Introduction
Research based on microblogs and models of socially constructed knowledge analyzes the quality of the content generated, the dissemination of information, decision-making, and collective intelligence, among others. This article focuses on determining the meaning of cacerolazo according to frames given to it by messages published on Twitter by digital media and Ecuadorian citizens during the protests of October 2019. This specific case will allow us to apply data science to the analysis of social networks, to measure public opinion in Latin America in the current communication context. Since data science allows collecting and analyzing large volumes of data produced in real time by users in their interactions (González 2019), it is particularly well suited to study social media data.
In particular, the analysis of the dynamics of communication in the context of political crises (Aragón et al. 2013) has become a focus of exploration on Twitter. The study of this type of data is used to determine government political communication strategies, detect trends, and identify the polarization of discourse in the public arena, among others (Ardini and Nahúm Mirad 2020). Although social media signals might have a weak link to public opinion as a whole, they can activate powerful possibilities for social support and influence public perceptions (Cabrera et al. 2021).
For the community of political communication researchers, Twitter is an appropriate platform to study collective protest practices (Reilly et al. 2017). The immediacy and multi-directionality of messages facilitate a rapid and spontaneous citizen reaction. It provides a space for a rapid and wide dissemination of messages while allowing for real-time engagement (Caldevilla et al. 2021). Therefore, it is a resource of “mass self-call” on the web (Castells 2007).
To determine the meaning of cacerolazo in a protest context, we will first present the case study and the state-of-the-art on the subject. Then, we will explain the methodology used to extract and analyze tweets to identify framings given to the word “cacerolazo” in the context of the 2019 protests in Ecuador. To conclude, we will discuss the obtained results and reasons for future research.
Case Study: Ecuadorian Protests in Twitter and Cacerolazo
On October 1, 2019, President Lenín Moreno did a nationwide broadcast to announce a set of economic adjustments. Moreno informed the Ecuadorians about the economic regulations contained in the Executive Decree 883. The decree ordered the elimination of fuel subsidies, some fiscal and labor measures, the reduction of vacations for public employees, the contribution of one day of monthly salary to the state by public employees, an exceptional contribution by big companies, and, as a compensation measure, an increase of bonuses given to the most impoverished families. Initially, the decree provoked protest in the transport sector, which declared a national strike. However, other social movements rapidly joined the protest. These demonstrations prompted the government to issue a state of emergency decree throughout the country. Despite efforts to contain the protests, on October 3, 2019, the government suspended classes in schools and colleges, and since October 7, after 124 years, Guayaquil once again became the seat of government.
Ten days after the beginning of the carriers’ strike and having convened a dialogue table between the government and the protesters, a day of protests took place in Quito and Guayaquil on October 12, 2019. It was led by citizens not belonging to the collectives in conflict and took the form of a cacerolazo convened mainly by social media. The occasion gave rise to a double interpretation of the meaning of cacerolazo by mass media. Either it was a protest against the government, or it was a request to the contending parties to engage in a dialogue for peace.
This second interpretation prevailed in the media, which favored the government’s point of view and was consistent with a tacit alliance between Moreno and the mainstream media. Indeed, unlike the confrontational relationship of the previous Correa administration with media, Moreno reformed the Communication Law, eliminated the Superintendence of Communication (Supercom), and made a positive change in the government’s relationship with media. For the Committee to Protect Journalists (CPJ), Moreno’s government sought an openness to dialogue with the media, unlike Correa’s government (Radio Atalaya 2018).
Nevertheless, the interpretation according to which cacerolazos are protests against governments has a more solid history. This form of protest was born in Chile against President Salvador Allende in the 1970s and had an important milestone in the 2001 crisis in Argentina. In these cases, mainstream media tended to take the side of the protest when it was against a leftist government, as was also the case in Argentina during the 2012 cacerolazos against Cristina Kirchner. An immediate antecedent in this country were the protests of April 2005, during the political crisis that culminated in the ousting of President Lucio Gutiérrez.
Different is the case of protests during a liberal government, such as the one in Ecuador, with a pre-existing alliance with the media. In 2019, the interpretation of cacerolazo by the media is ambiguous: they support it, but do not consider it primarily oriented against the government.
Social media were involved in this debate on the interpretation of the meaning of cacerolazo. In several cases, they expressed the idea that the media were distorting the meaning by claiming that the motive of the cacerolazo was to ask for peace and not, according to its historical anchor, to protest against the government. This is evidenced in the following tweets: “#Cacerolazo #CacerolazoContraElPaquetazo no a favor de la paz por si acaso (not for peace, just in case) @teleamazonasec @ecuavisa”; “@tctelevision el #Cacerolazo no fue por la paz. Las personas salimos a las calles a protestar contra las medidas neoliberales del señor @Lenin…Como periodistas primero investiguen” (it was not for peace. People took to the streets to protest against the neoliberal measures of Mr. @Lenin...As journalists you should first investigate); “#FueraLeninFuera el #cacerolazo siempre ha sido símbolo de descontento e indignación!!! No es paz, es indignación!!!” (the #cacerolazo has always been a symbol of discontent and indignation!!! It is not peace, it is indignation!!!).
Thirteen days after the protests started, the government and the CONAIE (an indigenous movement) initiated a dialogue mediated by the Ecuadorian Episcopal Conference and the representative of the United Nations (UN). These conversations led to the abolition of Decree 883 by the Executive and the cessation of demonstrations (El Universo 2019c). The balance of the protests was “1,192 people detained, of whom 96% were men and 4% women. The highest number of people detained was registered in Pichincha (532), followed by Guayas (310) and Tungurahua (59)” (Defensoría del Pueblo 2019).
Literature review
Hybrid media system
The first theoretical approach to our subject focuses on the concept of mediatization. It refers to the relationship between the media system and other social systems at each stage of its history, as diverse cultural practices-such as politics-change in the presence of the media (Verón 1994, 2001). Thus, mediatization expresses socialization processes produced by the media (Escudero Chauvel 2018) and, more generally, their intervention in the construction of social reality. The concept of mediatization seeks to account for the relationship between historical changes in the media and other processes of social transformation (Hepp 2012).
Nowadays, conventional media and native Internet media coexist in a hybrid media system (Chadwick 2013), which results from the interactions between old and new media and their technologies, genres, norms, behaviors, and partner organizations. Both types of media operate on large technological platforms. Platforms are infrastructures, predominantly digital, that allow two or more groups to interact. They are intermediaries between different users, comprise a series of tools that allow them to build their products, services, or transaction spaces, and have an infrastructure to detect, record, and analyze data (Srnicek 2017). Digital media operate within platforms, such as Google, Facebook, Instagram, or YouTube, and are themselves platforms. In this second sense, Scolari states that a media platform is a complex multimodal system of discursive exchanges, where all the codes known until now coexist and whose influence reaches, in some sense, every aspect of social life (2019).
Among the significant changes introduced by the Internet for the world of content, the universalization of consumption stands out, which is described (Verón 2013) as the “access revolution.” A transmedia reading (Albarello 2019) of news within the convergence culture (Jenkins 2008) implies that audiences consume news from multiple sources, on diverse platforms, and through different devices. This consumption behavior emerges from different information sources, audience fragmentation, and circulation expansion. Even though audiences no longer share the same media space, there are testimonies of how the media increasingly promote affective expressions of the public at times of historical changes in a country. Affective audiences involved in the debate tell their own stories and still mobilize in public space (Papacharissi 2015).
In his classic model, Wolton (1989) considers public opinion as an actor in political communication alongside politicians and the media. The mediatization inherent to a hybrid media system forces us to rethink the way in which citizens exchange opinions today, the weight of social media in the framing of problems, and the emergence of publics digitally engaged in social protests. The formation of public opinion also takes place within platforms.
2.2. Framing and social media
The decision of users to share or ignore a content, or comment and edit contents to produce their own contribution constitutes a “collective conformation of news frames.” Indeed, “by coordinating their preferences, these users gain structure” (Calvo and Aruguete 2020, 15).
Mass media, digital media, and users propose interpretative frames for events in the current digital media context. However, this concept has been transferred to sociology, understanding the frame as the principle of organization of a social experience (Goffman 1974). Bateson (1972) contributes a notion about the frame based on psychology that conceptualizes it as an element of the psyche that allows us to demarcate distinctions and attend to certain aspects of reality while ignoring others.
The application of frames in media studies began by analyzing the resources employed by these privileged enunciators to organize social reality from their position of hegemony (Gitlin 1980). According to Koziner (2013), by using frames, journalists decide what is news and what is not, and assign cognitive categories to facts. Indeed, these operations tend to serve the political definitions of the elites; Koziner (2013) synthesizes those main definitions in the field of media studies.
Entman (1993) states that framing is “to select some aspects of a perceived reality and make them more salient in a communicating text, in such a way as to promote a particular problem definition, causal interpretation, moral evaluation, and/or treatment recommendation” (52). For Tankard (2001), a frame is “a central organizing idea of news content that supplies a context and suggests what the issue is through the use of selection, emphasis, exclusion, and elaboration” (11). Finally, Reese et al. (2001) characterized frames as socially shared and persistent over time that work symbolically to meaningfully structure the social world (5).
In a hybrid media system, in addition to the frames proposed by the media, the framing mechanisms employed by the users of different platforms must also be considered. The distinctive aspect of framing in social media is “cascade activation.” This feature is significant given “the process by which we allow content to which we agree to appear on our contacts’ walls.” Then, by doing so, “we modify the frequency and speed with which these contents circulate” (Calvo and Aruguete 2020, 10). There are different aspects of the content that each frame highlights and relates to for each news event. “The framing elements converge on the users’ walls and provoke an interpretation, assessment or solution that supports their alignment with some users and justifies their opposition with others” (Calvo and Aruguete 2020, 10).
2.3. Twitter and network mobilizations
In 2019, there were multiple sources of collective reaction in the world and especially in Latin America. Lago (2015) argues that collectives and social movements re-appropriated the symbolic space to intervene in the dominant imaginary and grant new meanings, which are meanings and images from communication practices articulated in networks. For Castells (2007), the protests respond to various causes, including social, ecological, and political problems. In recent years, collective protests have taken over Twitter and have given way to an audience that amplifies the demands and citizen discontent. It has become an effective platform for social convening and mobilization (Gaffney 2010; Lotan et al. 2011).
Some examples from the last decade are the Arab Spring, where Twitter was a key space for logistics among MENA citizens (Chaudhry 2014; Eltantawy and Wiest 2011; Lotan et al. 2011). There are also other cases such as Occupy Gezi in Turkey, Indignados in Spain, Occupy Wall Street in the United States, and #YoSoy132 in Mexico.
There are multiple studies on the subject, such as García et al. (2014), who studied Chilean student protests and identified that the protest’s centrality changed the actors, and the patterns of behavior on the network were different in each case. In the case of “Spanish Revolution” on Twitter, Gualda et al. (2015) identified the importance of hashtags to generate connections with other collectives in the network.
Another study conducted by Said-Hung and Valencia-Cobos (2017) examines the use of the hashtags #SOSVenezuela and #PrayForVenezuela in mobilizations during the first quarter of 2014. These hashtags allowed monitoring the protests due to the censorship suffered by newspapers in that country.
Objectives
The general objective of the article is to explore the capacity of Twitter users to activate frames of political events with autonomy from those activated by the media. To do so, it is necessary to use data science methods in the study, which make possible to control and analyze the rapid formation of public opinion in the context of current mediatization and during social protests.
Specifically, it seeks to describe the framing produced by Twitter users of a critical moment of the social protest in Ecuador in October 2019. The Ecuadorian cacerolazo is of interest because the media interpreted its meaning in two contradictory ways: a protest against the government or a request for the cessation of protests. The following hypothesis proposes to clarify the meaning of cacerolazo; the hypothesis will be tested by analyzing the data extracted from Twitter during the case study.
H1: During the 2019 strike in Ecuador, the media and social networks activated different frames about the cacerolazo.
H1a: The framework proposed by Twitter for the cacerolazo differs from that proposed by the media.
H1b: The meaning of cacerolazo is interpreted oppositely by two frames within Twitter publications; one of them criticizes the interpretation of the meaning of cacerolazo proposed by the media.
Methodology
In this section, we present the main tasks that were implemented to i) identify, label, and validate both the Twitter accounts and hashtags to use them in our analysis and provide a general view; and ii) extract and configure online media news to narrow the analysis in the frames of cacerolazo during the protest. The approach consists of:
Dataset definition and characterization. The process to get valid tweets for our analysis is based on Ecuador Trending Topics that emerged during the protests.
Hashtags model and tweets clustering. Using word2vec (Mikolov et al. 2013), we find a vector representation for the words used in the selected tweets of our dataset. Then, a strategy is employed to define tweets clusters and reveal how different audiences used relevant hashtags.
Frames identification and cacerolazo case study. Extract cacerolazo-related news using the Google News service. The selected newspapers are those named in the tweets during the protest.
The following subsections will present the details of our approach considering each task.
4.1. Dataset definition and characterization
This section describes the strategy used to obtain the dataset for the study. The tweets extraction process and their exploration are based on the following components.
a. “Trending topics” identification
Twitter includes a list of trending topics in the application as part of its user experience.1 To filter the topics, location, city, or country may be customized by the user in their profile. The trends listed by Twitter are nouns, hashtags, or noun phrases that are popular in the network in that specific moment.2 Our study collected the trending topics in Ecuador using the Twitter API (Application Programming Interface, available to software developers). Requests to the API run from October 5 to October 21, 2020. Among the topics collected during the national strike, only those related to the Ecuadorian social and political scene at that moment were chosen and tracked. The advice of two experts in political communication with knowledge of the context was sought to manually select the relevant trending topics related to the protest and mobilizations, to filter thus the rest. Finally, 54 trending topics were chosen (See Table A1 in the Appendix); for example, #cacerolazo (or pot banging), #EcuadorEnResistencia (or Ecuador in resistance), #lafuerzadelpueblo (or the strength of the people), Decreto 883 (or Decree 883), and #paronacionalec (or national strike). As explained the next section, the 54 topics were employed as keywords to extract the tweets.
b. Tweets collection
The Twitter API allows researchers to search and collect the latest tweets that contain a particular keyword or search query. We employed the selected list of trending topics to query the API and extract tweets and their related data, such as tweet id, text, hashtags (#), mentions (@), URLs, post date/time, the user who created or retweeted the tweet, geolocation, language, among other tweet-related data. The extraction process was run for each trending topic. As a result, 54 datasets of tweets and retweets with their related data were collected.
Finally, the dataset for our study was built from the union of the datasets mentioned before. To do so, we i) carefully removed the duplicates, since the possibility existed that a tweet containing two trending topics appear in two of the collected datasets, and ii) filtered out retweets to work with the created posts and avoid reproduced content (which also implies duplicity). The definitive protest dataset contained 130,382 tweets.
c. Dataset characterization in the context of protests
To develop our research questions and evaluate the methods proposed in this article, we started with a descriptive analysis that depicts the details and behavior of the collected data. As it may be inferred, the extracted tweets contain hashtags that appeared as trending topics. The content creators tend to include more than one hashtag to provide their posts with a folksonomy (Laniado and Mika 2010). Therefore, after detecting and organizing the hashtags found in the tweets, we created a list that presents the hashtags and their occurrence frequency. Table 1 details the top ten popular hashtags.
During dataset exploration, we classified the users’ accounts considering their activity regarding the number of tweets they published. Table 2 shows a list of the 20 most active users and the type of accounts defined as Media, Twitter user, Political party, and University. The relevant role of media is clear during the protests.
To conclude the dataset description, we created a directed graph with weights (Bang-Jensen and Gutin 2008), having the user account as the source node, the hashtag (used at least ten times by the user) as the destined node, and the frequency of hashtag use as the weight (Recalde et al. 2019). This example presents the users who employed the listed hashtags more than 100 times. The tools used in this step were NetworkX (a library of Python to create and manage graphs by programming means) and Gephi for the visual representation of the graph. Gephi can read graphs generated by NetworkX and represent them visually, i.e., colors and sizes to represent nodes. Indeed, Figure 1 was modeled by using Gephi. The figure shows an example where #paronacionalec (or national strike) accumulated more usage given the solid green color, and the official account @ecuainm employed more hashtags (source of three arrows).
Table 2. The twenty most active Twitter accounts according to the number of tweets posted
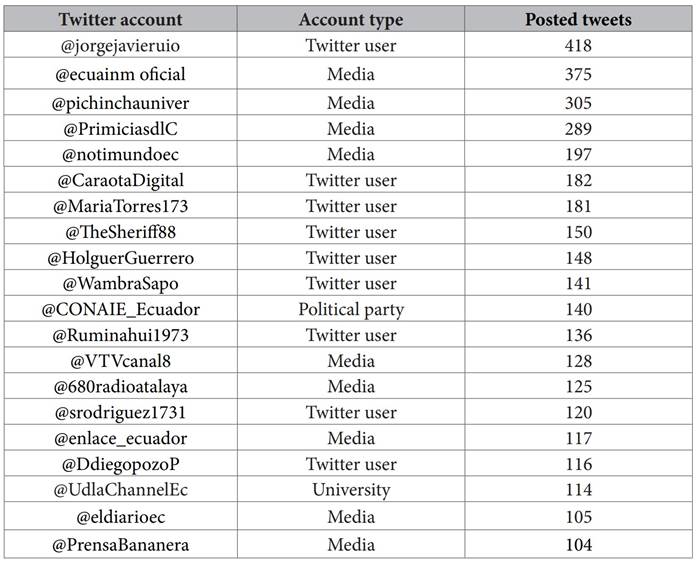
Source: Own elaboration.
4.2. Hashtags model and tweets clustering
This section presents the method used to clean the tweets that belong to the study dataset to later generate tweets clusters. This pre-processing phase is required to get clean training data and to obtain a formal representation (vector model) for the words that appear in our tweets. The method has been used to cluster the tweets. The clusters analysis is helpful to study the behaviors of different actors (and groups of actors) and the purpose of the mobilizations. This method consisted of four phases:
Tweet pre-processing. The content of the tweets was pre-processed to remove characters that would make the process of knowledge extraction from the tweets ambiguous. This cleaning step was needed for the next step.
Text modeling. The dataset of clean tweets was used to create a trained model with word2vec.3 The model generates a (vector) representation of the main words of the tweet corpus. This model was used to define clusters of words (including hashtags).
Vocabulary clustering. The classification of the words that are part of the corpus vocabulary was conducted by employing their representation model. Generally, the analysis of the clusters allows determining whether there is a polarization of groups.
Tweets clustering. This step allowed grouping the tweets whose content was against or in favor of the government.
What follows is a detailed explanation of how these tasks have been implemented.
a. Tweet pre-processing
To be parsed, the text of the tweets must be pre-processed. For example, punctuation or prepositions found in a document do not provide any meaning or context; therefore, they must be removed. In this phase, we took the following actions:
Capital letters conversion into lower-case letters.
Special letters conversion. Vowels with accents and special characters, such as ‘ñ,’ ‘á,’ ‘é’ (part of Spanish grammar), took the form of ‘n,’ ‘a,’ ‘e,’ respectively.
Spanish stop words, such as ‘yo’ (‘I’), ‘de’ (‘of’), ‘en’ (‘in’), and punctuation marks were removed.
Tokenization of tweets to recognize mentions, URLs, hashtags, and emoticons in the vocabulary. In other words, in this phase, each tweet was separated into its words.
After cleaning, the number of tweets obtained was 119,756 (out of a total of 130,382). This process also means that tweets made up of non-words such as “hahahahaha!” were removed once the pre-processing method was applied. The cleaned dataset now called training corpus is employed in the next section.
b. Text modeling
In our method, the neural language model word2vec is used. Its purpose is to create a vector representation of a given word, where words with similar meanings have similar (mathematical) representations. The model generated is known as word embeddings and extracts syntactic information from a text corpus, independently of the language used.
Word embeddings can give the vector representation of a word depending on the context in which it is commonly used (semantic sensitivity). Consequently, through the application of operations, it is possible to determine whether two words have been used in the same context or, for example, how far one hashtag is from another. Indeed, the operations may say that hashtag #protestsEC is similar to hashtag #protestsEcuador. Some of the authors of this article applied word2vec in another setting (Recalde et al. 2017). This contribution showed that the neural model was useful to identify hashtags that were hijacked in tweets collected during another protest in Ecuador during 2016.
For this scenario, the model needs to be primarily trained by learning the words and their context given by the training corpus’s text. The training corpus contains the tweets dataset once it is cleaned up; then, it is used as input for the model. After using word2vec, the vocabulary size (words taken from tweets in the training dataset) represented as vectors were 10,753.
To get an overview of the quality of the model, we took some of the main hashtags (to avoid making data visualization too cluttered) and other words that can be used naturally in the same context (city names and synonyms of the word protest), and we evaluated how word2vec places them in a two-dimensional vector space (Figure 2). As can be seen in the figure, the words related to dialogue and peace are close to each other. The same behavior shows that the words that promote resistance and the exit of the government are grouped, as well as words about the cacerolazo.
c. Vocabulary clustering
Part of our approach is to validate whether the words and hashtags used (vocabulary) are polarized in our model. Word2vec creates semantically related words in the provided corpus; therefore, groups of words in their given context can be assigned efficiently. To group words, word2vec clustering needs the corpus and the desired number of clusters as input parameters. Therefore, we decided to group the vocabulary into three clusters: i) neutral, ii) against the government, and iii) in favor of the government.
Once the number of clusters is defined as a parameter, word2vec clustering is executed. The information describing the clusters is presented in Table 3. After building the model and finding the clusters, it is possible to identify the clusters given the set of assigned words to each one. For example, in Cluster 0, general words like police, military, today, protest, Quito, people, among others, were found. Words denoting opposition to the government were in Cluster 1: repression, fight, dead, terrorists, violence, strength, among others. On the other hand, words that describe support to the government, such as dialog, government, president, together, country, peace, among others, were found in Cluster 2.
As our analysis is intended to define how different protest actors used hashtags, we applied this method to group “words” into clusters and tweets. Then, we calculated the clusters’ centroids. The centroid may be considered a general and average representation of the context in the cluster and provides a point of reference to determine whether other elements in the space (words, tweets, users) are near or far from it. After building the vocabulary clusters and finding the set of cluster centroids (means), we can assign the tweets to their corresponding cluster. Accordingly, the next section describes the steps to obtain the vector representation of tweets to cluster them later.
d. Tweets clustering
In our method, it is necessary to identify the centroids of clusters (previous section) because it is a baseline for the further classification of tweets. Therefore, given a tweet, we sum up the vectors of the words that it contains to calculate the tweet vector. Later, considering our three centroids that correspond to the clusters, we can measure how similar a given tweet is to different clusters.
The resulting values ranged from 0 to 1, with 0 or close to 0 for the least similar vectors and 1 or close to 1 for the most similar pair of vectors. In this phase, we classified each tweet as belonging to the corresponding cluster if they had the maximum similarity. Thus, the tweets in the training dataset were assigned to one of the three clusters. Table 4 shows a sample of six tweets and the cluster ID to which they were assigned.
We evaluated the tweet clustering method by manually labeling 100 random tweets with the actual class (neutral, against the government, and in favor of the government) and then compared them to the clustering result. We obtained an accuracy of 86% for tweet clustering; it is worth mentioning that some of the misclassified observations were due to the presence of irony in the tweet. For instance, the post “When in your life have you seen that a #cacerolazo is in favor of the government?” was classified as “in favor of the government” by the clustering method. However, as it shows sarcasm, the correct class is “against the government.”
This phase aimed to validate whether, during the protests, the groups of actors adopted specific hashtags to represent their opinion and political stance. To do so, we first created a heat map to visualize the use of a particular hashtag given another one. In other words, the heat map in Figure A2 (in the Appendix) was employed to see the relevance of a combination of two hashtags and how often they appear together in the same tweet. The selection of relevant hashtags, according to Figure A2, was used to determine their usage distribution among the three clusters. Then, we identified how many tweets containing hashtags belong to Cluster 0, how many to Cluster 1, and how many to Cluster 2.
After this analysis, the manual hashtag selection of interest was simplified because, according to this figure, the frequency of their appearance showed a context of their use and a starting point of polarization exploration.
For instance, we took the hashtags in Table 5. The table presents the number of tweets with the given hashtag that were classified as part of Cluster 0, 1, and 2. It is observed that 92% of the population using the hashtag #GetOutLenin belongs to Cluster 1. During the analysis, it was labeled as “against the government” or, in turn, “supporting the protests.” Something similar occurs with the hashtag #GetOutMoreno because 96% of the tweets that contained it were classified as belonging to Cluster 1. Indeed, it is not surprising, given that #GetOutLenin and #GetOutMoreno are synonyms. On the other hand, #DialogueForPeace, #DialogueAndPeace, and #DialogueIsTheWay were used by people supporting government stability (Cluster 2).
4.3. The “cacerolazo” case
The previously described method was applied to the hashtag #cacerolazo. Therefore, we extracted the subset of all tweets that contained this hashtag to study them. First, we verified which cluster they belonged to and obtained the results presented in Table 6. For this specific case, we may confirm that #cacerolazo, which was considered controversial (employed by pro- and anti-government groups as shown in Figure A2), becomes apparent after this analysis.
The results showed that 67% of the tweets with the hashtag were found in Cluster 1 (against the government). Then, the act of participating in a cacerolazo maintained its traditional use by populations as an actual sign of disagreement with a government and not as a sign of peace, as some political groups wanted to make Ecuadorians think.
Table 6. Distribution of the use of #cacerolazo among tweet clusters
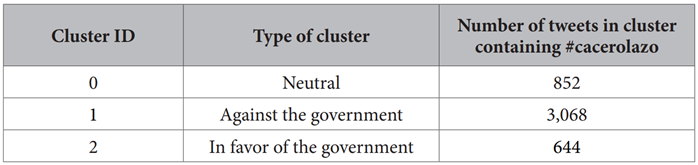
Source: Table created by the authors.
a. Media news analysis
This section describes the method of extracting, pre-processing, and analyzing the news related to the protests. Once the analysis of the tweets has revealed which are the local newspapers involved in the protests as content generators, the respective extraction of their news was carried out. We collected news headlines from local newspapers (e.g., eluniverso.com, elcomercio.com, larepublica.ec, metroecuador.com.ec, pichinchauniversal.com.ec, eldiario.ec, and eltiempo.com.ec), using the Google News content aggregator. This aggregator gave way to the collection of news by executing a query. The retrieved results included the newspaper’s name, the headline, and the link to the news (depending on the corresponding newspaper’s website). The extraction of news from an aggregator allowed us to search in each source. However, given a large amount of information, automatic techniques were required to extract more specific data.
Given the need for automatic extraction, web scraping was employed to create the corpus of news. Web scraping is the process of automatically gathering information from the Web. In this case, we used the Selenium software (https://www.selenium.dev/), a framework for website testing. Selenium can programmatically control the browser (such as Google Chrome) to navigate the websites like humans.
The scraper program was developed in the Python programming language. In this way, we extracted 985 news items related to the national strike in Ecuador that were published by local newspapers. Thus, with this collection, we created the news corpus.
Having this corpus, first, the sources of information for press releases were identified to define whether the writer is a local media or not. Then, 46 local news items were randomly drawn in which the frame was manually observed. The news framed the topic “cacerolazo” in two ways: 1) as a mobilization that seeks to recover social peace, and 2) as a protest against the economic measures decreed by President Lenín Moreno. The results of the analysis were compared with 26 international media reports.
The news frame that refers to “cacerolazo as a protest” is exemplified next. The October 4 issue of Radio Pichincha was titled as “Student from Quito could lose an eye after being hit by tear gas canister.” Later, on October 7, El Universo published among its headlines “Protests against the Government keep 9 de Octubre Avenue closed” (El Universo 2019a) and continued saying, “with posters against the Government and President Moreno, the protesters demand the repeal of Decrees 883 and 884.” In this publication, the mobilization is framed as an intense protest against the government of Lenín Moreno. On the other hand, between October 12 and 14, the news from El Comercio announced, “Neighbors of Quito called for a potluck,” and in the text, it is clear that it is for peace, to ask for “the end to the violence in the streets.” Likewise, in one of the news items from El Universo on October 14, 2019, we can read the headline “Cacerolazo and riots in the curfew in Quito” (El Universo 2019b). However, in the body of the text, it is understood that various social sectors participated in the cacerolazo to promote peace.
There are also news items that fit into both frames, such as the news from Metro Ecuador published on October 12 and entitled “Neighborhoods of Quito join the cacerolazo.” This news explains that “the neighbors came out with pots, spoons, or what they had at hand in an act that took several nuances: some ask for peace, others ask for the rights that the State must grant, others to remember the protesters who died during the days of the strike, and the rest against the economic measures.”
In conclusion, after analyzing the headlines and texts of 46 local news items, it is evident that the predominant framing of the media is to refer to the cacerolazo as a demonstration of citizenship for peace. In the analysis of 26 news items from international media, we confirmed that the predominant framing is the scenario of a cacerolazo for peace called by residents in the face of the violence unleashed among opposition organizations.
Discussion
Once the timeline was drawn following the media narratives, using the Twitter API and the experts’ knowledge of the context, we selected 54 trending topics. We collected tweets corresponding to these trending topic hashtags, generating a dataset with 130,382 tweets, of which 119,756 remained after the cleaning process. We listed the hashtags according to their occurrence frequency with the following result for the top-five: #nationalstrikeec: 12,950; #ecuador: 7,293; #EcuadorInResistance: 5,828; #dialogueforpeace: 5,358; and #sosecuador: 5,259.
We listed the most active users in the sample based on their number of posts and identified the type of their accounts. We found Twitter users, media, political parties, universities, among other actors. The five most active accounts were @jorgejavieruio with 418 posts; @ecuainm_oficial with 375; @pichinchauniver with 305; @Primicias with 289, and @notimundoec with 197.
A weighted graph allowed us to represent the interaction structure, with user account as the source node, hashtag as the target node, and frequency as the weight. The results showed, for example, that the hashtag #nationalstrikeec was the most used, while @ecuainm_oficial was the account that used more hashtags in its published content.
By using word2vec, 10,753 words were vectorized in 300 dimensions. Then, clusters of words were created to group words that were semantically related to each other. Three clusters were clearly identified regarding the context concerning the government: 0. Neutral (with 3,434 words), 1. Against the government (with 2,107 words), and 2. In favor of the government (with 4,258 words). In Cluster 1, words that denote opposition to the government, like “repression,” “struggle,” “deaths,” “terrorists,” “violence,” and “force,” among others, were found. On the other hand, in Cluster 2, words that describe support to the government, such as “dialogue,” “government,” “president,” “together,” “country,” “peace,” among others, were grouped. Finally, in Cluster 0, general words were grouped, such as “police,” “military,” “today,” “protest,” “Quito,” “people,” among others.
Then, we calculated the center of the clusters as a representation of the context and a reference point to measure the greater or lesser distance of the elements from that center (centroids). An equation was constructed to classify the tweets by their degree of similarity with respect to those centroids and to subsequently assign them to one of the three clusters. For instance, the tweet “#Quito, the night of this October 12, 2019, the Quiteños participated in cacerolazo” belongs to Cluster 0 (neutral); while the tweet “I am participating in the #cacerolazo in La Concordia #GetOutLeninGetOut” was assigned to Cluster 1 (against the government), and “The #Cacerolazo is to hurry with the #DilogueForThePeace” belongs to Cluster 2 (in favor of the government). The tweet clustering model was 86% accurate.
A heat map allowed us to visualize the frequency with which users combined two hashtags in the same tweet. It was easier to select the tweets of greatest interest from this visualization since the most combined hashtags appeared in them during the protest. Then, we proceeded to a qualitative analysis of them, which showed that 92% of the tweets containing the hashtag #GetOutLenin belong to Cluster 1 (against the government). Something similar occurred with the hashtag #GetOutMoreno, because 96% of the tweets that contained it were classified as belonging to Cluster 1. On the other hand, #DialogueForPeace, #DialogueAndPeace, and #DialogueIsTheWay were used by people supporting government stability (Cluster 2).
We applied the proposed method to analyze the tweets that contain the hashtag #cacerolazo. The results of the method grouped the tweets related to the word “cacerolazo” as follows. In Cluster 0 (neutral), 852 tweets were located, 3,068 in Cluster 1 (against the government), and 644 in Cluster 2 (in favor of the government). The analysis allowed us to disambiguate the meaning of the tweeted word “cacerolazo”: actually, 67% of the tweets with the hashtag were found in Cluster 1. Then, the act of performing a cacerolazo maintained its traditional interpretation by populations as an actual sign of disagreement with the government and not a sign of peace. Figure 2, which shows relevant words placed in the vector space, illustrates this phenomenon as well. For instance, in the semantic use of content, the words “dialogue” and “peace,” which are related to each other, form a cluster far from the word “cacerolazo.” On the other hand, the word “cacerolazo” maintains its closeness with hashtags such as #QuitoIsBrave, #ThePeopleDon’tGiveIn, and #curfew.
To contrast the frame presented on Twitter and the media frame, we extracted 985 news pieces through Google News. We identified the frames of 29 news items that explicitly referred to cacerolazo. According to our findings, the media framed the news in two ways: 1) as a mobilization that seeks to recover social peace; and 2) as a protest against the economic measures decreed by Moreno.
For a predominant group on Twitter, the cacerolazo was a protest against the government’s actions, which could be summarized in the hashtag #GetOutLenin. For another group, it supported peace dialogue to stop the social protest and return to institutional normality. However, the tweets that interpreted cacerolazo as a protest against Moreno denounced the media (like El Comercio, El Universo, or Teleamazonas) that claimed that the cacerolazo was for peace. Indeed, virtual protesters implicitly accused the media of interpreting the protest to promote an agreement between the government and the transporters. Thus, some tweets talk about a “corrupt press” and “media censorship.” In a defensive tone, on the other hand, some media tweets tried to recall the origin and widespread use of cacerolazo in social protests. For example, on October 12, the newspaper El Comercio stated that “the call for cacerolazo has no political connotations,” and describes that “families go out to the rooftops asking for peace.”
The other frame is related to the fatigue of the population with so many days of protest and destruction of cities. In addition, this frame denounces those who want to appropriate the indigenous protest and destabilize the democratic government, presuming that they are the supporters of former President Correa.
Despite this, both frames appear to stand against the fuel increase decree. The difference is that for those who oppose the government, the decree is yet another proof of Moreno’s anti-popular character; therefore, he must be delegitimized for not fulfilling his electoral promises. In fact, he is called “repressive” and “fascist” during crisis management. For the other audience or frame, it would be enough to repeal the decree to regain social peace.
Conclusion
The “cacerolazo case” is an example of Twitter’s capacity to activate frames about political events, with autonomy from those activated by the media. This episode is of interest because the media interpreted the meaning of cacerolazo in two contradictory ways: a protest against the government or a request for the cessation of protests and recovery of peace.
The meaning of cacerolazo was a topic of debate on Twitter. One meaning was predominantly assigned by the media and another one by the Twitter community. We have proceeded to disambiguate the word under the conjecture that clarifying the value of this event for different enunciators of the hybrid media system would allow us to delimit the conflicting frames of the meaning of social protest. Cacerolazo functions as an “empty signifier” (Laclau 1996) in the debate, in which different frames struggle to give it a unique meaning.
Using word2vec, we have confirmed the hypothesis that in the context of the 2019 strike in Ecuador, the media and social media activate different frames about the cacerolazo. The framework proposed by Twitter for cacerolazo differs from that proposed by the media. We have also verified that the meaning of cacerolazo is interpreted differently by two frames within Twitter publications; one of them is critical of the interpretation of the meaning of cacerolazo proposed by the media. We can conclude this by identifying three clusters on Twitter: Cluster 1, against the government (predominant); Cluster 2, in favor of the government; and Cluster 3, neutral.
Concerning the media framing, Cluster 1 can be related to what Hall (2001) refers to as a “reading of resistance.” It discusses the very core of the ideological frame of social protests on the part of the media. Cluster 2 corresponds to a “hegemonic reading,” which accepts the interpretative frame of the media.
Finally, a “negotiated reading,” or neutral, seems to want the dialogue for peace to prosper without taking sides in favor or against the government that would correspond to Cluster 0.
This case study shows the use of data analysis or social web mining as an alternative tool to surveys to study the state of public opinion about a public event. The data produced spontaneously without sampling techniques allow us to visualize issues on the public agenda in real-time.
In the study case, the conformation of communities, identified by clusters according to their political attitude, is concomitant with the diffusion of a frame. This identification of frames and clusters results from data structuring that uses analysis algorithms and does not respond to the researcher’s guidelines (González 2019).
In sum, from the analysis of this data, it was possible to appreciate how the frames of the most active groups on Twitter can be distinguished from those preferred by the traditional media.