Servicios Personalizados
Revista
Articulo
Indicadores
-
Citado por SciELO
-
Accesos
Links relacionados
-
Citado por Google
-
Similares en SciELO
-
Similares en Google
Compartir
Ingeniería y Universidad
versión impresa ISSN 0123-2126
Ing. Univ. vol.18 no.2 Bogotá jul./dic. 2014
https://doi.org/10.11144/Javeriana.IYU18-2.mpmr
Mathematical Programming Modeling and Resolution of the Location-Routing Problem in Urban Logistics1
Modelación en programación matemática y resolución del problema de localización-ruteo en logística urbana2
Andrés Felipe Muñoz-Villamizar3
Jairo R. Montoya-Torres4
Nilson Herazo-Padilla5
1Reception date: April 19,h, 2013. Acceptance da: June 27th, 2014. The work presented in this paper was developed by Operations & Supply Chain Management Research Group (formerly Logística y Negocios Internacionales) from Universidad de La Sabana, Chía, Colombia, under the framework of ANNONA Research Project funded by the French National Association for Research (ANR) and by Universidad de La Sabana, Colombia, under Research Grant EICEA-86-2014.
2Fecha de recepción: 19 de abril de 2013. Fecha de aceptación: 27 de junio de 2014. Este artículo se deriva de un proyecto de investigación denominado ANNONA, financiado por la Agencia Nacional Francesa de Investigación (ANR) y por la Universidad de La Sabana, Chía, Colombia, bajo el código EICEA-86-2014, desarrollado por el grupo de investigación Operations & Supply Chain Manageent (anteriormente Logística y Negocios Internacionales).
3Industrial Engineer, Escuela Colombiana de Ingeniería Julio Garavito, Bogotá, Colombia. Master in Operations Management, Universidad de La Sabana, Chía, Colombia. Currently Part-Time Lecturer at the School of Economics and Management Sciences, Universidad de La Sabana. E-mail: andresmuvi@unisabana.edu.co.
4Industrial Engineer, Universidad del Norte, Barranquilla, Colombia. Master of Science in industrial Engineering and Management, Institut National Polytechnique de Grenoble. PhD in Industrial Engineering, Ecole Nationale Supérieure des Mines de Saint-Étienne, France. Full professor and director of the Operations & Supply Chain Management Research Group, School of Economics and Management Sciences, Universidad de La Sabana, Chía, Colombia. E-mail: jairo.montoya@unisabana.edu.co.
5Industrial Engineer, Universidad de la Costa (CUC), Barranquilla, Colombia. Master in Operations Management, Universidad de La Sabana, Chía, Colombia. Currently Lecturer at the Department of Industrial Engineering, Universidad de la Costa, Barranquilla, Colombia and Junior Researcher at Fundación Centro de Investigación en Modelación Empresarial del Caribe (FCIMEC), Barranquilla, Colombia. E-mails: nherazo1@cuc.edu.co and nherazo@fcimec.org.
How to cite this article
MUÑOZ-VILLAMIZAR, A. F.; MONTOYA-TORRES, J. R., and HERAZO-PADILLA, N. Mathematical Programming Modeling and Resolution of the Location-Routing Problem in Urban Logistics. Ingeniería y Universidad. 2014, vol. 18, no. 2, pp. 271-289. http://dx.doi.org/10.11144/Javeriana.IYU18-2.mpmr
Abstract
The implementation of urban distribution centers near to city centers to allow freight consolidation is a widely extended initiative worldwide, seeking to improve traffic congestion and quality of life in downtown, among others. This paper considers the problem of locating urban distribution centers and proposes an exact method, based on integer linear programming for strategic, tactical and operational decision-making. The aim is to solve, in an integer manner, location, sizing and operation (vehicle routing) problems in these logistics platforms. The model is validated using real-data taken from the city of Saint-Étienne, France. Computational experiments are also carried out in order to compare the proposed model with existing procedures from the literature. Results show the efficiency and effectiveness of the proposed model and its applicability in real decision-making for medium sized data sets.
Keywords: Urban distribution centers; freight consolidation; traffic; decision levels
Resumen
La creación de centros urbanos de distribución y consolidación de carga cerca del centro de las ciudades es una iniciativa implementada alrededor del mundo que busca mejorar la congestión del tráfico vehicular en las calles y la calidad de vida sus habitantes. Este artículo considera el problema de localización de centros urbanos de distribución y propone un modelo exacto de programación lineal entera para la toma de decisiones integrando los niveles estratégico, táctico y operativo. Se busca resolver los problemas de localización, dimensionamiento y operación (ruteo de camiones) en estas terminales logísticas. El modelo es validado usando datos reales provenientes de la ciudad de Saint-Étienne, Francia. Así mismo, se realizan comparaciones experimentales con procedimientos existentes en la literatura. Los resultados muestran la eficiencia y efectividad del modelo propuesto y su aplicabilidad para instancias hasta de tamaño mediano.
Palabras clave: centros urbanos de distribución; consolidación de cargas; tráfico vehicular; niveles de decisión
Introduction
City logistics or urban logistics is defined in the literature as the process of totally optimizing urban logistics activities by considering social, environmental, economic, financial, and energy impacts of urban freight movement (Taniguchi et al., 2001). According to the data presented by Koriath and Thetrich (1998), freight vehicles in urban areas with vehicles of less than 3.5 tonnes correspond to more than 10% of the total traffic flow in urban areas. The creation of urban distribution centers (UDC) within cities seeks to solve the problems of traffic congestion, negative environmental impacts and high energy consumption (Taniguchi et al., 1999). This is particularly targeted in cities with a structure inherited from the Middle Age that do not possess a suitable road structure for small but abundant stores presented in downtown areas (Ligocki and Zonn, 1984). According the advantages appointed by Taniguchi et al. (1999), the creation of UDCs allows a much more efficient urban logistics system, with the same capacity of service than conventional systems but with lower environmental impacts. Thus, several cities have decided to implement these UDC's that may offer different possibilities such as: the use of electric vehicles, the scheduling of multiple successive delivery or collection routes (Guyon et al., 2012) and the implementation of cooperative freight systems (Nemoto, 1997; Yang and Odani, 2006).
Optimally determining the location and operation of UDC's would have an impact not only economic but also the environment and on the society. The problem of UDC's location corresponds to a strategic decision-making level while the decision of defining its size corresponds to the tactical decision-making level and the operational distribution itself (i.e., delivery routes of vehicles assigned to UDC) corresponds to the operational decision-making level. In order to implement a design and operational methodology for UDC's, these three decision levels (strategic, tactical and operational) can be integrated as a location-routing problem (LRP). As discussed later in this paper, the LRP has been a problem largely studied in the Operations Research literature, mainly from theoretical
cases; practical applications in urban logistics have been few and limited solely to the work of Montoya-Torres et al. (2012). These authors proposed a hierarchical heuristic method in which the location, sizing and routing problems of an UDC are evaluated for the city of Saint-Etienne, France. As this is a heuristic method, there is no guarantee of obtaining the optimal solution for the problem.
This paper seeks to identify and develop a mathematical model based in integer linear programming, which integrally optimizes the location of the urban distribution center(s) in one city and the routes to visit its clients. It is noteworthy that the concept of UDC is relatively new and needs more intensive investigation in several areas such as their function, size, location, management, etc. Moreover, since the problem under study can be modeled as an LRP, the reader should note that authors like Nagy and Salhi (2007) conclude that the exact methods are often very successful for solving special cases of the LRP. Given these appreciations, the development of an exact method to determine the location, size and operation of urban distribution centers could serve as an important tool for decision making which, applied to real situations of cities willing to create these logistics terminals, can improve the competitiveness of its logistics system and its environmental and social conditions previously mentioned. The proposed model is validated and compared with the data and the results obtained from the application of the hierarchical model of Montoya-Torres et al. (2012), which considers the current situation of the city of Saint-Etienne, France.
The paper is organized as follows. Section 1 presents in detail the problem under study and the related literature. The mathematical model is described in Section 2, while section 3 is dedicated to the analysis of experiments. Finally, last section draws some conclusions and suggests opportunities for future research.
1. Problem Description and Related Literature
Urban Distribution Centers are public logistics terminals with complex installations and multiple functions, including transshipment yards, stores, wholesale markets, information centers, exhibition halls and meeting rooms, etc. These are designed to meet various needs of the urban logistics system by using advanced information systems, in order to help implement algorithms and heuristics to develop more efficient routing and scheduling systems for pickup/ delivery trucks in urban areas. This would be useful to reduce the number of trucks that are required to provide same or even higher level of service to customers compared with conventional systems. Figure 1 shows a schematic representation of an urban distribution center. UDC may also help SME (small and medium enterprises) to implement efficient freight transportation through the mechanization and automation of materials handling (Taniguchi et al., 1999). These terminals can also facilitate the implementation of cooperative freight transportation systems (Nemoto, 1997; Yang and Odani, 2006) by sharing the costs generated by products distribution (i.e. gasoline and driver wages) and reducing the costs of vehicles and facilities acquisition and maintenance needed for the operation. As concluded by Taniguchi et al. (1995), truck traffic can be reduced by adopting cooperative loading systems. This reduction of vehicles leads to a monetary savings for companies, decreases city traffic levels, reduces the environmental impact (fewer pollutants), and improves, as a result, residents' life quality.
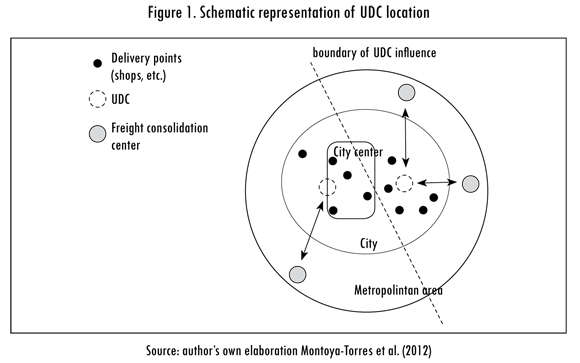
As mentioned above, the making-decision problem related to the location, size and operation of UDC can be represented as a facility location problem and vehicle routing problem, as known in the Operations Research literature. The problem is called Location-Routing Problem (LRP) and handles, integrally, the location of facilities within a set of potential points and the design of routes between customers assigned to them. This allows, as a result, to determine the capacity or size of the facility and, if necessary, of the vehicles. The LRP is conceptually more difficult than the classical location problem and also than the classical Vehicle Routing Problem (VRP). Both problems can be viewed as special cases of the LRP. If all customers are required to be directly served by a given depot, the LRP becomes a standard location problem. If, on the other hand, depot locations are fixed, the LRP reduces to a VRP (Nagy and Salhi, 2007). Thus, the LRP is an NP-hard problem, as it reduces to any of two NP-hard problems (facility location and vehicle routing).
In the academic literature, the review carried out by Nagy and Salhi (2007) suggests the following classification of studies regarding the LRP problem:
- Objective function. The usual objective for location-routing problems is the minimization of total cost, including depot location costs and vehicle operational costs. There are few papers where a different objective functions are considered, either single of multiple: Averbakh and Berman (1994, 1995, and 2002), Averbakh et al. (1994), and Jamil et al. (1994).
- Solution space. This can be discrete, network or continuous. Most of the LRP literature deals with discrete location.
- Number of depots. This may be single or multiple. Most papers on the LRP deal with multiple depots, except the works of Laporte and Nobert (1981), Averbakh and Berman (1994 and 2002), Simchi-Levi (1991), and Schwardt and Dethloff (2005), who restrict themselves to single depots. Special cases devoted to only one depot include the travelling salesman location problem and most round-trip location problems. Furthermore, when multiple depots are considered, it is generally assumed that the number of depots is not given in advance, the exceptions to this is the work of Branco and Coelho (1990).
- Number and types of vehicles. For most location-routing problems, the number of vehicles is not fixed in advance and a homogeneous fleet is assumed. However, a heterogeneous fleet is considered by Bookbinder and Reece (1988), Salhi and Fraser (1996), Wu et al. (2002), and Ambrosino and Scutella (2005). Laporte and Nobert (1981) and Averbakh and Berman (2002) investigated problems when the number of vehicles is given in advance. Furthermore, Laporte et al. (1983), and Branco and Coelho (1990) looked at special cases of exactly one vehicle per depot. Traveling salesman location problems also have this structure.
- Route structure. The usual structure of vehicle routes in an LRP is to start out from a depot, traverse through a number of customer nodes, delivery goods at each customer and finally return to the same depot. For most location-routing problems, this structure holds, but some exceptions are present in the literature: vehicles may be allowed to carry out multiple trips (Lin et al., 2002), and vehicle routes may contain both deliveries and pickups (Mosheiov, 1995).
The literature about the resolution of the LRP mainly focuses on deterministic context. There are few studies on the stochastic context (Herazo-Padilla et al., 2013). Readers interested on reviews regarding published literature on LRP may consult the works of Balakrishnan et al. (1987), Laporte (1988 and 1989), Berman et al. (1995), Min et al. (1998), Nagy and Salhi (2007), and Herazo-Padilla et al. (2013).
Due to space restrictions in this journal, the synthesis of the review carried out is presented. Most of the papers published on the LRP discussed theoretical problems. In terms of real applications, the literature has primarily considered facility location problems for the design of large logistics networks, in which interurban transport activities are performed. The academic literature has emphasized the conceptual and practical differences between optimization models for long-distance logistics and for city logistics (e.g., Barceló et al., 2007). The two main characteristics are its contribution to traffic flows and the subsequent environmental impact (Taniguchi et al., 2001; Barceló et al., 2007). For the particular case of urban distribution centers, the only work available in the literature is developed by Montoya-Torres et al. (2012), which solves the problem of raw materials and goods distribution to shops and stores located in the historic center of a European city. Such paper proposes the application of a hierarchical heuristic procedure, solving each of the relevant issues in each step of the process: distribution center location, its sizing and finally routes for customer delivery (shops, stores, etc.). For UDC location, these authors used a classical model of location based in mixed integer programming (MIP); while for the vehicle routing they used the "nearest neighbor" heuristic with load balancing. Although this is the first approach in the existing literature, there are more opportunities to solve the problem either integrally and/or using more efficient resolution methods (e.g. meta-heuristics algorithms). This paper seeks to contribute to understanding and solving the problem of UDC location and operation through a formal integral problem using mathematical programming. The following sections present the used models and its validation in detail using real data.
2. Formulation of Mixed Integer Linear Programming Model
The mathematical model employed to solve the problem of optimal location and operation of urban distribution centers is presented next in detail. This model assumes the following parameters as input:
- Number of customers and urban centers as well as its location and the respective distances or costs between each.
- Number of distribution centers to use / open.
- Number of vehicles used and their respective capacities.
- Customers' demands.
With these parameters the model restrictions are set. The model seeks to minimize the cost of supplying the demand of each customer, determining the location, size and operation of UDC.
2.1. Main Sets
I = Urban Distribution Centers UDC {1, 2, ..., i}
J = Costumbers {1, 2, j}
K = Vehicles {1, 2, ., k}
2.2. Auxiliary Sets
H, F = alias of J
L = alias of K
2.3. Decision Variables
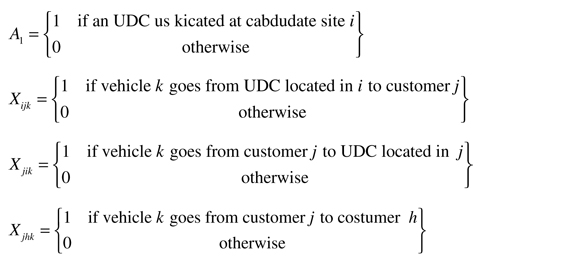
2.4. Model Parameters
C1ij = cost matrix of traveling from UDC located in i to costumer j
C2ji= cost matrix of traveling from costumer j to UDC located in i
C3jh = cost matrix of traveling from costumer j to to costumer h
C = number of UDC to open
V = number of available vehicles
Dj = demand of costumber j
CAPk= capacity of vehicle k
N = number of clients
2.5. Objective Function
Equation (1) expresses the objective function. It seeks to minimize the total distance or cost of supplying the demand of all customers, according to the location of urban distribution centers.

2.6. Constraints
Model constraints, identified during the research process, are presented next. The set of Constraints (2) specifies the maximum number of UDC to open or locate. It should be noted that for the specific case of the city of Saint-Etienne only one location is to be defined (e.g. C= 1). The set of Constraints (3) ensures that vehicle routes can be defined only if from an UDC which is open. The set of Constraints (4) computes the minimum number of routes / vehicles required to meet the total demand. If desired, the number of vehicles to be used can be defined as a fixed value; this is done by changing the right side of the inequality by the corresponding desired value. The set of Constraints (5) concerns two binary variables and ensures that if a client is visited from a UDC, the vehicle must go to another customer, starting from the same visited customer and in the same vehicle. This constraint initializes the route and relates the first customer visited with the sequence of customers that should visit. The set of Constraints (6) establishes the sequencing of the entire route for all clients, establishing that if a client is visited, it should go to another client or to return to the UDC in the same vehicle performing the route. The set of Constraints (7) ensures that all customers are visited exactly once, from a client or from a UDC. The set of Constraints (8) ensures that after a vehicle visits a customer it goes to another client or return back to a UDC. The set of Constraints (9) forces all vehicles routes to begin and end in the same UDC. Vehicle capacity constraints are defined by Constraints (10), through the delimitation of the route length. The set of Constraints (11) limits at most one route per vehicle. The sets of Constraint (12) and (13) ensure that the size of each route is similar; that is a load balancing constraint. These restrictions are not binding and can be ignored by several factors: heterogeneity of vehicle capacity. In addition, if desired, the model can calculate the optimal size of each route. Finally, the set of Constraint (14) avoids subtours within the solution.

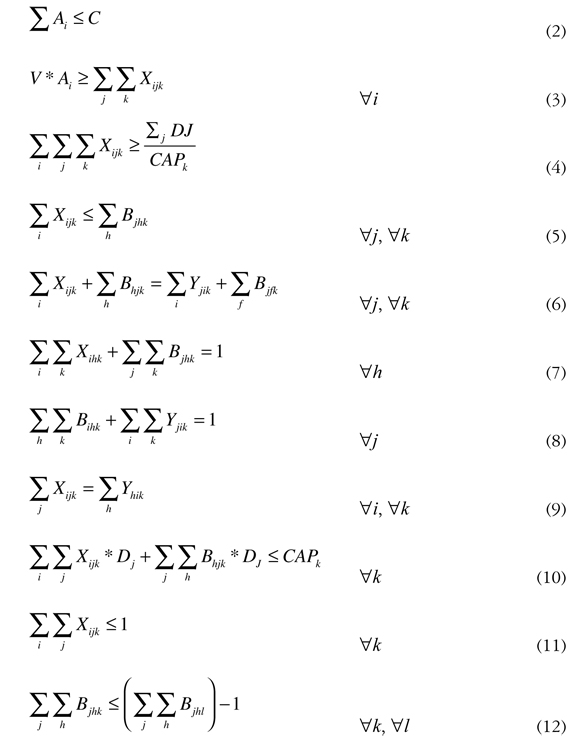
3. Solution Procedure
The solution procedure consisted of the following steps. First, the mathematical model was programmed in the GAMS (General Algebraic Modeling System) language. As a method of solving, the CPLEX solver was used. The objective function was defined as the minimization of the total distance to meet demand of all customers. Subsequently, with the actual coordinates of the stores in the city of Saint-Etienne, France, the matrix of Euclidean distances was computed. In order to facilitate replication of the experiments, data used in this study are available on the website http://jrmontoya.wordpress.com/research/instances/data-lrp-saint-etienne/. The other model parameters (number and location of urban distribution centers, number and location of customers to visit, etc.) were determined according to the instance tested in each experiment and are discussed below. Finally, the execution time of each and every instances was 15 minutes on a computer with Intel® Core i5 4GB RAM processor.
4. Experiments and Analysis of Results
Model validation was carried out in two phases. In the first phase a comparison between the results of supply the demand of all customers applying the proposed model and the obtained by applying the model of Montoya-Torres et al. (2012) was carried out. It should be remained that the proposed model integrates the location and vehicle routing to determine the exact (optimal) location, size and operation of the UDC's, while the hierarchical model of Montoya-Torres et al. (2012) subsequently states location (strategic decision), then the size (tactical decision) and finally the routing of vehicles (operational decision). For a better comparison, the hierarchical model was also programmed in the GAMS language with the same conditions and parameters (e.g. execution time) of the proposed model. In the second phase of the experiment, modifications to the model parameters are made (e.g. increase the number of potential locations of the UDC's and/ or increase the number of customers) to measure the quality of the solutions. In both phases, real data of distances, number and location of stores and shops in the city of Saint-Etienne, France, were used. There is a total of 1227 points (shops or stores) outside the central area of the city.
4.1. Results of the First Phase: Comparison with the Hierarchical Approach of Montoya-Torres et al. (2012)
The hierarchical model of Montoya-Torres et al. (2012) proposes the location of one UDC in the city center from 20 potential locations. According to their work, both the 20 potential locations and the 20 clients are the same and were chosen randomly from the total 1227 points belonging to the central area of the city. It is important to remark that the hierarchical model of Montoya-Torres et al. (2012) used the nearest neighbor algorithm, with one, two and three routes, to obtain the routing solution (operational level). Therefore, the hierarchical model was programmed in GAMS, as a model of mixed integer linear programming (MILP), to determine the optimal solution of this approach.
The results of the comparison between the proposed model and the hierarchical approach are shown in Figure 2. Initially a comparison between the hierarchical model Montoya-Torres et al. (2012) is performed when the vehicle routing is done using the nearest neighbor heuristic and then when routing is done with an exact method (i.e., mixed integer programming model in GAMS). With the exact method, which obtains the optimal solution just for the routing part of the procedure, it is seen an improvement up to 10.25% in the total cost. From these results, an initial hypothesis can be stated that consists on applying the proposed model to solve integrally the location and routing problems can generate even better results than simply optimizing the final phase of the problem (routing). Figure 2 also shows the routes obtained using the model proposed in this paper, based on the optimal solution for the location-routing problem (LRP). The results show that the proposed model obtains better solutions, for two and three routes, than the hierarchical approach and located the UDC in a central position with respect to other customers. The integral model solution is 22.12% better than the solution of the hierarchical approach for two routes; and 30.57% for three routes, as shown in Table 1.
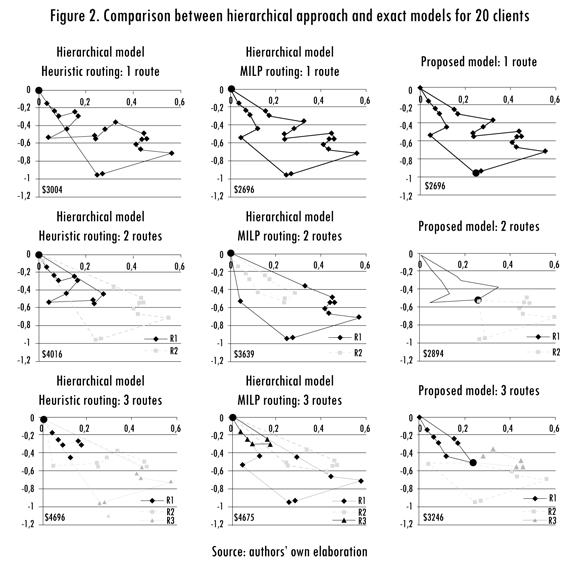
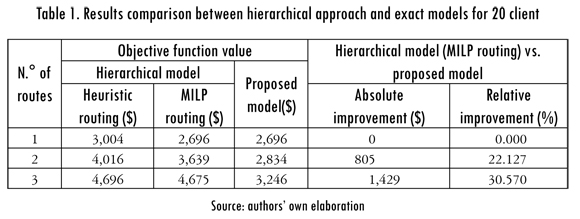
To conclude this first experiment with a relatively small number of clients (20 clients and 20 potential locations for the UDC), it is important to highlight that the performance of the proposed model is excellent concerning the value of the objective function, achieving the results in a short computational time: about 15 minutes. It can also be highlighted that solving the problem in an integral manner is better than solving it sequentially (hierarchically). In addition, it is found, in part, the flexibility of the proposed model as it allows solving the vehicle routing problem (VRP) for the mentioned instances.
4.2. Results for the Second Phase: Proposed Model's Performance
The second phase of the computational experiment on the proposed model consisted of carry out various performance tests with multiple data sets from the city of Saint-Etienne, France. Specifically, two instances were analyzed: 5 potential locations for the UDC and 50 customers and 5 potential locations for the UDC and 100 customers. These experiments sought to test the capacity of the model to provide feasible and real solutions, increasing the number of customers and reducing the potential locations for the UDC. In fact, given the characteristics of the real problem, it is likely that decision-makers (public authorities) have a small and preselected number of potential locations of the UDC's (e.g. old abandoned buildings) and a larger set of costumers. In both cases, potential points of UDC locations are different from the points where customers are located. For these two scenarios, the selection of 50 or 100 customers (out of 1227 points in the city) sought to cover 100% of the central area perimeter and all major routes. This ensures that the model solution to be closer to reality.
In the instance of 5 potential locations for the UDC and 50 clients (see Table 2), the results for one and two routes show the preponderance of making the journey with a single route; the routing has an over-cost when two routes are used equivalent to 0.99% compared to the cost of using a single route. In the three routes case, the model obtains a sub-optimal solution, although it is "feasible"; the inefficiency of the model to solve this size of problems is evidenced. For this instance, the difference between the model solution and the best possible solution (lower bound) corresponds to 20.24%. Based on these results, Figure 3 shows the distribution of the 50 preselected clients and the optimal solution for one route. Likewise, the distribution of all shops and commercial areas of the city is observed, showing that the obtained solution cover around 80% of the 1227 total points.
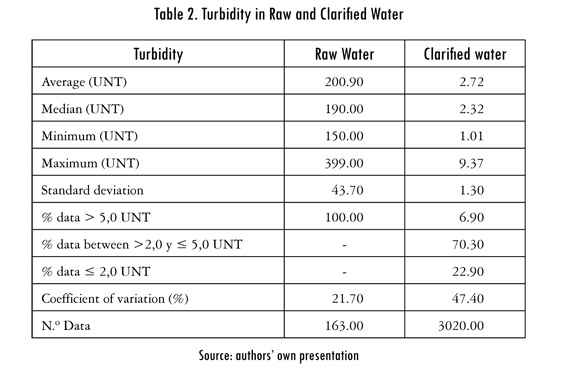
Figure 3 also shows the resulting route for the instance with 5 potential points to locate the UDC and 100 costumers. With this number of clients the result given by the model is no longer optimal. In this instance, the difference between the model solution and the best possible solution (lower bound) corresponds to 7.05% (i.e., the solution of the model is 6318.00, while the lower bound has a value of 5872.55). Although it is a "feasible" solution, it is graphically seen that it is not the best one.
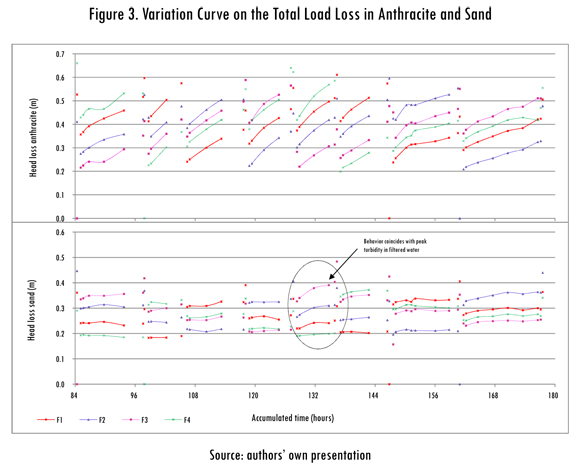
Conclusions and Perspectives
The mixed integer linear programming model discussed in this research is presented as an exact method to integrally define location, size and operation of UDC, minimizing the total cost/distance of the routes and respecting the limitations and constraints of the problem. The model has the ability to solve or the LRP or the VRP, with the following characteristics:
- Single and multiple depot problems can be solved, according to the needs of the problem. The number of depots can be determined as an initial data or the model can determine the optimal number according to the relevant constraints. For the specific case of the city of Saint-Etienne a single depot is defined.
- The number of vehicles can be given as initial parameter, or the model can calculate the optimal number of routes.
- The number of routes per vehicle and/or depot may be constrained.
- Vehicles capacity can be homogeneous or heterogeneous.
Comparisons between the approach of Montoya-Torres et al. (2012) and the proposed model show that the integral solution is better than the hierarchical approach in all cases, with improvements up to 30.57%. Likewise, performance tests of the model with data from the city of Saint-Etienne, France, show that it is better to make one trip for all customers than have multiple routes with multiple vehicles assigned to the UDC.
As future work, one line for research could be using of meta-heuristics with good performance for solve the location-routing in the particular case of the distribution of products to a large number of shops and commercial stores in the city of Saint-Étienne. Preliminary works (Muñoz-Villamizar et al., 2013) show that hybrid iterative local search and Monte Carlo simulation procedures allow obtaining good solutions. In the other hand, due that large cities need to restrict the movement of trucks in the center or in certain residential areas, formal tools for decisions-making with integrated geographical information systems can be designed. In this way, decision-makers (usually public administration entities) can use these tools to "simulate" the economic (but also social or environmental) impacts that depend on the urban distribution centers location.
References
AMBROSINO, D. and SCUTELLA, M.G. Distribution network design: New problems and related models. EuropeanJournal of Operational Research. 2005, vol. 165, no. 3, pp. 610-624. [ Links ]
AVERBAKH, I. and BERMAN, O. Probabilistic sales-delivery man and sales-delivery facility location problems on a tree. Transportation Science. 1995, vol. 29, no. 2, pp. 184-197. [ Links ]
AVERBAKH, I. and BERMAN, O. Routing and location-routing p-delivery men problems on a path. Transportation Science. 1994, vol. 28, no. 2, pp. 184-197. [ Links ]
AVERBAKH, I.; BERMAN, O. Minmax p-traveling salesmen location problems on a tree. Annals of Operations Research. 2002, vol. 110, no. 1-4, pp. 55-62. [ Links ]
AVERBAKH, I.; BERMAN, O. and SIMCHI-LEVI, D. Probabilistic a priori routing-location problems. Naval Research Logistics. 1994, vol. 41, no. 7, pp. 973-989. [ Links ]
BALAKRISHNAN, A.; WARD, J.E. and WONG, R.T. Integrated facility location and vehicle routing models: recent work and future prospects. American Journal of Mathematical and Management Sciences. 1987, vol. 7, no. 1-2, pp. 35-61. [ Links ]
BARCELÓ, J.; GRZYBOWSKA, H. and PARDO, S. Vehicle routing and scheduling models, simulation and city logistics. Dynamic Fleet Management. 2007, vol. 38, pp. 163-195. [ Links ]
BERMAN, O.; JAILLET, P and SIMCHI-LEVI, D. Location-routing problems with uncertainty. In: Drezner, Z. (Ed.). Facility location: a survey of applications and methods. New York, New York: Springer, 1995, pp. 427-452. [ Links ]
BOOKBINDER, J.H. and REECE, K.E. Vehicle routing considerations in distribution system design. European Journal of Operational Research. 1988, vol. 37, no. 2, pp. 204-213. [ Links ]
BRANCO, I.M. and COELHO, J.D. The Hamiltonian p-median problem. European Journal of Operational Research. 1990, vol. 47, no. 1, pp. 86-95. [ Links ]
GUYON, O.; ABSI, N.; FEILLET, D. and GARAIX, T. A modeling approach for locating logistics platforms for fast parcel delivery in urban areas. Procedia - Social and Behavioral Sciences. 2012, vol. 39, pp. 360-368. [ Links ]
HERAZO-PADILLA, N.; NIETO ISAZA, S.; MONTOYA-TORRES, J.R.; RAMIREZ POLO, L.; MUÑOZ-VILLAMIZAR, A. Coupling ant colony optimisation and discrete-event simulation to solve a stochastic location-routing problem. Proceedings of the 2013 Winter Simulation Conference, 2013, pp. 3352-3362. [ Links ]
JAMIL, M.; BATTA, R. and MALON, D.M. The traveling repairperson home base location problem. Transportation Science. 1994, vol. 28, no. 2, pp. 150-161. [ Links ]
KORIATH, H. and THETRICH, W Urban goods transport: COST 321 - Final Report of the action. Bruxelles: Office of the Official Publications of the European Community, 1998. [ Links ]
LAPORTE, G. A survey of algorithms for location-routing problems. Investigación Operativa. 1989, vol. 1, pp. 93-123. [ Links ]
LAPORTE, G. Location-routing problems. In: Vehicle Routing: Methods and Studies. Amsterdam: North-Holland, 1988, pp. 163-198. [ Links ]
LAPORTE, G. and NOBERT, Y. An exact algorithm for minimizing routing and operating costs in depot location. European Journal of Operational Research. 1981, vol. 6, no. 2, pp. 224-22. [ Links ]
LAPORTE, G.; NOBERT, Y. and PELLETIER, P. Hamiltonian location problems. European Journal of Operational Research. 1983, vol. 12, no. 1, pp. 82-89. [ Links ]
LIGOCKI, C. and ZONN, L.E. Parking problems in central business districts. Cities. 1984, vol. 1, no. 4, pp. 350-355. [ Links ]
LIN, C.K.Y; CHOW, C.K. and CHEN, A. A location-routing loading problem for bill delivery services. Computers & Industrial Engineering. 2002, vol. 43, no. 1-2, pp. 5-25. [ Links ]
MIN, H.; JAYARAMAN, V. and SRIVASTAVA, R. Combined location-routing problems: a synthesis and future research directions. European Journal of Operational Research. 1998, vol. 108, no. 1, pp. 1-15. [ Links ]
MONTOYA-TORRES, J.R.; MARQUES, G. and BURLAT, P. A conceptual framework for location, sizing and operation of urban distribution centers with sustainable performance metrics. Proceedings of the 4th International Conference on Information Systems, Logistics and Supply Chain (ILS 2012), Quebec, Canada, August 26-29, 2012. CD-ROM. [ Links ]
MOSHEIOV, G. The pickup delivery location problem on networks. Networks, 1995, vol. 26, no. 4, pp. 243-251. [ Links ]
MUÑOZ-VILLAMIZAR, A.; MONTOYA-TORRES, J.R.; JUAN, A.A.; CÁCERES-CRUZ, J. A simulation-based algorithm for the integrated location and routing problem in urban logistics. Proceedings of the 2013 Winter Simulation Conference, 2013, pp. 2032-2041. [ Links ]
NAGY, G. and SALHI, S. Location-routing: Issues, models and methods. European Journal of Operational Research. 2007, vol. 177, no. 2, pp. 649-672. [ Links ]
NEMOTO, T. Area-wide inter-carrier consolidation of freight in urban areas. Transport Logistics. 1997, vol. 1, no. 2, pp. 87-103. [ Links ]
SALHI, S. and FRASER, M. An integrated heuristic approach for the combined location vehicle fleet mix problem. Studies in LocationalAnalysis. 1996, no. 8, pp. 3-21. [ Links ]
SCHWARDT, M. and DETHLOFF, J. Solving a continuous location routing problem by use of a self-organizing map. InternationalJournal of Physical Distribution and Logistics Management. 2005, vol. 35, no. 6, pp. 390-408. [ Links ]
SIMCHI-LEVI, D. The capacitated traveling salesman location problem. Transportation Science. 1991, vol. 25, no. 1, pp. 9-18. [ Links ]
TANIGUCHI, E.; NORITAKE, M.; YAMADA, T. and IZUMITANI, T. Optimal size and location planning of public logistics terminals. Transportation Research Part E. 1999, vol. 35, no. 3, pp. 207-222. [ Links ]
TANIGUCHI, E.; THOMSON, R.G.; YAMADA, T. and VAN DUIN, R. City logistics: networks modelling and intelligent transport systems. n.e.: Pergamon, 1999. [ Links ]
TANIGUCHI, E.; THOMPSON, R.G. and YAMADA, T. Recent advances in modelling city logistics. In: City Logistics II, n. d., 2001, pp. 3-34. [ Links ]
TANIGUCHI, E. ; YAMADA, T. and YANAGISAWA, T. Issues and views on cooperative freight trans-portation systems. 7th World Conference on Transport Research, 1995. [ Links ]
WU, T.-H.; LOW, C. and BAI, J.-W. Heuristic solutions to multi-depot location-routing problems. Computers and Operations Research. 2002, vol. 29, no. 10, pp. 1393-1415. [ Links ]
YANG, D. and ODANI, M. Analysis on movement of profit for the partner companies in joint-delivery system. Proceedings of Infrastructure Planning. 2006, vol. 33, p. 327. [ Links ]