1. Introduction
The electricity markets have been restructured to achieve efficiency and competitiveness. In the timespan 1990-2008, several countries made a liberalization process to have a competitive, transparent, and liable market 1,2. These structural changes determined a trade that, in contrast to financial markets, is characterized by power storage limits, the low correlation between short-term and long-term prices, and a seasonal dynamic complex 3.
On the other hand, the competitiveness introduction in the generation sector originated a spot market, wherein the electricity price is defined through an auction between suppliers to meet the hourly demand of the next day. Also, the difficulty in storing electricity causes the spot price to present characteristics such as high frequency, mean and variance are not constant, seasonal dynamics, high variability, and sensitivity to demand, besides changes in the generation sources 3-7. Therefore, modeling and forecasting electricity price is defiance for academics and electricity market agents.
For a better price dynamic understanding, it is necessary to observe the fundamentals or the factors that explain its characteristics in the deregulated electricity markets. Therefore, a bibliometric analysis is proposed as a systematic tool for monitoring the research efforts on-field, allowing an overview of the electricity price determinants in the timespan January 1979 - April 2021. In general, variables that determine electricity prices in competitive markets were observed, such as economic conditions, weather and temperature, power system operations, market structures, agent strategies, and historical factors.
This document is divided into five sections after the introduction: in section 2, the methodology for bibliometric analysis is defined. In section 3, the results are described; in section 4, the determinants of the electricity price in competitive markets are presented through bibliometric analysis results and the case of the Latin American electricity markets is included. In section 5, the summary and conclusions are presented.
2. Methodology
The research was made with the Scopus database and included: (i) citation information, (ii) bibliographical information, (iii) abstract and keyboard, and (iv) other information. Further, the search equation (Eq. 1) includes the terms in the title of the article, abstract, and keywords and was limit to articles. The search equation is described below:
The software VOSviewer 1.6.14 was used for data analysis and visualization 8.
3. Results
636 documents published in the study period were observed. Figure 1 shows the number of articles per year. An increase can be noticed since 2003 due to the deregulation and liberalization of the electricity markets during 1990-2008. These structural changes introduced competition, transparency, and efficiency in power markets 1,9. Therefore, the spot electricity price or market clearing price is defined as the intersection of supply and demand curves by aggregated bids for a particular hour. That is because the spot electricity contracts are auctioned the day before and once per day. Besides, these contracts are hourly agreements with physical delivery 5.
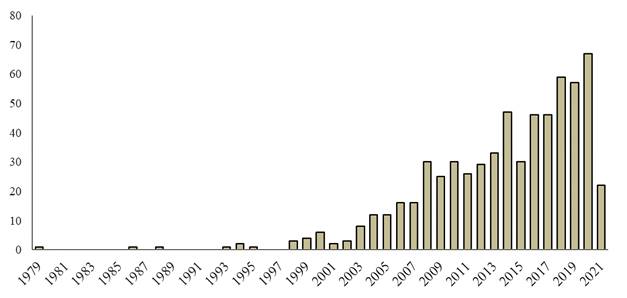
Source: Scopus database
Figure 1 Evolution of the number of documents related to the electricity spot price between 1979 and 2021.
However, the spot price structure caused a set of questions about its dynamics and volatility. Therefore, it was observed that the average of documents per year. Before 2003, was one document, but between 2003-2020, the average per year was above 32 manuscripts. Likewise, 22 manuscripts were identified until April 2021.
In the following subsections, the results are described according to the countries and institutions related to modeling the spot price dynamics and its determinants; the subject areas and methodology approach applied to analysis and forecasting of spot prices; and the leading documents and authors focusing on this topic.
3.1. Leading countries and institutions related to the electricity spot price study
Table 1 shows the number of documents by country. The top-five of leading countries that have studied the electricity price dynamics are China with 122 manuscripts, the United States with 98, Germany with 69, the United Kingdom with 57, and Spain with 46. Besides, it was considered ranking the research impact through the number of citations, and the ratio between the number of citations and the number of publications (CP ratio). The United States and the United Kingdom were always within the top five. Despite China was in second place in citations, this country occupied the last place in the CP ratio, where The United States, Canada, and Iran were the most important countries.
Table 1 Top-10 leading countries of studies related to electricity price fundamentals.
Country | Publications | Citations | Citations/Publications |
---|---|---|---|
China | 122 | 1,983 | 16.3 |
United States | 98 | 3,079 | 31.4 |
Germany | 69 | 1,144 | 16.6 |
United Kingdom | 57 | 1,576 | 27.6 |
Spain | 46 | 1,034 | 22.5 |
Australia | 30 | 641 | 21.4 |
Canada | 23 | 694 | 30.2 |
Norway | 20 | 414 | 20.7 |
Italy | 20 | 322 | 16.1 |
Iran | 20 | 554 | 27.7 |
Source: Scopus database and VOSviewer analysis.
Furthermore, Figure 2 shows the countries’ collaboration network for studies on electricity price topics (countries contributing a minimum number of five documents and citations), and seven clusters were observed: red, green, blue, yellow, purple, grey, and orange. The United States, Germany, China, the United Kingdom, Spain, Australia, and Norway have the strongest collaboration network. In Latin America, Brazil and Colombia are the countries with more documents and citations. The first one has eight documents and 70 citations, and the second one has six documents and 51 citations, but Colombia presents a high CP ratio. Likewise, it was found that Mexico has three manuscripts and 30 citations, followed by Uruguay, Chile, Argentina, and Guatemala with one document per country.
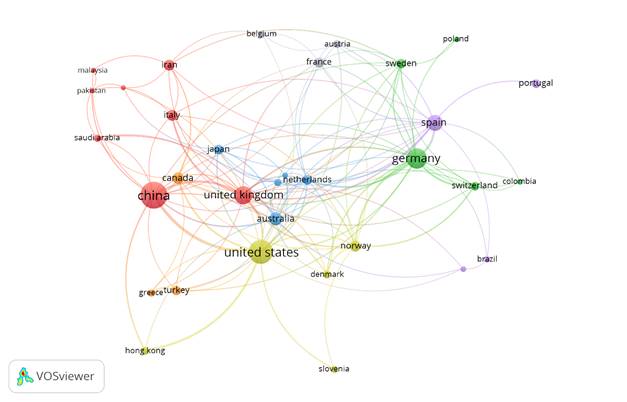
Source: Scopus database and VOSviewe analysis
Figure 2 Visualization of the country collaboration network for studies on electricity price fundamentals.
Otherwise, Figure 3a shows the number of documents by affiliation, and Figure 3b provides the number of manuscripts by funding sponsor. Firstly, the leading universities in this research area are North China Electric Power University with 18 documents, Norges Teknisk-Naturvitenskapelige Universitet with ten manuscripts, Zhejiang University, and London Business School with eight manuscripts, respectively. Secondly, the most relevant sponsors in this topic are the National Natural Science Foundation of China, the European Commission, the Ministry of Education of People's Republic of China, and the UK Research and Innovation. The top-10 of affiliations and funding sponsors were involved in 15% and 16% of all publications, respectively. Besides, a close relation between countries, universities, and funding sponsors was observed through this analysis.
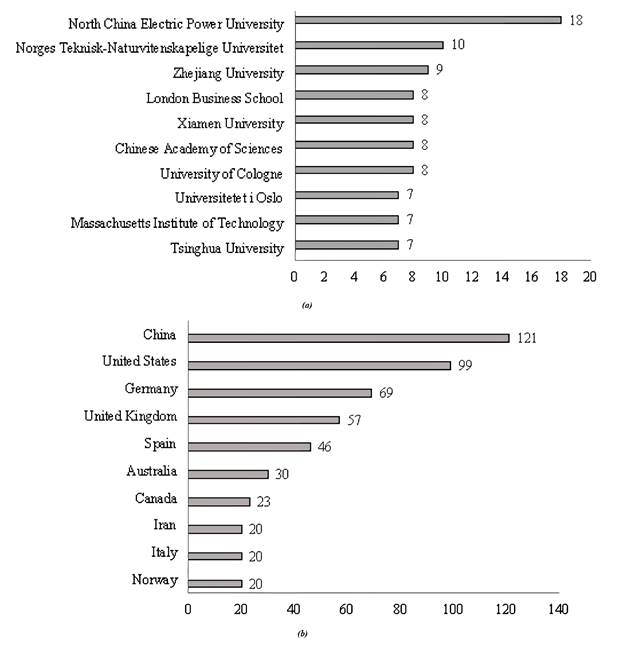
Source: Scopus database
Figure 3 (a) Top-10 affiliations related to electricity price fundamentals. (b) Top-10 funding sponsors related to electricity price fundamentals.
Figure 4 describes the number of documents in percentage by subject area. The most relevant knowledge areas in this topic are Energy (30.9%), Engineering (18.5%), Environmental Science (14.6%), and Economics, Econometrics, and Finance (14.4%).
3.2. Subject areas and methodology approaches
Given the deregulated process in energy markets, the modeling and forecasting of electricity prices require different methods. Therefore, several engineering techniques are applied to explain price dynamics such as simulation algorithms and artificial intelligence models. By contrast, econometric and statistical approaches as autoregressive or regression models are other methodologies used. According to 10, techniques used in the literature can be classified into three groups: simulation models, game theory models, and times series analysis, as described below in Table 2. The time series models present more extensive applicability through stochastic models, causal models, or artificial intelligence models such as neural networks, machine learning, or data-mining models.
Table 2 Methods applied for the electricity spot price modeling.
Group | Model | References |
---|---|---|
Simulation models | Numerical simulation | 11,12 |
Monte Carlo Method | 13-15 | |
Computer simulation | 12,16,17 | |
Optimization algorithm | 18 | |
Counterfactual simulation | 19 | |
Time series models | Neural network and machine learning | 20-25 |
ARMA-GARCH | 26-29 | |
Regression models | 7,30-34 | |
Game theory models | Cooperative and non-cooperative game | 35,36 |
Nash-Stackelberg game | 37 | |
Cournot model | 38,39 | |
Option-game-based | 40 | |
Linear supply function equilibrium model | 41 |
Source: Scopus database
3.3. Leading authors and documents
Table 3 shows the most relevant authors with their affiliation and the number of documents in the period of study. The top five leading authors of electricity price fundamentals are Zarnikau with six documents and Woo, Reneses, Bunn, and Benth with five manuscripts each of them. These findings are coincident with the information reported in Table 1 and Figure 2, i.e., the most productive authors and the countries where their institutions are located.
Table 3 Top-10 leading authors of studies related to electricity price fundamentals.
N° | Authors | Affiliation | Documents |
---|---|---|---|
1 | Zarnikau, Jay W. | The University of Texas at Austin, Austin, United States | 6 |
2 | Woo, Chikeung. | The Education University of Hong Kong, Hong Kong, China | 5 |
3 | Reneses, Javier | Instituto de Investigación Tecnológica, ICAI, Universidad Pontificia Comillas, Madrid, Spain | 5 |
4 | Bunn, D.W. | London Business School, London, United Kingdom | 5 |
5 | Benth, F.E. | Universitetet I Oslo, Oslo, Norway | 5 |
6 | Lin, B. | Xiamen University, Xiamen, China | 4 |
7 | Mandal, P. | The University of Texas at El Paso, El Paso, United States | 4 |
8 | Gubina, A.F | Univerza v Ljubljani, Ljubljana, Slovenia | 4 |
9 | García-Martos, C. | Universidad Politécnica de Madrid, Madrid, Spain | 4 |
10 | Bello, A. | Instituto de Investigación Tecnológica, ICAI, Universidad Pontificia Comillas, Madrid, Spain | 4 |
Source: Scopus database
By contrast, the authors’ keywords, electricity markets, electricity prices, electricity price forecasting, renewable energy, and electricity, had 127, 126, 44, 37, and 36 occurrences, respectively. The top 10 author keywords with their number of occurrences are shown in Table 4. Likewise, Figure 5 shows the research topic network visualization of publications related to electricity price fundamentals, and six clusters were observed (the minimum number of occurrences of a keyword is five). The primary node is the electricity market and has links with electricity prices, empirical methods (quantile regression, neural network, or forecasting), uncertainty, risk, and market characteristics (deregulation, electricity generation, energy efficiency, energy policy, or renewable energy).
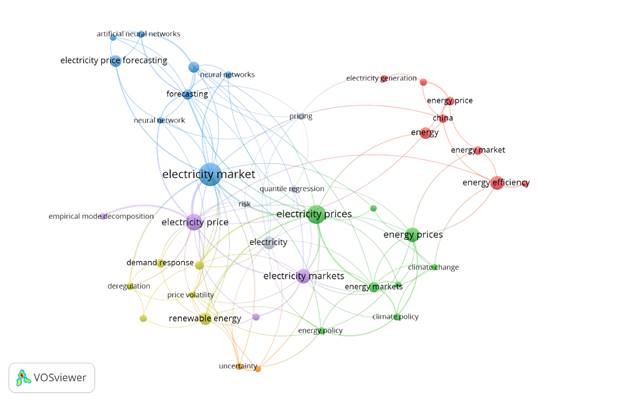
Source: Scopus database and VOSviewer analysis
Figure 5 Research topic map for publications related to electricity price fundamentals.
Table 4 Top-10 author keywords of electricity price fundamentals studies.
N° | Keywords | Occurrences |
---|---|---|
1 | Electricity markets | 127 |
2 | Electricity prices | 126 |
3 | Electricity price forecasting | 44 |
4 | Renewable energy | 37 |
5 | Electricity | 36 |
6 | Energy efficiency | 27 |
7 | Deregulation | 14 |
8 | Demand response | 10 |
9 | China | 9 |
10 | Quantile regression | 7 |
Source: Scopus database and VOSviewer analysis.
Furthermore, Table 5 shows the most cited research related to electricity price fundamentals based on Scopus reports. The most cited paper analyzed the market liberalization effects on the elasticity of the demand for electricity 42. After electricity market liberalization, the consumers are more exposed to the electricity prices volatility and may decide to modify their demand profile to reduce their costs. Therefore, the authors considered a pool electricity market with scheduling generation and setting the electricity prices, using 26 generator systems. The results showed that the elasticity of the demand is an important factor to be considered when the price is setting in a centralized competitive market. In the next section, the different fundamentals of electricity prices found in the bibliometric analysis are discussed.
Table 5 Most cited documents in the global research related to electricity price fundamentals.
Title | Authors | Year | Journal | Citations |
---|---|---|---|---|
Factoring the elasticity of demand in electricity prices | Kirschen, D. S., Strbac, G., Cumperayot, P., and de Paiva Mendes, D. | 2000 | IEEE Transactions on Power Systems, 15(2), pp. 612-617 | 476 |
Electricity price forecasting in deregulated markets: A review and evaluation | Aggarwal, S. K., Saini, L. M., and Kumar, A. | 2009 | International Journal of Electrical Power and Energy Systems, 31(1), pp. 13-22 | 321 |
RBF neural network and ANFIS-based short-term load forecasting approach in real-time price environment | Yun, Z., Quan, Z., Caixin, S., Shaolan, L., Yuming, L., and Yang, S. | 2008 | IEEE Transactions on Power Systems, 23(3), 853-858 | 293 |
Energy Price Forecasting in the Ontario Competitive Power System Market | Rodriguez, C. P., and Anders, G. J. | 2004 | IEEE Transactions of Power Systems, 19(1), pp. 366-374 | 257 |
A quantitative analysis of pricing behavior in California’s wholesale electricity market during summer 2000 | Joskow, P. L., and Kohn, E. | 2002 | Energy Journal, 23(4), pp. 1-35 | 219 |
Source: Scopus database.
4. Determinants of electricity price in deregulated markets: Discussion
After the liberalization of electricity markets, the price has shown high volatility, mean-reversion, seasonality, extreme spikes, and its dynamic is explained by a set of fundamentals 6. According to 5,10, the most important factors to explain the electricity price dynamics were categorized into five groups: i) market characteristics, ii) fundamental factors, iii) operational factors, iv) strategic factors, and v) historical factors. Table 6 summarizes the principal determinants of electricity spot prices observed in the literature.
Table 6 The principal determinants of the electricity spot price.
Group | Determinant | References |
---|---|---|
Market characteristics | Demand and supply | 6,7,16,19,32,42,42-49 |
Electricity imports-exports | ||
Market power of suppliers | ||
Market-clearing quantity | ||
Energy policy | ||
Fundamental factors | Fuel prices | 7,18,19,30,45,50-53 |
Weather | ||
Hydrological conditions | ||
Price measure frequency | ||
Operational factors | Load rate | 6,7,45,54-60 |
Electricity production | ||
Energy sources: nuclear, hydric, or thermal | ||
Congestion index | ||
Line status and limits | ||
Power transmission costs | ||
Strategic factors | Energy purchasing agreements | 14,45,61-66 |
Bilateral contracts | ||
Bidding strategy | ||
Historical factors | Electricity price lags | 44,49,67 |
Demand and supply lags | ||
Hydric reserve lags |
Source: own elaboration.
In the short term and as of the electricity spot price characteristics, its high variability is related to stational patterns transmit by variables such as hourly demand, load conditions, and generating capacity for a particular day. Besides, the electricity storage limitations cause relevant changes in price distribution by external event shocks as weather fluctuation, production and transmission failures, or shortage in generation sources (e.g., water, gas, or coal).
Likewise, electricity demand captures consumption changes of different economic sectors as industrial, commercial, and residential. Therefore, the dynamic of these activities modifies the price structures due to the demand is inelastic in the short term.
Other factors related to increasing price variances are the market structure, energy policy, agent strategies, and the impossibility of achieving the demand and supply equilibrium in real-time. Therefore, modeling the electricity spot price dynamic represents a challenge to market agents. In the last years, the investigation trend about modeling the electricity spot price has shown a significant effort to applied different forecasting methodologies. Since the introduction of the competitive electricity markets, spot price forecasting has become a relevant decision-making mechanism by many companies.
According to 68,69, the inception of smart grids and renewable integration has increased the uncertainty on some market indicators as the spot price, demand, and supply. For example, renewable energies can reduce price volatility in Hydrothermal power markets 70. Thus, the bibliometric analysis showed that approximately 60% of the papers of the last five years had the price forecast as the main objective 29,66,71-74. In this way, price prediction has become a principal method for planning and operations in energy systems. Besides, the growing popularity of artificial intelligence methods, characterized by fast fitting and low memory usage, allow simple price forecasting 21-23,25,75-78.
4.1. Latin America case
Figure 6 shows the electricity generation by fuel in 2019. There is a high dependence on natural gas and coal for power generation in North America (57%), the Commonwealth of Independent States (CIS) (67%), the Middle East (64%), the Asian Pacific (70%), and Africa (68%). By contrast, Europe has a balanced energy matrix where each source weight, except oil, is between 16%-23%. However, South and Central America gets more than half of its power from hydroelectricity, with a share far higher than any other region. Besides, fuel fossil has a total percentage of 31%. Therefore, in S. & C. America, the generation is based on hydroelectric and thermal power sources.
Despite countries such as Paraguay, Uruguay, and Costa Rica, the market is integrated and depends on the state, liberalization process in Latin America showed that energy policy, fuel prices, and hydrological conditions are the fundamentals that explain electricity price fluctuations 80,81. In Central America, 31 described that electricity price fluctuations are related to hydrology changes and fossil fuel price variations in Guatemala, El Salvador, and Panama. Similarly, in Mexico, the prices are correlated with fuels because close to 46% of the energy market depends on fossil sources 82. Also, the price increase depends on growing congestion in the national electricity transmission network 83.
In Chile, the empirical evidence shows that price dynamics depend on fuel prices and hydrology 84-86. Besides, 87 observed that spot prices are related to uncertainty and investment decisions making. Thus, the fundamentals of prices in the long term were the structure of the transmission system, the hydrology, and contract prices. Meanwhile, in Brazil, the spot price variations are linked to historical factors, hydrological conditions, generation sources, and energy demand 88-91. However, 92 described that electricity prices were skyrocketing during 2011-2015 not only to unfavorable hydrology but also to problems in the planning and execution of the expansion of power generation and transmission, and difficulties with the NEWAVE dispatch software used by the government.
Colombia has many studies about price dynamics since its energy market is based on hydrothermal power generation. Hydropower represents close to 70% of energy production and the rest depends on thermal power for periods of high demand or temperature shocks 70. The studies showed that variables such as electricity demand and supply, reservoir levels, weather, fuel prices, power transmission failures, energy policy, bilateral contracts, and agent strategies are fundamentals to determine spot price structure 4,93-97.
5. Conclusions
A total of 636 articles related to electricity price fundamentals were published in timespan January 1979 - April 2021. A significant increase in the number of documents after 2000 was observed due to electricity market liberalizations during 1990-2008. Furthermore, countries such as the United States and the United Kingdom are the research leaders. However, the United States, Canada, and Iran had a high impact on their CP ratio. Also, the main investigation areas related to the fundamentals of electricity prices were Energy, Engineering, Environmental Science, and Economics, Econometrics, and Finance.
On the other hand, the most cited papers showed that the factors are categorized into five groups: i) market characteristics, ii) fundamental factors, iii) operational factors, iv) strategic factors, and v) historical factors. However, and independent of market structure, price volatility always is given by factors such as economic conditions, weather, operation of power systems, and customer demands.
Besides, the investigation trend observed in this study is related to forecasting the electricity spot price since the inception of competitive power markets. The academics and market agents have understood that electricity spot price and load forecasting are relevant for energy systems and operations than ever before. Therefore, the price fundamentals give a new perspective to understand the price dynamic. Likewise, the growing renewable sources and the inception of smart grids reduce the gap between the technical business and financial business, consequently the uncertainty increase.
Finally, the future research proposal can be divided into two topics: first, apply advanced empirical methods of artificial intelligence and time series analysis to evaluate the fundamentals of the electricity price on these markets. Second, to identify the electricity prices effects on different sectors such as industry or economic activity.