Servicios Personalizados
Revista
Articulo
Indicadores
-
Citado por SciELO
-
Accesos
Links relacionados
-
Citado por Google
-
Similares en SciELO
-
Similares en Google
Compartir
Ingeniería y Universidad
versión impresa ISSN 0123-2126
Ing. Univ. vol.19 no.2 Bogotá jul./dic. 2015
https://doi.org/10.1114/javeriana.iyu19-2.eaar
Effects of the Attributes Associated with Roadway Geometry, Traffic Volumes and Speeds on the Incidence of Accidents in a Mid-Size City1
Efectos de los atributos asociados a geometría vial, volúmenes vehiculares y velocidades en la incidencia de accidentes en una ciudad intermedia2
Thomas Guerrero-Barbosa3
Yenica Espinel-Bayona4
Darwin Palacio-Sánchez5
1Reception date: December 13th, 2013. Acceptance date: February, 9th, 2015. This study was supported by research funds from Research and Extension Division (DIE) of University Francisco de Paula Santander Ocaña through the Project with code 158,04,009 and Record start A-IV-DIE-001 of May 27th, 2012.
2Fecha de recepción: 13 de diciembre de 2013. Fecha de aceptación: 9 de febrero de 2015. Este estudio fue apoyado por fondos de investigación de la División de Investigación y Extensión (DIE) de la Universidad Francisco de Paula Santander, Ocaña, a través del Proyecto con código 158,04,009 y Acta de inicio A-IV-DIE-001 del 27 de mayo de 2012.
3Ingeniero civil, Universidad Francisco de Paula Santander Cúcuta, Colombia. Maestría en Ingeniería Civil, Universidad del Norte, Barranquilla, Colombia. Docente del Departamento de Ingeniería Civil, Universidad Francisco de Paula Santander, Ocaña, Colombia. E-mail: teguerrerob@ufpso.edu.co
4Ingeniera civil, Universidad Francisco de Paula Santander, Ocaña, Colombia. E-mail: yenika-ryk@hotmail.com
5Ingeniero civil, Universidad Francisco de Paula Santander, Ocaña, Colombia. E-mail: darwinjose10@hotmail.com
Para citar este artículo / To cite this article
T. Guerrero-Barbosa, Y. Espinel-Bayona, and D. Palacio-Sánchez, "Effects of the attributes associated with roadway geometry, traffic volumes, and speeds on the incidence of accidents in a mid-sized city", Ing. Unv., vol. 19, no. 2, pp. 351-367, 2015. http://dx.doi.org/10.1114/javeriana.iyu19-2.eaar
Abstract
Currently traffic accidents are the second cause of death, after homicides, in Colombia. Hence, government institutions require decision-making tools to identify and tackle the causes of traffic accidents. This research aims to determine the influence of factors related to roadway geometry and conditions, and traffic volumes and speeds on the frequency of accidents on the urban road network in the city of Ocaña (Colombia) This study took a methodological modeling approach, which included measuring variables in the field, creating an accidents database, and conducting a subsequent analysis of the data by calibrating a model based on Poisson and Negative Binomial regressions. The results showed that variables such as road width, number of intersections, pavement type, traffic volumes (broken down into motorcycles, light, and heavy vehicles), and average driving speed (50th percentile) relate to accident rates.
Keywords: road accidents; accident frequency; roadway geometry; traffic volumes; average speed
Resumen
Actualmente en Colombia, los accidentes de tránsito constituyen la segunda causa de muerte después de los homicidios; por esta razón, las instituciones gubernamentales necesitan herramientas de decisión con las cuales sea posible conocer y atacar las causas que provocan este tipo de accidentes. La presente investigación desea determinar la influencia de los factores asociados a la geometría y entorno vial, a los volúmenes vehiculares y a las velocidades en la frecuencia de accidentes en la red vial urbana de la ciudad de Ocaña (Colombia), partiendo de un enfoque metodológico de modelación que incluye medición de variables en campo, conformación de una base de datos de accidentes y su posterior evaluación, que calibra un modelo basado en regresiones tipo Poisson y Binomial Negativa. El análisis de los resultados arrojó que variables como el ancho de calzada, número de intersecciones, tipo de pavimento, volúmenes vehiculares (desagregado en motocicletas, vehículos livianos y pesados) y la velocidad media de circulación (Percentil 50) se relacionan con las tasas de accidentes.
Palabras clave: accidentalidad vial; frecuencia de accidentes; geometría vial; volúmenes vehiculares; velocidad media
Introduction
The World Health Organization (WHO), an international leading agency in this field, confirms the fact that road accidents have become a growing epidemic. As such, this phenomenon is deeply troubling because every year it claims the lives of about 1.3 million people worldwide, becoming a public health issue with a high economic impact. This has led to the creation and development of a large number of alternatives and/or policies intended to provide solutions that might reduce the different metrics used to measure this phenomenon [1].
Colombia is not the exception to this problem. According to statistics released by the National Institute of Legal Medicine and Forensic Sciences (INMLCF), every 2.5 minutes there is a traffic accident, every 10 minutes someone is injured, and every 69 minutes someone dies, which means that 20 Colombians die every day, and 144 are injured, as a result of unsafe roads. Specifically, roads are unsafe due to roadway conditions, reckless drivers, traffic increase, and speeding, which is usually one of the leading causes of accidents on the highways and country roads of the nation [2]. In 2009, there were 39,167 people injured and 5,796 dead as a result of traffic accidents; motorcycle riders were the most prominently involved with 47,662 injured (equivalent to a 45%) and 6,274 dead (equivalent to a 39% of the total). Compared to 2008 figures, the percentage of deaths remained the same, while the number of injuries increased by 2%. Pedestrians were the second most affected with 9,542 injured (24%) and 1,748 deaths (30%) [2].
In the province of Ocaña [3] statistics show a 175% increase in the number of accidents from 2007 to 2011; in 78% of cases at least one motorcycle was involved. There is no research evidence demonstrating the significant factors that caused the accidents in the study area.
This statistical record demonstrates the need for developing policies aimed at reducing the frequency of accidents along with their severity. Relevant literature addresses the use of discrete-choice models such as the multinomial logit model or the ordinal logit model, used alternatively to model collision severity, where the accident record can be initially estimated from univariate count models such as the Poisson model or the Negative Binomial (NB) model [4], [5]. Some studies reported by Wang et al. [6] and Qi et al. [7] model collision frequency and severity separately. The first step in addressing this epidemic the right way is to understand how the traffic accident may occur, so that we may then look at preventing it and reducing its severity. It is important to clearly understand the causes of accidents, their characteristics, and the places where they occur frequently. In this case, there is extensive literature that has studied the effect of the factors that cause accidents, as sometimes the implementation of coun-termeasures and their subsequent evaluation -based on before-after approaches with Empirical Bayes and Full Bayes techniques [8], [9]- does not guarantee solving the problem of road accidents, seeing as reality is a little more complex, and thus tackling the addressed problem differently.
There are several factors involved in the occurrence of traffic accidents. Kar-laftis and Golias [10] looked at the relationship between the geometric characteristics of rural roads, accident rates, and related predictions using a regression model based on a hierarchical tree. Their research focused on two main goals: the first, to develop a methodology that quantitatively evaluates the effects of various geometric characteristics on accident rates, and the second, to provide a robust mathematical model for predicting the accident rates on rural roads. The results show that variables associated with design geometry and pavement conditions are relevant factors affecting the frequency of accidents. Other studies conducted in Malaysia [11] identified the factors that influence the frequency and severity of frontal collisions that occurred in 448 segments of five federal roadways. Model results revealed that the degree of horizontal curvature, type of terrain, volume of heavy vehicles, and number of intersections have a proportional direct relationship with the frequency of frontal collisions, while speed limit and shoulder width decrease in relation to accident frequency. Ayati and Abbasi [12] used Negative Binomial regression (NB) and Poisson models to identify the factors that affect the occurrence and severity of traffic accidents. Accidents were classified into two groups: no injuries (property damage only) and serious (injuries and fatalities); whereas motor-vehicle traffic was grouped into three types (private cars, heavy vehicles, and light vehicles), based on the records of past accidents on urban highways in Mashhad (Iran). The results showed that light vehicles play the most important role in the occurrence of accidents on urban roads for both types of accidents. In the state of Tennessee (U.S.), Dong et al. [13] established a relationship between collision frequency, road conditions, traffic conditions, and geometric-design features for 603 intersections. The available accident data corresponded to 7,840 events that occurred between 2001 and 2005. The results of the investigation determined that factors such as traffic volume and percentage of heavy vehicles were identified as significant in explaining collision frequency in the multivariate fixed-parameters zero-inflated negative binomial model; whereas the multivariate random-parameters zero-inflated negative binomial model showed that parameters such as International Roughness Index (IRI), the number of exclusive left-turning lanes, the number of exclusive right-turning lanes, and the established speed limit, significantly influence the occurrence of accidents.
The main objective of this research is to determine the effect of factors related to roadway geometry and conditions, and traffic volumes and speeds on the frequency of accidents within the urban perimeter of a mid-size city (Ocaña, Colombia). This study will take a methodological modeling approach, based on the measurement of variables in the field, the creation of an accidents database and its subsequent evaluation by calibrating a model based on regressions. Although the aim of this research is not to assess which is the best methodological approach to study the influence of these variables, suggestions of possible models are made nonetheless, seeing as during the course of the investigation, improvement in outcomes became evident when using multivariate models such as random-effect Negative Binomial, truncated Poisson, truncated Negative Binomial, zero-inflated Poisson, zero-inflated Negative Binomial, all of which yielded good estimation results from the relevant parameters. However, these models have their limitations, especially their inability to handle excess of zero records [13].
The current research did not consider the effect of variables associated with the physical and/or mental condition of the driver (e.g., alcohol/drug use, age, fatigue), weather, features of the vehicles involved, and the severity of accidents, which constitutes a limitation thereof.
The hypothesis of the study focuses on the premise that said effects can be quantified and analyzed through the calibration of statistical models, which will allow the agencies responsible for combating this epidemic to know the causes and, thereby, reduce the number of accidents. Calibration of the accident-frequency model was based on Poisson and NB regressions, seeing as there are plenty of studies in literature where they have been widely utilized [14]-[20]. Some studies make recommendations on the estimation of the model, as sometimes possible sources of error are evident in the prediction model due to the existence of collinearity between the dependent variables and an incorrect functional form of dependent and independent variables [21], [22]. The rest of the paper is divided as follows: the second section describes the methodological approach used and the characteristics of the data and variables used. The analysis and interpretation of parameter estimation are discussed in the third section. The fourth and final section summarizes and presents the conclusions.
1. Materials and Methods
1.1. Methodological Approach
According to Gazmuri [23], road accidents can be assimilated into a Poisson count process, for they occur in a given time interval and yield a non-negative integer value. In turn, this process of counting accidents must meet the following conditions, so that the probability distribution of the number of traffic accidents can approximate Poisson distribution: (i) The number of accidents observed in a time interval does not depend on previous time intervals (independent increments property); (ii) the amount of accidents observed in a time interval depends only on the length of the interval (stationary increments property); (iii) if the time interval approaches zero, chances are that no accident or that only one accident occurs (order property), and (iv) the two aforementioned properties mean that the number of accidents divided by the interval length tend toward a steady positive value, which represents the rate of occurrence of accidents per unit of time.
Accident-frequency models are based on modeling the number of accidents on a road section during a given time. In this case, the Poisson regression is estimated based on the following expression:

Where P (nij) is the probability of occurrence of n accidents on a road section i during period j. λij can be expressed as a vector of explanatory variables describing road geometry, traffic volumes, speeds, and other conditions that affect the frequency of accidents:

In our case, Xij is a vector that characterizes road geometry, traffic volumes, and speed, while β is a vector of calculable coefficients.
One of the main problems that may arise when using Poisson regression-based models is data overdispersion, that is, the variance tends to be larger than the average, thus underestimating the dispersion level of the result and affecting with some bias the estimated coefficients and their efficiency. For this reason it is advisable to use a NB distribution based on a Gamma distributed error term (εij). In this case, the expected value for the number of accidents is rewritten:

Thus, the variance (Var [nij]) can be expressed as a function of the mean (E[nij]) as follows:

The term a is the overdispersion parameter, so that if α is significantly different from zero, data is overdispersed, but if a is zero the NB distribution collapses to the Poisson distribution. The resulting probability distribution under the NB hypothesis is:

In the previous model the term Γ (.) is the value of the Gamma distribution. This widely used model can be estimated by maximum likelihood [14], [15], [24].
Note that a model consisting of several variables may present problems in its specification and subsequent calibration if multicollinearity is not controlled. This is because, although there is evidence that the property would not cause biased parameters nor affect the predictive ability of the model, it could, however increase the standard errors of the coefficients, especially those having little significance [25]. Multicollinearity could be detected in the low estimates of goodness-of-fit tests, due to high values in the coefficients of correlation between the variables and the sensitivity of the estimated coefficients as per specification.
1.2. Accident Data and Variables Used
A database was prepared containing the records of accidents that occurred in the urban perimeter of the municipality of Ocaña between the years 2007 and 2013 (up to August), which is suitable for this type of study according to reports of other investigations that calibrate models based on historical data for a shorter time period [12], [14], [20]. At the same time, and based on previous studies [3], we identified 15 road-traffic corridors for Ocaña. Figure 1 shows a photographic record of some of the sections studied. In summary, a total of 1,062 accidents were reported, spread out among the 15 sections studied. It must be clarified that in countries such as Colombia (particularly in Ocaña), accident records are obtained from the National Police and other entities such as the Volunteer Firefighters' Corps and/or Civil Defense, Ocaña Branch which, given the way the information is collected, may entail some disadvantages [26], [27]. For this study, the response variables are the number of accidents per road section analyzed. The geographically referenced location of the accident allowed us to link it to one of the 15 sections studied; in some cases the accident records did not include a georeferenced location, which led to section aggregation by lengthening sections. This was also an additional reason for not considering a greater number of sections in this study.
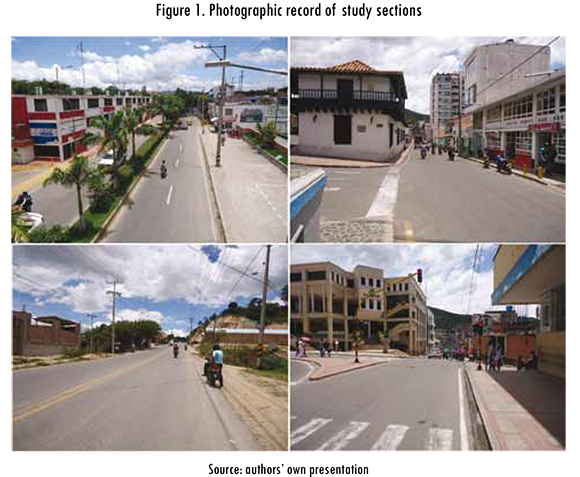
The explanatory variables for the calibration of the accident-frequency model were divided into three groups: the first group contains factors associated with roadway geometry and conditions, the second corresponds to traffic volumes, and the third one to speeds. The reason for considering these variables in the study basically relates to evidence previously reported in other research, which is referenced in the Introduction section of this document, as well as to certain firsthand information of our own; other variables which we would have liked to evaluate (e.g., weather conditions or severity of the accident) were not available. The summary of the variables and their description is shown in Table 1, as well as the minimum, maximum, mean, and standard deviation (SD).
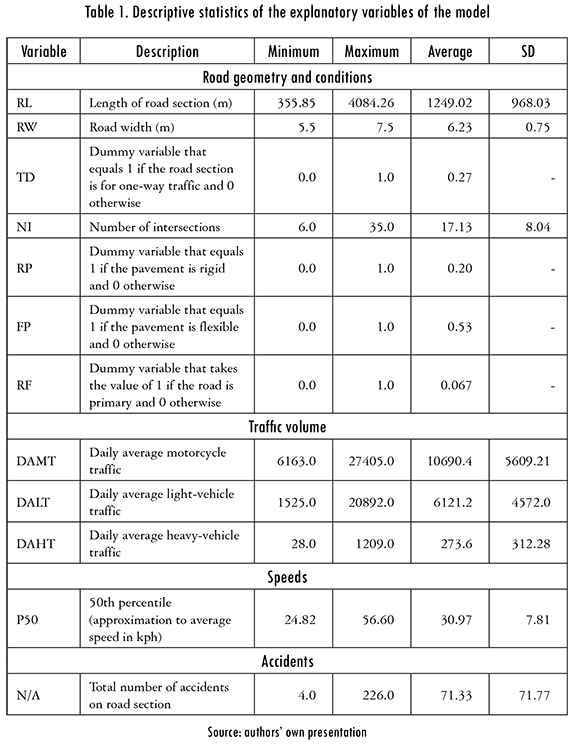
All the variables associated with road geometry and conditions, and traffic volumes and speeds were measured during field visits to the sections under study. The length of the sections was considered taking into account the geometric uniformity along the section, having recorded minimum and maximum values for this feature ranging from 355.85 m to 4,086.26 m respectively. There is evidence in the literature where the length of the sections varies between 25 m and 3,000 m [28], and between 80 m and 11,200 m [29], hence concluding that the section length in our case falls within the range of other previous research. The variable characterizing pavement type considered three types of pavement variations: rigid, flexible, and rigid-flexible (mixed); for the purposes of this study, rigid pavement (RP) and flexible pavement (FP) were taken as fixed variables. The variable Road Functionality (RF), made up of primary, secondary, and tertiary roadways, was adopted from the information reported in the Basic Land-Use Plan [30]. The measured traffic volumes vary widely among the dataset analyzed; the greatest degree of data dispersion in relation to the average value is found in the average motorcycle traffic (SD = 5,609.21). Regarding the P50 variable, an average speed of 30.97 km/h was estimated for the study sections. This study had no access to records of variables associated with the driver, weather, features of the vehicles involved, and accident severity, which constitutes a limitation thereof.
2. Results
The accident-frequency models were estimated based on Poisson and NB regressions which, as already indicated above, are widely used to model regressions for this type of phenomena. The results of the estimation are summarized in Table 2. For both regressions, the statistical significance of the coefficients of the variables RW, NI, RP, FP, DAMT, DALT, DAHT, and P50 is conveyed by the P_value (in parentheses), as a P_value of less than or equal to 0.05 indicates that the coefficient is significantly different from zero for a confidence level of 95%. The estimates calculated for variables RL, TD, and RF variables were not found to be significant. The variables show the expected sign according to work performed. The positive sign accompanying the parameters indicates an increase in the number of accidents, while a negative sign indicates a decrease. Estimators such as the Akaike Information Criterion (AIC) [31], the Bayesian Information Criterion (BIC) [32], and the Log likelihood were considered for the evaluation and measurement of the relative quality of the statistical model extracted from the dataset. The model works best when the test values are smaller.
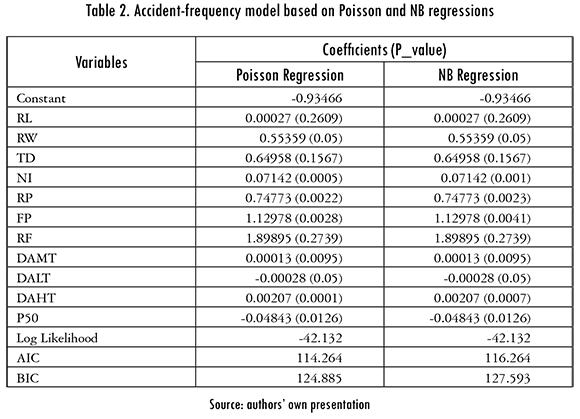
For the RW, NI, RP, and FP variables, the parameters of which are estimated in the models, they exhibit a behavior that is directly proportional to the frequency of accidents, so that if any of these variables increases, the frequency of accidents will also show an increase and vice versa. In particular, the positive value in the parameters of the RW variable might be due to the fact that the wider the road is, the more interaction there is between vehicles, so the probability of collisions increases, a result consistent with Noland and Oh [33], Chang [34], and Milton and Mannering [35]. The NI variable yielded a similar behavior: the more intersections in the road section, the greater the number of accidents, a hypothesis equally confirmed by Hosseinpour et al. [11], Ivan and O'Mara [36], and Garcés [37]. This is due to the fact that the more access points a road section has, the higher the risk of collisions is between vehicles and/or pedestrians due to crossing conflicts. Among the variables associated with road geometry and conditions, NI was found to be the most significant. Regarding the positive values for the RP and FP variables, it means that in those sections where the pavement is rigid or flexible the occurrence of accidents is more likely, while the opposite occurs when the pavement structure is rigid-flexible (mixed). Moreover, allow us to clarify that in those sections where the pavement is flexible there are more accident events than in those where the pavement is rigid. Research reported by Choocharukul [38] shows the significance of pavement surface in the predictions of accident-frequency models, which are consistent with the results of this research.
The DAHT variable was the most significant of the whole set. The positive values of the coefficients associated with DAMT and DAHT indicate that the higher the traffic volume of motorcycles and heavy vehicles, the higher the number of accidents. In the case of heavy vehicles, specifically, this could be because these vehicles travel at lower speeds, thereby increasing the risk of accidents with vehicles that will attempt to overtake them. This is consistent with the findings of Hosseinpour et al. [11], who found that 88% of the sections studied are two-way roads without separation; in our case it corresponds to 93%. In these circumstances, overtaking vehicles increase their speed to pass slow-moving vehicles, and therefore the probability of finding an oncoming vehicle from the opposite direction increases. The calibrated model shows the statistical significance of the DALT variable, and its negative value indicates that the expected number of accidents increases with a decrease in light-vehicle traffic. This may be because in the examined corridors where accident frequency is higher, the predominant vehicles are motorcycles and heavy vehicles; in fact, other research done in the study area indicates that in 78% of the reported accident cases at least one motorcycle is involved [3], highlighting the need to focus road safety policies on the drivers of such vehicles. Similarly, the driving behavior displayed by motorcycle and heavy-vehicle drivers is much more aggressive and evidences little training compared with drivers of light vehicles.
Regarding the calculation of the P50 variable, the statistical significance for both models that relate the occurrence of accidents is clear; however, its negative value in the parameter indicates that with an increase in the average speed on the sections studied, the number of accidents will decrease, a result which is not common despite being consistent with that obtained by Navon [39] and Rangel et al. [20]. One of the possible causes of this result is that the standard deviation of the average speed on the sections studied is very low, so that the speed range of those sections would be small. In our case the SD value for the P50 variable is 7.81 kph, a value consistent with the evidence reported by Rangel et al. [20], which shows SD variations for the speed parameter of 13.83 kph, 7.00 kph, and 6.42 kph for the years 2007, 2008, and 2009, respectively.
Although the RL, TD, and RF variables were not significant for a confidence level of 95%, the values found are consistent with other research, that in some cases does significantly explain the frequency of accidents [6], [17], [20], [37].
With regard to the two types of regressions used in this research, a remarkable equality between the estimated parameters for each one of the variables considered is evident. This situation shows that the term a, associated with the overdispersion of the data with which the models were estimated, is not significantly different from zero, i.e., the data is not overdispersed, which is the reason why the NB distribution collapses to the Poisson distribution. In this research case it is appropriate to say that the best approximation to the modeled phenomenon occurs when the estimates are calculated using the Poisson regression.
Conclusions
The predictive models for estimated frequency of accidents establish that the frequency of accidents has a causal relation with respect to geometric road features, conditions, type of vehicles, and average speed. Models were evaluated using a systemic approach characterized by functional form, considering not only technical components, but also factors related to human behavior, reflected in the speed component. From this study it can be concluded that factors associated with road geometry and conditions (road width, number of intersections, rigid or flexible pavement), traffic volumes (daily average traffic of motorcycles, light vehicles, and heavy vehicles), and speeds (average speed) influence the frequency of accidents on the urban road network in the city of Ocaña. By contrast, variables such as length of road section, traffic direction, and road functionality are not variables that explain the phenomenon of accident frequency in a significant way.
The estimated model exhibits the statistical significance of traffic volumes in accident frequency, showing the effect of different vehicle compositions within the studied phenomenon. It is clear that a greater number of motorcycles and heavy vehicles increases the number of accidents, while the effect is inversely proportional with respect to the number of light vehicles. The effect of the P50 variable was contrary to the one initially expected; however, this result was consistent with the relevant literature explored.
The result of the variables road width, number of intersections, and pavement type (rigid or flexible) was significant, seeing a higher incidence of accidents in those sections where pavement is flexible. In general, it may be concluded that if government agencies wish to reduce accident rates in the study area, they will need to make investments and generate public policies in relation to some aspects associated with road geometry and conditions, considering that this research provides evidence for undertaking a review of the rules governing road-geometry design (specifically as it relates to road width and design of intersections), and urban road construction.
Other policies must focus particularly on heavy vehicles and motorcycle drivers, especially the latter, since the activity known as mototaxi is very common in our country. This form of transportation is an informal means of employment with very few safety standards, which contributes to increasing not only the number of accidents, but the number of people involved. The use of public transportation needs to be encouraged as to cause a reduction in the use of informal transportation and, hence, motorcycle traffic. Clearly, a reduction in motorcycle traffic will decrease the number of accidents. Police control of urban roads in the study area needs to increase as a preventive measure. Measures such as segregation of traffic flows according to vehicle type, exclusive lanes for motorcycles, construction of acceleration and deceleration lanes, and variants in populated areas (restricting the passage of heavy vehicles in urban centers) are all actions that will contribute to improving urban road safety.
The two types of regressions used were appropriate for our modeling purposes; however, the value of the parameter a tends to zero, collapsing the NB to a Poisson distribution. The main reason behind this result could be that the sample is not large enough to provide conclusive results, with the number of sections analyzed at 15, which constitutes a limitation of the study since, as mentioned in Section 1.2, some accident records lacked the exact georeferenced location of the event, and this led to section aggregation by lengthening the sections.
References
[1] Organización Mundial de la Salud, Estadísticas mundiales de salud. Ginebra: OMS, 2008. [ Links ]
[2] Instituto Nacional de Medicina Legal y Ciencias Forenses, Forensis 2012. Datos para la vida. Bogotá: INMCF, 2012. [ Links ]
[3] T. E. Guerrero, R. J. Gallardo y J. A. Córdoba, "Análisis estadístico y espacial de corredores viales críticos de accidentalidad en Ocaña, Colombia", Revista Institucional Universidad Tecnológica del Chocó, vol. 2, pp. 103-115, 2012. [ Links ]
[4] S.R. Geedipally, S. Patil and D. Lord, "Examination of methods to estimate crash counts by collision type", Transport. Res. Rec.: J. Transport. Res. Board, vol. 2165, pp. 12-20, 2010. [ Links ]
[5] G. H. Bham, B. S. Javvadi and U.R. Manepalli, "Multinomial logistic regression model for single-vehicle and multivehicle collisions on urban us highways in arkansas", J. Transport. Eng., vol. 138, no. 6, 786-797, 2012. [ Links ]
[6] C. Wang, M. A. Quddus and S. G. Ison, "Predicting accident frequency at their severity levels and its application in site ranking using a two-stage mixed multivariate model", Accident Analysis and Prevention, vol. 43, no. 6, pp. 1979-1990, 2011. [ Links ]
[7] Y Qi, R. Srinivasan, H. Teng and R. Baker, "Analysis of the frequency and severity of rear-end crashes in work zones", Traffic Injury Prevention, vol. 14, no. 1, pp. 61-72, 2013. [ Links ]
[8] R. Elvik, "A before-after study of the effects on safety of environmental speed limits in the city of Oslo, Norway", Safety Science, vol. 55, pp. 10-16, 2013. [ Links ]
[9] B. Persaud, B. Lan, C. Lyon and R. Bhim, "Comparison of empirical Bayes and full Bayes approaches for before-after road safety evaluations", Accident Analysis and Prevention, vol. 42, pp. 38-43, 2010. [ Links ]
[10] M. G. Karlaftis and I. Golias, "Effects of road geometry and traffic volumes on rural roadway accident rates", Accident Analysis and Prevention, vol. 34, pp. 357-365, 2002. [ Links ]
[11] M. Hosseinpour, A. Shukri-Yahaya and A. Farhan-Sadullah, "Exploring the effects of roadway characteristics on the frequency and severity of head-on crashes. Case studies from Malaysian Federal Roads", Accident Analysis and Prevention, vol. 62, pp. 209-222, 2014. [ Links ]
[12] E. Ayati and E. Abbasi, "Investigation on the role of traffic volume in accidents on urban highways", Journal of Safety Research, vol. 42, pp. 209-214, 2011. [ Links ]
[13] C. Dong, D. B. Clarke, X. Yan, A. Khattak and B. Huang, "Multivariate random-parameters zero-inflated negative binomial regression model: An application to estimate crash frequencies at intersections", Accident Analysis and Prevention, vol. 70, pp. 320-329, 2014. [ Links ]
[14] V. Shankar, F. Mannering and W Barfield, "Effect of roadway geometrics and environmental factors on rural freeway accident frequencies", Accident Analysis and Prevention, vol. 27, no. 3, pp. 371-389, 1995. [ Links ]
[15] M. A. Abdel-Aty and E. Radwan, "Modeling traffic accident occurrence and involvement", Accident Analysis and Prevention, vol. 32, pp. 633-642, 2000. [ Links ]
[16] L. W. Hiselius, "Estimating the relationship between accident frequency and homogeneous and inhomogeneous traffic flows", Accident Analysis and Prevention, vol. 36, pp. 985-992, 2004. [ Links ]
[17] C. Caliendo, M. Guida and A. Parisi, "A crash-prediction model for multilane roads", Accident Analysis and Prevention, vol. 39, no. 4, pp. 657-670, 2007. [ Links ]
[18] Thailand Accident Research Center, Development of Accident Prediction Model - Road Safety Knowledge Development and Dissemination. Bangkok (Tailandia), 2009. [ Links ]
[19] A. Couto and S. Ferreira, "A note on modeling road accident frequency. A flexible elasticity model", Accident Analysis and Prevention, vol. 43, pp. 2104-2111, 2011. [ Links ]
[20] T. Rangel, J. M. Vassallo and I. Herraiz, "The influence of economic incentives linked to road safety indicators on accidents. The case of toll concessions in Spain", Accident Analysis and Prevention, vol. 59, pp. 529-536, 2013. [ Links ]
[21] P. Greibe, "Accident prediction models for urban roads", Accident Analysis and Prevention, vol. 35, no. 2, pp. 273-285, 2003. [ Links ]
[22] M. Reurings, Accident Prediction Models and Road Safety Impact Assessment: A State of the Art. Final report. Deliverable D2.1 of the RiPCORD-iSEREST project. European Commission, Directorate-General for Transport and Energy. Bruselas, 2006. [ Links ]
[23] P. Gazmuri, Modelos estocásticos para la gestión de sistemas. Santiago de Chile: Ediciones Universidad Católica de Chile, 1995. [ Links ]
[24] S. P. Miaou, "The relationship between truck accidents and geometric design of road sections. Poisson versus negative binomial regressions", Accident Analysis and Prevention, vol. 26, no. 4, pp. 471-482, 1994. [ Links ]
[25] R. Ramanathan, Introductory Econometrics with Applications. Fort Worth, TX: The Dryden Press, 1995. [ Links ]
[26] J. Also and J. Langley, "Under reporting of motor vehicle traffic crash victims in New Zealand", Accident Analysis and Prevention, vol. 33, pp. 353-359, 2001. [ Links ]
[27] T. Brenac and N. Clabaux, "The indirect involvement of buses in traffic accident processes", Safety Science, vol. 43, pp. 835-843, 2005. [ Links ]
[28] S. Washington, M. Haqueb, J. Oh and D. Lee, "Applying quantile regression for modeling equivalent property damage only crashes to identify accident blackspots", Accident Analysis and Prevention, vol. 66, pp. 136-146, 2014. [ Links ]
[29] Y Lao, G. Zhang, Y Wang and J. Milton, "Generalized nonlinear models for rear-end crash risk analysis", Accident Analysis and Prevention, vol. 62, pp. 9-16, 2014. [ Links ]
[30] Alcaldía Municipal de Ocaña, Plan Básico de Ordenamiento Territorial del Municipio de Ocaña, Norte de Santander (PBOT), 2012. [ Links ]
[31] H. Akaike, "A new look at the statistical model identification", IEEE Transaction on Automatic Control, vol. 19, pp. 716-723, 1974. [ Links ]
[32] G. Schwarz, "Estimating the dimension of a model", The Annals of Stati, vol. 6, pp. 461-464, 1978. [ Links ]
[33] R. B. Noland and L. Oh, "The effect of infrastructure and demographic change on traffic-related fatalities and crashes. A case study of Illinois county-level data", Accident Analysis and Prevention, vol. 36, no. 4, pp. 525-532, 2004. [ Links ]
[34] L. Y Chang, "Analysis of freeway accident frequencies: negative binomial regression versus artificial neural network", Safety Science, vol. 43, pp. 541-557, 2005. [ Links ]
[35] J. Milton and F. Mannering, "The relationship among highway geometrics, traffic related elements and motor vehicle accident frequencies", Transportation, vol. 25, no. 4, pp. 395-413, 1998. [ Links ]
[36] J. Ivan and P. O'mara, "Prediction of traffic accident rates using Poisson regression", in Proc. 76th Annual Meeting of the Transportation Research Board, National Research Council, Washington, DC, 1997. [ Links ]
[37] IP Garcés, "Factores influyentes en la ocurrencia de colisiones de tránsito en zonas urbanas. Un enfoque bayesiano empírico. Aplicación a Cartagena de Indias", Tesis de maestría, Fundación Universidad del Norte, Atlántico, Barranquilla, Colombia, 2010. [ Links ]
[38] K. Choocharukul, "Accident prediction models for minor arterials in Thailand", in Proc. of the12th National Convention on Civil Engineering, 2007. [ Links ]
[39] D. Navon, "The paradox of driving speed. Two adverse effects on highway accident rate", Accident Analysis and Prevention, vol. 35, no. 3, pp. 361-367, 2003. [ Links ]