Serviços Personalizados
Journal
Artigo
Indicadores
-
Citado por SciELO
-
Acessos
Links relacionados
-
Citado por Google
-
Similares em SciELO
-
Similares em Google
Compartilhar
Ingeniería y Universidad
versão impressa ISSN 0123-2126
Ing. Univ. vol.19 no.1 Bogotá jan./jun. 2015
https://doi.org/10.11144/Javeriana.iyu19-1.ahhc
A Home Health Care Districting Problem in a Rapid-Growing City1
Un problema de zonificación en servicios de hospitalización domiciliaria en una ciudad de rápido crecimiento2
Elena Valentina Gutiérrez-Gutiérrez3
Carlos Julio Vidal4
1Submitted on: February 3rd, 2014. Accepted: November 24th, 2014. This article is derived from the doctoral research Project titled Home Health Care Logistics Management Problems, Developed by the Research Group on Logistics and Production from Universidad del Valle, Cali, Colombia.
2Fecha de recepción: Febrero 3, 2014. Fecha de aceptación: Noviembre 24, 2014. Este artículo se deriva del proyecto de investigación denominado Home Health Care Logistics Management Problems, desarrollado por el Grupo de Investigación en Logística y Producción de la Universidad del Valle, Cali, Colombia. Desarrollado por el grupo de investigación en Logística y Producción de la Universidad del Valle, Cali, Colombia.
3Ingeniera Industrial, Especialista en Logística, Maestría en Ingeniería-Énfasis Industrial, Doctora en Ingeniería-Énfasis, Ingeniería Industrial, Universidad del Valle. Profesora Vinculada, Universidad de Antioquia, Medellín, Colombia. Correo electrónico: elena.gutierrez@udea.edu.co.
4Ingeniero Mecánico, Magíster en Ingeniería Industrial y de Sistemas, Universidad del Valle. M.Sc. in Industrial Engineering, Ph.D. in Industrial Engineering, Georgia Institute of Technology. Profesor Titular, Universidad del Valle, Cali, Colombia. Correo electrónico: carlos.vidal@correounivalle.edu.co.
Para citar este artículo / To cite this article
E. V. Gutiérrez and C. J. Vidal, "A Home Health Care Districting Problem in a Rapid-Growing City", Ing. Univ., vol. 19, no. 1, pp. 87-113, Ene.-Jun., 2015. http://dx.doi.org/10.11144/Javeriana.iyu19-1.ahhc
Abstract
The districting problem in home health care (HHC) is part of the logistics decisions that healthcare providers face when designing service networks to deliver coordinated medical care to patients' homes. In this paper we study such problem in the context of a rapid-growing city, phenomenon that refers to the increment of the population in urban areas, and which results in problems such as the proliferation of marginal neighborhoods, increment of epidemic diseases, absence of governmental control and security, and lack of basic health services. Consequently, three factors derived from this phenomenon are integrally studied: geographical disposition of the population, security conditions to access basic units, and trends on demand for HHC services. We propose a bi-objective mathematical model and identify trade-offs, allowing finding better compromised solutions. We evaluate the model with real data instances from a HHC institution which delivers services in the largest cities in Colombia. Results show that better districting configurations can be obtained and deteriorations of less than 10% in Travel Workload can produce improvements of more than 80% in Workload Deviations.
Keywords: home health care; logistics management decisions; districting problems
Resumen
El problema de zonificación en servicios de hospitalización domiciliaria (HHC, por sus iniciales en inglés) hace parte de las decisiones logísticas que los proveedores de salud deben enfrentar en el diseño de redes para llevar cuidado médico coordinado a los hogares de los pacientes. En este trabajo se estudia dicho problema en el contexto de una ciudad con rápido crecimiento, fenómeno que se refiere al incremento de la población en áreas urbanas y que da lugar a problemas como la proliferación de barrios marginales, el incremento de enfermedades y epidemias, la ausencia de control y seguridad por parte del gobierno y la carencia de servicios básicos de salud. Consecuentemente, tres factores derivados de este fenómeno se consideran de manera integral: la disposición geográfica de la población, las condiciones de seguridad para acceder a las unidades básicas y las tendencias de la demanda de servicios de HHC. Se propone un modelo matemático biobjetivo y se identifican las compensaciones entre los objetivos, lo cual permite encontrar soluciones mejor balanceadas. El modelo es evaluado con instancias de datos reales de una institución de HHC que ofrece los servicios en las ciudades más grandes de Colombia. Los resultados muestran que es posible obtener mejores configuraciones de zonificación y que deterioros de menos del 10 % en la carga de trabajo de viaje producen mejoramientos de más del 80 % en las desviaciones de cargas de trabajo.
Palabras clave: hospitalización domiciliaria; decisiones de gestión logística; problemas de zonificación
1. Introduction
Colombia is the second most populated country in Latin America with nearly 46 million inhabitants [1]. As other countries in the region, Colombia faces a severe health crisis with a very restrictive budget for health services and a growing population. In this context, Home Health Care (HHC) services have appeared as an alternative to improve the performance of health care providers and the utilization of scarce resources, providing a lower general cost for the health system. In Colombia, the health care system is regulated by the government through the Ministry of Health (MSPS: Ministerio de Salud y de Protección Social) which provides health services to the under-privileged non-working population through a subsidized system. However, to date, an important fraction of the service is delegated to the private sector, under an employer-based social insurance scheme, creating a combined Social Security System (SSS). As of December 2013, 482 institutions were certified by the MSPS to provide HHC services in Colombia [2].
When providing HHC services, the partitioning of an urban area into suitable districts is a major decision known as districting [3]. The districting problem (DP) consists of defining districts made up of several territorial basic units (e.g. zip codes, neighborhoods or communes) in order to ensure that the service is delivered to the patients' location at the prescribed times, and to assign balanced workloads to the medical staff. The World Health Organization (WHO) identified that to strength district health care, it is essential to improve tactical and operational management [4]. According to the author, six key issues pertain to this type of management, which includes: (i) personnel and training, (ii) organizational structure, (iii) the health care team, (iv) delegation, supervision and incentives, (v) supplies, logistics and maintenance, and (vi) financial management. These issues must be integrally coordinated to ensure that HHC goals are achieved.
Given a districting configuration, medical staff will be then assigned to take care of patients in each district, and therefore, the size of an urban district will partly define the time that medical staff will spend traveling among patients' houses, and actually taking care of patients when performing medical activities. From the total workload assigned to a medical staff in HHC, these two activities (traveling and taking care of patients), are usually known as travel workload and visit workload, respectively. The authors in [5] defined these activities as Road time and Face-To-Face time, and identified that an important aim when assigning medical staff is to maximize the Face-To-Face time. Equivalently, it is expected that the districting configuration contributes in the maximization of the time that medical staff spends taking care of patients (visit workload), minimizing likewise the time they have to spend traveling among patients (travel workload). The total workload is defined by the sum of the visit and the travel workload.
The DP has been studied in political contexts, school and police districting, and sales territories [6]-[16]. These decisions have been also studied in the context of health care [17]-[22], and few works study the problem in HHC. To the best of our knowledge, three authors have studied this problem in the HHC context. In Blais et al. [23] the authors solved a practical districting problem in the management of public HHC services for a local community in Montreal, Canada. In Bennett [24] a HHC nurse districting problem is studied through a set partitioning model, which was solved by a column generation heuristic that integrated ideas from optimization and local search. In Benzarti [25] the problem was studied in the French context. More recently, [26] described the HHC nurse districting problem as a tactical decision, and identified formulations and solution methods. The author presented a survey of the relevant literature of the problem and found that the works of [23], [24], [27] are the three studies published that present computational results for the districting problem in HHC. A detailed analysis of these works is presented in [28].
Currently in Colombia all decisions related to districting in HHC are made empirically, and there is not a formal methodology to estimate demand among basic units, neither to define districts nor assign resources to them [2]. As a result, HHC providers face unbalanced workloads among medical and administrative staff among districts, and long travel distances of medical staff. The districting configuration has a direct impact on medical staff capacity and productivity and on the quality of the service provided. Furthermore, we have not found an application of the problem in HHC that considers the socio-economic conditions of developing countries. Thereby, in this paper we study the districting problem in HHC (DPHHC) in the context of a rapid-growing city, phenomenon that refers to the increment of the population in urban areas, and which results in problems such as the proliferation of marginal neighborhoods, increment of epidemic diseases, absence of governmental control and security, and lack of basic health services. We also describe how socio-economic conditions of a developing country can be integrally included in the analysis.
The paper is organized as follows. In Section 2 we briefly present previous research on the problem and identify how different mathematical formulations can be used to support DPHHC decisions. Then we propose a bi-objective mathematical model, which includes arising issues in rapid-growing cities, and explain our solution strategy. In section 3 we present a case of study of a rapid-growing city, and explain real data collection and parameter estimation procedures. We then provide an analysis and discussion of the results obtained. Finally, in Section 4 we present our conclusions and future research.
2. Problem Definition and Modeling Approach
2.1. The districting problem in HHC: Prior Research and Literature Review A recent review of the literature showed that two formulations are appropriate for the DPHHC: location-allocation (LA) and set partitioning (SP) [26]. Moreover, a variation from the first formulation, known as facility-location (FL), has proved to be appropriate for conditions of the DPHHC, in which a previous definition of district centers is not required. The first formulation requires a fixed set of district centers, and decisions include the assignment of each basic unit to exactly one district center. The facility-location (FL) formulation does not require a predefined set of district centers. Instead, this formulation allows m district centers to be selected from n basic units. In the set partitioning (SP) formulation, a subset of the potential feasible districts is selected such that each basic unit is assigned to exactly one district center.
Table 1 presents a summary of the three formulations found in the literature for the DPHHC. For each formulation we identify its authors, the notation, the mathematical formulation, and an explanation of each equation. This summary identifies common mathematical structures that can be implemented, and provides elements to justify the selection of a particular formulation to include arising issues of the problem in a given context.
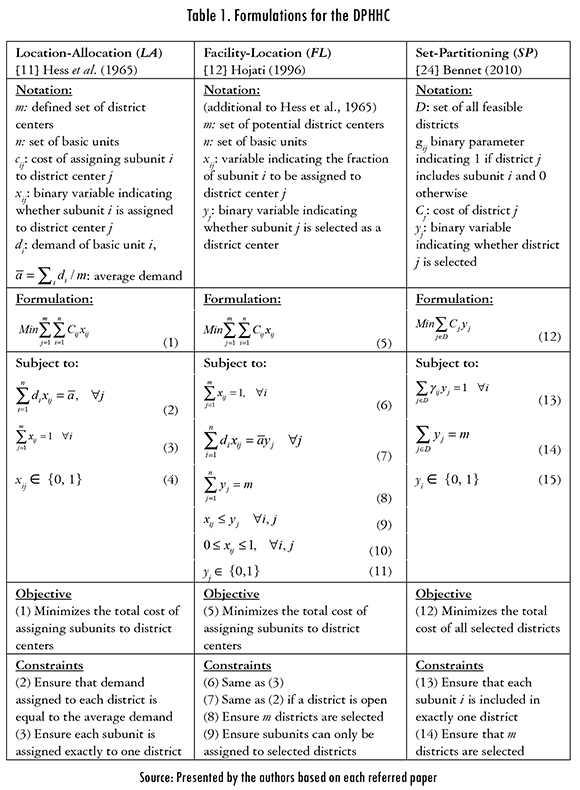
The LA formulation is attributed to [11], who presented a political districting model easy to understand and implement. The FL formulation was presented by [12] in a political context. This model offers the same advantages of the LA and does not require a predefined set of district centers. As it is unlikely that exact balance of attributes can be achieved, typical LA and FL models replace the equality constraints (2) and (7) in Table 1, with a set of lower and upper bound inequality constraints.
The SP formulation provides the flexibility to construct feasible districts within an auxiliary problem (AP) and to evaluate their cost in a master problem (MP) scheme that efficiently connects both problems. Exact and approximate solution approaches have been used for solving this formulation in the context of political districting problems. A main drawback of this formulation is that, given the structure of the MP and AP, the inclusion of variants of the original DPHHC has to be done in the AP, generating a greater computational complexity to solve this problem and possible infeasibilities when connecting both problems. Moreover, the increase in the number of basic units generates an exponential increase in the number of feasible districts, and therefore generates a larger computational complexity to solve both the AP and the MP.
2.2. Modeling the DPHHC in a rapid-growing city
Common criteria considered in the DPHHC include contiguity, compactness, balance or equity, continuity of care, respect of natural boundaries and socio-economic homogeneity. In [25] a definition and a detailed classification of DPHHC criteria is provided into three categories: geographical aspects, activity measure, and comparison among different districting configurations. Besides these criteria, we consider key features arising in rapid-growing cities and developing countries that are closely related with social and economic phenomena occurring in such contexts.
The rapid-growing city feature refers to the increment of the population in urban areas, mainly due to the decrement of the population in rural areas [29]. This phenomenon has intensified in the last decades in Latin America, where 79% of the population lives in cities. This trend makes this region the one with the biggest proportion of population in urban areas among the developing countries in the world [30]. This condition results in common problems of urban areas: proliferation of marginal neighborhoods, increment of epidemic diseases, absence of governmental control and security, and lack of basic services such as health services [31].
The implication of the rapid-growing city feature on the DPHHC is due to three factors: geographical disposition of the population, security conditions to access certain neighborhoods, and trends of demand for HHC services. In general, new population arriving from rural areas locates in the periphery of the city, incrementing the areas needing to be covered. Usually, the access to these neighborhoods is limited due to geographical reasons or to the lack of street networks. Furthermore, because of the social and economic difficulties that these populations face, the security of medical staff is in danger, so that many HHC providers have limited their coverage policy to certain neighborhoods and day-time hours. Likewise, the quantity and types of demand for HHC has increased, due to the growth of the population, its aging, and its social and sanitation conditions.
Consequently, we include the described features into four arising issues. First, due to the increase in the diversity of demand for HHC services, we consider the set A, which identifies the different type of medical activities. This implies that demand for HHC services must be indexed by the type of activity a ∈ A. Second, given the complexity to measure service times for each type of patient and pathology, we include the set P to identify each type of patient. Therefore, service times must be indexed by the type of activity a ∈ A and the type of patient p ∈ P, as stap. Third, the diversification of patient types, pathologies, and medical activities generates the need to differentiate medical professional skills. Hence, we include the set K that defines the type of medical staff, and the subset Sk that identifies the set of activities a ∈ A that can be performed by the each type of staff member k ∈ K. Fourth, due to security risks that medical staff face, we include a variation of security levels in each basic unit i, using the parameter ri ∈ [0,1]. This parameter works as follows: for any basic unit i, the parameter ri will take the value of 1, if the security level in such unit is guaranteed and demand requirements can be fully satisfied. Conversely, if the security level is not guaranteed in some degree, the parameter ri will take a value less than 1, and therefore, demand requirements will be partly satisfied in such basic unit.
To include the four arising issues in the DPHHC, we adapted common formulation structures from the LA model and from ideas presented by [17] and [23]. The LA formulation offers a flexible structure that allows including variants of the original problem. Moreover, ideas from the selected authors allow improving the quantification of workloads and the estimation of travelling distance among districts. As previously indicated, the total workload W assigned to a district includes the travel workload and the visit workload, which correspond respectively to the time dedicated to travel among patients' homes and the time dedicated to take care of patients performing medical activities. The travel workload is defined by the total time that the entire workforce will travel among all districts in the planning horizon. The visit workload is defined as the total time that the entire workforce will dedicate to take care of all type of patients, performing all type of medical activities, in the planning horizon. Because the DPHHC decisions are made for a strategic-tactic horizon, workload estimations are integrally completed for all type of patients and all medical activities for such period.
Advisedly, we did not include constraints to force contiguity among basic units of a given district. Given the strategic-tactical scope of the decision and the geographical distribution of demand for different types of HHC services, each type of staff member may be required to travel among non-contiguous basic units to cover all types of services, while obtaining a good utilization of working time and reducing travel workloads. The work of Galvis and Mock-Kow [32] proposes first and second order contiguities in a DPHHC in similar contexts; their results show that larger travel workloads are generated when including such constraints.
Besides the sets and parameters explained above, the model formulation includes a set of basic units I that must be assigned to one and only one district m from the set of districts, M. The demand for HHC services, that is the number of annual visits required in each basic unit i ∈ I, for each medical activity a, for each type of patientis denoted as diap. The service time for each medical activity a and each type of patient p is denoted as stap. The time to travel between basic units i, j ∈I is defined as tij
The model identifies the inclusion of each pair of basic units into each district. Consequently, the binary decision variable xijm takes the value of 1 if both basic units i and j are assigned to the same district m; 0, otherwise. This formulation allows quantifying the total distance travelled by the medical staff in each district m. Similarly, the binary variable yim controls the assignment of each individual basic unit i to a district m. Furthermore, decision variables and δm and δ+m quantify the lower and upper deviations of the workload of each district m, from the average workload W. Auxiliary variables Vm, Tm, and Wm, denote the visit, travel, and total workloads for each district m, respectively. The proposed bi-objective mixed-integer program follows:
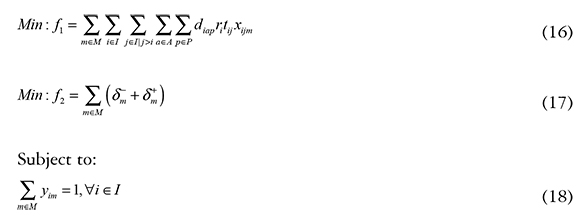
The objective function (16) minimizes the sum of the total Travel Workload between basic units for every district, such that (i, j) pairs e I | j > i (selecting i and j in this way helps reduce computational time). Including parameter ri in this equation allows considering the security level of each basic unit, and therefore quantifying the total or partial satisfaction of demand requirements for each basic unit i ∈I. On the other hand, the objective function (17) minimizes the sum of the total Workload Deviations from the average workload w, among all districts. Constraints (18) ensure that each basic unit is assigned to only one district. Constraints (19)—(21) are logical inequalities that control the assignment of each basic unit to each district, and the assignment of both units i and j to district m. Constraints (19) and (20) ensure that xijm must be equal to zero, unless both y and y are equal to 1. Constraints (21) ensure that if both basic units i and j are selected for district m, then xijm must have value 1. Note that the inclusion of decision variables xijm and constraints (19)—(21), corresponds to the linearization of the product of variables (yim * yjm) as an equivalent quadratic formulation in the objective function f1 (i.e. replacing xijm for (yim * yjm) in f1). Constraints (22)—(25) estimate respectively visit Vm, travel Tm, and total Wm workloads for each district m, and the average workload W for a selected districting configuration. All workload metrics are measured in time units (hours/year). In constraints (26), lower and upper deviations from the average workload W of each district m are quantified. Finally, constraints (27)—(29) define the domain of the decision variables.
In order to evaluate improvements in the structure of the model proposed in (16)—(29) and achieve possible reductions in computational times, we changed the formulation estimating the Workload Deviations as the range between the maximum and minimum workload of all districts Wmax and Wmin, i.e. (Wmax — Wmin). We did this including constraints Wmax > W and Wmin < Wm, and replacing f2 with Min: f2 = (Wmax - Wmin). These changes also allowed excluding the strict equality constraints (25) and (26) defining a larger feasible region. We performed a set of computational experiments with these changes and found that when values of i ∈I and m ∈M increase, computational times are equivalent to those obtained with the initial formulation of Workload Deviations. Therefore, we maintained the original formulation proposed.
2.3. Solution Strategy
We follow a lexicographic approach [33]-[35], to solve the proposed mathematical model presented in equations (16)-(29). This solution strategy allows decision makers to interactively incorporate their preferences as the decision criteria are optimized under the lexicographic order. Moreover, this approach narrows the set of possible solutions to only those with a good compromise of objectives, facilitating the final choice to the decision maker [36]. The ordering of objectives f1 and f, as they are shown in equations (16) and (17), was established based on the regional study of HHC providers [2]. The study identified that reducing travel time of the medical staff is a priority for HHC providers. Moreover, it was evidenced that no attention has been given to balance workloads among districts.
Firstly, each objective (16) and (17) is optimized individually, subject to constraints (18)—(29), referred as Ω. The optimal value of each objective l is denoted by f1*. This process was implemented testing different districting configurations varying the values of m. Besides objectives f1 and f2, we evaluated two performance metrics: Total Workload TT and Average Workload. Since the total Visit Workload VT is independent of the districting configuration (VT represents constant yearly demand for services), these two metrics depend on the Travel Workload, and thus they vary according with the configuration. Therefore, we also evaluated the ratio TT/VT (i.e. the ratio between visit time and travel time).
Secondly, we evaluate the efficient frontier and identify the trade-offs between objectives (16) and (17). For doing so, we implement the ideas from [37] and estimated a compromise threshold for objective f1, denoted by (1 + α), where α represents the maximum acceptable deterioration of objective f1. We solved the model to optimize the objective shown in (17), subject to the set of constraints f1. We added constraint (30), which guarantee that the new solution is at least 100 (1 + α)% of the total travel distance achieved in f1*.
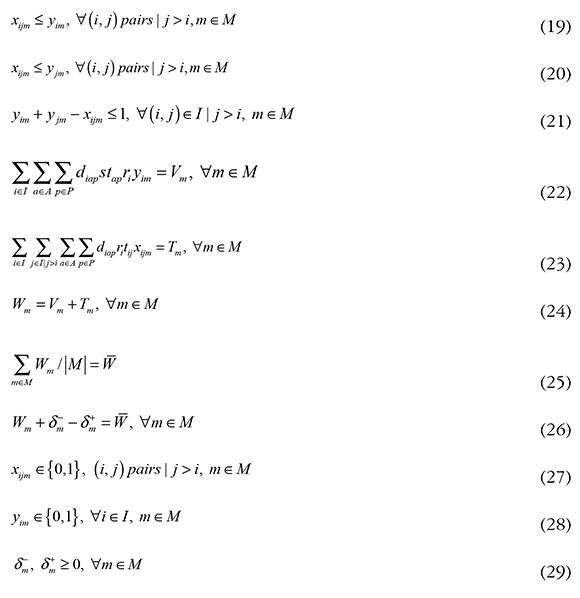
This procedure allows identifying acceptable trade-offs between objectives to produce good compromise solutions. Moreover, HHC managers can evaluate possible deteriorations on Travel Workloads to obtain more balanced districting configurations and improve Workload Deviations.
We implemented the solution approach in Xpress-IVE (version 7.4), in a Dell computer with a 2.67 GHz processor and 4,00 GB in RAM. Computational times to solve each objective functions varied between 86 seconds and 72 minutes, and complete solutions for all the approaches were obtained in less than 120 minutes.
3. Results
The model proposed to incorporate the factors generated by a rapid-growing city feature into the DPHHC and the solution strategy were evaluated with a case study of a rapid-growing city, located in Colombia, Latin America. Real data were used to solve a particular instance of a large HHC provider in the country, and to randomly generate a set of data instances to evaluate the robustness of the model. In this section we provide a detailed explanation of the case study, the data collection and estimation, and the obtained results.
3.1. Case Study: City of Cali, Colombia
According to the city annual report, Cali's population in 2013 reached 2,253,797 inhabitants, and projections to 2015 estimate a population nearly 2,4 million in the urban area [38]. By Agreements 15 of 1988, 10 of 1998 and 134 of2004, the city was divided into 22 communes. The total surface of the city is 560.3 square kilometers, which results in a density of 4,200 inhabitants per square kilometer. Figure 1 illustrates that Cali's population varies significantly among communes. Furthermore, socio-economic distribution varies among communes, which induces a higher variability between the different types of SSS, including the population covered by the Sistema de Selección de Beneficiarios para Programas Sociales (SISBEN).
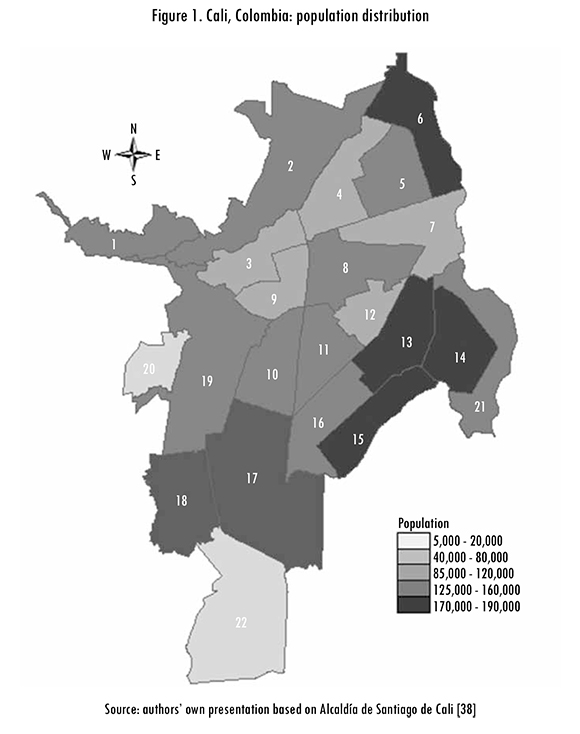
Based on a study of HHC providers in the state of Valle del Cauca [2], and a collaborative work with one of the two largest HHC institutions in Colombia, we modeled, implemented, and validated a DPHHC of the urban area of Cali, capital of the state. Problem instances are based on real data provided by a HHC institution in Cali, which annually takes care of nearly 16.000 patients, employs 560 people of more than five types of medical staff, and provides eight types of HHC services. This institution offers HHC services in 12 cities in the country, which includes Bogotá, Cali, Medellín, Barranquilla and Cartagena, among the largest cities in Colombia.
As many other HHC providers that participated in the study of the state, the institution that provided real data reported improvement challenges in the districting configuration. As a major issue, the institution claimed an inefficient staff assignment to patient visits, which has led to hire more medical staff. However, despite the increment of workforce, managers and administrative staff complain about unbalanced work assignments among districts. As it could be evidenced, this imbalance is due to the diversity of demand for medical activities, the types of patients, and the types of medical staff. Having a district with 100 acute patients that require a single Practitioner Visit each, is different from having a district with 100 chronic patients that require Auxiliary Nursing Care each or a set of Therapies. Moreover, HHC providers reported that risk levels in communes are becoming a security issue for medical staff.
3.2. Data Collection and Parameter Estimation
We obtained real data of demand for HHC services in Cali and estimated the annual demand for the eight types of services delivered in each of the 22 communes of the city. We also estimated the standard service time for each service, and the definition of each type of medical staff to perform every service (see Table 2). General characteristics of the HHC services data obtained are as follows:
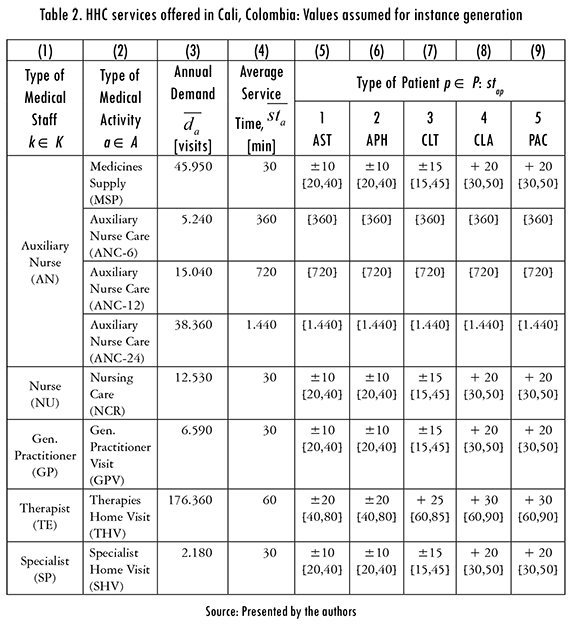
- Families of medical activities a ∈A are defined based on the type of staff member k ∈K that can perform each type of activity, identified by the subset Sk.
- The set of medical activities a ∈A include eight types: (1) Medicine Supply (MSP), (2) Auxiliary Nurse Care 6-Hours (ANC-6), (3) Auxiliary Nurse Care 12-Hours (ANC-12), (4) Auxiliary Nurse Care 24-Hours (ANC-24), (5) Nursing Care (NCR), (6) General Practitioner Visit (GPV), (7) Therapist Home Visit (THV), and (8) Specialist Home Visit (SHV).
- The set of type of medical professionals k ∈K includes five types: Auxiliary Nurse (AN), Nurse (NU), General Practitioner (GP), Therapist (TE), and Specialist (SP).
- The subset Sk is defined as follows: AN can perform medical activities (1) to (4), NU performs activity (5), GP activity (6), TE activity (7), and SP activity (8).
- Five types of patients are considered based on the results of [27]: (1) Acute Short-Term (AST); (2) Acute Post-Hospitalization (APH); (3) Chronic Long-Term (CLT); (4) Chronic Loss of Autonomy (CLA); (5) Palliative Care (PAC). In order to generate random test instances we considered the following rules:
- Service times for each type of medical activity and each type of patient are presented in Table 2. Column (4) indicates the average service time sta for activity a ∈A, and columns (5) to (9) indicate the ranges sta ± βp for each type of patient p ∈P, where βp represents the time services deviation for the type of patient p ∈ P.
- Security level estimations for each basic unit were based on violence registers of the city [38], due to the lack of real data availability in HHC providers' registers. Violence acts registers were used to estimate relative violent acts of the total violent acts of the city. Nine basic units correspond to those with the largest proportion of violent acts (42% approx.), and were given a value of r = 0.6. Seven basic units correspond to the 30% of violent acts and were given a value of r = 0.8. Six basic units represented 28% of violent acts and were given a value of r = 1.0.
- To randomly generate security levels ri were generated for each basic unit i ∈I. The factor will be one of the three scales of security: [0.6; 0.8; 1.0].
- Given the size of the case study city, we considered an urban area of a size of I = 20. This size corresponds to an urban area of 1.600 square kilometers (40km x 40km).
- To estimate distances and travel times among basic units, the Euclidian distance was computed first, from the center point of each pair of basic units (i, j) e I. Then, based on results of circularity indexes studied by [39] and [40] in the Colombian context, we considered a circularity index factor of 1,302 and an average speed of 40 km/hour.
3.3. Results Analysis and Discussion
Table 3 presents the results obtained when minimizing objective f1, for values of m, from 1 to 12. As expected, and given the structure of equation (16) to estimate Travel Workload, the value of objective f1 decreases as the number of districts increases. Furthermore, Total Workload, Average Workload, and Ratio TT/ VT decrease as the number of districts increases (see Figure 2). However, Workload Deviations increase from m = 1 to m = 3 and reach the minimum possible value at 79.658 hours/year, when m = 4. The improvement of objective function f1 implies a deterioration of objective f2, which evidences the trade-off between the two objectives.
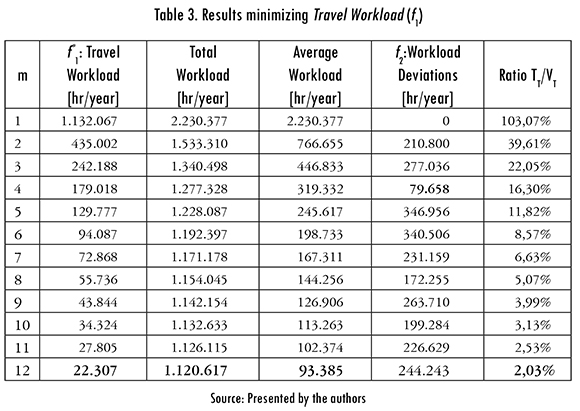
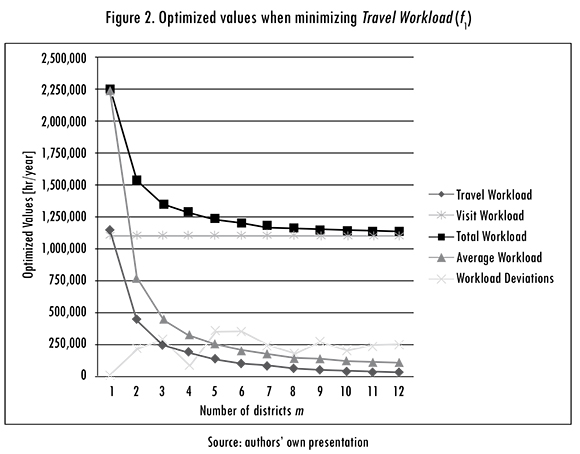
Table 4 presents the results obtained when minimizing objective f2, for values of m, from 1 to 12. As expected, Workload Deviations increase as the number of districts increases. Similarly to the results obtained when minimizing objective f1, Total Workload, Average Workload, and Ratio TT/VT decrease when increasing m. However, reduction rates behave differently. Although these metrics become larger when minimizing f2 (except when m = 5), higher reduction rates are achieved when values of m increases from 3-4, 4-5, and 7-8. Furthermore, variations of Travel Workload behave at different rates. As it is likely in this case, reduction rates are smaller to the point of impair Travel Workload when m increases from 6 to 7.
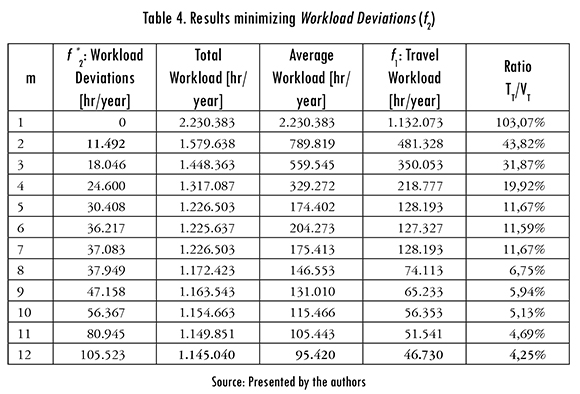
To identify the trade-offs between objectives f1 and f2, we obtain their efficient frontier (see Figure 3). We also identify the current districting configuration of the HHC institution that provided real data instances and the average configuration of the sector obtained in the study of the state. As observed, the highest improvements are achieved when the districting configuration changes from m = 1 district (no districting at all), to m = 2,3 (see Figure 2). Moreover, when comparing the frontier against real configurations (m = 3 for the HHC institution, and m = 8 for the sector), significant improvement opportunities are evidenced.
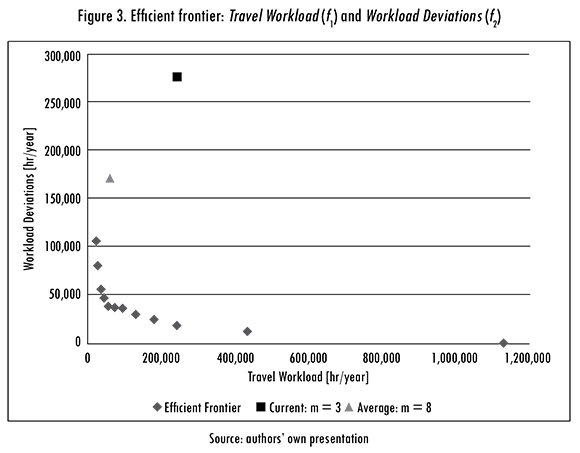
Better districting configurations, measured in Travel Workload and Workload Deviations, can be obtained when features of a rapid-growing city are integrally included in the analysis. The inclusion of the population disposition improves the estimation of patient' locations, distances and travel times, and their accessibility factors. The differentiation of types of medical activities, types of patients, and types of medical staff produces more accurate demand estimations, and therefore allows a better resource assignment. Also, the inclusion of security factors for each basic unit provides a more detailed control for services delivery.
To estimate compromise thresholds between objectives f1 and f2, we performed a parametric analysis of α, considering the average configuration of the HHC sector in the city, with m = 8. Furthermore, we selected such configuration given the stability that analysis values reach when m = 8 for bothf1 and f2Wfe evaluated values of α = 1%, 5%, 10%, 15% - 30%, since 30% is the maximum deterioration allowed for objective f1 (see Table 5). Given the structure of the mathematical model (16)-(29) and the integer-binary nature of variables xijmand yim, allowing deteriorations of (1 + α) f1* of Travel Workload results in districting configurations with values of f1 < (1 + α) f1*, and larger significant improvements of original Workload Deviations (f2) when minimizing objectivef1 in isolation (i.e. new f << original f2). To illustrate the effect of the compromise thresholds, the solutions of the optimization models are displayed using ArcGIS [41] (see Figure 4).
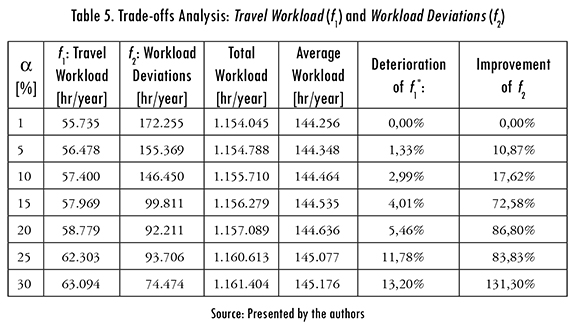
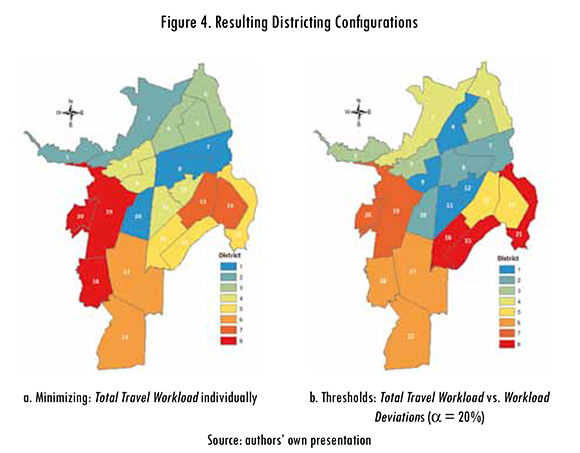
The trade-offs between objectives f1 and f2 are also illustrated in Figure 5. Deteriorations of f1* of less than 10% produce improvements off larger than 80%. See for example Table 5 and Figure5 when a = 20%. In that case, deterioration of f1* results in a 5.46% and produces an improvement off of 86.80% from the original value of f2 when minimizing objective f1 in isolation. These results show that small deteriorations in Travel Workload (less than 10%) can produce large improvements in Workload Deviations (more than 80%), obtaining therefore more balanced workloads and better districting configurations.
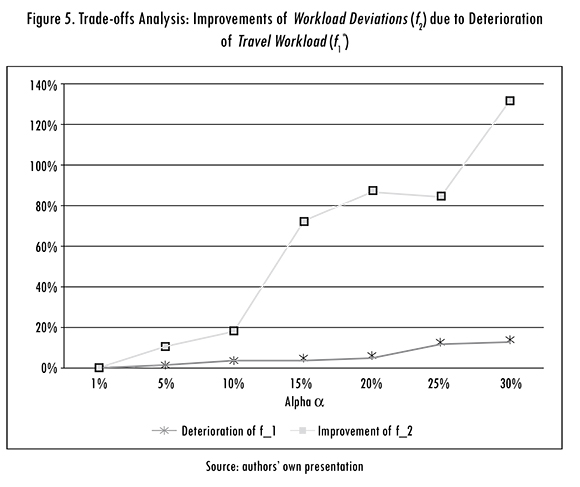
To evaluate the robustness of the model and the solution strategy proposed, we performed two sensitivity analysis oriented to evaluate the effect of key parameters. First, we evaluated the effect of variations on demand for HHC visits on the districting configuration, based on population growing patterns. Second, we solved a set of 30 instances in which service times, security levels and distances between basic units were randomly generated.
Consequently, first demand for HHC visits for five years ahead was estimated based on historical registers of demand for families of HHC services, and based on populations projections for the city of Cali, Colombia [38]. Using demand registers of three years in the past, we found a correlation of r = 0.98 between the increase of the number of visits and the increase of the annual population. We estimated the number of annual visits based on a lineal regression of the projected population [38]. The estimation of annual visits is illustrated in Table 6.
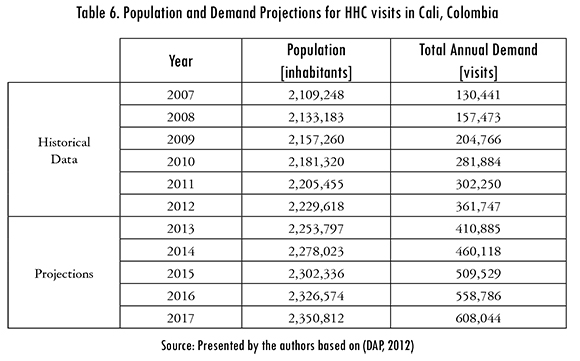
After applying the solution approach proposed, we found that the districting configuration obtained when m = 8 illustrated in Figure 5b generates the best thresholds between objectives f1 and f2 for the five years ahead in the projections for demand of HHC visits. The optimal values found for each objective and the performance metrics achieved are presented in Table 7. These results allowed demonstrating the robustness of the model and the solution method proposed in terms of the stability of the results given changes in demand for HHC services.
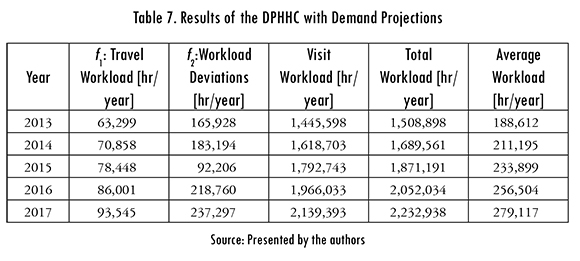
For the second sensitivity analysis we solved a set of 30 random instances, which were generated based on values defined for real parameters. First, we evaluated the DPHHC (16)-(29) for different values of the number of districts m, in the range [1%, 50%] of the number of basic units I. Then, after selecting the best value of m* in terms of objectivef1, we evaluated the compromised threshold (1 + α), for different values of α in the range [1%, 30%], and found the best possible combination of (1 + α) that provided the best trade-off between objectivesf1 and f. In other words, we found the best value of α that provided simultaneously the minor deterioration inf1* and the major improvement in f*2. The averages of the optimum values f1* and f* found by following this procedure for each set of instances and their corresponding standard deviation (indicated within parenthesis) are presented in columns (2) to (5) of Table 8. As it can be deduced from these values, coefficients of variability for these metrics vary from 3.56% to 5.90%, which evidences the stability of the experiments.
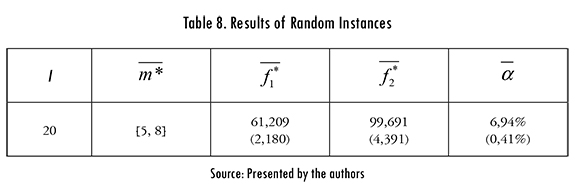
The range in which the best number of districts m* varies, for an instance of size I = 20, is [5,8]. This finding offers HHC managers better and more accurate ranges when defining districting configurations. In practice, most of the time the number of districts m is decided based on the detail of the basic unit data availability or by the numbers used in urban planning processes. This range allows ensuring that good compromised solutions can be found for districting decisions, in terms of the total travel workloads (f1) and balanced workloads (f2) among districts.
4. Conclusions and Future Research
We have studied the DPHHC in the context of a rapid-growing city, and described how socio-economic conditions of a developing country can be integrally included in the analysis through a bi-objective mathematical model. It was shown that better districting configurations, measured in Travel Workload and Workload Deviations, can be obtained when arising features are integrally included. The inclusion of the population disposition improves the estimation of patient' locations, distances and travel times, and their accessibility factors. The differentiation of types of medical activities, types of patients, and types of medical staff produces more accurate demand estimation, and therefore allows a better resource assignment. Also, the inclusion of security factors for each basic unit provides a more detailed control for services delivery.
The proposed solution method included a lexicographic order of the objectives and a maximum deterioration of the objective with higher priority. This approach allowed identifying the efficient frontier and trade-offs between objectives. These analyses showed that small deteriorations in Travel Workload (less than 10%) can produce large improvements in Workload Deviations (more than 80%), obtaining therefore more balanced workloads and better districting configurations. Furthermore, the analysis allows HHC managers studying a set of different possible solutions, varying the values in which each objective is deteriorated or improved. We are currently working on the staffing and the staff assignment problems, considering the hierarchical interdependency among districting and staffing decisions.
Finally, although the proposed model and its solution method for the DPHHC were implemented and validated in the case study for the urban area of Cali, the approach can be easily extended for other HHC providers in other cities in Colombia. This study allowed us to work with a wide range of actors of HHC services: general managers, administrative and health staff, medical staff and practitioners, and most importantly, patients. This experience showed that quantitative and qualitative techniques can be used to help health providers to improve the delivery of HHC services. Moreover, it was evidenced that medical criteria and better patients' characterizations can be integrally included in the analysis, and that administrative and economic criteria can be optimized, ensuring a good quality service to patients. Future research opportunities include the evaluation of the impact of the DPHHC on tactical and operative decisions in HHC logistics management.
References
[1] World Bank, (2012), "World Population Prospects", Total Population [online]. Available: http://data.worldbank.org/indicator/SPPOP.TOTL. [ Links ]
[2] E. Gutiérrez et al., "Gestión logística en la prestación de servicios de hospitalización domiciliaria en el Valle del Cauca: Caracterización y diagnóstico", Estudios Gerenciales-Journal Management Economics Iberoamerica, Vol. 30, no. 133, pp. 441-450. [ Links ]
[3] E. Gutiérrez and C. Vidal, "Home health care logistics management: Framework and research perspectives", International Journal Industrial Engineering Management (IJIEM), vol. 4, no. 3, pp. 173-182, 2013. [ Links ]
[4] E. Tarimo, Towards a healthy district: Organizing and managing district health systems based on primary health care. Ginebra: World Health Organization, 1991. [ Links ]
[5] M. Rasmussen et al., "The home care crew scheduling problem : Preference-based visit clustering and temporal dependencies", European Journal Operational Research, vol. 219, no. 3, pp. 598-610, 2012. [ Links ]
[6] B. Bozkaya, E. Erkut, and G. Laporte, "A tabu search heuristic and adaptive memory procedure for political districting", European Journal Operational Research, vol. 144, pp. 12-26, 2003. [ Links ]
[7] C. Chou, "A knowledge-based evolution algorithm approach to political districting problem", Computer Physics Communications, vol. 182, no. 1, pp. 209-212, 2011. [ Links ]
[8] J. Ferland and G. Guénette, "Decision support system for the school districting problem", Operations Research, vol. 38, no. 1, pp. 15-21, 1990. [ Links ]
[9] R. Garfinkel and G. Nemhauser, "Optimal political districting by implicit enumeration techniques", Management Science, vol. 16, no. 8, pp. B495-B508, 1970. [ Links ]
[10] S. Hess and S. Samuels, "Experiences with a sales districting model: criteria and implementation", Management Science, vol. 18, no. 4, pp. 41-54, 1971. [ Links ]
[11] S. Hess et al., "Nonpartisan political redistricting by computer", Operations Research, vol. 13, no. 5, pp. 998-1006, 1965. [ Links ]
[12] M. Hojati, "Optimal potilical districting", Computers Operations Research, vol. 23, no. 12, pp. 1147-1161, 1996. [ Links ]
[13] A. Mehrotra, E. Johnson, and G. Nemhauser, "An optimization based heuristic for political districting", Management Science, vol. 44, no. 8, pp. 1100-1114, 1998. [ Links ]
[14] F. Ricca and B. Simeone, "Local search algorithms for political districting", European Journal Operational Research, no. 189, pp. 1409-1426, 2008. [ Links ]
[15] N. Smith et al., "A hybrid metaheuristic approach to optimize the districting design of a parcel company", Journal Applied Research Technology, pp. 19-35, 2011. [ Links ]
[16] D. Wang, L. Jing, and Z. Zhou, "Dynamic modeling of political districting problem", New York, no. 1, pp. 1-4, 2009. [ Links ]
[17] D. Bandara and M. Mayorga, "Optimal geographical districting of emergency vehicles", en T. Doolen and E. Van Aken (eds.), Proceedings of the 2011 Industrial Engineering Research Conference, Reno NV, 2011. [ Links ]
[18] N. Bullen, G. Moon, and K. Jones, "Defining localities for health planning: a GIS approach", Social Science Medicine, vol. 42, no. 6, pp. 801-816, 1996. [ Links ]
[19] A. Iannoni, R. Morabito, and C. Saydam, "An optimization approach for ambulance location and the districting of the response segments on highways", European Journal Operational Research, vol. 195, no. 2, pp. 528-542, 2009. [ Links ]
[20] S. Lapierre, J. Myrick, and G. Russell, "The public health care planning problem: a case study using geographic information systems", Journal Medical Systems, vol. 23, no. 5, pp. 401-417, 1999. [ Links ]
[21] F. Pezzella, R. Bonanno, and B. Nicoletti, "A system approach to the optimal health-care districting", European Journal Operational Research, vol. 8, no. 2, pp. 139-146, 1981. [ Links ]
[22] N. Shortt et al., "Defining regions for locality health care planning: a multidimensional approach", Social Science Medicine, vol. 60, no. 12, pp. 2715-2727, 2005. [ Links ]
[23] M. Blais, S. Lapierre, and G. Laporte, "Solving a home-care districting problem in an urban setting", Journal Operational Research Society, vol. 54, no. 11, pp. 1141-1147, 2003. [ Links ]
[24] A. Bennett, Home health care logistics planning. Georgia: Institute of Technology, 2010. [ Links ]
[25] E. Benzarti, Home health care operations management: Applying the districting approach to home health care. Paris: École Centrale des Arts et Manufactures, 2012. [ Links ]
[26] A. Bennett Milburn, "Operations research applications in home healthcare", en R. Hall (ed.), Handbook of healthcare system scheduling. Boston MA: Springer, 2012. pp. 281-302. [ Links ]
[27] A. Hertz and N. Lahrichi, "A patient assignment algorithm for home care services", Journal Operational Research Society, vol. 60, no. 4, pp. 481-495, 2009. [ Links ]
[28] E. Gutiérrez and C. Vidal, "Home health care logistics management problems: A critical review of models and methods", Rev. Fac. Ing. Univ. Antioquia, vol. 68, pp. 160-175, 2013. [ Links ]
[29] United Nations, World urbanization prospects: The 2011 Revision. New York: United Nations, Department of Economic and Social Affairs, Population Division, 2011. [ Links ]
[30] Programa de las Naciones Unidas para el Medio Ambiente (PNUMA), Perspectivas del medio ambiente de América Latina y el Caribe. Panama, Panamá: PNUMA, 2010. [ Links ]
[31] United Nations Population Fund (UNFPA), Population dynamics in the least developed countries: Challenges and opportunities for development and poverty reduction. New York: UNFPA, 2011. [ Links ]
[32] O. Galvis y J. Mock-Kow, Propuesta metodológica para la zonificación del sector urbano en la prestación del servicio de hospitalización domiciliaria: Caso aplicado en Santiago de Cali. Cali: Universidad del Valle, 2014. [ Links ]
[33] V Chankong and Y Haimes, Multiobjective decision making: Theory and methodology. New York: North Holland, 1983. [ Links ]
[34] J. Cohon, Multiobjective programming and planning (2da. ed.). New York: Dover, 2003. [ Links ]
[35] A. Goicoechea, D. Hansen, and L. Duckstein, Multiobjective decision analysis with engineering and business applications. New York: Wiley, 1982. [ Links ]
[36] M. Alves and J. Clímaco, "A review of interactive methods for multiobjective integer and mixed-integer programming", European Journal Operational Research, vol. 180, no. 1, pp. 99-115, 2007. [ Links ]
[37] J. Sefair et al., "Locating neighborhood parks with a lexicographic multiobjective optimization method", en M. P Johnson (ed.), Community-based operations research: Decision modeling for local impact and diverse populations. New York, NY: Springer, 2012. pp. 143-171. [ Links ]
[38] Alcaldía de Santiago de Cali, Departamento Administrativo de Planeación (DAP), Cali en cifras. Cali: DAP, 2012. [ Links ]
[39] G. Vidal, Introducción a los sistemas de transporte y optimización de cadenas de abastecimiento. Santiago de Cali: Programa Editorial, Universidad del Valle, 2013. [ Links ]
[40] D. López y I. Zapata, Modelo para el diseño de rutas del personal asistencial en hospitalización domiciliaria en la ciudad de Cali. Cali: Universidad del Valle, 2014. [ Links ]
[41] ESRI, ArcGIS 10.2: Getting started with ArcGIS. Redlands, CA: ESRI Press, 2013. [ Links ]