INTRODUCTION
Decision making in public policy is a process that requires understanding fast changing needs and complex contexts within populations of interest and where policy makers aim to assess the impact of public programmes. Impact evaluation as the field of evaluation approach that specifically deals with causal change (Bamberger et al., 2019, p. 63) has evolved as a method to ascertain the impact of alternatives on multiple outcomes. As a result, in the process of impact evaluation multiple methodological designs have emerged to empower the reasoning of social scientists and policy makers to better explain related changes. These designs may include high speed information driven by the expansion of the internet and big data.
The aim of this research is to present the contributions that specific methods derived from big data analytics (also BDA) have provided to a couple of evaluation designs in the context of sustainable development goals in Colombia as a case study. The main purpose is to track opportunities of integrating these BDA models in order to develop programme evaluation designs by examining their potential in improving methodologically weak conventional designs in summative evaluation. Methodological weakness refers to diverse limitations of current evaluation designs when judging hypotheses that assess the impact of a particular programme (Gertler et al., 2016a). We can ask for example if there is any added value in making use of these techniques to address risks such as wrong impressions of programme failure when programme effects are not statistically significant (Rossi et al., 2003b).
In the last few decades, impact evaluation has employed statistical tools, specifically econometric models to determine the internal validity of models using significance tests in econometric designs and random sampling. However, lack of statistical significance may be a consequence of poor-quality data among other data collection issues, and not necessarily weak designs in programme evaluation. Conflicts exist when programme designs are discarded under traditional statistical assessments because of low quality data but not because of poor quality designs. Therefore, BDA seems a promising field that attempts to overcome some of these issues to reflect pragmatic results and offer valuable feedback.
Current high-speed generation of information brings about a data revolution (Bamberger, 2016; Connelly et al., 2016) that offers an unprecedented opportunity to exploit useful data inputs that can alter the policy cycle as we know it nowadays (Poel et al., 2018). One of the assumptions introduced here is that policy decision making could be simplified to a more automated process given the terms provided by Information Communication Technologies (ICT) for collecting and analysing data in the task of understanding contexts in different and complex projects.
Despite the blurry definition of big data analytics as a concept it is expected to become a major innovation in impact assessment, as relevant and effective as any traditional data collection and analysis method (Task Team of the UN Global Working Group on Big Data, 2020). Trendy or not, new tools in BDA constitute a promising field to simplify the interpretation of findings in complex assessments involving big amounts of data. Tools provided by the field of big data analytics may help to better understand problems that are interconnected, not only within a specific sector but within a multi-sector approach (Rodríguez et al., 2017). Moreover, diverse contributions are practicable to different types of analyses including prescriptive, descriptive and predictive designs where the aim is to determine how data generated from new sources of information may offer renovated insights to provide better informed decisions.
This document incorporates an empirical overview of the adoption of the big data initiative in Colombia, which was selected as a case study since it is a country leading this sort of initiatives in the region, including those for programme evaluation purposes within the framework of the UN 2030 Agenda for Sustainable Development. The Colombian context is also a good example to get an idea on how government institutions still lack proper mechanisms for collecting and analysing data with the aim of monitoring sustainable development goals (SDGs).
This research is expected to contribute to the scope of knowledge regarding the subject, and also in the fields of digital era governance and evidence-based decision making in public policy. The interest here is to present smart illustrations as an empirical exercise to evidence how these 'enhanced methods' for evaluation design could be applied to programmes in the Colombian context. The target behind this idea is to conduct a comparison of programme design and data collection strategies where these big data methodologies may have an advantage over conventional evaluation designs such as statistical models, econometric techniques, hypothesis testing and so on.
The method here is expected to allow the research to present the contributions that BDA can make in the evaluation of SDG programmes in Colombia as a policy field of application. The design of impact indicators is expected to improve in programmes that use easily measurable variables, and where there is a clear relation as to how input variables can achieve intended outcome(s) aligned with SDGs (United Nations, 2020). Results may vary based on new and smart methods for evidence-based decisions in the evaluation of development programmes to strengthening the preciseness of indices that assess the external validity of pilot programmes, thus avoiding wrong impressions of programme failure. Furthermore, this article intends to present brief insights concerning decision making based on pragmatic evidence to discern overoptimistic assumptions regarding BDA applications in the public sector.
CONCEPTUAL DISCUSSION
Impact evaluation as a field of study comprises multiple alternative methods that are used simultaneously in other phases of the policy cycle such as programme design and policy formulation. Also called impact assessment or outcome evaluation, impact evaluation posits whether or not a programme produces the intended outcomes in the social environment it addresses (Rossi et al., 2003a, p. 58). It has become a requirement for determining whether the value of an intervention is expedient when addressing the specific needs of a community or target group. This section presents a literature review in order to understand the most widespread concepts related to the subject with the aim of selecting evidence-based methods in designing programme evaluation where BDA is expected to make relevant contributions. Different theories that have emerged in the last few decades are considered.
Few evaluation approaches explained
The literature concerning impact evaluation defines several types of evaluation that can be identified as follows: I. policy and programme evaluation, II. formative evaluation, III. developmental evaluation, IV. summative evaluation (Bamberger, 2016). Nevertheless, and based on the aim of this article, the focus here is on the latter category which is centred mostly in causality (Bamberger et al., 2016) and where two types of evaluation design are normally considered: experimental and predictive analytics.
Experimental & quasi-experimental designs
These models of evaluation usually contrast over time the effects of a programme -also called an intervention- on a treatment group or recipients compared to a control group, which is normally denoted as the counterfactual or theoretically equivalent group in the remaining conditions other than the intervention. The pure experimental design is a case where the equivalence is given by chance, and these models are normally strong in terms of internal validity (Bryman, 2012). However, because of the rare availability of pure experimental designs in real life conditions some alternative constructions are utilised, despite not being a hundred percent randomised such as the so-called quasi-experimental versions. Generally, all designs define one or several outcome variables according to the intervention purpose.
Accepted methods in the community of impact evaluation include Randomised Control Trials (RCT). These designs are based on counterfactual analyses (Bamberger et al., 2019) built in random assignments. They are said to be strong models for avoiding issues such as selection bias.
Statistical designs
Multiple statistical methods are used in social research and impact evaluation namely regression discontinuity and multivariate statistical models that attempt to predict values with regard to the outcome variables or hypothetical values, if there is no possibility of finding appropriate counterfactuals or control groups. On the other hand, there are the so-called reflexive designs such as time-series models where target groups are compared with themselves at different points of time in order to assess causality trends. Lastly, propensity score matching strives to find a similar unit in the group of non-participants or counterfactual -as it has been called here- based on observed characteristics (Gertler et al., 2016a, p. 194).
In general, the preferred method is the one that best fits the operational context and requires the weakest assumptions and the least data (Gertler et al., 2016a). These criteria normally determine the suitability of a specific evaluation design. It is worth noting that the applicability of each design will depend on the specific programme outcome, aside from the questions that policy makers intend to respond to.
Programme evaluation and its main challenges
The typical question for evaluators in public policy concerns the effect of a programme on an outcome of interest and whether the magnitude of that effect can be attributed to the programme. Under statistical methods this is examined by means of testing whether there is any significant change in the outcome of interest provided by the intervention. In developing assessments, the designs mentioned above have contributed to providing solutions and universal knowledge regarding how to deal with complex programme assessment in many countries and contexts. Nevertheless, common limitations of classic statistical methods in impact evaluation include type I and type II errors in significance tests and the statistical power of an impact evaluation (Bamberger, 2016; Gertler et al., 2016b). The issue behind these limitations is that multiple contextual factors and the non-linear patterns of causality affect the design and implementation. 'These factors seriously challenge the validity of conventional evaluation designs that assume a linear relationship between programme inputs and outcomes' (Bamberger, 2016, p.54).
The three most frequent evaluation challenges that all evaluations must address are: design, data collection and data analysis (Bamberger, 2016). A research gap worth studying could be the limitations that econometric designs and other statistical significance tests face regarding external validity assumptions. This added to the difficulty of collecting and analysing aggregate data from existing datasets covering long periods of time as in the case of time series. Further, there exists the risk of bias and more specifically selection bias that occurs when the criteria for selecting the individual is correlated to the outcomes of the programme (Gertler et al., 2016c, p. 59). Take RCT for instance, which incidentally is the evaluation approach most widely criticised by big data analysts (Bamberger, 2016). It also contains various limitations, such as low external validity when the counterfactual is designed for a specific scenario and ignores processes between causes and effects (Bamberger et al., 2016, p. 68). Limitations also extend in terms of interpretation which is a key fact in complex programmes that involve large numbers of stakeholders and unclear connections between them.
The advent of big data analytics (BDA) as an alternative
BDA may contribute to minimising limitations in terms of design related shortfalls by means of extreme intuitive or experimental analyses that lack more evidence formulated processes (Escobar Gutiérrez et al., 2021). Incorporating big data into existing programme evaluation designs is clearly not a straightforward task. Only consider the theoretical difficulty of the definition of what big data means as a concept. Multiple definitions still complex and ambiguous are suggested. However, one characteristic that can be attributed to big data is that it is not normally collected for social research purposes but rather re-purposed by social research scientists as an evidence-based method for policymaking (Connelly et al., 2016, p. 2).
Big data is the term widely used to describe vast amounts of information and variables and can be understood as 'high-volume, high-velocity and/or high-variety information assets that demand cost-effective forms of information processing that enable enhanced insight, decision-making, and process automation' (Gartner as cited in Karlsson et al., 2019, paragraph 10). Also, the World Bank presents big data as 'the term used to describe constantly increasing flows of data emitted from connected individuals and things, as well as a new generation of approaches being used to deliver insight and value from these data flows' (The World Bank, 2016, p.72). Big data may also refer to the identification of technological challenges due to the generation of data, its volume and velocity as well as a variety of outtake traditional systems (Laney, 2001, as cited in CONPES, 2018, p.27).
Volume, velocity and variety are the three most relevant attributes when attempting to comprise the definition of big data (Laney, 2001; Laney, 2012, as cited in AAPOR Big Data Task Force, 2015, p.8; European Commission Directorate General for Informatics, 2016, p.14). Firstly, volume refers to the amount of data available for analysis. Secondly, velocity defines the speed associated to data generation, collection processes and how this data moves around. Thirdly, variety refers to the diverse types of data we rely on nowadays. Other definitions also include attributes such value, veracity and viability which normally refer to the accuracy, feasibility and trustworthiness of the data (European Commission Directorate General for Informatics, 2016, p.16; Vaca & Vega, 2019). Large sets of digital information available could be also considered in multiple types of data, such as structured data, semi-structured data and unstructured data. Predefined patterns or formats in which information is presented will determine these types accordingly (CONPES, 2018). In connection with these definitions, Bamberger and York (2020) propose a classification of big data in three categories depending on how it is generated and used, these include human-generated (centred), administrative (transactional) data and geospatial data.
On the other hand, data analytics may be understood as assisting procedures that analyse large volumes of information. The World Bank (2016, p.72) defines this as 'the emerging set of tools and methods to manage and analyse the explosive growth of digital information. It includes visualisation, machine learning techniques and algorithms.' In a pragmatic perspective, analytics is a term which many different methods and approaches converge to, including statistics, data mining, business intelligence, decision support systems and others (Tsoukias et al., 2013, p. 123). Data analytics can be a discipline aimed at analysing data using different automated techniques to extract relations, pattern correlations and tendencies in order to interpret phenomena that otherwise would be difficult to analyse in large sets of data (CONPES, 2018).
In recent times, analytics has had high acceptance in policy decision-making as another type of evidence, because of the growing demand for accountable and up-to-date methods to vindicate decisions. However, the vast amount of this information is normally unstructured and not one, but several processing methodologies must be applied. Specific typologies exist for analysing different formats namely text, audio, video, social media, among others. Following the European Commission Directorate General for Informatics (2016, p.22), in a very abstract way the most considered types of data analytics are the following four:
Descriptive: This represents main features and trends of past events by means of data visualisation tools.
Diagnostic: Methods such as correlation analyses, which try to understand relations between variables and subsequent events.
Predictive: Models such as time series, based on historical patterns to forecast future events and the likelihood that these may happen.
Prescriptive: This type includes multiple approaches to provide solutions or 'optimal sequences' helping to make complex decision processes or to automate repetitive tasks.
Applicability of BDA in programme evaluation design
A new paradigm has emerged between data scientists and evaluators if one understands that traditional impact evaluation methods are normally 'backward looking' and rely mostly on ex-post analyses. These designs assess situations of the past trying to understand the causes and effects of public policies which have been implemented (Tsoukias et al., 2013). Traditional evaluation relies on experimental and quasi experimental designs (Bamberger, 2016, p.62). In contrast, big data analytics tends to be mostly predictive or 'forward looking' (Bamberger, 2016, p.46). Questions concern comprehending whether the purpose is to predict an occurrence rather than understanding causality or vice versa, and data analytics may fulfil multiple functions for evaluators, either predictive or prescriptive.
The exponential digitalisation has made data-driven policymaking a popular trend among social scientists, particularly statisticians, economists and public managers. Big data becomes especially attractive because of the features described above, but particularly because of real-time and high velocity information that offers the opportunity to have more continuous evaluation processes (Olsson & Bull-Berg, 2015). There are several forms in which big data analytics can modernise programme evaluation design: by strengthening conventional models, enhancing indicators and integrating larger amounts of data never considered by traditional techniques (Bamberger, 2016). One cannot deny that there is a huge trend in social sciences for evidence-based approaches and BDA can be considered as one of those experiments that policymakers are still testing (Poel et al., 2018), and who seek to overcome situations such as biased estimations or wrong impressions of programme performance.
Organisations such as the United Nations and the European Union have started to adopt smart evaluation methodologies based on the resources provided by BDA in order to direct more impactful policies based on evidence and well informed decisions (European Commission Directorate General for Informatics, 2016, p.9). Moreover, international experiences in countries such as Australia and the United Kingdom convey a lot regarding the success of BDA as a methodology for improving abilities in measuring programme impact (Australian Department of Industry, Innovation and Science, 2018; UK Department for Digital, Culture, Media & Sport, 2020).
How can big data analytics lead to more assertive evaluation designs? This question does not have one single answer, because predicting results of an evaluation design could be translated into anticipated decision-making processes. For example, when it comes to predictive analytics there are many interesting opportunities such as simulating policy implementations thus predicting potential results and foreseeing better evaluation designs. The data analytics approach allows governments to focus on prevention rather than to simply react and remediate existing troublesome conditions in an environment (European Commission Directorate General for Informatics, 2016, p.12). When it comes to specific policy fields, The Big Data UN Global Working Group (2015) presents an interesting approach that describes how BDA could be used to monitor SDG indicators integrating data originated from new technologies with traditional data generated by government agencies qualified for monitoring sustainable development (The Task Team of the UN Global Working Group on Big Data, 2020).
On the other hand, for BDA to be useful across the policy cycle -and particularly to the exercise of impact evaluation- basic conditions such as those within many other designs must be fulfiled. For instance, the cleanliness of data, in other words, the format with regards to how these information sets are collected and presented. Note that the term analytics also describes the meaning the researcher is assigning to information assets according to the goal of his analysis. Therefore, the usefulness of the information will depend upon the context of the programme and of course the formulation of its outcomes. On the other hand, the information collected by these procedures must encircle features of average individuals in the group of interest. Also, many other factors such as measurability and availability are indeed important to contribute to purposeful assessments.
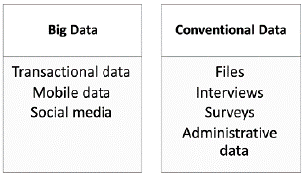
Source: Developed by the author.
Figure 3 Several types of big data and conventional evaluation data
Take for example the Coronavirus (COVID-19) Information Centre of Facebook and the survey systems available on its platform (Facebook, 2020). Users can easily report up-to-date information here related to possible symptoms and flows of people across the globe to track the spread of the virus. Another example also presented in Annex 1 of this article refers to The Crop Survey Data of United Nations Office on Drugs and Crime (UNODC, 2020). BDA is currently supporting the assessment of programmes such as this one where the collection of data is costly, incomplete, and even risky if using traditional data collection methods such as surveys or statistical inference exercises. In this example, analysis of patterns in applications such as Google maps took place to assist policy makers in identifying updated trends in either the eradication or extension of illicit crops in remote places of Colombia.
Table 4 Some potential contributions of BDA to standard evaluation methods
Challenge | Contributions of BDA |
---|---|
Selection bias | Strengthening counterfactual designs, new algorithms for randomisation processes. |
External/ internal validity | Better contextualisation of causalities and external validation of results. Provides triangulation as a source for verification. |
Data collection issues | Simplifies collection processes for long periods of data records in the case of time series analyses. Faster availability, greater coverage than the traditional cross-sectional, time-series or panel data models. |
Statistical significance tests | Refined indicators with up-to-date data. |
Interpretation for stakeholders | Simpler and more participative results to legitimate decisions. Richer descriptive analyses. |
Source: Developed by the author.
Decision makers should not have the same excuses as they did twenty years ago when it was more difficult to collect data than it is nowadays. However, this does not mean that current approaches for designing public policy evaluation do not have their own complications and the same issues as usual, nevertheless the methods to cope with these issues have changed to become less time consuming and more cost-effective. In this regard, BDA in particular is seen by many experts as an attractive alternative, and can reinforce specified knowledge in situations in favour of stronger programmes and policy design (The World Bank, 2014, p.30).
On the other hand, it is crucial to point out that similar to any other experiment, new methods always incorporate uncertainty and risk. Another virtue of BDA modelling is to simulate potential scenarios when implementing certain programmes without altering real life conditions. However, there may be important drawbacks in these models brought about by BDA, particularly spurious correlation and incidental endogeneity. These are situations that occur when many uncor-related random variables present high level correlations amongst themselves and with the residual noise, aside from noise accumulation effects which are systematic aggregations of estimation errors that may threaten the validity of models such as regressions (Fan et al., 2014, p. 298).
Programme evaluation design is also at the core of this context, where experts and decision makers are able to minimise uncertainty when attempting to make efficient decisions that exploit the potential of a given programme aimed to fulfil any SDG. BDA may be a powerful engine to help generate evidence for the evaluation of specific policies, which in turn will support governments in providing better services for citizens (Rodríguez et al., 2017, p. 16). Public value intelligence is a concept presented backing this idea, and can be defined as a smart way of consuming big data in order to measure and overcome previously invisible and unsolvable issues associated mainly to data quality and accessibility (Rodríguez et al., 2017, p. 8).
Case study overview
Rich in many aspects such as natural and cultural traditions, Colombia is like most developing countries one that still struggles in terms of poverty, inequality, unemployment and many other 'wicked' problems. As with other policy processes, diverse stakeholders of the Colombian society are involved in the adoption of big data analytics into the design of public policy evaluation. Different points of view must be considered to justify the big data initiative in programme evaluation. The purpose of reviewing these perspectives is to bring into consideration potential alliances among multiple sectors in building data exploitation capacities and conducting joint projects based on BDA under the current institutional framework (Escobar Gutiérrez et al., 2021, p. 86). Partnerships with strategic stakeholders is an essential requirement for the successful implementation of this policy that supports multiple initiatives, including those aimed at the SDGs (Letouzé et al., 2016, p. 34).
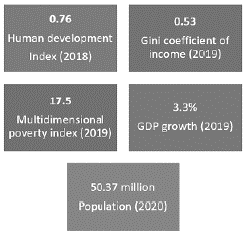
Source: Adapted from United Nations Development Programme (2021).
Figure 4 Main social indicators for Colombia
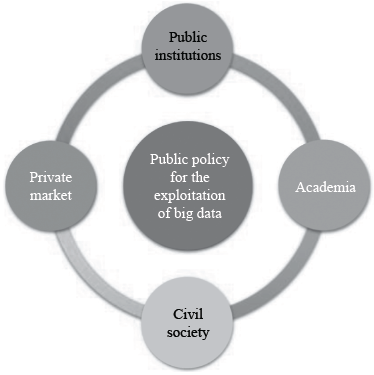
Source: Developed by the author.
Figure 5 Multiple stakeholders involved in the Colombian policy of big data
Concerning the institutional framework, one could mention Colombian institutions such as the National Planning Department (hereinafter DNP) as the institution in charge when it comes to providing the guidelines for public policy design, implementation and evaluation in Colombia. Also, in promoting initiatives such the public policy for the exploitation of big data. Other bodies, namely the Ministry of ICT, and the National Department of Statistics (DANE) play a crucial role as well when coordinating efforts in this matter. Moreover, one must not forget international organisations that have been important supporters of these types of initiatives dealing with big data for programme evaluation purposes, for instance the United Nations Statistic Division and the UN Global Working Group on Big Data. These organisations have supported the realisation of events such as the 4th International Conference on Big Data for Official Statistics in Bogotá Colombia (2017).
Big data analytics for sustainable development goals in Colombia
The rapid development of digital technologies has indeed wrought new sources of information for official statistics. Colombia has been ranked in third place after Korea and France when it comes to the OURData Index1 2019 (OECD, 2020).
Moreover, Karlsson et al. (2019) present an overview of how BDA has been used as an innovative solution for problems faced by most Latin American countries, regarding programme design, implementation and evaluation. Colombia became the eighth country worldwide and the first in Latin America in establishing a policy to enable conditions for the adoption of BDA in the public sector (Escobar Gutiérrez et al., 2021, p. 28).
The policy field of this document is focused on SDG programmes and a survey presented by the World Bank and the Big Data UN Global Working Group to illustrate how BDA has been used to enable actions when designing and monitoring indicators in projects aimed at SDGs (Escobar Gutiérrez et al., 2021; The Task Team of the United Nations Global Working Group on Big Data, 2020). To name a concrete example, the DANE jointly with NASA have conducted workshops on the use of data analytics regarding Earth Observation for SDGs related to the environment (The Global Partnership for Sustainable Development Data, 2017). Colombia is also the first country in Latin America to adopt a public policy framework to enable social and economic development based on the exploitation of big data (Departamento Nacional de Planeación [DNP], 2018). This framework includes private partnerships and non-government organisations to leverage the first ever national big data strategy in the country (Innpulsa Colombia, MIT Media Lab & Data Pop Alliance, 2020).
Nevertheless, public intervention is indeed required to provide the conditions for a gainful and responsible exploitation of data, to overcome government failures and legal concerns that may threaten the benefits these new sources of information may represent for evaluation purposes. Likewise, as it is the case with many other public services, there is no supply created by the market to distribute the added value of public big data sets to stakeholders, as this can happen only within the private sector. A major issue that one could anticipate in this regard is the limitation in terms of internet penetration levels as in the case of Colombia. Approximately only 60% of the households have any internet connection either fixed or mobile solutions (DANE, 2019, p. 12). Web-based measures still risk not being representative, but as internet penetration continues to grow, non-representativeness becomes less troublesome (Letouzé et al., 2016, p. 29). Despite these shortfalls, it is still interesting to describe how big data sources form part of an enormous digital transformation taking place in Colombia over the last decades.
METHODOLOGY AND DATA COLLECTION APPROACHES
Specific public programmes are presented as a way of illustration to frame the contributions that BDA may provide to programme evaluation design in Colombia. This exercise also intends to map specific and relevant trends of BDA applications in evaluation design under the context of SDGs. A qualitative analysis allows this research to establish a conceptual assessment in the explanation of BDA contributions. The goal here is to build upon concepts that rapid technological improvements of BDA as an emerging phenomenon have brought to public policy evaluation processes as a research topic. The process presents a literature review in a documentary analysis to provide researchers new ideas regarding relevant applications of BDA to conduct meaningful policy analyses. This review relied mainly on scientific articles and publications on topics closely related to BDA, programme evaluation and management. The literature on big data analysed here provided quite useful definitions and clear explanations of current trends and applicability to programme evaluation designs.
As in a practice of triangulation the empirical section of this research relies also on interviews to contrast the documentary analysis results in a way to better explore the applications of BDA in SDG assessment in Colombia, and analyse how the use of BDA may strengthen typical evaluation designs such as the ones presented in section 'Few evaluation approaches explained'. The philosophy behind this idea is to analyse projects that assess outcomes using BDA typologies described in section 'The advent of big data analytics (BDA) as an alternative'. The presumption here is that this reflection will strengthen monitoring systems connected to SDGs, as well as complementing official data sources (Vaca & Vega, 2019).
Similar to other exploratory studies such as Olsson and Bull-Berg (2015) the empirical approach of this research incorporates semi-structured interviews. The best way to obtain in-depth insight in exploratory research like this is by interviewing people involved in the field of expertise (Sekaran & Bougie, 2010; Myers, n.d., as cited in Urquhart, 2017). With this argument in mind qualitative interviewing was prepared to encounter experts on the subject2. This exercise aims to count alternative viewpoints regarding the themes that frame the interview model which is presented in annex 3 at the end of this document. The operationalisation of this process replicates a method set forth by Björkman and Franco (2017) consisting of exploratory questions with the goal of getting a broader interpretation of the topic.
Figure 6 shows the steps proposed under this methodology.
RESULTS
Documentary analysis
Colombia is a country that provides multiple examples of pilot programmes that have incorporated BDA to design evaluation models for SDGs related projects. In examining these programmes several conditions were considered: first, only verifiable publications mostly from public institutions, official documents and projects supported by the Colombian Government were selected. Second, the aim, policy field, origin of data and BDA typology adopted were also considered in the classification phase. The review evidenced that descriptive and predictive designs, as well as propensity score matching were employed in these attempts. Annex 1 displays an overview of the studies analysed in the literature review to inform the reader with respect to this exercise. The BDA typologies employed here are the ones described in section 'The advent of big data analytics (BDA) as an alternative'.
This summary allows to present the reader ongoing pilots led by diverse organisations in Colombia and present applications of BDA typologies ranging from social media to satellite imaging in social projects connected to SDGs. However, the interpretation should be one confirming that most of these interventions have adopted BDA methods mostly for data collection or programme design purposes rather than for impact assessment. In other words, most of these programmes rely on BDA to enhance their informational sources rather than to construct new evaluation methodologies to measure programme impact. Few exceptions exist such as the Colombian programme for monitoring coca crops. For that specific example, satellite imagery was taken to contrast the number of hectares of land used for illicit crops after implementing antidrug policies (UNODC, 2020) in a pre-post comparison design. The results here present some advantages of BDA methods mostly related to data collection simplification. One can easily ascertain that large amounts of information are rapidly organised when compared to standard methodologies such as field surveys. However, in terms of validity standards and selection bias, BDA per se will not guarantee the necessary conditions for overcoming related limitations. Further research on this is being considered on both conceptual and empirical levels.
Finally, these illustrations synthetise this review concluding that there is no awareness, or at least too little knowledge regarding the potential of BDA models to assess outcomes in these programmes. Unfortunately, Colombia does not yet have a policy framework to employ these BDA models in evaluation designs. To confirm our expectations, it would have been necessary to have an explicit methodology in data analytics assessing the changes in outcome indicators in these examples.
Interview results
Six interviews were conducted for the data collection strategy of this research, all of them with experts in data analytics and public policy from institutions such as DNP3. The aim of this process was to reveal applications of BDA that are relevant and realistic to programme evaluation with potential for monitoring SDGs. At the operational level open ended questions guided the conversation to promote a richer discussion. Codifying the themes of the interviews with topic questions4 was the way to evidence extreme points of view in the spectrum of answers provided by the participants. The method to document the results relied on broad descriptions that use anonymised extended verbatim extracts and pattern analysis typically considered in qualitative research (Jensen & Laurie, 2016, p. 279).
Many organisations have data that may be purposeful, but it remains unexploited. Policies should be based on evidence to allocate the budget for those programmes with higher expected impacts and to create links between public organisations. All the information collected should be in principle aimed at improving public policies. On the other hand, Colombia is perceived as a leader in the region and one of the first countries to have a public policy related to big data. 'We have been following good practices in other places such as the UK, the EU, Korea and Australia' is what two interviewees mentioned.
In terms of the types of analysis conducted with BDA examples include descriptive studies and designing interactive dashboards for users. Depending on the maturity level of the project, the organisation and data management, the more sophisticated the analyses were. One of the interviewees claimed: 'I can mention that in machine learning we considered decision trees, Natural Language Processing (NLP), data clouds, neuronal networks, statistical inference and time series.'
When the interviewees were asked if they considered that data analytics could be useful to assess the impact of public programmes most of them agreed that big data makes more data available to the organisations, hence they are less dependent on official statistics such as surveys. BDA complements and broadens the richness from the analytics perspective and strengthens the design of policies to allow better focalisation and characterisations of populations and social problems. Tools such as real-time data analyses allow to make more timely and realistic decisions; data analytics also allows to identify bottlenecks in the execution of programmes.
BDA may be considered as well to assess SDG achievement in examining how much the National Government and local governments are aligned with the SDGs based on their own investment plans although there is no existing framework to articulate data analytics in the monitoring and evaluation of SDG indicators, nor to allow the provision of feedback in the formulation of investment policies. Big data analyses can contribute to the measurement of one or more SDGs.
Within the organisations the interviewees wrought several changes in the process of decision making after the adoption of BDA took place when evidence in the form of data helped to make informed decisions. However, the main challenges of using BDA in the context of public programme assessment were interoperability limitations, together with data quality and management. Other challenges were the uncertainty levels regarding data usage and data protection issues. When asked about prospects and examples they could provide for SDG oriented programmes using BDA most interviewees indicated that the articulation of data analytics for monitoring SDGs is still a pending task, and this can even function to realise the perception of measures taken by governments.
As a general result it can be claimed based on these extracts that Colombia turns to be a revelatory case study able to illustrate what was anticipated in the introductory part of this document. Colombia is making major progress in incorporating BDA in the public sector mostly due to its policies regarding open and big data. It was relatively easy to demonstrate that BDA was applied mostly for descriptive purposes, however the opposite was true for prescriptive analyses and particularly difficult for predictive studies given the technical and ethical reasons explained by the experts interviewed.
On the other hand, the confirmation of BDA typologies was not entirely verifiable as a methodology to simplify decision-making processes related to the assessment of the programmes examined here, as there was no direct evidence found for this. Nevertheless, the formulation and implementation phases were enhanced. One can ensure that policy makers rely on more sophisticated tools based on data evidence and improved evaluation criterion to guide decision-making processes. The risks of any wrong judgements of programme failure can be reduced based on a richer body of evidence. The empirical exercise conducted here, both in the literature review and qualitative interviews supports the idea that BDA supplements but does not substitute typical evaluation approaches in terms of evidence-based decision making.
All in all, these initiatives need to align key stakeholders in these expectations that incorporate BDA. Apparently, maturity levels and other variables such as solid data management procedures and a transparent, ethical and legal framework are required to manage these sources of information to serve the institutions of the Colombian Government. Practically all interviewees agreed that interoperability, legal and organisational challenges are issues that must be overtaken to allow multiple public organisations to cooperate with each other to potentialise and refine the use of BDA in programme evaluation. In this regard, Colombia still has many tasks in building a methodological framework to fully articulate data analytics in the monitoring of SDGs. On the other hand, BDA can produce new insights in re-using administrative data already existent in many government offices as informational assets for smart evaluation designs, also less dependent on traditional data.
Nonetheless and as explained before, at the decision-making stage these sources serve as input but are almost never considered to automatise decision making tasks. In addition, personnel training is still lacking and specifically in top-level management due to the unrealistic expectations still provoked by these procedures. Furthermore, and critically important is to consider data protection standards aimed at protecting citizens' privacy rights and personal data.
CONCLUSION
This document so far has intended to answer the research question concerning the contributions that big data analytics can provide to assess programmes for sustainable development goals in Colombia. A qualitative exploratory study was conducted using a literature review and semi-structured qualitative interviews were carried out to present main trends regarding the multiple applications of BDA taking place within the Colombian context. One of the main ideas presented here as a justification to leverage BDA in providing solutions is the existing shortfalls of standard designs in programme evaluation.
However, one must acknowledge that in a country like Colombia there is still the need for certain conditions to reach an adequate maturity level for these initiatives to materialise. As this research has presented in prior sections, there are still issues related to interoperability, legal framework and information quality that require concentrated attention from the researchers and policy makers. This is also summarised in the Colombian big data policy, which also highlights the need for having high quality digital data as a condition for creating economic and social value in an appropriate legal framework (CONPES, 2018, p.28).
On the other hand, one of the main purposes of the analysis was to develop theory revealing situations where BDA has demonstrated not just to be a rich approach for the evaluation models selected here, but also revealing a lot regarding the phenomena these programmes addressed, specifically in terms of SDGs. Even though not all the pilot projects considered in this research presented BDA a suitable tool for assessing the effectiveness of government initiatives. It is important to under stand however, that given the scope of this research, external replication is possible only if made cautiously, this because of specific contexts, data availability and the intervention itself which are crucial elements for any external validation purposes.
The results allow me to present BDA to the reader as a practice that includes multiple benefits for the case study specified in this research including better informed decision processes for SDGs that complement official data sources and better monitoring of financial efforts aimed at SDGs. Beyond this research the next step is to promote the use of BDA in evaluation design contexts, specifically policy development. The philosophy behind this is that extreme intuitive or experimental analyses should be more the exception than the rule, we must formulate more evidence-proven sources.
The theoretical integration proposed in this article between programme impact evaluation and big data analytics has strived for establishing a reliable and valid emergent theory. What this means is that in the context of programme assessment BDA could work well in triangulation processes that are normally undertaken to overcome reliability issues. When it comes to validity most authors analysed in the literature review converged in the idea that the main contribution of BDA in impact evaluation design is to potentialise specific assessment methods (i.e., randomised control trials, score matching, regression discontinuity), rather than to completely become a new paradigm in policy evaluation theory.
Although presenting new possibilities for programme assessment, there is a high risk of failing in unnecessary or undesirable complications when dealing with big data. BDA is neither recognised as a scientific approach in impact assessment (Poel et al., 2018) nor it is certain if it will become scientifically accepted in economic research (Einav & Levin, 2013). Just because it is 'in fashion' to design social experiments for evidence-based decisions with sophisticated or trendy techniques such as BDA this does not mean there are no more complications. Bias for example is one of the main concerns for programme evaluators and BDA is not the exception for this, since these big datasets may deal with higher bias risks due to the sources they originate from or for whom this data have been designed without scientific validity (Poel et al., 2018).
Big data is not a panacea but a tool to strengthen evaluation design procedures if one correctly understands its constant evolution as well as its limitations discussed throughout this document. More data does not mean more information, and hidden biases might exist in both the collection and analysis stages (Crawford, 2013). Likewise, there are methodological constraints regarding the distinction between predictive modelling and causal inference when researchers use methods such as machine learning statistical tools (Einav & Levin, 2013, p.16).
On the other hand, there are still large informational gaps even using BDA, especially for communities which still do not have access to internet connections and digital information, or simply are reluctant to use them. Furthermore, data privacy management and legal constraints are also an issue that dominates the discussion among the scientific community when dealing with alternatives such as BDA. Other drawbacks may refer to the high dimensionality of big data, also computational challenges such as scalability and storage bottleneck (Fan et al., 2014). High sophisticated computational methods and high trained professionals are much required.
Finally, this research has presented innovative ways for interested parties to interpret complex data outputs with the use of BDA techniques, providing insights on how to overcome the interpretation of complex results. Not just lack of information but also poor interpretations can limit proper measurement of the effectiveness of public programmes, as well as any other challenges such as budget and expertise constraints.