Mansbridge et al., 2011). We argue that the concept of cyberpolitics captures several tensions that arise between the rulers and the ruled (Choucri, 2012). Social media platforms provide an opportunity for a constant and inclusive dialogue between citizens. However, they also tend to polarize and prevent deliberation (Sunstein, 2018). Therefore, the use of Twitter by political elites becomes a highly effective means of engaging the public audience, mainly through the transmission of elite cues. As a result, since citizens often rely on signals from political elites to shape their opinions on specific provisions of peace agreements, this study sheds light on the usefulness of soft moderation strategies adopted by social media companies. Thus, the Colombian context of cyber peacebuilding provides an ideal setting to examine the effectiveness of these soft moderation strategies in dealing with information about political concessions disseminated by elite cues.
In highly polarized post-conflict contexts in the Global North, the relationship between deliberation and democracy in the cybersphere has been analyzed (Steiner, 2012; Steiner et al., 2017). However, these studies have been limited in the Global South. In particular, Steiner et al. (2017) conducted a multi-site study of deliberation in highly polarized societies in Colombia, Bosnia and Herzegovina, and Brazilian favelas. They identified four types of deliberative transformative moments that reduce polarization and improve dialogue: the use of personal stories, rational arguments, humor, and silence. Although personal stories are beyond the scope of this study, rational argumentation, humor, and silence can be encouraged through gentle facilitation interventions. These specific actions can also encourage users to question whether a post is based on reason and facts, change the tone of the discussion by introducing humor, and question the need to intervene by sharing, replying, or commenting on a post. However, it is important to note that the study conducted by Steiner et al. (2017) was limited face-to-face interactions and may have a more limited impact on reducing polarization and improving dialogue on social media.
Similarly, it can be argued that the four types of deliberative transformative moments discussed above can be observed in the interactions between political leaders and social media users in cyber peacebuilding contexts. However, given the sheer volume of information and interactions shared on social media platforms, it is understandable that these platforms prioritize the implementation of standardized measures to assess the quality of shared information rather than tailor-made measures to enhance deliberative democratic processes. For example, the primary purpose of soft moderation interventions on Twitter is to provide users with additional context and information about specific content. While these labeling actions may prompt social media users to question the accuracy or credibility of the information, their aim is to promote critical thinking and informed decision-making rather than to actively shape public opinion (Roth & Pickles, 2020). In this context, the inclusion of labels in tweets posted by social media users emerges as a potential solution to address the challenges posed by misinformation and disinformation emanating from official state sources, news sources, and political figures in cyberspace.
The Colombian peace process aims to rebuild political trust and mutual understanding in order to resolve conflicts peacefully through democratic channels. However, social media interactions reveal the highly polarized context in which this process is taking place. The reintegration of ex-combatants into political and social life stands out as one of the most divisive issues. In the realm of cyber peacebuilding, characterized by multi-stakeholder governance and political stability, where social media companies act as both regulators and actors (Chenou & Bonilla-Aranzales, 2022), we argue that soft moderation interventions could have a limited but noticeable impact on promoting deliberation in the consolidation of the political transition. By introducing rationality, humor, and encouraging self-moderation in online discussions, these interventions could promote constructive dialogue. However, it is important to note that elite cues play a pivotal role in the implementation of peace agreements and support for certain provisions (Garbiras-Díaz et al., 2021). Consequently, citizens who are social media users may reject or ignore soft moderation interventions, perceiving them as platform-imposed intrusions that reinforce their existing beliefs.
We therefore propose the following hypotheses:
H1: Users engage with soft moderation interventions and do not perceive them as intrusive or aggressive.
H1a: Soft moderation labels have an effect on user behavior (Papakyriakopoulos & Goodman, 2022).
H1b: Soft moderation messages do not reinforce users’ polarized opinions, nor do they have a ‘backfire effect’ when they are not intrusive (Sharevski et al., 2022).
H2: Soft moderation is negatively associated with users reinforcing their original viewpoint and positively associated with users challenging their original viewpoint.
H2a: A label that refers to a trusted, authoritative and responsible source of information leads users to question their viewpoint (Chadwick et al., 2021).
H2b: A label suggesting further information on an issue leads users to question their original position.
H2c: A label that warns of dubious information and raises awareness of the consequences of engaging with dubious information leads to users to question their position and self-moderate.
H2d: A label that refers to a source of information that is notoriously based on humor leads users to question their viewpoint.
METHODOLOGY
This study used an exploratory approach, using convenience sampling to create a database of 625 emails, divided into a control group and four treatment groups.3 Participants were randomly assigned to one of these groups, and the experimental questionnaires were distributed to all participants in October 2021.
The questionnaires began by asking participants to provide demographic information about themselves, including age, gender, race, years of education, etc. Participants were also asked whether they agreed or disagreed with the following statement: “The political participation of ex-rebels contributes to peacebuilding in Colombia”. Participants who said they agreed were shown three hypothetical tweets from left-wing politicians about the peace process (Figures 1-3).
In contrast, participants who reported disagreeing with the proposed statement were shown three hypothetical tweets from right-wing politicians about the same process (Figures 4-6).
It is important to note that participants were informed that the tweet-like-texts presented to them were all hypothetical and could have been written by recognizable female politicians from Colombia, as they were written to closely resemble real statements made by each of the six politicians chosen for this study. The choice to use female politicians was deliberate in order to suppress any unconscious or unadvertised gender-bias. The tweet-like-texts presented to all participants were intended to represent elite cues to political concessions in a peacebuilding context. Accordingly, the content presented to participants reflected the asymmetries present in left/ right-wing political discourse and sought to remedy this potential problem by selecting equally prominent and well-known individuals from both sides of the aisle.
The use of plausible tweet-like-text by female politicians could have negative ethical implications, such as imitating and appropriating the ideas of public figures. However, this does not represent a significant increase in the participants’ baseline risk of everyday social media use.
Participants in the control group saw three tweet-like-texts from left- or right-wing politicians, depending on their stance on the political participation of former rebels in Colombia, without the addition of any soft moderation interventions. In contrast, the treatment groups were labeled to indicate the different types of interventions using Google-like surveys. Treatment 1 consisted of a label that directed participants to official documents from a trusted, authoritative, and responsible source of information—in this case, the United Nations (Figure 7).
Treatment 2 included a hyperlink inviting participants to “learn more about the topic,” referring to post-conflict and rebel reintegration into civil society (Figure 8).
In Treatment 3, participants were made aware of potentially misleading information (Figure 9).
In Treatment 4, participants were asked to watch a comedic interview with an emblematic politician from the opposite end of the spectrum. Thus, left-leaning participants were shown an interview with Álvaro Uribe—a recognizably right-wing politician—and right-leaning participants were shown an interview with Gustavo Petro—a well-known left-wing politician in Colombia (Figures 10 and 11).
Participants in the treatment groups were then asked additional questions, such as whether the labels made them feel uncomfortable and whether they would hypothetically click on the labels in a real-world situation. All 625 participants were asked whether the publications they were presented with reinforced or challenged their opinions about former rebels participating in Colombian politics. The questions were presented on a 1-5 Likert scale with the following possible responses: strongly disagree; disagree; neither agree nor disagree; agree and strongly agree.
To ensure transparency, efficiency, and to simulate a real social network scenario, participants were informed that their answers would be anonymous. In addition, the survey would end if participants answered “neither agree nor disagree” to the political characterization question, in order to limit the scope of the study to the dynamics of political polarization.
It is important to note that the way in which the treatments were allocated to participants has certain limitations that affect the study’s interpretation of the results and the external validity of the study. Although the treatments were randomly allocated, the groups were not balanced, which means that the demographic characteristics of the participants between groups were not statistically equal. Therefore, it is important to note that well-executed randomization in the assignment of treatment groups does not necessarily make the experimental groups equal or balanced (Mutz & Pemantle, 2012).
After data cleaning, the number of observations was reduced from 625 to 502, representing the correctly completed questionnaires with no missing values in the variables of interest. This means that more than one hundred observations had missing values on key covariates or answers to key questions that led to the selection of the two dependent variables, which will be expanded shortly. These variables would have no effect on the regression analysis, which allowed for them to be removed from the analysis without introducing any bias or altering the results. However, for various reasons not all of the 625 responses were complete, as participants did not complete all the surveys.
Table 1 provides a broad overview of the characteristics of the respondents. First, the first two variables in the table, which will be defined later as the dependent variables, show whether participants questioned or reinforced their initial opinions about the political participation by ex-rebels. On average, respondents do not seem to have questioned their initial opinions after receiving the treatments. However, more participants seem to have reinforced their initial opinions about the political participation of ex-combatants. The next five variables in Table 1 represent socio-demographic characteristics that were asked of the respondents ex-ante. The age variable divides the values into age groups (the sample ranges from 18 to 65+). On average, participants were mainly between 35 and 44 years old. The gender variable, where (1 is male and 0 is female), shows that on average the sample seems to be balanced between both genders. The next variable, which indicates the socio-demographic conditions of the participants, ranges from 1 to 6, the latter being the highest possible categorization. The mean of this variable suggests that the participants belong to the highest end of the socio-economic categorization spectrum. In addition, the next variable is designed to categorize participants as left or right on the political spectrum. On average, participants appear to lean left, as they tend to support the political participation of ex-rebels. Finally, the population variable indicates whether the respondents considered themselves to be disabled or to be part of the LGBTQ+ community or a racial minority. The mean of this variable, which is close to 0, indicates that the average respondent does not belong to any of these groups.
In order to determine whether any of the four treatments had an effect—i.e., whether they made participants reinforce or question their political opinion about former rebels participating in politics—the study used a difference in means and a Linear Probability Model (LPM) with two different versions. The dependent variables used were dummies that were equal to “1” if the participants’ perceived position was strengthened or challenged, and “0” when the opposite occurred (Figure 12).
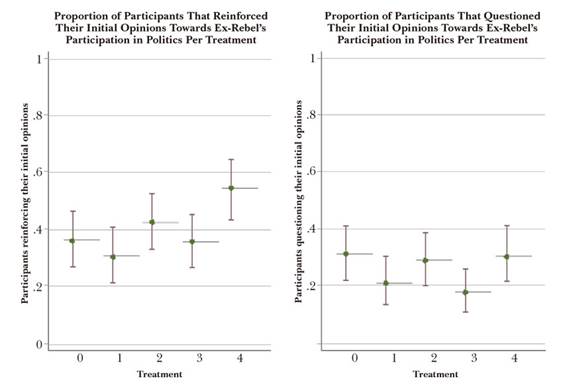
Figure 12 Mean and confidence intervals on whether participants questioned or reinforced their initial positions towards ex-rebel’s participation in politics
The difference in means was carried out using analysis of variance (ANOVA), which is used to test the null hypothesis that the mean of a variable is equal for, in this case, five different groups. The results show two different conclusions. First, when comparing whether participants reinforced their initial opinions per treatment, the p-value allows the null hypothesis to be rejected with 99% confidence. This means that between controls and treatment groups, there is statistical evidence to suggest that the group in which a respondent was assigned affects if they reinforce their initial opinions. The opposite occurred when analyzing if participants questioned their initial opinions towards ex-rebels’ participation in politics. The p-value suggests that the group in which a respondent was assigned does not affect whether they question their initial opinions or not.
This being said, models (1) and (2) were estimated using an LPM methodology:
Both models were estimated using the same methodology and dependent variables. The models included the respective treatment dummy variables relative to the control, a matrix of covariates and an error term defined as . The matrix covariates contained variables such as age, gender, years of education, socio-economic strata and participants’ opinions on the political participation of former rebels in Colombia. These control variables were chosen to isolate the effect of the treatment variables on the likelihood of participants either questioning or reinforcing their opinions. It is important to note that the sub-index (i minuscula) refers to each different respondent.
After running both Bonferroni and Chi-square tests on each of these variables, the treatment groups were found to be statistically unbalanced. In other words, after conducting these tests there was not enough statistical evidence to maintain that the group’s characteristics were equal. The null hypothesis for these tests implied that there were no significant differences in the characteristics of the control variables between the control and treatment groups, while the alternative hypothesis stated the opposite. The results of the chi-squared and the corresponding p-values allowed for the null hypothesis to be rejected most of the time. Only for populations and years of education could the null hypothesis not be rejected with a 90 or 95% confidence, confirming that the groups in this experiment were not balanced. Figure 13 provides visual representations of the results as the means and confidence intervals (95%) are not statistically the same, as some of them do not overlap between treatment groups. However, the addition of control variables allowed this problem to be corrected by isolating the effect of the variables of interest and providing a more reliable estimate.
Nevertheless, when controlling for the unbalanced groups, the appropriate method for interpreting the relationship between the treatments and the likelihood of either questioning or reinforcing one’s opinion is the LPM model, as it allows for an explanatory interpretation. This model simplifies the analysis and provides insight into the potential development of this study. In addition, both models predict that the probabilities of the dependent variables being equal to “1” fall between “0” and “1.”
Inverse Probability Weighting was used to account for group imbalances in the specified covariates. The addition of controls to isolate the effect of treatments on the dependent variables may not be sufficient. IPW assumes that Conditional Independence is achieved, i.e., that the treatment is not influenced by any unobserved cofounders, and was added to both LPM models (1) and (2). As the treatments were randomly assigned, this assumption was met (Huber, 2014). This means that we can identify the Average Treatment Effects (ATE) of our models while correcting for imbalances in the covariates (or controls) using the IPW.
Essentially, the goal of applying IPW to the regression analysis is to weight observations based on their probability of being treated given the cofounders—in this case, the aforementioned controls (Mansournia & Altman, 2016). This method weighs the observations based on their propensity scores, specifically the inverse probability of being assigned to any specific treatment group given the cofounders, in this case, the demographic information obtained from the participants prior to receiving the treatment. It is expected that this will reduce the bias of the treatment effects and provide a more reliable estimation on the coefficients of models (1) and (2).
Nevertheless, the use of IPW and the improvement of the group imbalances do not aim to eliminate the imbalance of the estimators and the ATE. Although the imbalance is reduced, the inherent problem of not being able to draw causal conclusions about the coefficients of the models remains. The use of IPW allows us to observe the difference in treatment effects after the groups are subject to an imbalance improvement.
RESULTS
After estimating both models, the coefficients show statistically significant results (Table 2).
Table 2 Linear Probability Model (LPM) estimating both models (1,2)15
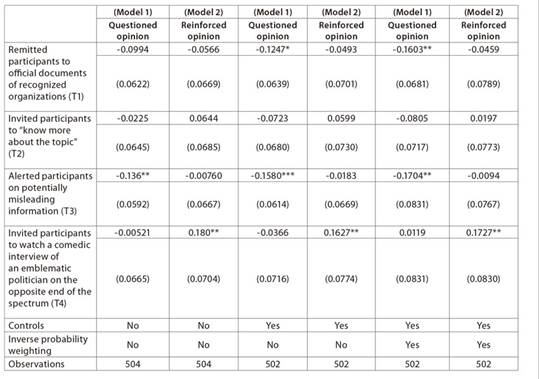
Robust standard errors in parentheses, *** p<0.01, ** p<0.05, * p<0.1
In Model 1, the coefficients of Treatment 3 are always statistically significant (95%). When the model is estimated without controls, Treatment 1 is not statistically significant, but its coefficient is significant at the 90% and 95% confidence intervals when estimated with controls and IPW, respectively. As the intention is to isolate the treatment effects, the addition of controls and/or IPW increases the magnitude of the coefficients. In particular, the coefficient changes from -9.9 percentage points (p.p.) to -16 p.p. from the model estimated without controls to the one with controls and IPW. This means that, in this case, in order to isolate the effects of the treatments on the dependent variable, the coefficients increase in size. This suggests that being in Treatment 1 with respect to the control increases the likelihood of participants not questioning their opinion compared to the control.. The coefficients of Treatment 3 are always significant at the 95% confidence level and increase in magnitude as controls and IPW are added to the estimation. The coefficients range from -13.6 p.p. to -17 p.p. This suggests that being in Treatment 3 relative to the control increases the probability of participants not questioning their opinions to a greater extent than in Treatment 1.
These results for Model 1 suggest the use of controls and IPW, with the aim of isolating the treatment effects and reducing the group imbalances, removes the noise affecting the treatment coefficients. The results suggest that the group imbalances absorbed the effect of the model on the dependent variable and therefore affected the treatment coefficients.
In Model 2, only Treatment 4 has a statistically significant effect at the 95% confidence level on the likelihood of participants reinforcing their opinions. All three estimations of Model 2 show significant coefficients for Treatment 4, which vary in magnitude. These coefficients show positive effects on the dependent variable: 18p.p, 16p.p and 17.3p.p in the estimations without and with controls and IPW, respectively. This means that being in Treatment 4 increases the probability of participants reinforcing their opinion compared to the control.
Estimates from Model 2 suggest that the group imbalances did not absorb the treatment effects on the dependent variable, as the significance and magnitude do not vary significantly across the different versions of the model.
Another important precision to make, is that in model (1) all treatment effects are statistically equal. On the other hand, on model (2) some treatment effects are statistically different from each other. In particular, treatment 4 is statistically different from treatment 3 and treatment 1 at a confidence level of more than 95%.
Another significant finding is the low proportion of participants in the treatment groups who said they would have clicked on the label presented to them is low. Figure 14 shows that these proportions never exceed 30% of participants in any group. However, the differences between the four groups are statistically significant meaning that the percentage of participants encouraged to click on the labels varied according to the treatment provided. The same cannot be said for the percentage of participants who reported feeling uncomfortable with the labels (detailed in Figure 14); there are no statistically significant differences in the proportions between the four treatment groups.
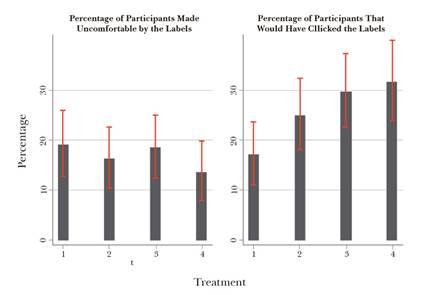
Source: own elaboration (2021).
Figure 14 Percentage of participants who would have clicked on the labels and felt uncomfortable by them in each of the four treatments
It is also worth noting that more than two-thirds of the treated participants read the labels that they were exposed to (Figure 15). However, around 82% of the treated participants reported that the labels did not make them feel uncomfortable. Furthermore, around 73% of these participants would not hypothetically click on the labels. Surprisingly, this suggests that even if most participants read the labels, they are not effective or useful in fulfilling their purpose.
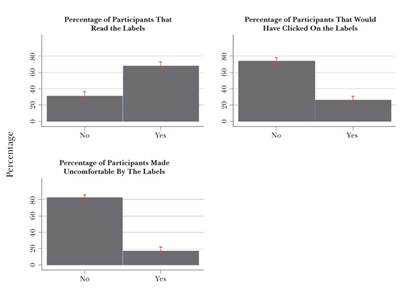
Source: own elaboration (2021).
Figure 15 Percentage of participants who would have clicked on the labels and felt uncomfortable with them overall
Table 3 Linear Probability Model (LPM) estimating both Model 2 using controls and conditional to four different cases16
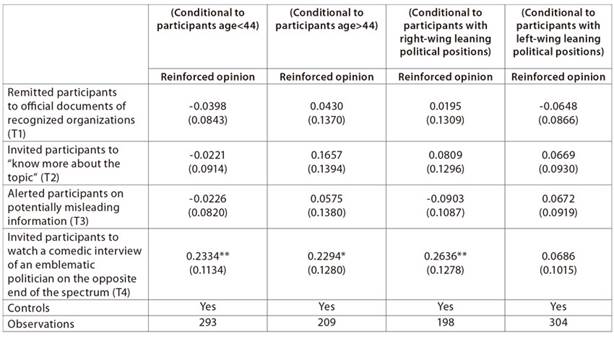
Robust standard errors in parentheses, *** p<0.01, ** p<0.05, * p<0.1
In order to explore the potential impact of age or political position on the likelihood of participants reinforcing their opinion, Model 2 was estimated and restricted to the sample conditions presented in Table 3. Surprisingly, the treatment coefficients showed signs of heterogeneity given the estimated sample. In the first sample, which includes participants younger than 44 years of age, Treatments 1 to 3 have a negative effect on the probability of participants reinforcing their opinion when compared to the control group. However, Treatment 4 is also significant, in line with the results presented in Table 1. In particular, Treatment 4 increased the likelihood of participants reinforcing their opinion for both samples.
Interestingly, the coefficient of Treatment 4 is statistically significant in the sample of right-wing leaning participants, but not in the sample of left-leaning participants. This suggests that the effectiveness of Treatment 4 is lost within the left-leaning group. Specifically, right-leaning participants in this group had a 26.4p.p probability of reinforcing their opinion, particularly with Treatment 4.
It is also important to note that, not all treatment effects are statistically equal in these four regressions. In the first three regressions, Treatment 4 is statistically different from Treatment 1 at a confidence level of more than 95%. Also, in the first and third regression output, Treatment 4 is statistically different from Treatment 3.
DISCUSSION
The results of the main survey question measuring political inclination show that 48.8% of participants believe that the political participation of ex-combatants contributes to peacebuilding in Colombia. This figure represents slightly less than half of the sample, which is similar to the results of the question posed in the October 2016 referendum in Colombia, “Do you support the final agreement to end the conflict and build a stable and lasting peace?” (Registraduría Nacional del Estado Civil, 2016).
There is a clear trend found in the results regarding the role of the state as a regulator and the duty of social media platforms to seek strategies to mitigate polarization around peacebuilding discussions. Of the 625 respondents, 66% felt that social media companies should seek strategies to mitigate polarization on their platforms around peacebuilding issues. However, 54.4% felt that the state should not regulate social media platforms to mitigate polarization on peacebuilding issues.
Contrary to expectations, a notable “backfire effect” was observed when users acknowledged the presence of soft moderation interventions, leading in one case to a statistically significant effect of reinforcing their opinion. In addition, the insertion of labels generally had a negative effect on users’ questioning their opinion, with statistically significant results observed for Treatment 1 and Treatment 3. Treatment 1 referred to an authoritative source of information, in this case, the United Nations. Contrary to what the literature shows on topics such as vaccine-related information (Chadwick et al., 2021), the reference to the United Nations appeared to have a negative effect on the potential for users to question their opinion about the political participation of ex-guerrilla members in Colombia. Such an outcome could be due to the public’s perception of the United Nations as a partial organization. While the Charter of the United Nations emphasizes its neutrality in internal conflicts, the reality of peacebuilding processes in post-conflict contexts, often generates conflicting perceptions among different actors (Bertram, 1995). A possible explanation to the reaction to the label referring to the United Nations as an authoritative source of information might be related to the existing perception of partiality by some sectors in the Colombian population. In this highly polarized context, identifying an authoritative source of information is a challenging task, as all actors are perceived as partial and possibly interested in the advancement of a particular agenda.
Treatment 3, the bluntest statement of all four soft moderation labels, also produced a statistically significant negative effect on users questioning their original position. It reads as follows: “Conoce por qué esta publicación podría ser engañosa” which can be translated as “Learn why this post could be misleading” (moderate interpretation) or “Learn why this post could be deceptive” (strong interpretation). The effect of this treatment could be explained by the prevalence of the strong interpretation of the label, and the fact that it was generally perceived as intrusive or even aggressive, making users less likely to challenge the original point of view. The content and design of soft moderation interventions are crucial, as the existing literature shows (Sharevski et al., 2022).
Another significant finding of the experiment is that Treatment 4 has the strongest statistically significant effect on users reinforcing their original opinion. This goes against the existing literature, which has shown that humor can trigger deliberation (Steiner et al., 2017). Two possible phenomena could explain this finding. On the one hand, Treatment 4 could have the same problem as Treatment 1. In this treatment, the comedian proposed as part of the label could be perceived as biased by some sectors of the Colombian population. Perhaps, the results of this experiment could also be influenced by the specificities of online interactions. Steiner et al. (2017) conducted their study in the field, which could mean that humor, in order to improve deliberation and reduce polarization, requires social cues that are only available in face-to-face interactions and are difficult to transfer to the cybersphere.
These exploratory findings have broader implications for the future of soft moderation by social media platforms in highly divided societies. While soft moderation seems to have a limited impact in more peaceful environments, it may not be the ideal solution in post-conflict and transitional societies. The involvement of all sectors in the design of content moderation practices is necessary to avoid rejection and the backfire effect. Indeed, soft moderation requires shared norms and a clear understanding of the issues at stake by social media users. It also requires institutions that are trusted to provide authoritative information. As a result, content moderation cannot be treated as a purely technical matter, nor can it be decided from above without public participation.
There is no one-size-fits-all solution to global content moderation. Debates in the Global North between social media platforms and the political sphere are too limited in scope to address the issues at stake in post-conflict and transitional societies. For example, while Twitter appears to have abandoned soft moderation under its new leadership, it remains a key strategy for combating hate speech, misinformation, and fake news on other platforms such as Meta, YouTube, and TikTok. Based on the exploratory experiment proposed in this inquiry, we argue that these efforts will not be sufficient to address the fundamental problem of communication in highly divided societies. Social media platforms offer unprecedented opportunities for inclusive deliberation, deepening democratic dynamics, and to consolidate peacebuilding processes. However, the issue of polarization needs to be addressed with the specificities of post-conflict societies in mind, in order to avoid exacerbating online manifestations of political polarization that may be expressed in an offline sphere.
CONCLUSIONS
Communication in cyberspace in highly divided societies, such as post-conflict Colombia, is a complex issue that requires consideration of the specific social and political context. The results of our exploratory experimental study suggest that soft moderation on social media platforms, such as Twitter, may have little impact on the opinions of those questioned.
The use of labels with authoritative sources of information or warnings about deceptive or misleading information did not lead participants to question their opinions about the political participation of former rebels in Colombia. This may have been due to the perceived bias of authoritative institutions such as the United Nations in highly divided countries. In addition, the use of labels with humorous sources of information backfired. Surprisingly, participants in this treatment group reinforced their original opinion. Furthermore, the results of this exploratory model show that only a small proportion of the participants would have clicked on the labels presented to them (which also did not seem to make the participants particularly uncomfortable).
These empirical findings based on the design and implementation of our exploratory experiment suggest that soft moderation interventions are ineffective in highly divided contexts such as Colombia. In some cases, labeling actions on social media platforms can have the opposite effect of what is expected based on existing research. We do not yet understand the particular conditions that mediate online communication in highly polarized, post-conflict societies. In this regard, state agencies and social media platforms need to carefully consider these conditions before implementing soft moderation interventions, as poorly designed interventions may exacerbate political polarization and undermine reconciliation processes.
Further research is needed to examine the effectiveness of soft moderation interventions on different social media platforms beyond Twitter, which updated its use of labels as a strategy to tackle misinformation in November 2022 (Capoot, 2022; Dale, 2022). However, other social media platforms such as Youtube, Facebook, and TikTok continue to use targeted soft moderation interventions as a fact-checking measure to address misinformation challenges around public health and electoral issues (Hutchinson, 2022; Kennan, 2022; META Oversight Board, 2022). A more controlled experimental design could help compare elite cues on social media platforms and test the effectiveness of soft moderation interventions, such as labels, on issues beyond those related to misinformation and propaganda promoted by state-controlled media sources in cyber peacebuilding contexts.