1. Introduction
Anthropogenic activities generate atmospheric emissions that increase as the population grows, these cause notable effects attributed especially to CO2, which is derived from the burning of fossil fuels. Among the most relevant effects are global warming, climate change and damage to the biosphere, which are key issues today, since it causes multiple negative impacts according to Claesson & Nycander 1, Lefevre 2, and Koçak, Ulucak, & Ulucak 3.
Among the characteristics that make CO2 creditor the most representative of the greenhouse gases that accelerate global warming, are long periods of permanence in the atmosphere for up to centuries, where about 46% of total emissions remain in the atmosphere, the high capacity to retain heat, in short, the large amounts emitted by human activities on a daily basis 4,5. Thus, it has been shown that the adverse effects of this compound come to suffer from marine ecosystems 6,7.
In effect, the oceans receive high amounts of CO2, of approximately 30% of the total emitted 4, this is possible due to the relationship between the water surface and the atmosphere where there is an exchange of gases 8. Therefore, consequences are generated in the marine environment due to the acidification of the oceans, particularly in coral reefs, which are vulnerable to these changes, which causes the availability of the necessary minerals that make up their skeletons (aragonite) and that carbonate ions are decreased, which produces a reduction in their calcification, which can cause the net loss of these strategic ecosystems, along with their ecosystem services 9,10.
In this sense, in Colombia there is little scientific knowledge about the effects of climate change on its marine and coastal areas, which makes it impossible to structure national policies for the conservation of marine ecosystems, so it is necessary to establish indices that allow to know the state of the country's maritime sector.
Therefore, this article aims to establish a CO2 flux index for the coral complex system of San Andrés Islas using inference systems through the GeoFIS tool, that is, this place is part of a conservation area, the largest declared Seafluxer Biosphere Reserve, where the diversity of marine ecosystems stands out, among the most important being coral reefs 11.
2. Methodology
2.1. Place of study
San Andrés islands is an archipelago that belongs to Colombia located in the southwest of the Caribbean (12-16 degrees latitude N. and 78-82 degrees latitude W.), declared by UNESCO as a Biosphere Reserve in 2001 under the administration of the Corporation for the Sustainable Development of San Andrés, Vieja Providencia and Santa Catalina, CORALINA 12. In particular, the island of San Andrés is the largest with approximately 25 km2 of emerging area, located about 290 km off the coast of Nicaragua (Central America) and 480 km off the coast of Cartagena (Colombia) 13, is characterized by being one of the main tourist attractions in the country, which has a wide diversity of marine fish and important ecosystems such as coral reefs, prairie beds, sandy coasts and mangroves 12-14. The study area is shown in Figure 1.
2.2. Estimation of the CO2 flux index
The determination of the CO2 flux index requires defining the dependency functions that describe the behavior of each of the input variables in the fuzzy model, which can be obtained through specialized scientific literature, which helps to establish each variable, the function of relevance and function parameters.
Once the database is normalized, two files are created in comma-separated values (.csv) format for the aggregate variables of the transfer constant k or also CO2 flow constant (made up of wind speed and sea surface temperature) and the partial pressure differential (ΔP) of CO2 both in seawater and in the atmosphere.
For which, a partition is generated at 75% of the data for k and ΔP, a FIS without rules is generated and finally the performance of each sample is estimated. In this way determine the FIS rules to proceed to induce the rules through supervised learning type OLS. This procedure was repeated with each of the learning files, where it established the performance of each one by comparing the indices pi, RMSE, MAE, coverage, and maximum error.
Subsequently, the normalized database is loaded again to GeoFIS, with the corresponding coordinate system for Colombia (WGS 84 zone 18N), and then proceed to perform a data merge, where the membership functions that describe the behavior are defined. of each of the input variables in the fuzzy model.
On the other hand, for the determination of the CO2 flux index, the Weighted Arithmetic Mean (WAM) operator is used, by means of the induced weights of the autonomous learning of the database, in this way to obtain the fusion of data for the calculation of the CO2 flux indicator.
2.3. Performance criteria considered
Coverage ratio: Data rows are labeled as active or inactive for a given rule base. A row is active if its maximum match degree in all rules is greater than a defined threshold.
Following this definition, a coverage index value is calculated, which is a quality index complementary to classical precision.
Performance indices: Next, the error rate only considers the number of active elements defined above. For regression cases, the performance index available in FisPro (Fuzzy Inference System Professional) is based on the root mean square error (RMSE), Eq. 1:
Where
Linguistic Variable and Fuzzy Partitioning: Variable partitioning is the first step in FIS design. The conditions necessary for fuzzy partitions to be interpretable. The main points are distinction, a justifiable number of fuzzy sets, normalization, overlap and sufficient coverage: each data point, x, should belong significantly (l (x)> at least one fuzzy set is called the coverage level).
In this way, the linguistic rules can be interpreted as overlaps between functions, as shown in Figure 2:
Induction of rules: FIS learning involves adjusting many parameters, so in this case it is feasible to consider only single output systems in the present study. Whatever its complexity and origin (fuzzy grouping, statistical methods, machine learning or ad hoc data-based designed specifically for fast learning of fuzzy rules). Once the input fuzzy partitions have been defined, we proceed to generate the complete set of rules corresponding to all combinations of fuzzy sets. For this case, due to the nature of the phenomenon and the available data, the Orthogonal Least Squares (OLS) method was used, which is a prototype-based learning method.
2.4. Data analysis
From three CO2 flux scenarios, developed from a comparison of the fuzzy indicator with the linear method of estimating CO2 flux, the results of each indicator can be placed within a range that allows us to know the effect of the flux, as shown in Table 1.
2.5. Limiting factors
The principal component analysis is carried out with the purpose of establishing the variables or limiting factors of the CO2 flux phenomenon. This procedure is executed through the Primer V6 software, available at https://www.primer-e.com/, which produces a graph showing the components that determine the behavior of the CO2 flux.
3. Results y discussion
The relevance functions of the input variables in the fuzzy model and their characteristics are shown in Table 2.
Table 2 Membership functions for calculating the CO2 flux index
Input variable | Membership function | Function parameters | References |
---|---|---|---|
Wind speed |
![]() |
Semi trapezoidal sup. Bottom help: 2.5 lower Kernel:14.3 | 23-25 |
Sea Surface Temperature |
![]() |
Semi trapezoidal sup. Bottom help: 24 lower Kernel:29 | 24,26 |
CO2 Partial Pressure |
![]() |
Semi trapezoidal sup. Bottom help: 141.38 lower Kernel: 129.56 | 17,19 |
CO2 Partial Pressure |
![]() |
Semi trapezoidal sup. Bottom help: 343.26 lower Kernel:331.64 | 18,25,27 |
Table 2 summarizes the conceptual considerations regarding the relevance function and function parameters of each input variable included in the calculation of CO2 flux.
Considering the information shown in Tables 3 and 4 about the performance and coverage indices for the data of k and ΔP, it was determined that the FIS (Fuzzy Inference System) rules to use correspond to sample 8 (simple 8) and sample 9 (simple 9) for each of these aggregate variables.
Table 3 Performance and coverage indices for the induction of OLS rules for k
Sample 1 | Sample 2 | Sample 3 | Sample 4 | Sample 5 | Sample 6 | Sample 7 | Sample 8 | Sample 9 | |
---|---|---|---|---|---|---|---|---|---|
Pi | 0.009 | 0.006 | 0.007 | 0.007 | 0.008 | 0.005 | 0.005 | 0.008 | 0.007 |
RMSE | 0.05 | 0.034 | 0.034 | 0.038 | 0.042 | 0.027 | 0.029 | 0.046 | 0.039 |
MAE | 0.036 | 0.027 | 0.029 | 0.031 | 0.035 | 0.02 | 0.023 | 0.038 | 0.031 |
Coverage | 93 | 84 | 81 | 87 | 84 | 84 | 87 | 100 | 90 |
Maximum error | 0.147 | 0.097 | 0.058 | 0.089 | -0.0106 | 0.052 | 0.068 | 0.105 | -0.11 |
Table 4 Performance and coverage indices for the induction of OLS rules for ΔP
Sample 1 | Sample 2 | Sample 3 | Sample 4 | Sample 5 | Sample 6 | Sample 7 | Sample 8 | Sample 9 | |
---|---|---|---|---|---|---|---|---|---|
Pi | 0.006 | 0.004 | 0.002 | 0.003 | 0.004 | 0.003 | 0.004 | 0.003 | 0.004 |
RMSE | 0.031 | 0.022 | 0.011 | 0.019 | 0.019 | 0.019 | 0.024 | 0.014 | 0.02 |
MAE | 0.02387 | 0.017 | 0.009 | 0.015 | 0.016 | 0.015 | 0.02 | 0.012 | 0.015 |
Coverage | 87 | 93 | 90 | 93 | 87 | 90 | 93 | 90 | 90 |
Maximum error | -0.101 | 0.069 | 0.033 | 0.058 | 0.042 | 0.039 | -0.045 | 0.034 | 0.066 |
Thus, the normalized database is loaded with the information again to GeoFIS, for the fusion of the data and subsequent use of the Weighted Arithmetic Mean (WAM) operator, obtaining the data of the fusion of data for the calculation of the CO2 flux indicator shown in Table 5.
Table 5 Data fusion for the CO2 flux indicator
Station | Flux _CO2 Index | K estimated | Temperature | Wind | Delta_P | PP_CO2_Water | PPT_CO2_Air |
---|---|---|---|---|---|---|---|
C1 | 0.49 | 0.4935 | 0.5400 | 0.8571 | 0.4898 | 0.4248 | 0.7304 |
C1a | 0.47 | 0.4319 | 0.4000 | 0.8357 | 0.4983 | 0.5160 | 0.7168 |
C1b | 0.50 | 0.5205 | 0.5400 | 0.8786 | 0.4851 | 0.4248 | 0.7036 |
C2 | 0.45 | 0.4638 | 0.6000 | 0.8214 | 0.4373 | 0.3876 | 0.4948 |
C2a | 0.44 | 0.4581 | 0.7000 | 0.8214 | 0.4250 | 0.3272 | 0.4948 |
C2b | 0.44 | 0.4581 | 0.7000 | 0.8214 | 0.4250 | 0.3272 | 0.4948 |
C3 | 0.40 | 0.3891 | 0.7800 | 0.7857 | 0.4155 | 0.2808 | 0.4948 |
C3 a | 0.40 | 0.3777 | 0.8000 | 0.7857 | 0.4155 | 0.2808 | 0.4948 |
C3 b | 0.43 | 0.4486 | 0.7000 | 0.7857 | 0.4155 | 0.2808 | 0.4948 |
Cove Seaside | 0.42 | 0.3691 | 0.7400 | 0.0714 | 0.4607 | 0.3040 | 0.6904 |
Cove Seaside a | 0.43 | 0.3840 | 0.8000 | 0.0714 | 0.4550 | 0.2696 | 0.6768 |
Cove Side b | 0.42 | 0.3342 | 0.6000 | 0.0714 | 0.4765 | 0.3876 | 0.7036 |
Evans Point | 0.43 | 0.3935 | 0.8800 | 0.1071 | 0.4515 | 0.2252 | 0.6904 |
Evans Point a | 0.45 | 0.4521 | 0.9600 | 0.1071 | 0.4445 | 0.1824 | 0.6768 |
Evans Point b | 0.43 | 0.4013 | 0.9000 | 0.1071 | 0.4519 | 0.2144 | 0.7036 |
Evans Point c | 0.32 | 0.3630 | 0.8000 | 0.1786 | 0.2979 | 0.2696 | 0.2992 |
Genie Bay | 0.32 | 0.3518 | 0.7400 | 0.1786 | 0.2930 | 0.3040 | 0.2860 |
Genie Bay a | 0.32 | 0.3630 | 0.8000 | 0.1786 | 0.2979 | 0.2696 | 0.2992 |
Genie Bay b | 0.39 | 0.2897 | 0.5000 | 0.3557 | 0.4612 | 0.4504 | 0.5340 |
German Point | 0.31 | 0.3402 | 0.6600 | 0.1429 | 0.2975 | 0.3512 | 0.2992 |
German Point a | 0.33 | 0.3687 | 0.8000 | 0.1429 | 0.3034 | 0.2696 | 0.3120 |
German point b | 0.30 | 0.3123 | 0.6000 | 0.1429 | 0.2936 | 0.3876 | 0.2992 |
Low Bight | 0.34 | 0.1783 | 0.4600 | 0.2143 | 0.4438 | 0.4764 | 0.4816 |
Low Bight a | 0.37 | 0.2805 | 0.6000 | 0.2143 | 0.4305 | 0.3876 | 0.4868 |
Low Bight b | 0.33 | 0.1783 | 0.4600 | 0.2143 | 0.4348 | 0.4764 | 0.4712 |
Low Bight c | 0.33 | 0.1337 | 0.2000 | 0.2143 | 0.4653 | 0.6552 | 0.4816 |
Old Point | 0.35 | 0.1783 | 0.3000 | 0.2143 | 0.4622 | 0.5840 | 0.4868 |
Old Point a | 0.33 | 0.1337 | 0.2000 | 0.2143 | 0.4561 | 0.6552 | 0.4712 |
Old Point b | 0.26 | 0.1373 | 0.2800 | 0.4286 | 0.3483 | 0.5984 | 0.3380 |
Pleasant Point | 0.24 | 0.0874 | 0.3600 | 0.4286 | 0.3351 | 0.5428 | 0.3432 |
Pleasant Point a | 0.26 | 0.1373 | 0.2800 | 0.4286 | 0.3442 | 0.5980 | 0.3328 |
Pleasant Point b | 0.28 | 0.2646 | 0.6000 | 0.2500 | 0.2936 | 0.3876 | 0.2992 |
San Andres Bay | 0.27 | 0.2295 | 0.5600 | 0.2500 | 0.3009 | 0.4124 | 0.3120 |
San Andres Bay a | 0.29 | 0.3043 | 0.6600 | 0.2500 | 0.2880 | 0.3512 | 0.2860 |
San Andres Bay b | 0.27 | 0.2170 | 0.6600 | 0.4643 | 0.2975 | 0.3512 | 0.2992 |
Sound Bay | 0.22 | 0.1540 | 0.6000 | 0.4643 | 0.2681 | 0.3876 | 0.2656 |
Sound Bay a | 0.24 | 0.1540 | 0.6000 | 0.4643 | 0.2976 | 0.3876 | 0.3044 |
Sound Bay b | 0.22 | 0.0910 | 0.5400 | 0.4643 | 0.2996 | 0.4248 | 0.3120 |
South End | 0.40 | 0.3342 | 0.6000 | 0.0714 | 0.4470 | 0.3876 | 0.5212 |
South End a | 0.40 | 0.3254 | 0.5600 | 0.0571 | 0.4543 | 0.4124 | 0.5340 |
South End b | 0.44 | 0.4320 | 0.8600 | 0.5000 | 0.4527 | 0.2360 | 0.6904 |
Sukey Bay | 0.44 | 0.4320 | 0.8600 | 0.5000 | 0.4527 | 0.2360 | 0.6904 |
Sukey Bay a | 0.42 | 0.3600 | 0.8000 | 0.5000 | 0.4581 | 0.2696 | 0.7036 |
Sukey Bay b | 0.40 | 0.3120 | 0.7600 | 0.5000 | 0.4584 | 0.2924 | 0.6824 |
From the analysis presented as a similarity matrix in Table 6, the principal components analysis is performed, the result of which is shown in Figure 3.
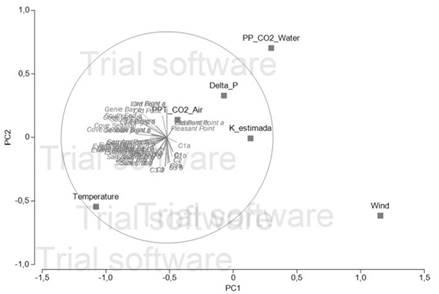
Figure 3 Principal component analysis for the CO2 flux indicator on San Andrés Island. Source from: Author, 2020
According to the vector distribution of the variables that make up the CO2 flux phenomenon in the different study areas on the San Andrés Island, it can be inferred that the CO2 partial pressure and the wind speed do not condition the flux behavior in the marine environment, at least for the areas and at the time of study.
Table 6 Triangular matrix of similarity according to the Euclidean distance.
Samples | ||||||
---|---|---|---|---|---|---|
K_estimated | Temperature | Wind | Delta_P | PP_CO2_Water | PPT_CO2_Air | |
K_estimada | ||||||
Temperature | 1.6515 | |||||
Wind | 1.5543 | 2.2521 | ||||
Delta_P | 0.88599 | 1.3934 | 1.5708 | |||
PP_CO2_Water | 1.3359 | 1.8632 | 1.6119 | 0.74628 | ||
PPT_CO2_Air | 1.1797 | 1.1839 | 1.8198 | 0.58104 | 1.2248 |
On the other hand, the CO2 transfer coefficient, closely related to the sea surface temperature and the chemical nature of this gas, have a significant influence on the incorporation of this gas into the marine environment, these two variables being the flux limiting factors. CO2 for this case study.
4. Conclusions
The application of fuzzy methods to determine the acidification of coral ecosystems allows establishing an approximation of the effects that the gradual incorporation of CO2 would have into the marine environment, in addition to offering excellent advantages in terms of its determination based on reliable satellite information and easy access.