Services on Demand
Journal
Article
Indicators
-
Cited by SciELO
-
Access statistics
Related links
-
Cited by Google
-
Similars in SciELO
-
Similars in Google
Share
Cuadernos de Desarrollo Rural
Print version ISSN 0122-1450
Cuad. Desarro. Rural vol.11 no.73 Bogotá Jan./June 2014
Application of Prospective Analysis and Cluster Analysis as a Tool to Aid in the Design of Rural Development Policies: the Case of Andalusia*
Aplicación de análisis prospectivos y análisis de conglomerados como herramientas para apoyar el diseño de políticas de desarrollo rural: el caso de Andalucía
Application d'analyses prospectives et d'analyses typologiques (analyse de grappes) comme des outils pour supporter la conception de politiques de développement rural: le cas d'Andalousie
Pedro-Pablo Perez-Hernández**
José-Manuel Martin-Lozano***
Mercedes Torres-Jiménez****
Luis-Antonio Fernández-Portillo*****
*Technical and Logistical Support to Rural Development Board of Counseling for Agriculture and Fisheries of the Andalusian Government (Spain) for Rural Diagnosis and Future Strategies Planning (NERA). Funded by Andalusian Regional Government. The project lasted 12 months (January to December 2007).
**PhD Universidad de Sevilla, Professor in the Universidad Loyola, Andalucía. Email: ppperez@uloyola.es
***PhD Universidad de Córdoba, Professor in the Universidad Loyola, Andalucía. Email: jmmartin@uloyola.es
****PhD Universidad de Córdoba, Professor in the Universidad Loyola, Andalucía. Email: mtorres@uloyola.es
*****PhD Universidad de Córdoba, Professor in the Universidad Loyola, Andalucía. Email: portillo@uloyola.es
******Authors would like to thank Mr. Francisco Alba in Counseling for Agriculture and Fisheries of Andalusian Regional Goverment, as well as Mr. Juan Manuel Valderas and his team in Development, Agrarian and Fishery Working for Andalusian Regional Goverment.
Recibido: 2013-10 -02 Aprobado: 2013-12-15 Disponible en línea: 2014-05-30
Cómo citar este artículo
Perez-Hernández, P. P., Martin-Lozano, J. M., Torres-Jiménez, M. y Fernández-Portillo, L. A. (2014). Application of prospective analysis and cluster analysis as a tool to aid in the design of rural development policies: the case of Andalusia. Cuadernos de desarrollo rural, 11(73), 61-86.
Abstract
The EU has promoted initiatives to develop its rural areas. In this research, we apply the last of those initiatives to Andalusia (southern Spain). These are the main aims pursued in this study: first, to present the methodology selected to identify the most influential variables that act as catalysts for development in each rural county of Andalusia; second, to identify rural county groups that show basic similarities in terms of the variables selected and third, to contribute to the integration of development strategies. To reach those objectives, it was applied the Prospective Structural Analysis as well as Cluster Analysis along with Discriminant Analysis to validate it. The results show the existence of four clearly differentiated clusters in the region.
Keywords authors: new rural strategic in Andalusia; EU rural development
Keywords plus: rural development; Andalusia, rural developement; European Union
Resumen
La Unión Europea ha promovido diversas iniciativas de desarrollo rural en sus territorios. En este artículo se analiza la estrategia seguida para su aplicación en Andalucía en el periodo 2007-2013. Los objetivos perseguidos son: en primer lugar, presentar la metodología elegida para identificar las principales variables de mayor influencia en el desarrollo de las comarcas rurales andaluzas; en segundo lugar, identificar grupos de comarcas rurales que muestran similitudes en las variables seleccionadas y, tercero, contribuir a la integración de las estrategias de desarrollo. El logro de estos objetivos fue posible mediante la aplicación del análisis estructural de prospectiva y análisis clúster con análisis discriminante para su validación. Los resultados muestran claramente la existencia de cuatro grupos de comarcas diferentes en la región.
Palabras clave autores: nueva estrategia rural en Andalucía; desarrollo rural en la UE
Palabras clave descriptores: desarrollo rural; Andalucía, desarrollo rural; Unión Europea
Résumé
L'Union Européenne a encouragé des différentes initiatives de développement rural dans ses territoires. Dans cet article se fait l'analyse de la stratégie suivie pour sa mise en marche en Andalousie au période de 2007-2013. Les buts à réussir sont: en premier lieu, présenter la méthodologie choisie pour identifier les variables clés plus fortes dans le développement des régions rurales andalous; en deuxième lieu, identifier les groupes de régions rurales montrant des similitudes dans les variables retenues et, en troisième lieu, contribuer à l'intégration des stratégies de développement. La réussite de ces buts a été possible grâce à l'application de l'analyse structurelle de prospective et l'analyse typologique (analyse de grappes) avec une analyse discriminante pour sa validation. Les résultats montrent clairement l'existence de quatre (4) groupes de différentes régions en Andalousie.
Mots-clés auteurs: nouvelle stratégie rurale en Andalousie; développement rurale à l'UE
Mots-clés descripteur: développement rural; Andalousie, développment rural; Union européenne
Introduction
The publication of the document The Future of the Rural World (European Commission, 1988) marked a new direction in Europe for rural development in the Member States. It was a systematic process put into action by various governments. This fundamental change in approach in what had been the EU's agricultural policy up to that time went into effect in 1991 on the passing of the Leader Initiative (European Commission, 1991) and was reaffirmed in the theoretical Cork Declaration (European Commission, 1996). To make it work, a network of local action groups (LAGs) was set up in each of the regions considered Objective 1 and 5b of the Member States. The aim of these groups, evident since their creation (European Commission, 1991), has been to design and implement the planning process and promotion of development in local areas (González, 2006), streamlining the various economic and social agents in these rural areas.
This work has its origin precisely in the realization of a concrete project for rural development in the region of Andalusia, in southern Spain. Similar to other ones developed in different European regions (Convery, Soane, Dutson & Shaw, 2010). The project entitled New Rural Development Strategy for Andalusia (NERA) was launched by the Rural Development Directorate General of the Junta de Andalucía Agriculture and Fisheries Ministry for the current European funding framework program 2007-2013.
Andalusia has a number of unique features that have made it the target of European Community rural development policies for a long time. What makes the Andalusian region a paradigm in the application of the rural development policies designed from Brussels are the different climates, geography and development of its regions, its land area (87 597 km2 which represents 17.3% of Spanish territory and 2% of that of the European Union), its agricultural tradition (its agricultural production accounts for 7% of the GDP) and its marke dly rural population (about half of those in the region live in rural areas, 90% of Andalusian territory being eligible for action in rural development policies). Indeed, during the last fifteen years, under the supervision of the Rural Development Directorate General, an intensive effort has been made in the economic, social and environmental development of rural territories thanks to contributions from structural European funds and co-financed by the State and the Autonomous Region itself.
For this programme, the Rural Development Directorate General decided to launch a process of reflection about the socio-economic development of rural territories in the region, about the situation of the rural world and about the needs that this world will have in the coming years, suggesting that this process be led by LAGs. Thus it was a question of setting up for the first time a strategic coordinated proposal for the fifty-two local action groups that make up the rural territory of this region. Having analyzed the situation in the region, as well as rural development proposals elaborated for each individual region (Junta de Andalucía, 2008), the following specific objectives are proposed in this paper in order to facilitate coordination and take advantage of synergies among counties to enhance development:
1) The identification of the key or most influential macro-variables that are the driving force behind the development of each region. To achieve this, the methodology for Prospective Structural Analysis (PSA) (Godet, 1985) was applied.
2) Identification of groups in similar rural counties in terms of their key or influential macro-variables, which help public government to apply common development policies, thus contributing to the integration of county strategies and an approach to development on a global scale, as proposed in the objectives of the NERA project. For this end Cluster Analysis was applied (Lloyd, 1982; Ward, 1963) while Discriminant Analysis was used for the validation of the groups (Johnson & Wichern, 2002).
3) Characterization of groups or clusters identified based on the above-mentioned objective, identifying the common characteristics of the counties included in each cluster as well as characteristics distinguishing different groups.
So the structure of the paper is as follows: the second section describes the data used in Andalusia; the third section presents the methodology used in the various phases of this research; the fourth paragraphs show and discuss the results achieved according to the groups formed, as well as their suitability for achieving the objectives proposed and, finally, the fifth section presents the conclusions drawn by the authors.
I. Theoretical framework
The rural development model implemented in Europe during the last decades belongs to endogenous development model in which the main features are the following factors (Ward, Atterton, Tae-Yeon, Lowe, et ál., 2005; Buchenrieder, Mõllers, Happe, Davidova, et ál., 2007); harnessing local (natural, human and cultural) resources for sustainable development; local initiative and enterprise, diverse service economies, limited capacity of areas and groups to participate in economic activity, capacity-building (skills, institutions, infrastructure): overcoming exclusion.
A very complete synthesis of the endogenous development theories in Europe is made by Vázquez-Barquero (2002). Those theories support different rural development iniciatives apply in the region: high development theory, dualistic growth theory, dependence theory and territorial development theory. However, they are not able to explain the mechanism of the economical development in each European region (Terluin & Post, 2000; Bryden & Hart, 2004). Nevertheless, in rural development, it has been stressed territorial development theory for its special relevance on the implementation of the European rural development initiatives (Leader initiatives). Thus, territorial development theory has been widely considered in Lationamerica from the point of view of fundamental analysis (Pérez, 2002; Llambí, 2004) as the implementation of specific initiatives (i.e. Interamerican Development Bank, World Bank). Main features of this theory are that development is based on local resources; it is made by local initiatives and that there is a local control on development.
On the other hand, other authors point out how development should contain endogenous and exogenous factors in a globalized economy (High & Nemes, 2007). The challenge is to find the equilibrium between those factors, having in mind the best benefits for rural areas.
In that sense, the main objective of the European initiatives was the promotion and economic development of the most disadvantaged rural areas of the European Union member states at that time, thus implementing a territorial and participatory approach (Ray, 1998; Schejtman & Berdegué, 2003; Sumpsi, 2006) and demonstrating regional differentiation in rural development strategies along with the need for non-agricultural development in rural areas. The development strategy implemented in the Andalusian region has been based on the territorial rural development theory which is the fundamental for Leader initiatives.
2. Description of the field data used
The first thing that had to be done was to obtain descriptive information about the situation in the region being analysed. Participating as methodology consultants in the NERA Project allowed us access to all field information for subsequent phases.
Figure 1 reflects the planning methodology of that project. First of all, there are three territorial levels of planning: the local or county (52 counties), provincial (8 provinces) and regional (only one, Andalusia) level. From a conceptual point of view, planning is structured in the following phases: presentation and organization of the process, diagnosis, formulation of strategic objectives and strategies, and the reports on performance. Clearly, after the project was ready, the phases of putting them into practice began, along with the control of the respective plans.
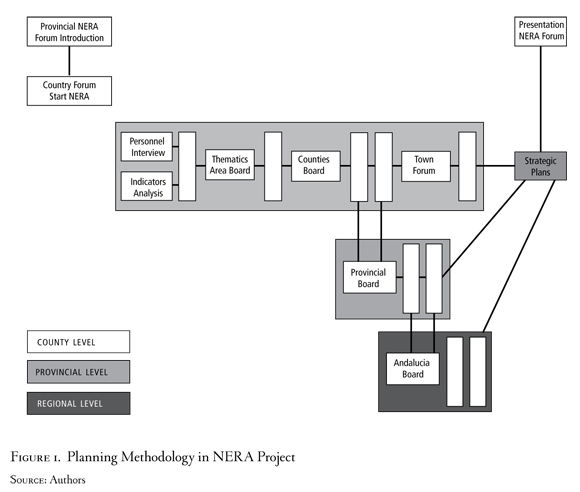
In order to respond to these conditioning factors, it was considered appropriate for all local action groups to work within a common thematic framework, namely, that their approximations to the reality of the territory (both in their diagnosis and to set goals and develop strategies) was carried out by classifying this reality into the six following subject areas: Territory and Environment; Population and Society; Infrastructure and Transport; Economy; Administrative Organisation and, finally, their surroundings in general.
These areas are based on the subject classification set up by the Multiterritorial Information System of Andalusia, whose database was used for part of the diagnosis. But to further refine the research, subject areas were classified as macro-variables, which were then broken down into variables, which were the basic conceptual unit for the work carried out in different phases of the project. Each topic area contained a series of macro-variables (reproduced in Table 1), comprising the unit under analysis. Each macro-variable clearly defined the part of the area under consideration and added a very large number of variables but of a smaller size than the previous one. Without taking these smaller variables into consideration, it would have been impossible to apply the methodology selected, or to interpret the results or their usefulness in the formulation. A total of 33 macro-variables were considered. In turn, each macro-variable contained a group of variables, and the resulting set of variables was 177.
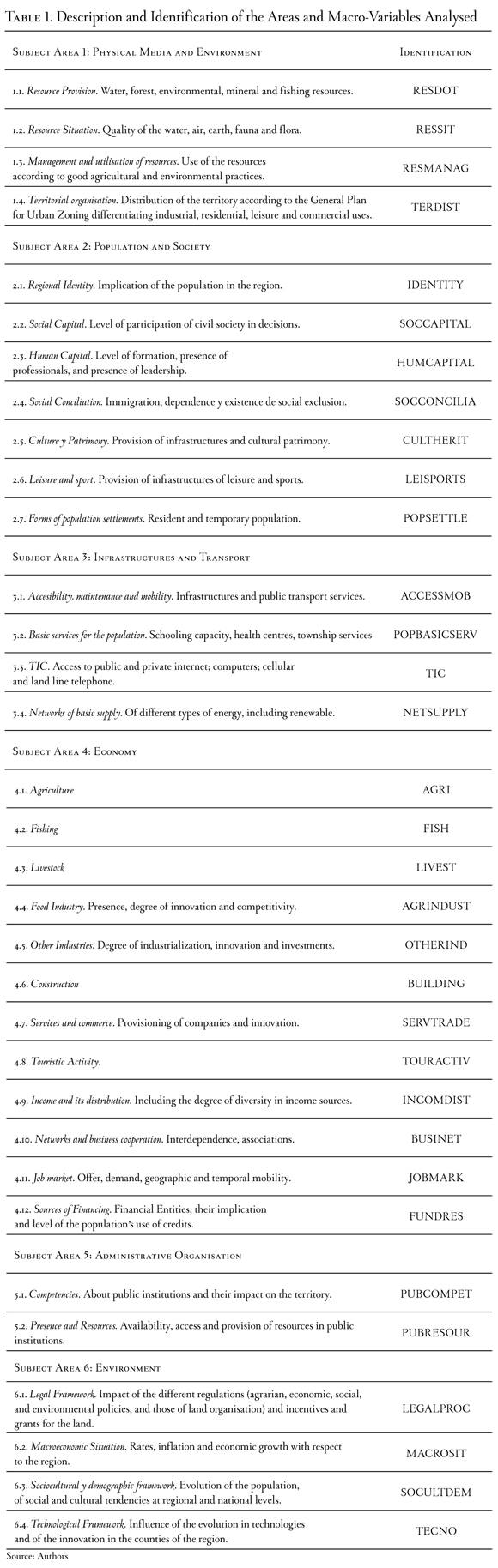
Next, the diagnostic phase aimed to avail itself of a participatory assessment of the reality of the territory, both at the present time and especially with respect to future trends. In turn, this diagnosis was composed of two sub-phases. The first was a basic description of the area (considering the different thematic areas mentioned above) to identify the main agents and variables present therein. This information would serve as input for the following phases of the process. The second sub-phase involved the development of matrices for Strengths, Weaknesses, Opportunities and Threats (SWOT analysis), both at the level of each subject area as well as at the level of each region. In the third phase, provincial SWOTs were generated, and finally came the elaboration of the corresponding diagnoses and the generation of strategic options at local, provincial and regional levels.
The structure of the development of strategies was hierarchical: from each target came a set of strategic guidelines and from each of these, a series of performances. The determination of the lines of action was carried out through thematic SWOT matrices (that included problems and solutions, measures to enhance strengths, etc.) and structural-analysis information about the prospective applied to macro-variables; all of this detected not only possible courses of action, but also the factors of territory intervention that would be most appropriate or have the highest priority, for their potential to multiply rippling effects on other elements. Next we will describe the work methodology followed to arrive at the final results of this research.
3. Methodology
Several methodologies have been applied to prepare this study: Prospective Structural Analysis (PSA) to obtain scores for the influence of the macro-variables analysed in each region, and Cluster Analysis together with Discriminant Analysis, to create and validate clusters.
3.1. Prospective Structural Analysis
The study of rural areas as complex, systemic and evolutionary realities requires certain tools to consider, holistically, their constituent elements, their interrelationships, their dynamic nature and the impacts any change in them cause on the system as a whole (Habegger, 2010). When following a systemic approach, it is common to develop formal models in line with system dynamics and the logic of networks; along with the difficulty in obtaining county-level data, these methods often have limitations when applied to social systems (Rosenhead, 1996).
In recent decades, the field of systemic thought has been incorporating new areas of interest related to dialogue and interactive learning (Schlange & Jüttner, 1997). From this cognitive perspective, the social construction of a rural area can be considered an "unstructured problem" because multiple stakeholders and perspectives interact, and conflicts of interest and interpretation arise that hinder developing an encompassing and unique object of analysis. To resolve this problem of constructing the object of study, tools are needed to organize thought and collective deliberations, and at the same time help to generate a shared perspective on the same problem (Bolanos, Fontela, Nenclares & Pastor, 2005). For practical purposes, this type of tools must be accessible to agents with different skills and perspectives, to facilitate the exchange of views between them and work iteratively while decisions are being deliberated (Mingers & Rosenhead, 2004).
By considering rural territory as a social construction, the interpretation made by local agents concerning restrictions, potential and incentives for change within their communities should be fundamental input to use rural territory as an "object of study". To this end, we propose the application of Prospective Structural Analysis (PSA) (Pérez, Martín & Ambrosio, 2009). With PSA you can describe existing dependence or influences on situations in the territory, but it also works to design strategies, encouraging and structuring collective thought processes that generate a future vision of rural areas and highlight the actions necessary for success (Godet, 2001; Gavigan & Scapolo, 2001).
With respect to agriculture, an application of the PSA has proved to be a systematic method to unravel the complex relationships among the variables that characterise this system, identifying the most important ones (Gómez-Limón, Gómez-Ramos & Sánchez, 2008; Gómez-Limón & Gómez, 2008).
With respect to rural development and PSA, we found a first study in Ambrosio & Delgado (2008). in which Its authors point out that this methodology is crucial for building rural territory through a process of reflection on the part of all those involved while enabling the detection of patterns still in development where similar policies can be applied.
The PSA technique helps to describe a system by identifying the relationships of influence (rather than cause-effect relationships) among the components of the system through a process of collective reflection reflected in a double-entry matrix. Applying the properties of Markov chains (Markov, 1971), this tool can generate hierarchies and classifications of elements according to their motor properties and dependence. As a result of these hierarchies, the method emphasizes the structure of relationships among system variables and identifies the key variables essential for their evolution. Because feedback exists in any system, this method makes a further distinction between direct and indirect influences. These are the result of adding feedback effects and influences of an order superior to 1. The importance of a variable should be measured not only by its direct relation with other variables, but also by millions of indirect influences (Godet, 1994).
The research presents the PSA technique adapted to the reality of the rural areas under study and is structured in three phases:
- Phase 1: List of macro-variables. The first step was to develop a list of the internal and external macro-variables that make up the system. Our study had a total of thirty three.
- Phase 2: Description of the relationships among macro-variables. The main purpose of this phase was to fill out a double-entry matrix, involving prospective workshops that were organised with local stakeholders, representing the value of the influences of some macro-variables over others. After several working sessions in the prospective county tables matrixes elaboration were made by local experts in each area. On those sessions, experts and GAL technicians filled in matrixes scoring (0 to 3) influence-dependence level among macro-variables (Junta de Andalucía, 2008).
- Phase 3: Identification of the key macro-variables. For the classification of the macro-variables in the system, the computer programme applied was the Matrix of Cross-Impact Multiplication Applied to a Classification (MIC MAC), which apples the properties of Boolean matrices to sort macro-variables according to the number and intensity of the paths and feedbacks that each variable initiates (driving influence) or receives (dependency influence) (Godet & Bourse, 1989; Godet, 1994).
The implementation of PSA in the NERA project gave rise to as many Directly Influencing matrices (DIM) as counties analysed, through which and by applying the MIC-MAC software, corresponding levels for driving/dependency influence were obtained, the step previous to the grouping of each macro-variable according to the logics outlined above. This analysis was fundamental for establishing different strategies for each group (Junta de Andalucía, 2008).
The DIM matrix provided each county with the total scores of influence exercised by each macro-variable on the rest of them, adding up in rows the scores obtained for each macro-variable represented by a., elements as described above in Phase 2. This information was included in a 42x33 order of matrix (42 is the number of counties; 33 represents the number of macro-variables analysed). The descriptive analysis of these scores (Table 2) shows significant inter-county variability in the scores of influence of the macro-variables analysed, especially in some macro-variables such as Leisure and sports (LEISPORTS), Livestock (LIVEST) and Technology (TECNO) whose Variation Coefficients exceeded 50%.
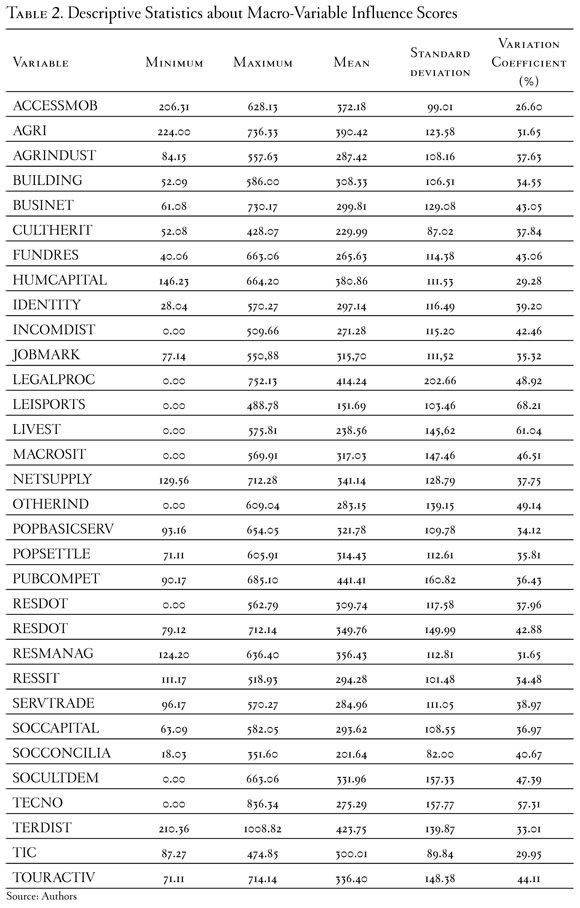
This variability highlights the significant differences in key or influential variables affecting development in the counties studied, further justifying the later analysis of cluster searches in counties that share the with respect to development.
3.2 Cluster Analysis
Cluster analysis is a multivariate statistical method of interdependence which is useful for classifying individuals into categories and can also be used to classify macro-variables. It has frequently been used in many different areas of the business world and it has also been applied to Agricultural research, especially in developing farm typologies (Pardos, Maza, Fantova & Sepúlveda, 2008; same key variable profiles, García-Martínez Balasch, Alcon & Fernández-Zamudio, 2010; Torres, Hervás & García, 2009) and, even in rural development (Cecatto & Persson, 2002; Frochot, 2005).
In this work, cluster analysis has been applied to identify and differentiate groups of counties sharing common macro-variables and who mutually influence one another while remaining heterogeneous with the rest of the groups. Cluster analysis was performed with the scores on these influences obtained in the previous phase for each macro-variable in each region. The SPSS Statistic 17.0. programme was used.
Previously, compliance had been confirmed with the necessary conditions for the application of cluster analysis (the representativeness of the sample and the absence of multicollinearity among the macro-variables). The representativeness of the sample is ensured because the analysis was performed on all the counties in Andalusia (52) (although 10 did not provide the requested information for the study). In case of the possible existence of multicollinearity, the matrix of Pearson Bivariate Correlations was determined. The higher value of this coefficient was obtained between the macro-variable of human capital and accessibility, maintenance and mobility (0.638); leisure and sport, culture and heritage (0.760); local identity and macroeconomic status (0.703); legal framework and macroeconomic status (0.673). In all other cases the correlation coefficient ranged between 0.2 and 0.4. It was decided not to eliminate, a priori, any of the macro-variables of influence in the cluster analysis, although there would be a later selection process.
In a first phase, the scores of influence of the 33 macro-variables are used as the initial information for doing the cluster analysis. The two existing methods, the hierarchical and the non-hierarchical, were combined to form the clusters, thus trying to exploit the advantages of each and simultaneously avoid their drawbacks (Hair William, Barry & Anderson, 2009). Therefore, to start out with, a hierarchical cluster was made with Ward's method as the stopping criterion (Ward, 1963) to establish the appropriate number of clusters (groups of counties) and the profiles of their centres; in a second stage, the non hierarchical k-means method was used (proposed by Stuart and used later by James MacÇHieen in 1967) taking as initial seed points the centres of the clusters previously obtained with the hierarchy, to "fine-tune" the formation of clusters obtained through the hierarchical procedure.
As there is no guarantee that the K-means algorithm will converge to the global optimum, and the result may depend on the initial clusters, the third stage proceeded to the validation of the groups formed. Linear Discriminant Analysis (LDA) (Johnson & Wichern, 2002) was used to evaluate the adequacy of the classification result obtained with Cluster Analysis, given the group memberships of the rural areas under study. Specifically, LDA was used to evaluate the generalization capacity of the Cluster Analysis as well as the sensitivity of its results with respect to the composition of the sample used (given its small size). LDA was applied on 10 different partitions of the total group of counties analysed (from which 70%, was selected for training and 30% for generalization) using macro-variables as inputs and the cluster membership of each region as the output. To measure the results, the Correct Classification Rate (CCR) was used, representing the percentage of correctly classified rural areas in training and generalisation sets, respectively. For a perfect classifier, CCR will be equal to 1.
Later a selection was made of the macro-variables that had influenced the formation of the clusters. The Weka programme (Witten & Frank, 2000) release 3.4.0.was used, through the method called "Correlation-based Feature Subset Selection Algorithm". This method evaluates the worth of a subset of attributes by considering the individual predictive ability of each feature along with the degree of redundancy between them. Subsets of features that are highly correlated with the class but with low inter-correlation are those preferred (more information can be seen in Hall & Smith, 1998). Finally, the validation of the clusters was repeated using only the macro-variables selected by the above method for the application of LDA.
4. Results and Discussion
The hierarchical analysis identified 4 clusters (the change in the agglomeration coefficient was too high, 16%, to pass from 4 to 5 groups) which included 3, 9, 22 and 8 rural counties respectively. LDA was applied to evaluate the reliability of the clusters formed and after, we sought to determine the profiles of the rural counties included in each of them.
With respect to the validation of the clusters, we can report that Table 3 shows the CCR obtained in the 10 partitions carried out, in both training and generalization groups. In the first stage, which included all the macro-variables as input (33), 100% of the regions of the training groups were correctly classified in all partitions. In generalization, however, the results were very different depending on the partition selected, the best score being 78% correct recognition of clusters, but on average only 38%. The coefficient of variation was 45%.
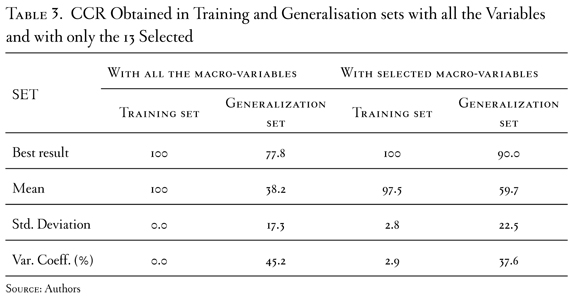
Subsequently, there was a selection of variables with the Correlation-based Feature Subset Selection Algorithm. This algorithm identified 13 of the previous variables: agriculture, competencies, resource allocation, livestock, management and use of resources, local identity, food industry, macroeconomic situation, legal framework, socio-cultural and demographic context, technological framework, leisure and sport, and resource situation. The LDA was then repeated with these 13 independent macro-variables on the 10 partitions. In the training group results were slightly worse than the previous case where all the macro-variables were considered inputs (average of CCR 97.54%), however, the generalisation ability improved significantly (90% of CCR in the best results and an average CCR of 60%).
Therefore the results improved considerably in the generalisation group when only the selected macro-variables were included, and also improved somewhat in stability in the results obtained in different partitions. It seems that including all of the macro-variables resulted in overfitting because, although the results in training were optimal in this case, they were not good in generalization. It was therefore decided to consider that the 13 macro-variables selected were the really influential ones in the formation of groups.
4.1 Determination of the group profiles
By analysing the mean values of the macro-variables selected for the formation of the 4 groups (see Figures 2a and 2b), we can describe the profile of each local group to facilitate the adaptation of the Public Administration Development Policy design for the territory, once the strengths and weaknesses of each group have been identified.
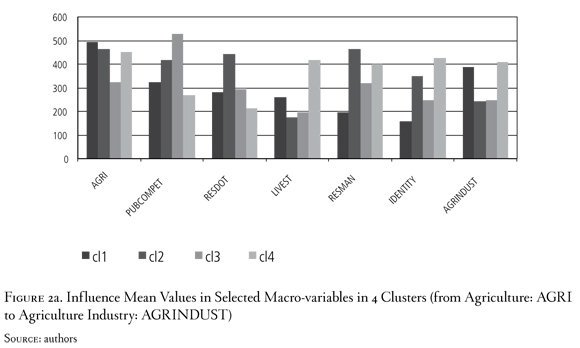
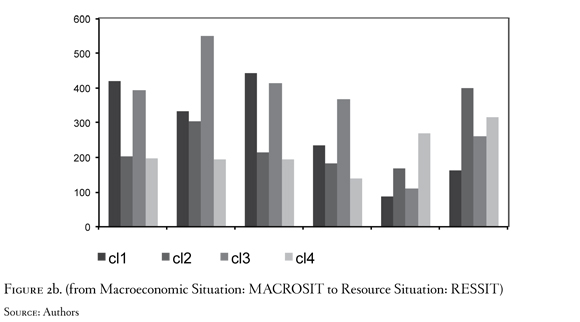
Table 4 shows the counties included in each cluster. The counties included in the first cluster are characteristically highly influenced by agriculture, the macro-economic situation, social-cultural and demographic context (macro-variables whose values are the highest in this group) and the food industry. However, it has lower average values with regard to the influence of macro-variables like the management and utilization of resources, the provision and situation of resources, local identity and sport and leisure. In addition, other macro-variables also showed reduced mean values that are not part of those selected, like social capital, culture and heritage, presence and resources.
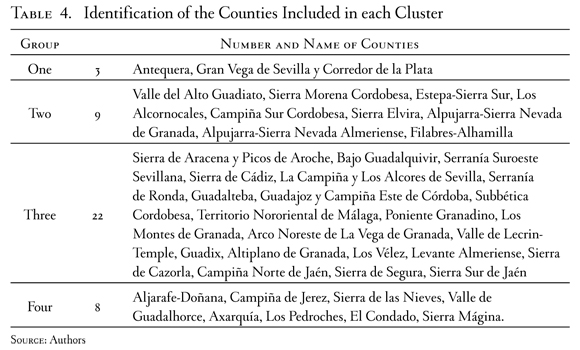
Among the macro-variables that most influence the regions included in cluster 2 are found the strength, status and management of resources, which reach their maximum average value in this group. Macro-variables with lower average values of influence include livestock and the food industry (that obtain the lowest average values in this group). However, agriculture maintains a high average value. The technological framework also shows a low average in this cluster.
We can draw the conclusion that in cluster 2 regions, the most important and influential activities are those that refer to the subject area of physical environs and the environment, that is, cluster 2 includes regions with a good provision and management of resources. Th ey are also characterized by the low weight of livestock.
On analyzing cluster 3, the most influential macro-variables are in the area of administrative organization and the environment; in particular, they reach their maximum average competencies (in public institutions with a great impact on the territory), legal framework (influence in regulations and incentives, and aid to the region) and technological framework. Those with lower values include agriculture (lowest weight), and that with the least influence which is tourism (although this variable does not form part of the selected group). In this group, the average macrovariable values are low in general.
Finally, in cluster 4, the macro-variables that have medium-high average values and therefore are the most influential are livestock, local identity, the food industry, recreation and sport. Other macro-variables that reach their maximum average value in this group, although not part of those selected for the formation of groups, are: tourism, construction, trade and services and other industries.
In terms of macro-variables that have lower values, there are: the authorities in public institutions, the macroeconomic situation, the legal framework, the allocation of resources, the technological framework as well as the socio-cultural and demographic ones. There are also minimum average values of other macrovariables like basic supply networks and basic services to the population.
Table 5 summarizes the relationships among macro-variables according to how much they influence one another. As Andalusia is the country's largest region, and has such varied geographical circumstances in the fifty-two rural counties that comprise it, the grouping of these counties was considered according to the influence of the macro-variables that define them. This would contribute to the integration of counties development strategies, enabling development policy measures to be applied to a larger territory than what corresponds to each individual county. In this sense, the results show that four clusters or groups of clearly different regions can be identified in terms of the variables motivating their development.
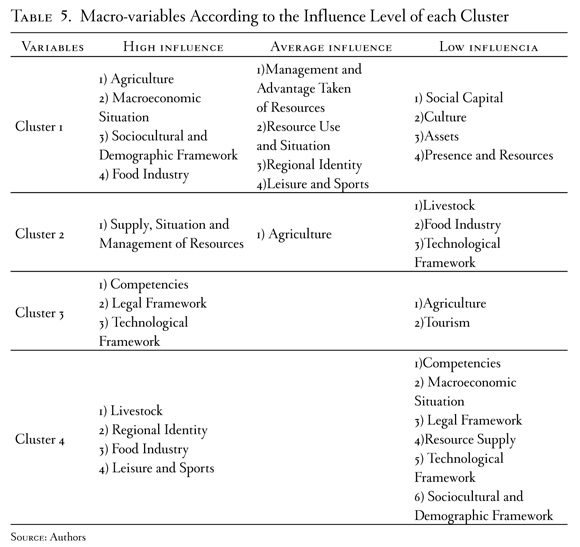
According to our theoretical framework, results highlights that county groups in each cluster show a clear participation of endogenous and exogenous factors although with differences among them. In this sense, we can appreciate that in cluster 1 exogenous factors are the macrovariables with the highest influential level while the less influential level corresponds to endogenous factors. Something similar occurs in the third place. But in the second cluster and, especially in the fourth one macrovariables with the highest influential level are the endogenous factors and the less influential ones are exogenous. This fact highlights that although methodology applied in the rural strategy is the same in each county, the perceptions made by each one has not followed the same behaviour according to the territorial rural development theory.
Observing counties composition in cluster 4 (9 in total), seven belong to mountainous area, 1 hilly area and the other one has agrarian production with a strong county identity. It is, in our opinion, the most homogeneous cluster besides cluster 1 with only three counties and a strong agrarian vocation. Cluster number 3 is the biggest one (21 counties), with a great geographical diversity (mixing 13 mountainous counties with 8 inland counties) and with a clear perceptions of influence of institutional and technological factors proba bly due to the lack of identity of some cases and to the remoteness from the highest developmental area in Andalusia.
Most of the counties included in the same cluster tend to have geographical proximity although there are exceptions (see Figure 3). Thus, for example, a first group with a strong economic bent was identified as being greatly influenced by the first sector and its derivative, the food industry. The second cluster is characterized by a greater influence of variables related to endogenous resources such as the amount, situation and management of resources. In this sense we can say that the counties included in this group are committed to the use of endogenous resources as their main driving force of development. The variables that primarily influence the development of the regions that make up the third group are mainly environmental ones. Therefore, public administration action, the legal norms related to the granting of incentives and assistance and the level of development of the technological framework all play a fundamental role. Finally, the counties included in the fourth group have a definite vocation for livestock together with its derivative industry, and another outstanding sector is tourism. Both macro-variables are therefore most influential in their development.
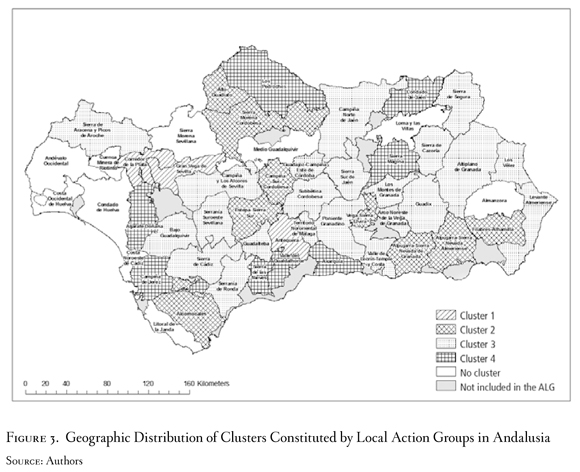
Conclusions
There is not a unique theory to explain European region development as it is explained by a mixture of theories which consider all endogenous and exogenous factors affecting the region development. Initiatives taken in EU rural development are mainly based on the endogenous development. Due to the complexity shown by EU regions and their counties, it is necessary to consider not only endogenous factors but also exogenous ones to build a suitable rural development strategy for each territory (Galdeano-Gómez, Aznar-Sánchez & Pérez-Mesa, 2011).
It is what we can appreciate in the new rural development strategy proposed by the regional government. Data analysis and their appropriate treatment has allowed to group 42 Andalusian counties into four different types according to the analysis made with the combination of the two methodologies (PSA and Cluster).
Although the applied methodology has made possible to group more than 80% of the counties in the four groups mentioned above, it has not been possible to group the 52 groups that composed the Andalusian rural territory.
Everyone knows about the scantiness and sometimes non-existence of statistical data for the variables that define rural areas. This is why decisions on development policies lack complete enough information and thus these decisions can be unreliable. With the application of the PSA, the absence of these statistics may be compensated for with guaranteed success, because decision making demands that the agents involved themselves must inform about the dependence and/or influence that variables defining a territory exercise on each other. Knowledge about the whole set of dependency and influence relationships among the variables that make up the territory will allow the government to take the decisions that will best affect the development of the territory.
However, we thinking that both methodologies can be very useful and a valid tool to aid in decision-making with respect to rural development policy. PSA provides information about the relationships of influence among the variables that define each of the counties analyzed, information that later will be used in cluster analysis to identify and characterize homogeneous regions. And these variables let to represent to endogenous and exogenous factors.
On the other side, the resulting information can be of great help to policy makers who are designing made-to-order development policy, namely, directed mainly toward the variables that the participants from the territory themselves considered as driving influences of development, and that are common to the county groups identified in each cluster. This will contribute to the integration of county strategies, building on synergies identified between regions and gaining a perspective for an overall development approach, as proposed in the NERA project objectives.
Finally, we believe that the research conducted in this article should go on to be completed once the current European framework programme has reached an end. Then we can make the necessary comparisons between what is designed in strategic planning, and the decisions taken by the regional government and local stakeholders themselves in each of the regions.
References
Ambrosio, M. & Delgado, M. (2008). Understanding Rural Areas Dynamics from a Complex Perspective. An Application of Prospective Structural Analysis. Paper on 12th Congress of the European Association of Agricultural Economists, Gante (Belgium), August 26-29. [ Links ]
Buchenrieder, G., Mõllers, J., Happe, K., Davidova, S., et ál. (2007). Conceptual Framework for Analysing Structural Change in Agricultural and Rural Livelihoods. Discussion Paper, 113. Last access on January 12, 2014, from http://www.iamo.de/dok/dp113.pdf [ Links ]
Bolanos, R., Fontela, E., Nenclares, A. & Pastor, P. (2005). Using Interpretive Structural Modelling in Strategic Decision-Making Groups. Management Decision, 43(6), 877-895. [ Links ]
Bryden, J. M. & Hart, K. (Eds.) (2004). A New Approach to Rural Development in Europe: Germany, Greece, Scotland and Sweden. Mellen Studies in Geography, volume 9. Queenston, Lampeter and Lewiston: Edwin Mellen Press. [ Links ]
Cecatto, V & Persson, L. O. (2002). Dynamics of Rural Areas: an Assessment of Clusters of Employment in Sweden. Journal of Rural Studies, 18, 49-63. [ Links ]
Convery, I., Soane, I., Dutson, T. & Shaw, H. (2010). Mainstreaming LEADER Delivery of RDR in Cumbria: an Interpretative Phenomenological Analysis. Sociologia Ruralis, 50(4), 371-391. [ Links ]
European Commission (July 29, 1988). The Future of Rural Society. Document COM (88) 501 end. Bulletin of the European Communities. European Commission (March 19, 1991). [ Links ]
Frochot, I. (2005). A Benefit Segmentation of Tourists in Rural Areas: a Scottish Perspective. Tourism Management, 26(3), 335-346. [ Links ]
Galdeano-Gómez, E., Aznar-Sánchez, J. A. & Pérez-Mesa, J. C. (2011). The Complexity of Theories on Rural Development in Europe: an Analysis of Paradigmatic Case of Almería (South-East Spain). Sociologia Ruralis, 51(1), 54-78. [ Links ]
García-Martínez, M. C., Balasch, S., Alcon, F. & Fernández-Zamudio, M. A. (2010). Characterization of Technological Levels in Mediterranean Horticultural Greenhouses. Spanish Journal of Agricultural Research, 8(3), 509-525. [ Links ]
Gavigan, J. P. & Scapolo, F. (November 2001). Regional Foresight - Future-Proofing and Validating Development Strategies. IPTS Report 59. Last acces on February 20, 2014, from http://vlex.com/vid/prospectiva-comprobacion-validacion-118774 [ Links ]
Godet, M. (1985). Prospective et planification strategique. London: Economica. [ Links ]
Godet, M. (1994). From Anticipation to Action: a Handbook of Strategic Prospective. Paris: United Nations Educational. [ Links ]
Godet, M. (2001). Creating Futures. Scenario Planning as a Strategic Management Tool. London: Economica. [ Links ]
Godet, M. & Bourse, F. (1989). MICMAC Ver.6.1.2. Laboratoire d'Investigation en Prospective, Stratégie et Organisation (LIPSOR). Last access on March 10, 2014, from http://www.laprospective.fr/methodes-de-prospective/les-methodes/51micmac.html [ Links ]
Goméz-Limón, J. A., Gómez-Ramos, A. & Sanchez, G. (2008). Análisis prospectivo de la agricultura en Castilla y León. Paper on 12th Congress of the European Association of Agricultural Economists, Vallad olid, España. [ Links ]
Goméz-Limón, J. A. & Gómez-Ramos, A. (2008). Análisis prospectivo de la agricultura en España. Revista Económica de Castilla-La Mancha, 13, 103-140. [ Links ]
González Fernández, M. (2006). Idas y vueltas en el desarrollo rural. De la diversificación de las economías locales a lo rural como categoría económica global. Revista Española de Estudios Agrosociales y Pesqueros, 211, 121-141. [ Links ]
Habegger, B. (2010). Strategic Foresight in Public Policy: Reviewing the Experiences of the UK, Singapore and the Netherlands. Futures, 42(1), 49-58. [ Links ]
Hair, J. F., William, C., Barry, J. B. & Anderson, R. E. (2009). Multivariate Data Analysis. México D.F.: Prentice Hall. [ Links ]
Hall, M. A. & Smith, L. A. (1998). Practical Feature Subset Selection for Machine Learning. Paper on Australian Computer Science Conference (181-191). Perth: Springer. [ Links ]
High, D. & Nemes, G. (2007). Social Learning in LEADER: Exogenous, Endogenous and Hybrid Evaluation in Development. Sociologia Ruralis, 47(2), 103-119 [ Links ]
Johnson, R. A. & Wichern, D. W (2002). Methods ofMultivariate Analysis. New York: Wiley-Interscience. [ Links ]
Junta de Andalucía (2008). Nueva Estrategia Rural para Andalucía. El proyecto NERA. Sevilla: Autor. [ Links ]
Llambí, L. (2004). Nueva ruralidad, multifuncionalidad de los espacios rurales y desarrollo endógeno. En E. Peréz, Desarrollo rural y nueva ruralidad en América Latina y la Unión Europea (pp. 91-107). Bogotá D. C.: Pontificia Universidad Javeriana. [ Links ]
Lloyd, S. P., 1982. Least Squares Quantization in PCM. IEEE Transactions on Information Theory, 28(2), 129-137. [ Links ]
Macqueen, J. B. (1967). Some Methods for Classification and Analysis of Multivariate Observations. Proceedings of 5th Berkeley Symposium on Mathematical Statistics and Probability. Berkeley: University of California Press. [ Links ]
Markov, A. A. (1971). Extension of the limit theorems of probability theory to a sum of variables connected in a chain. Dynamic Probabilistic Systems, volume 1: Markov Chains. Chichester: John Wiley and Sons. [ Links ]
Mingers, J. & Rosenhead, J. (2004). Problem Structuring Methods in Action. European Journal ofOperational Research, 152(3), 530-554. [ Links ]
Pardos, L., Maza, M., Fantova, E. & Sepúlveda, W (2008). The Diversity of Sheep Production Systems in Aragon (Spain): Characterization and Typification of Meat Sheep Farms. Spanish Journal of Agricultural Research, 6(4), 497-507. [ Links ]
Pérez, E. (2002). Lo rural y la nueva ruralidad. En E. Pérez y J. Sumpsi, Políticas, instrumentos y experiencias de desarrollo rural en América Latina y Europa. Madrid: Ministerio de Agricultura, Pesca y Alimentación. [ Links ]
Pérez, P. P., Martín, J. M. & Ambrosio, A. (2009). Prospective Structural Analysis: An application to Rural Development Strategies in the 83rd Annual Conference of the Agricultural Economics Society, Dublin, Agricultural Economics Society. [ Links ]
Ray, C. (1998). Territory, Structures and Interpretation-Two Case Studies of the European Union's LEADER I programme. Journal of Rural Studies, 14, 79-87. [ Links ]
Rosenhead, J. (1996): What's the problem? An Introduction to Problem Structuring Methods. Interfaces, 26(6), 117-131. [ Links ]
Schlange, L.E. & Jüttner, U. (1997). Helping Managers to Identify the Key Strategic Issues. Long Range Planning, 30(5), 777-786. [ Links ]
Schejtman, A. & Berdegué, J. (2003). Desarrollo territorial rural. En R. Echeverría (Ed.), Desarrollo territorial rural en América Latina y el Caribe. Washington D.C.: Banco Interamericano de Desarrollo. [ Links ]
Sumpsi, J. M. (2006). Experiencias de desarrollo rural con enfoque territorial en la Unión Europea y América Latina. Revista Española de Estudios Agrosociales y Pesqueros, (209), 41-71. [ Links ]
Terluin, I. J. & Post, J. H. (Eds.) (2000) Employment Dynamics in Rural Europe. Wallingford: CABI Publishing. [ Links ]
Torres, M., Hervás, C. & García, C. (2009). Multinomial Logistic Regression and Product Unit Neural Network Models: Application of a New Hybrid Methodology for Solving a Classification Problem in the Livestock Sector. Expert Systems with Applications, 36, 12225-12235. [ Links ]
Vázquez-Barquero, A. (2002). Endogenous Development Networking, Innovation, Institutions and Cities. London and New York: Routledge. [ Links ]
Ward, J. H. (1963). Hierarchical Grouping to Optimize an Objective Function. Journal of the American Statistical Association, 58(301), 236-244. [ Links ]
Ward, N., Atterton, J., Tae-Yeon, K., Lowe, P., et ál. (2005). Universities, the Knowledge Economy and the Neo-Endogenous Rural Development. Discussion Paper 1, Centre of Rural Economy, Newcastle University. [ Links ]
Witten, I. H. & Frank, E. (2000). Weka Machine Learning. Data Mining Software Release 3.4.0. [ Links ]