Introduction
Potato (Solanum tuberosum) is an essential crop for food security and the economic sustainability of several agricultural households worldwide (Campos & Ortiz, 2019), especially in the Nariño province, Colombia, where it is one of the three most important crops, along with coffee and plantain. Based on official data from the Colombian government, reported through Municipal Agricultural Assessments (Ministerio de Agricultura y Desarrollo Rural [MADR], 2020), we calculate that between 2007 and 2019, the total national production of potato grew at an average yearly rate of 2.4% for first half cycles and 3.3% on second halves (Supplementary materials, Table SM1). Meanwhile, the total harvested area increased at average yearly rates of 0.5% and 1.4% for the first and second halves, respectively. Nariño shows different patterns, with higher productivity growth rates during the first half seasons (2.9% compared to 1.9%), and more significant acreage growth rates than the national trend, with an annual 2.4% for first and 1.6% for second halves.
Furthermore, such different patterns between Nariño and the rest of Colombia are reflected in yield trends. During these 13 years, the overall national yield of potatoes grew at a rate of 1.8– 1.9%, while in Nariño, it had an average growth of 0.4–0.5%, although such growth does not appear to be statistically significant. In comparison, while the national potato yield went from 19.59 to 22.81 tons per hectare, Nariño went from 18.74 to 20.35 in the same metric. Hence, since the increase is statistically insignificant (SM, Table SM1), Nariño is not catching up with the national trend. Moreover, as prices perceived by farmers are becoming relatively steady (SM, Figure SM1), potato farmers in Nariño are in a likely urgent need to find either means to increase their productivity (and thus remain competitive) or means to change their agricultural and commercial practices to access (or create) high-value markets (Martinez-Delgado et al., 2014). Otherwise, their limited competitiveness will vulnerate their livelihoods in both the short and long run.
Miranda (2010) provides a thorough qualitative characterization of potato production in Nariño. Her findings suggest that production fits a traditional structure in developing countries; production units are passed down from one generation to the following, with relatively steady practices regarding input use and labor allocation, which explains why yields remain considerably low. Furthermore, the results of Miranda (2010) highlight how the technological capacities of these farmers are minimal. For example, machinery used for soil preparation and harvesting is scarce. As these practices are labor-intensive, and a lack of mechanization can negatively affect effective plot productivity and final exploitation, there is a clear need to improve capacities. Policy and research efforts should be in place to provide sustainable mechanisms that help improve farmers’ capabilities and means of living.
Although technology transfer could promote much-needed changes, is it enough to deliver the necessary transformation? Unfortunately, attempts to transfer technology to farmers may not imply results as good as suggested by experimental trials (Laajaj et al., 2020), nor as good as desired by policymakers. The literature provides evidence on how technology adoption can be successful when farmers learn about it either by experimenting with it themselves or learning from others (Conley & Udry, 2010; Foster & Rosenzweig, 1995). Nonetheless, it is also apparent that its final impact is exceptionally constrained in developing countries (Magruder, 2018). Market imperfections are usually considered the most relevant cause in differences between expected and observed changes from technology adoption. However, a new growing body of literature suggests that differences between controlled experimental trials and real-life farming conditions and adoption patterns could be better determinants (Abate et al., 2018; Dar et al., 2013; Laajaj et al., 2020). The latter, along with the need to understand how and on what dimensions the potato production system of Nariño can be improved, further motivates our research.
One standard approach to exploring agricultural production systems’ structure is the stochastic frontier analysis (Kumbhakar & Lovell, 2000). This method models the relationship between observed outputs with inputs and associated practices and defines a frontier (i.e., an optimum) to determine how efficiently individuals perform within a sample (or population) of interest. For example, if the average efficiency is said to be 50%, this means that the average farmer would only get half of what they could get in their production system. The literature is vast, but it is worth highlighting some works that take a particular focus on potato farming. Melesse and Ahmed (2015) make a comparative analysis of the productive efficiency of potato farmers in Ethiopia, capturing the differences between efficiency levels of irrigated and rainfed systems. Beyond the considerable differences in this metric, the authors emphasize that it will be crucial to meet better the needs of rainfed-based farming households, which are at a clear disadvantage when entering the market.
In another work in Ethiopia, Wassihun et al. (2019) find that the average efficiency level among potato farmers in the Amhara State is 46%; there is plenty of room for improvement. Moreover, the authors reveal that animal-powered soil preparation, fertilization, and the quantities of seeds used when establishing the crop are significant drivers of improved outputs. Focused on Irish potato farmers in Kenya, the analysis by Nyagaka et al. (2009) indicates that the average efficiency of farmers is also low (around 39%) and that there exist decreasing returns to the scale of production. Nevertheless, evidence suggested that education and membership to farmers’ associations positively relate to efficiency; thus, improvements towards farmers’ connectedness and access to education could boost production returns. Similar results were found in Bangladesh by Hossain et al. (2008). Despite a higher average efficiency among potato farmers (75%), they also suggest that investments in extension linkage and increasing seed use levels can significantly drive achievable production.
This research attempts to identify the most relevant attributes that a technology transfer program should include to increase the odds of successfully creating impact. The first goal of this study was to identify types of farmers that describe the production of potatoes in Nariño based on relevant socioeconomic and technical attributes, arguing how this kind of information can better help construct successful technology transfer efforts. As a second goal, we aimed to build a measure of technical efficiency across potato farms in Nariño to compare how it differs among types of farmers identified from the first objective. Both are valuable sources of information for policymakers looking to strengthen, from the bottom up, the potato sector of Nariño.
Materials and methods
This study relied on a representative sample of 1,018 farm households in Nariño. Participants were interviewed during the first half of 2016 and provided detailed information via a structured survey. The sample size was determined by simple random sampling (Levy & Lemeshow, 2008), based on an estimated population of 16,861 potato farming households, a confidence level of 98%, and an accepted error margin of 2%. These figures were based on information provided by the Departmental Government of Nariño, which also helped determine the collection area to achieve data representativity at the department level. Different from the available farm-level anonymized micro-data from the 2014 Agricultural Census, this survey allowed collecting detailed information on specific agricultural practices for potato farming at the plot level, as well as socioeconomic and family-related information. To our knowledge, this sample is the single department-level representative source of information about potato production available in Colombia.
Clustering data to identify types of farmers with statistically common attributes (Bartholomew et al., 2008) is a standard practice in descriptive and analytic agriculture studies in Colombia, with known examples in crops such as lulo (Solanum quitoense) (Gallego et al., 2004) and cacao (Theobroma cacao) (Mantilla-Blanco et al., 2000). To this end, this paper followed Ward’s linkage approach. This hierarchical method repeatedly identified groups of mutually exclusive subsets of observations based on their attributes’ (dis)similarity (Ward, 1963). To build a more robust analysis, technical variables that capture or directly affect the performance of potato farmers were used, namely: mechanization of labor (manual, mixed, mechanical), the elevation of the farm, farm size, area dedicated to potato crops, irrigation, and overall production. In addition, we included various socioeconomic variables that could indirectly affect productive outcomes: household size, age of the household head, monthly household income, type of farm labor used (hired, paid family labor, or unpaid family labor), access to technical assistance and credit, household size, multidimensional poverty index, and household’s cultural self-identification (peasant, indigenous, mestizo, or none). Finally, by analyzing the dendrogram structure produced by the adjusted dissimilarity measure (Ward, 1963), we determined the most suitable number of groups representing the types of potato farmers in Nariño.
A stochastic frontier model (Aigner et al., 1977; Meeusen & van den Broeck, 1977) was adjusted for potato production at the plot level to build a measure of technical efficiency. This stochastic frontier was adjusted for the whole sample, allowing us to compare an efficiency index across types of farmers detected in clustering. We followed a Cobb-Douglas production function (Greene, 2012; Nicholson & Snyder, 2012), adjusting it for production at a plot level 𝑗 for a farm 𝑖, where the theoretical model is:
such that 𝑞𝑖𝑗 is the production (kg) of potato of plot 𝑖 at the farm household 𝑗, which is determined by technical factors at the plot-level (𝑥𝑖𝑗𝑘) and at the farm level (𝑑𝑖ℎ). Also, there is a random shock 𝐴 that follows:
with 𝜀𝑖𝑗 being a composite error. It was assumed that 𝜀𝑖𝑗 is made of an independently and identically distributed (iid) error 𝑢𝑖𝑗, and a non-negative measure of inefficiency 𝑢𝑖𝑗, independent of productive technical factors since they are already included as explanatory variables. Therefore, taking logs in (1) the model yielded
Note that binary regressors can be added into a final empirical specification, assuming 𝑑𝑖ℎ = exp(𝑏𝑖ℎ), where 𝑏𝑖ℎ is a binary covariate; these are commonly known as frontier shifters (e.g., see Centorrino et al., 2021). The covariates used to econometrically model potato’s stochastic frontier production function and its variance are summarized in table 1; categorical covariates were split into binary variables, omitting a base category.
Table 1 Variables used in the stochastic frontier regression model to estimate productive technical efficiency of potato farmers Nariño Southwest Colombia 2016
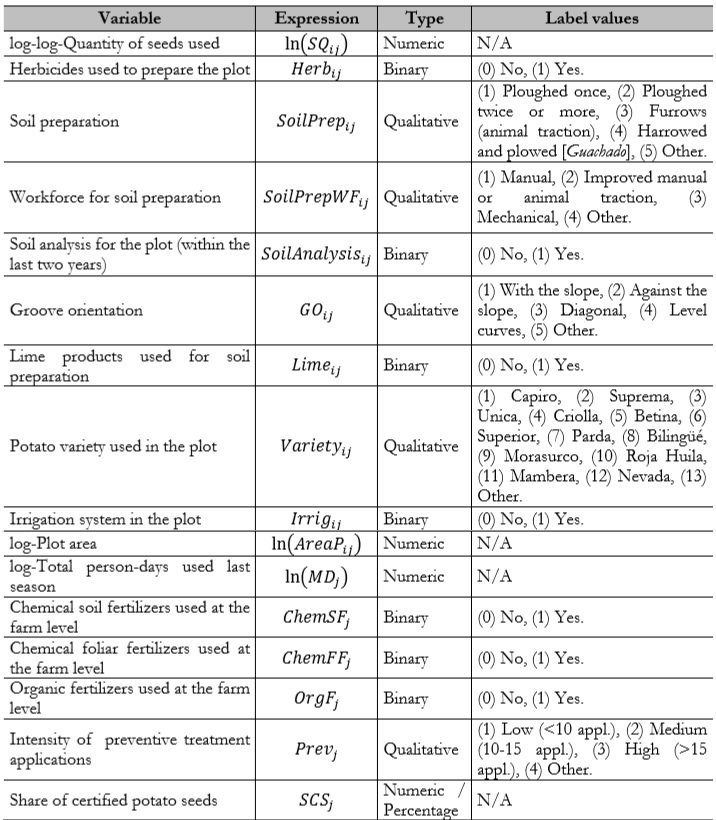
Source: Elaborated by the authors.
More specifically, the final expression for our stochastic frontier model was set as:
while the variance of the technical inefficiency measure was defined as a linear function of additional technical covariates:
with 𝜁𝑖𝑗 an iid random shock.
Since 𝑢 and 𝑢 are independent, then the probability density function of 𝜀 is expressed as mixed density (Belotti et al., 2013):
so that the maximum likelihood coefficient estimates for 𝜽 = (𝖰, 𝜹) optimized a target function:
where the marginalization of 𝑢, assuming a normal-exponential distribution, provided a closed- form that quickly converges to a unique solution (Belotti et al., 2013). Since the theoretical 𝑢 is a measure of inefficiency, a straightforward index of technical efficiency at the plot-level was:
For the final analysis, an ordinary linear regression model was used to determine the correlation between the overall efficiency measure and the identified types of farmers from the hierarchical cluster analysis. This approach was helpful to understand better how relevant attributes captured at the farm level may be related to measures of efficiency at the plot level (i.e., detecting whether farmers in “better” preliminary conditions were systematically more efficient than those who were less favored). It is worth highlighting that letting the stochastic frontier be based on the whole sample allows us to compare the efficiency measures across typologies directly. Estimating separate models for each typology would prevent explicit comparisons. For example, in such a case, 80% of average efficiency in one group and 80% on another would not tell whether they are equal or even close since the frontiers would come from different data generating processes.
Results
The average farm is at around 3,040 m a.s.l., with farm sizes ranging between 3.0 and 3.5 ha, and 90-95% of the land is dedicated exclusively to potato production. Also, these farms report an average yearly production of 47.6 tons, so the average yield ranges between 13.6 and 15.8 tons per hectare (table 2). Most of these households do not have access to credit (53%). Nevertheless, they have successfully overcome poverty vulnerability (over 77.3% of the time), and the only ethnic identification detected in a significant number of cases (24%) is indigenous (table 3). Otherwise, households either identify as peasant families (58.8%) or do not consider that any specific cultural or ethnic attribute describes them (15%). The workforce of potato farms is composed chiefly of paid (52.1%) and un-paid (37%) family labor and such works are either purely manual (44.1%) or mechanically-aided (49.3%). Use of technical assistance is low (26%), as most households have low or no access to it (jointly, they are over 70% of the sample), and just over 35% of them are part of a farmer’s association that provides it.
Table 2 Descriptive statistics of continuous variables used to identify types of potato farmers Nariño Southwest Colombia 2016
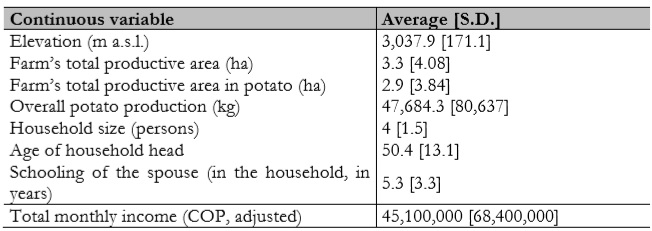
Source: Elaborated by the authors.
Table 3 Descriptive statistics of binary and categorical variables used to identify types of potato farmers Nariño Southwest Colombia 2016
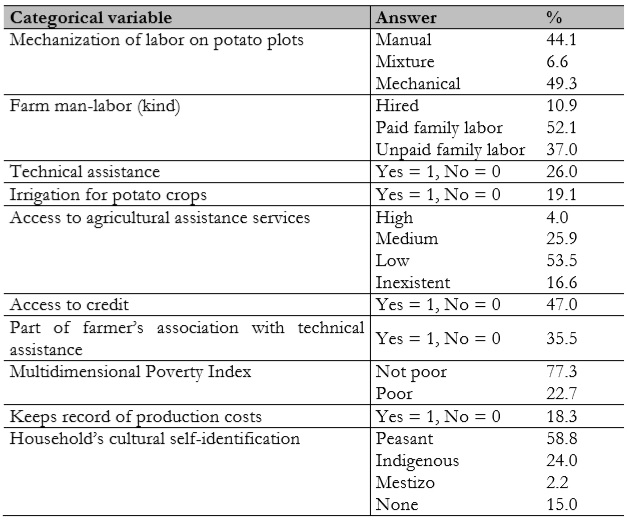
Source: Elaborated by the authors.
However, this view of the average farm does not fully reflect the different conditions that may describe farmers within comparable groups. Hence, detecting the types of farmers and their attributes gives a sense of group-specific attributes and how those groups describe the overall potato production system in Nariño. The result of the repetitive hierarchical algorithm of Ward’s clustering is described in figure 1, with a dashed line indicating the recommended cut-off for the number of groups (i.e., latest clades above the dashed line) that more efficiently reflect the variance within the sample. We follow Priegnitz et al. (2019) and Bartholomew et al. (2008) in defining groups based on dendrogram analysis and test for the difference in all covariates among groups for robustness (SM, table SM2). Since not all observations in the sample have the complete set of information for all the variables used in the clustering analysis, the farmer type was detected for a subsample of 773 farm households. Descriptive statistics by group are summarized in tables 4 and 5.
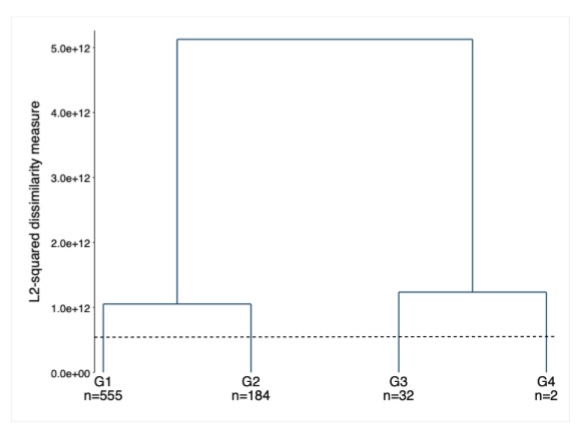
Figure 1 Dendrogram of cluster identification of potato farmer types [G(i) is a typology]. Nariño, Southwest Colombia, 2016. Source: Elaborated by the authors.
Table 4 Descriptive statistics mean of continuous variables among detected typologies of potato farmers Nariño Southwest Colombia 2016
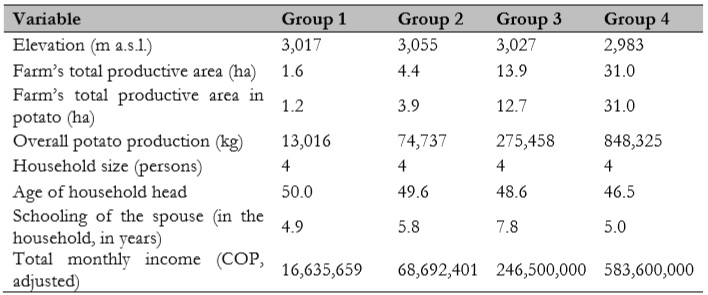
Source: Elaborated by the authors.
Table 5 Descriptive statistics percentages of binary and categorical variables among detected typologies of potato farmers Nariño Southwest Colombia 2016
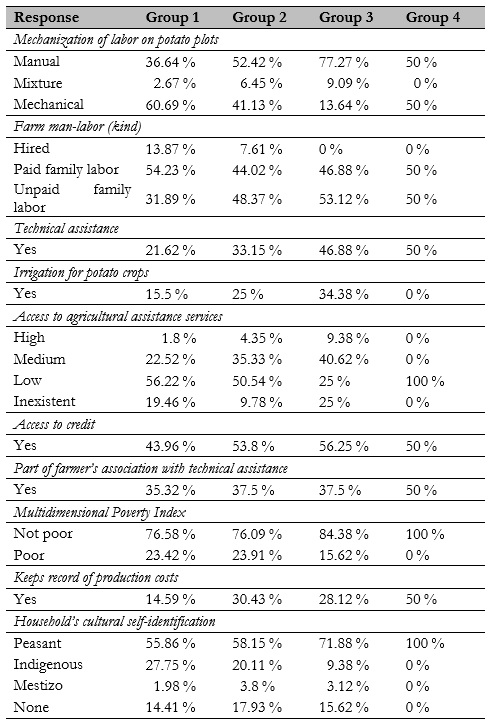
Source: Elaborated by the authors.
Based on the recommended clusters (figure 1), we describe the four potato farmer types in Nariño, which follow the summarized statistics in tables 4 and 5. Although the name of the descriptions is tied to the scale of farming, it does not mean that the critical differences observed in other attributes are discarded; instead, we follow a scale-based definition simply because it shows the most significant statistical difference.
Micro-scale farmers (n1= 555). Productive households with an average acreage of 1.6 ha and a dedication of 1.2 ha to potato production. Their yearly overall potato production is around 13 tons. Their tracking on production costs is severely low (14.5%), and most of these farmers have either no direct or low access to technical assistance (75.6%), nor are part of a farmer’s associations that can provide it (74.6%). Also, they are characterized by minimal use of irrigation systems (15.5%), and most of them lack access to credit (56%). Farmers in this section are self- identified mainly by their race (indigenous or mestizo, combined for 29% of cases), and one in four is considered poor.
Small-scale farmers (n2 = 184). Productive households with an average acreage of 4.4 ha and dedication of roughly 3.9 ha to potato production. Their yearly production of potatoes is around 74.7 tons. A third of them (33.1%) receive technical assistance, either directly or through farmer’s associations that can provide it, although most suggest that the access is relatively low (50.5%). In this section, most farmers have access to credit services (53.8%), but now one in four uses irrigation for their potato crops. The self-identification as indigenous or mestizo ethnic origin happens in about one in four cases, and poverty conditions are observed in about 24%.
Medium-scale farmers (n3= 32). Farmers with a total potato acreage of 12.7 ha within farms that roughly reach 14 ha in total size. With an average yearly production of 275.5 tons, these farmers report a higher rate of access to technical assistance (46.8%) than micro and small farmers, now with over 50% of the access being medium or high. In this segment of farmers, irrigation (34.3%) and access to credit (56.2%) are more common. Also, starting from this segment, the representation of self-identification with indigenous or mestizo ethnic origins now falls to 12.5%, showing a non-trivial inequality in capacities between non-ethnic and ethnic communities. Finally, poverty is only observed in around 15% of households.
Large-scale farmers (n4= 2). We are cautious and restrain from any in-depth interpretation of this category due to the constrained size, yet decide not to omit it; this follows that large scale farmers are “scarce” (in terms of the number of farmers) but significant in their relative share of the land. Our sample’s big-scale farmers have an average farm size of 31 ha, with their whole land dedicated to potato production. Their yearly average production is above 800 tons. We detect access to credit (one in two), which makes sense based on the size of their landholdings. These farmers keep a strict record of their production costs, which is understandable from the economy of scale. None of them are considered poor, and none self-identifies with an ethnic origin.
These identified farmer types make evident relevant asymmetries in conditions potato farmers face in Nariño. Thus, it would be naive to expect homogeneous technology packages to be easily transferred and adopted by a heterogeneous population. Assessing these differences is helpful to design better-targeted interventions, differentiate specific needs of specific transects of farmers, and increase the possibilities of adopting recommendations or technologies and their related overall impacts. These first results lead to building a measure of technical efficiency and comparing how each farmer type varies. Tables 6 and 7 summarize the descriptive statistics of the variables at the plot level used in the stochastic frontier regression model for the subsample of 773 households previously categorized in the cluster analysis.
Table 6 Summary of continuous and binary variables used in the stochastic frontier regression model for technical efficiency of potato farmers Nariño Southwest Colombia 2016
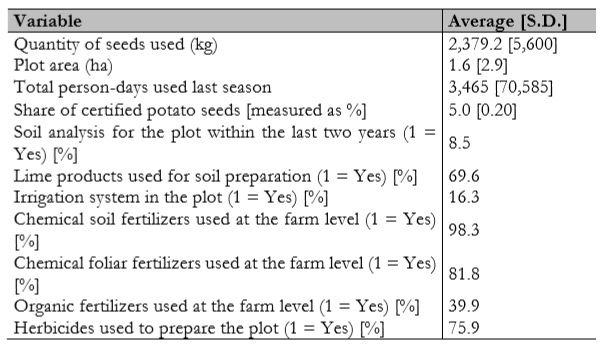
Source: Elaborated by the authors.
The average plot has about 1.6 ha and is established with over 2.3 tons of seeds. Only 8.5% of the plots revealed soil analysis to help make informed input use decisions, and roughly 5% of the seeds are certified. Different kinds of fertilizer use could be reported, so these do not add to unity. Nearly all plots (over 98%) received chemical soil fertilization, roughly four out of five made use of foliar fertilization, and just below 40% received some form of organic fertilizers. A large share of the plots made use of chemical herbicides (75.9%), as also happens with the use of lime products (69.6%) to reduce the acidity of the soil in its preparation, while the intensity of preventive treatments is relatively high (87.6% make over ten applications yearly). Unlike the farm level, data analyzed at the plot level reveals that roughly 16.3% have an established irrigation system for potato production. While the most significant proportion of the workforce used for soil preparation is purely manual (44.5%), a significant part of used labor was improved manual labor (semi-mechanization or animal traction) and mechanic means, with proportions of 27.4% and 26.5%, respectively. In addition, most farmers set their plot’s grooves either with the slope (43.4%) or decidedly against the slope (40.9%) of the terrain.
Table 7 Summary of categorical variables used in the stochastic frontier regression model for technical efficiency of potato farmers Nariño Southwest Colombia 2016
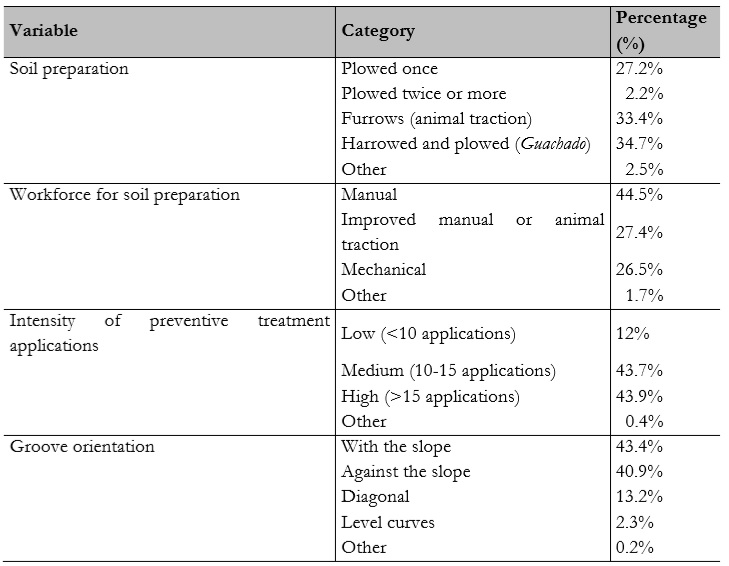
Source: Elaborated by the authors.
Previous testing (not reported here) suggests no significant relation between potato productivity and the use of specific varieties within our sample. However, this is usually an essential factor in producing any crop. Specific varieties, either improved or with better genetic attributes, may reduce the output variance (i.e., reduced uncertainty). Therefore, the main variety used in the plot (Table 8), as reported by the farmer, is included as a determinant of the inefficiency measure variance. The main four varieties—whose local names are Capiro, Suprema, Única, and Criolla—are identified in 82% of the plots, so if specific effects of other varieties are detected, it could be the case of a point inflated (deflected) variance due to outlier data.
Table 8 Selfreported main varieties used by farmers in Nariño on their potato plots Southwest Colombia 2016
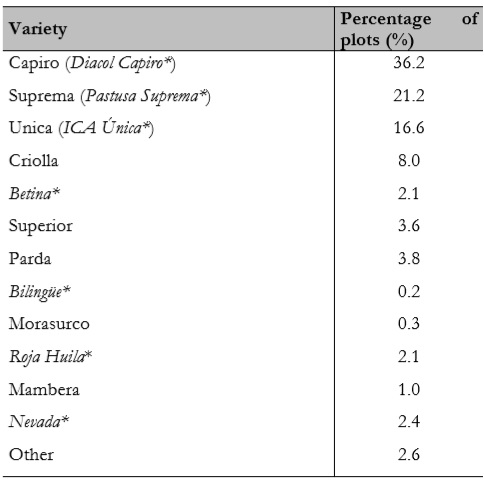
*Registered name of a variety Other varieties are grouped based on the reported name but cannot be attributed to a specific registered variety
Source: Elaborated by the authors.
Results from the stochastic frontier regression and the factors affecting the (in)efficiency measure variance are summarized in tables 9 and 10, respectively. Evidence indicates that only four main factors are significantly correlated with the productivity of the plots, yet the elasticities are at levels less than unity. A one percent increase in plot size increases production by about 0.6%, while an increase of the same scale in the number of seeds used is related to an approximate 0.39% increase in the plot’s production. The application of chemical foliar fertilization, on average, is related to an increase of 10.4% of the expected production of a plot. Finally, even though significant, the elasticity relation concerning total use of person-days is roughly 0.04%. While other factors, such as the share of certified seeds, organic fertilization, and specific categories of the intensity of preventive treatment reported coefficients with the expected sign, such correlations were not statistically significant, so their null hypotheses (coefficients equal to zero) cannot be rejected.
Table 9 Estimates from stochastic frontier regression of production among potato plots in Nariño Southwest Colombia 2016
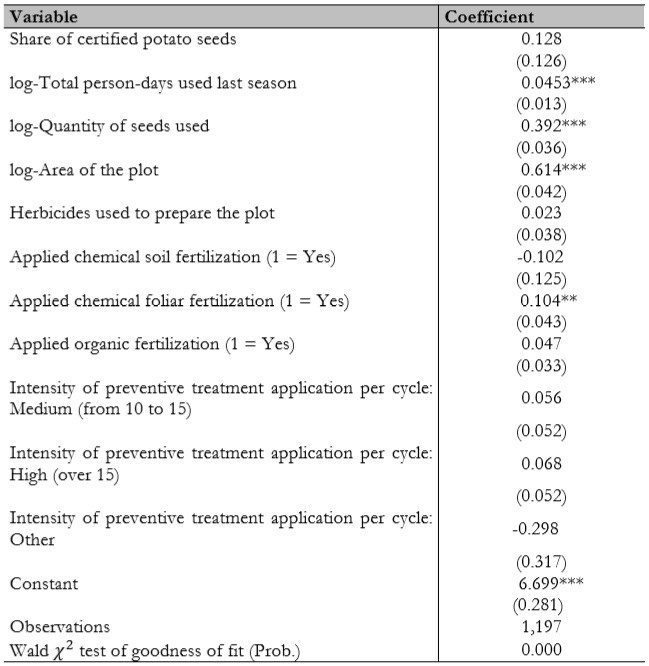
Robust standard errors in parentheses.
***p < 0.01
**p < 0.05
*p < 0.1
Source: Elaborated by the authors.
Two leading agricultural practices stand out when analyzing the factors associated with the (in)efficiency measure variance in the auxiliary regression (table 10). The local practice, guachado or wachado(Volverás-Mambuscay et al., 2020), reports a significant reduction in the expected variance concerning its base category, i.e., single plowing, a striking result; this practice is a minimum-tillage and sustainable alternative to other soil preparation techniques. On the other hand, using lime in the soil to reduce its acidity has an expected effect, significantly reducing the variance. However, systematic use of the product to adapt the soil to the crop’s needs may raise the question of whether the allocation of the current land is optimally used to produce a potato. Although “other practices” for soil preparation also reflect a significant difference concerning its base category, this is likely an outlier effect responding to barely 2% of the plots (recall table 7). The same holds for the potato variety “Mambera,” where we detected an effect concerning the base category (Capiro variety). Since the Criolla variety in Colombia is a small potato, the reported significant increase in the (in)efficiency variance was expected, as its production is of a different scale, hence reflected in this coefficient.
Table 10 Inefficiency variance factors from stochastic frontier regression of potato farmers Nariño Southwest Colombia 2016
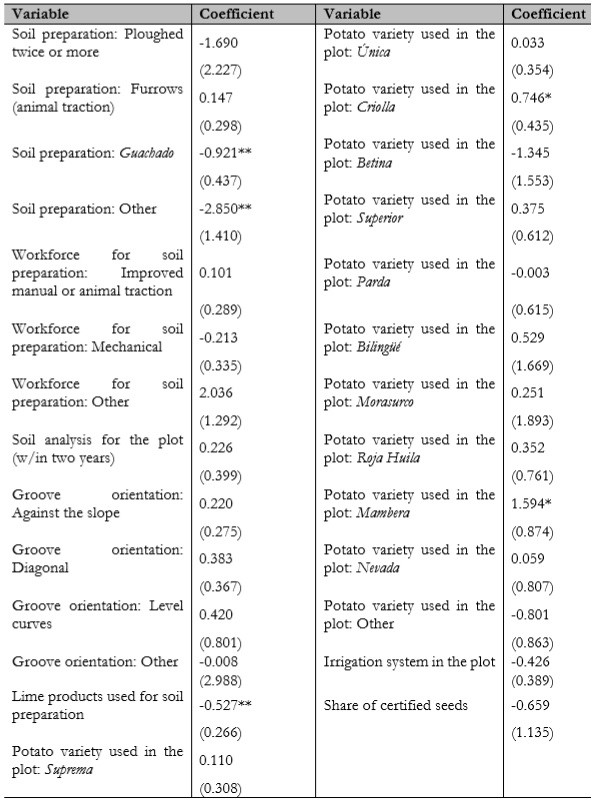
Robust standard errors in parentheses.
***p < 0.01
**p < 0.05
*p < 0.1
Source: Elaborated by the authors.
Finally, the two previous analyses (clustering and stochastic frontier) result in the concluding study of the relationship between the estimated index of technical efficiency across plots and the types of farmers managing such plots, summarized in the linear regression coefficients in table 11. While the detected and defined types of farmers are qualitative, they can describe an increasing scale of favorable conditions from 1 to 4, taking micro-farmers as a base category. The latter has a 79.4 % efficiency % on average, concerning those making the most out of input use (across the whole sample). Following an increasing and additive analysis, average small-scale and medium-scale farmers have an additional 5.9% (total 85.3%) and 7% (total 92.3%) of relative efficiency, respectively, compared to the most efficient farmers. However, large-scale farmers do not present a statistical difference in their efficiency compared to micro-farmers, yet this result should be treated carefully due to the considerably limited number of large-scale farmers available in the sample.
Discussion and concluding remarks
Our data clustering analysis suggests that most potato farmers (71.8%) in Nariño are micro- scale farmers who report low levels of innovation and limited access to credit, mechanization, and irrigation, along with a more considerable incidence of poverty in other farmers. These factors imply a harsh set of initial conditions that these farmers must overcome when entering or remaining in the market. Similarly, small-scale farmers, who are roughly 23.8% of the sample under analysis, also report limited access to credit and low adoption of improved production practices, despite being at a slightly better level than micro-scale farmers. Hence, with nearly 95% of farmers starting from a position of comparative disadvantage, creating change may not follow traditional technology transfer strategies (e.g., knowledge dissemination and exchange), as their needs for change are structural.
The previous does not necessarily imply that all micro- and small-scale farmers are considerably inefficient concerning the overall potato production system in Nariño. The average efficiency among micro-farmers is estimated to be close to 80%, whereas, for small farmers, it goes up to 85%. Nevertheless, differences still exist, and there is room for improvement. To better target the needs of potato micro-farmers, changes must be assessed both by policymakers and stakeholders in the potato production and value chain. While the liberalization of agricultural markets has meant an improvement in the consumption bundles (and the quality of life) of those in developed countries, it also worsened the livelihoods and capabilities of resource-poor farmers and the environment in developing countries (Dragun, 1999).
Government interventions should also address micro and small farmer strengthening via the public provision of high-quality education. As a proven mechanism to reduce poverty among rural households (Segrelles-Serrano & Vásquez-Sanchez, 2012), it would also remove the constraints on the needed structural change for micro-scale farmers. Moreover, providing extension services and promoting associativity could be a strategy. In addition to being attributes that partly describe farms reaching the highest efficiency in our study, these are also mechanisms identified to boost technology adoption among small- and medium-scale farmers in Latin America (Martinez et al., 2021).
Bottom-up interventions that rely on farmer-level planning to better exploit alternative markets or new commercialization opportunities to promote macro-level impacts are not unheard of in Latin America (Henry et al., 1999). Furthermore, these could prove successful in improving the quality of life of potato micro-farmers in Nariño. Although these farmers do not provide a significant portion of the overall trade in the sector, efforts in achieving their productive sustainability go hand in hand with guaranteeing their food safety. Similar experiences have taken advantage of non-formal social networks to directly connect small-scale farmers with markets located at a short distance (Contreras-Díaz et al., 2017) and proved successful in reducing intermediation margins and promoting consumption of agroecological products.
Small- and medium.scale farmers should prioritize the sustainability and resilience of their crops. Locally adapted technology transfer programs from universities or research institutes could improve their innovation capabilities and competitiveness (Kababe et al., 2018), together with SDGs. To this end, strengthening the National System of Agricultural Innovation (Law 1876/2017, Colombia) is of the utmost importance. Promoting contract farming could better integrate small- and medium-scale potato farmers into the value chain through its Agricultural Extension Subsystem. However, this commercial strategy must go along with recommendations of crop diversification, helping reduce the dependence on a single crop (i.e., lesser risk of financial loss from market shocks) and benefitting production, as crop diversity and rotation improves both the quality of soils and their potatoes (McDaniel et al., 2014; Twardowski et al., 2016) compared to monocropping systems.
On a final and side note, it is also worth stressing the need to enforce environmental policies that better help reconvert conventional agriculture into agroecological farming (Law 1930/2018, Colombia). Many of the farms analyzed here (particularly micro-farmers) produce well above 3000 m.a.s.l., hence endangering the ecosystems of the Nariño moors (páramos). For all scales (or types) of potato farming in Nariño, all transformation policies should undoubtedly aim to maintain the functionality of high mountain ecosystems while procuring to be aligned with climate change adaptation and mitigation needs.