Introduction
Metacognition has been studied from a variety of approaches. Studies have addressed, for instance, perceptual and memory tasks and their effect on learning (Rhodes & Castel, 2008) and on the allocation of study time and decision making (Weber, Woodard, & Williamson, 2013). Research is also abundant on cognitive aspects such as perceptual discrimination, eye tracking, and facial recognition (Boldt, Gardelle & Yeung, 2017; Fleming, Massoni, Gajdos, & Vergnaud, 2016; Weber, Woodard, & Williamson, 2013). Further, there are studies that have explored the effect of metacognition on performance in online cognitive tasks (Quiles, Verdoux, & Prouteau, 2014) and on the ecological and intrapersonal sources of metacognitive judgments of self-control around different cognitive tasks (Kleitman & Stankov, 2001). Finally, some studies explore the specific relationship between intellectual style and metacognition. Among them are research studies that have examined the relation between personality and cognition insofar as it is assumed that there are differences between people based on their intellectual styles. Intellectual styles are defined as the ways in which people choose to use their cognitive resources to solve problems and make decisions, which imply differences in the relation between intellectual style and metacognition, especially in relation to metacognitive knowledge (López-Vargas, Ibañez-Ibáñez, & Chiguasuque-Bello, 2014; López-Vargas, Ibáñez-Ibáñez, & Racines-Prada, 2017; Sadler-Smith, 2012; Zhang & Sternberg, 2006; Zohar & Ben-David, 2009). Intellectual style, thus, refers to individuals' preference to process information and to deal with tasks. It is a generic term used to refer to cognitive style, conceptual tempo, decision-making and problem-solving style, learning style, perceptual style, and thinking style, among others (López-Vargas et al., 2014; López-Vargas et al., 2017; Zhang & Sternberg, 2006).
There are three main approaches to the study of intellectual styles recognized in the literature (López-Vargas et al., 2014; López-Vargas et al., 2017; Zhang & Sternberg, 2006). The first is research focused on cognition, which includes studies on field dependence and independence (Witkin et al., 1962) and Kagan's (1966) reflexivity-impulsivity model. A second line of work is considered personality-centered and has been derived from Jung's (1923) theories of personality types, Hollan's (1973) vocational types, and the style model proposed by Gregorc (1979). Finally, a third activity-centered approach focuses on which styles are mediators of activities that arise from both cognition and personality, including the works on learning styles (Renzulli and Smith, 1978) and the studies on deep and superficial learning (Biggs, 1978; Entwistle, 1981; Marton, 1976; Schmeck, 1983). Thus, the primary purpose of the present research was to explore the relation between intellectual styles and metacognitive monitoring accuracy to better inform the dearth of research on these topics.
In the present research, the concept of cognitive style was defined as Field Dependence-Independence (FDI) (Witkin, Moore, Goodenough, and Cox, 1977). This theoretical framework emphasizes the way in which students manifest different ways of processing and organizing information based on individual differences (Chen, Liou, & Chen, 2018; Jia, Zhang, & Li, 2014; López-Vargas et al., 2014; López-Vargas et al., 2017; McKeachie & Svinicki, 2013; Rittschof, 2008; Slavin, 2000; Sternberg & Williams, 2002). More specifically, cognitive style is a habitual way of processing information, and it is a consistent and stable characteristic in the individual that is evident in the ways of functioning in any cognitive task (Hederich-Martínez & Camargo-Uribe, 2016). Curry (1983) proposed a classic three-layer "onion" metaphor, which posits that the styles located in the center of the onion, when representing features of the individual's cognitive personality, can be relatively stable and not so malleable. The innermost layer of the onion contains cognitive styles (i.e., cognitive personality traits) such as the style of field dependence and independence (Witkin, et al., 1962) and the style of reflexivity vs. impulsivity (Kagan, 1966). The intermediate layer contains the typologies of styles that evaluate information processing. These include styles that analyze the individual differences in the different subcomponents of an information processing model (e.g., perception, memory and thought), which assumes that styles are subordinate to the analytical-holistic dimension. In the analytical pole there are styles such as field independence, sharpening, convergence and serial information processing, while at the holistic pole, there are styles such as field dependency, leveling, divergence, and holistic information processing (Miller, 1987). The outermost layer of the onion contains learning styles that address people's preferences in relation to teaching and instruction (Honey & Munford, 1992).
Regarding the relation between cognitive style and metacognition, research reports that knowledge of aspects related to students' cognitive style, in relation to the way they process information or their preferences when using their own cognitive resources in their learning, constitutes an important contribution to their own metacognitive knowledge. In effect, this knowledge allows individuals to think about their own thinking and learn how to be more effective regarding the learning process, which should subsequently allow for the advancement of this same metacognitive knowledge (López-Vargas et al., 2014; López-Vargas et al., 2017; Zhang, & Sternberg, 2005; Zhang, & Sternberg, 2009; Zohar & Ben-David, 2009).
Research employing undergraduate students, for instance, explored the relationship between individual differences in the evaluation of cognitive style and different measures related to metacognitive judgments (e.g., decision time, precision, and confidence) during the performance of tasks related to three domains (vocabulary task, general knowledge task, and perceptual comparison task). Results revealed stable differences in performance in the three domains regarding measures of decision time, precision, and confidence responses (Blais, Thompson, & Baranski, 2005). Research with psychology students investigated which personality and cognitive style factors were related to the level of confidence expressed by the students, after making first and second order judgments regarding memory semantics in a general knowledge question format. Results indicated that personality and cognitive style factors were only weakly linked to the formulation of first and second order confidence judgments (Buratti, Allwood, & Kleitman, 2013), although theoretically it is plausible that people with a "grand opening" cognitive style might be more likely to remember the test item (i.e., having better knowledge or memory). More recent research, however, converges on the conclusion that cognitive style may influence the way individuals develop judgments of performance, and hence, how they approach metacognitive monitoring accuracy (Jia et al., 2014; López-Vargas et al., 2014; López-Vargas et al., 2017). However, more work is needed to provide additional empirical support for the effect of cognitive styles on metacognitive skills.
The Present Study
The present study sought to explore the relations between cognitive style in the field dependence-independence (FDI) modality and metacognitive monitoring accuracy. The following research questions guided the conduct of the study.
What is the effect of cognitive style (field dependent, field independent) on participants' metacognitive monitoring accuracy for three types of metacognitive judgments (prediction, concurrent, and postdiction)?
How do these judgments differ in relation to three different types of metacognitive questions involving: 1) students' knowledge regarding the cognitive task to be performed (a masked figures test); 2) students' knowledge of their own metacognitive resources (self-knowledge as a learner); and 3) the estimation of the expected score in completing the task of cognitive restructuring for each of the three evaluation moments (before, during, and after) of completing the indicated task?
Due to the lack of research on the relation between metacognitive monitoring and cognitive styles, the present study does not include hypotheses.
Method
Participants, Sampling, and Research Design
This study employed a non-experimental descriptive design with a convenience sampling approach. Information is available on a convenience sample of 57 undergraduate students in psychology from a private university in Colombia, of whom 43 (75.4%) are female and 14 (24.6%) male. The average age is M = 19.91 (SD = 1.61), with a minimum of 18 and a maximum of 24 years. Participants who were 18 years of age or older and who were university students were eligible to participate in the study. There were no exclusion criteria.
Instruments
Evaluation of cognitive style in the field dependence-independence dimension polarity (FDI). For the evaluation of cognitive style, a computerized version of the group form of the masked figures test (GEFT) developed by the Cognitive Styles Research Group housed in the National Pedagogical University of Colombia and available online through the following link was used: http://www.estiloscognitivos.com/aulavirtual/pruebas/eftf/EFT.html
This version is a true copy of the group version of the original Masked Figures Test (GEFT) (Witkin et al., 1971). The test contains a total of 25 items divided into three sections. The final score is the sum of the correctly completed items and varies between 0 and 18. For the classification in the various cognitive styles, it was assumed that people who achieved between 0 and 9 correctly completed figures are considered "field dependent (FD)", people with scores between 10 and 15 are considered "intermediate (I)", and only people with scores between 16 and 18 points are considered as "field independent (FI)". The version of GEFT employed in the present study was validated for use in Colombian university students by López, Hederich, and Camargo (2012).
The internal consistency coefficients on the 15 items, from which the score is obtained, show adequate levels of reliability (Cronbach's α = 0.785). A much stricter test than Cronbach's a, which is independent of the number of items, also shows high level of reliability (McDonald's ω = 0.799).
Estimation of the confidence and precision of metacognitive judgments. Data on confidence in performance was collected and used this to calculate calibration scores (i.e., accuracy and bias/error). The test was completed by the student in three different stages of evaluation. At each stage of the test, the student answered three metacognitive questions to score their level of confidence. Thus, the study included the following data.
Calibration accuracy was evaluated using a continuous scale. Absolute accuracy is the discrepancy between a metacognitive judgment and performance, and it is obtained by calculating the squared deviation between the confidence estimate and performance on the same scale. Smaller deviations correspond to greater accuracy. First, participants were asked to make feeling-of-knowing (FOKs) judgments, simultaneous judgments about the current task, and retrospective confidence judgments about the test (GEFT), at different moments of the test. These metacognitive judgments were measured on a continuous scale of 0-100 points (confidence from 0% to 100%), which guarantees a ratio scale and not only a matrix of correct and incorrect answers (e.g., Gamma coefficient), which only allows us to rate confidence as low or high.
Performance measures. Performance was measured by the total score obtained by the student in taking the test (GEFT), as expressed in the number of correctly completed figures.
Specifications on monitoring precision. Additionally, a measure of error or bias was calculated, as suggested by research (Keren, 1991; Nietfeld et al., 2006; Swe & Saleh, 2010; Yates, 1990), which consisted of estimating the difference established between the average confidence and the average performance scores in each moment of the test. Positive scores will indicate overconfidence, while negative scores will indicate underconfidence. The further away from "0" the score is, the more biased it will be. The average calibration accuracy was also calculated during the three moments of the test.
Procedures
The ethical guidelines proposed for the studies considered to be of minimum risk with human beings in the country in which data were collected were considered. Participation was voluntary and participants could withdraw from the study at any time without penalty. In relation to the estimation of the level of confidence for each type of metacognitive judgment in the different moments of the task (GEFT) online, students answered eight questions that involved estimating the level of confidence regarding their performance on the task. First, participants answered three metacognitive questions, once they had already reviewed the test instructions and had answered two test exercises on the type of task, to have some initial knowledge about the task requirements. After completing the practice exercises, and before starting the test, participants answered the following three metacognitive questions:
How confident are you that you will recognize the simple figure contained within the complex figure, among the different options given in each of the exercises contained in the test? (Question regarding the type of task).
How confident are you in your own performance if a test similar to the one you are taking today is given to you in the future? (Question about self-knowledge)
Prediction initial expected score (moment 1)
Once these questions were completed, the first section of the test began with its corresponding seven exercises. After completing this first section, participants had to answer a second group of questions related to metacognitive judgments simultaneous to the task. The second group of questions was identical to the first (questions 1 and 2 above); however, the third question (question 6) differing from question 3 above in that it investigated the confidence of the score concurrent to the task. (moment 2) rather than a prediction of future performance.
Finally, participants answered a final group of two questions, corresponding to the formulation of retrospective confidence judgments, in which they had to estimate the level of confidence and the expected postdiction score around the task (moment 3).
Data Analysis
Data were evaluated for univariate normality using skewness and kurtosis values and histograms with normal curve overlay. All variables approximated univariate normality across groups. No cases were classified as outliers through box-and-whisker plots by group, and thus, all 57 cases were retained for analysis. There were no missing data, as all participants completed all data points. Other assumptions such as homogeneity of variance were also met. Therefore, planned analyses proceeded without making any adjustments to the data. The Bonferroni adjustment to statistical significance was employed to control familywise Type I error rate inflation. All data were analyzed via IBM Statistical Package for the Social Sciences (SPSS) 25. A combination of descriptive statistics, bivariate/zero-order correlations, and inferential analyses (t-tests and ANOVA) were conducted to meet the research objectives.
Results
GEFT Test
The GEFT test average score was 9.30 (SD = 3.67). The distribution does not differ significantly from the normal curve (Kolmogorov-Smirnov = .076, p = .200). Interpreting these scores in terms of cognitive style in the FDI dimension, most of the participants are considered FD (31, 54.4%), or in an "I" cognitive style (23, 40 ,4%). Only 3 participants (5.3%) showed a preference for the FI cognitive style.
Descriptive Statistics of the Metacognitive Questions
Table 1 shows means and standard deviations of the eight metacognitive questions presented. As observed, the confidence levels estimated for the task type (TCT), the confidence levels estimated in a later test in relation to what one knows about oneself (SK) and the expected scores (ES) are quite similar and consistent within each moment.
Table 1 Descriptive Statistics of the Metacognitive Monitoring Questions
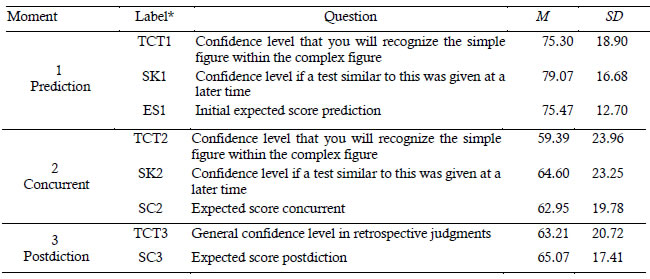
N = 57
* TCT = Estimated confidence levels for each item in relation to the Type of Cognitive Task; SK = Confidence level if a test like this was given at a later time in relation to Self-Knowledge; ES = Expected Score at (moment 1 / prediction score; moment 2 / concurrent score; moment 3 / postdiction score).
Differences between the Means of the Metacognitive Questions by Moment and Type of Question
Table 2 displays the differences in the means (paired samples t-tests) between the different moments controlling for the different types of question. The results show very significant correlations and differences between the first moment (prediction) and the second moment (concurrent), as well as between the first moment and the third moment (postdiction). However, there are no significant differences between the corresponding questions of the second and the third moment.
Table 2 Contrasts between the Different Moments Controlling for Type of Question
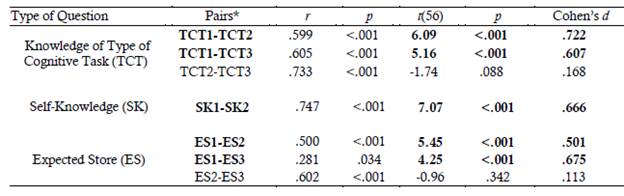
N = 57
*Statistically significant differences are bolded.
Key: TCT = Estimated confidence levels for each item in relation to the Type of Cognitive Task; SK = Confidence level if a test like this was given at a later time in relation to Self-Knowledge; ES = Expected Score at (moment 1 / prediction score; moment 2 / concurrent score; moment 3 / postdiction score).
Thus, results show that students start at their first moment showing very high levels of confidence. For the concurrent moment of application, confidence levels decreased significantly, and they remained without statistically significant differences, in the third moment of measurement. In general, the greatest sources of variation in the means appears between the different moments. Despite this, it is interesting to examine the presence of differences linked to the type of question within each moment. As previously mentioned, in the first and second moments three types of questions were asked while in the third moment two types of questions were asked. The results that compare the different types of question within each moment are presented in Table 3.
Table 3 Differences between Question Type Within Each Moment
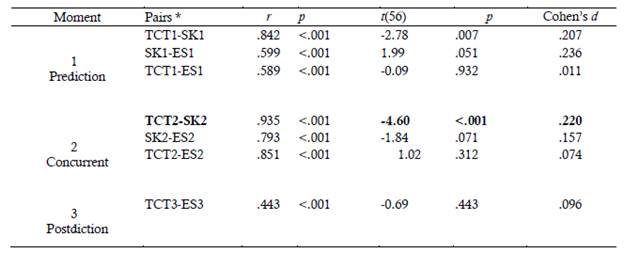
N = 57
*Statistically significant differences are bolded.
Key: TCT = Estimated confidence levels for each item in relation to the Type of Cognitive Task; SK = Confidence level if a test like this was given at a later time in relation to Self-Knowledge; ES = Expected Score at (moment 1 / prediction score; moment 2 / concurrent score; moment 3 / postdiction score).
As observed, the correlations between the different types of questions are very high and significant. Interestingly, results indicate that there are very significant differences within the first and second moments, in the sense that the question asked refers to "if a test similar to this one is given at a later time" presents answers that indicate higher levels of confidence than the questions asked in terms of self-perceived ability in relation to the knowledge individuals have about the type of task and the expected score on the test. Apparently, there is a general tendency to value a hypothetical future experience with the test more positively.
Discussion
In relation to FDI, results revealed that most of the participants in the sample showed a preference towards the FD and "I" cognitive processing style, which seems to make sense initially, given that the sample was made up of psychology students. The results of the present study are consistent with the findings of research in Spanish-speaking samples previously reported. For example, research that aimed to characterize cognitive style (FDI) through the use of the masked figures test found that the most common style of the participants was FD (Díaz, Cuevasanta, Grau & Curione, 2014). Along a similar vein, another study found that 59.6% of psychology students preferred the "I" cognitive style, 30.7% the FD style, and 9.6% the FI style (Díaz - Granados, Kantillo, & Polo, 2000).
In this regard, previous studies described that students with FD cognitive style are people who show a willingness to process information more globally and, in general, tend to be people influenced by the context (López, Hederich & Camargo, 2012; López-Vargas et al. 2017; López-Vargas et al., 2014). Likewise, in their role as students, they are sensitive to the information that comes from the environment, taking it in almost the same way in which it was presented, and they observe the field globally to structure the data of a conceptual and social order. This makes evident a preference in their study towards areas of mastery and professions that involve vocational fields such as the humanities and social sciences (Tinajero, Castelo, Guisande & Paramo, 2011; Jia et al., 2014). Similarly, research considers the "I" students as seeming to have cognitive ability to move in both polarities of processing style, FD and FI, with a form of processing that would go from analytical to global and vice versa, and that they adapt more to the processing style that may be necessary to face the types of academic tasks that they are going to perform (López-Vargas et al. 2017; López-Vargas et al., 2014).
Results also demonstrated that participants started with a relatively high degree of confidence in their performance with prediction judgments, but subsequently reduced their confidence with each successive judgment, so that by the postdiction (final) judgment, the confidence of participants was more closely aligned with their actual performance. These findings are consistent with research studies on the accuracy of metacognitive monitoring using English-speaking samples that conclude that postdiction confidence in performance judgments (i.e., those that occur after the participant has been exposed to the reference task) are much more precise in relation to actual performance than the prediction judgments (i.e., those that occur before the participant has seen the reference task; Gutierrez et al., 2016; Hadwin & Webster, 2013; Maki, 1998; Nelson & Dunlosky, 1991; Nietfeld et al., 2006). These studies support the phenomenon that individuals are better able to determine their actual performance when they have been exposed to the task itself and not when they have not. This series of studies also supports the finding that individuals are better able to align their confidence in performance with future tasks, like what they are currently undertaking, once they have been exposed to it, otherwise known as relative monitoring judgments (Nietfeld et al., 2006).
The finding that participants' confidence in performance and actual performance were more closely aligned when referencing similar tasks given in the future (i.e., relative monitoring accuracy) is noteworthy. Wissman, Rawson and Pyc (2012) argued that questions about beliefs help to establish whether performing beyond what is expected is due to a deficit in metacognitive knowledge or a deficit in its implementation. Similarly, some studies support the need to establish mechanisms that encourage students to question themselves in relation to their conditional knowledge, which allows them to improve their metacognitive monitoring to determine more effectively what they know and do not know about their learning (e.g., Gutierrez & Schraw, 2015; Schraw, Kuch & Gutierrez, 2013; Gutierrez et al., 2016). Thus, it seems students show some optimism in their confidence levels for future performance on similar tasks, a finding that should be more thoroughly examined in future studies.
Specific results across moments show that individuals initially are overconfident in their performance judgments, with greater overconfidence evident in the prediction judgment, before the first test, but that this overconfidence decreases with each successive moment. The only interesting exception here is that the confidence level increased slightly between the second and third moment. This slight increase between the second and third moment could be a function of people who continually seek to adjust confidence in performance, given the demands of the task and future performance on similar tasks (Gutierrez & Schraw, 2015; Gutierrez et al., 2016; Schraw, 2009). Research supports the notion that confidence will be more consistent with proximal than distal tasks (Marchand & Gutierrez, 2012, 2017), which may help explain why confidence is similar between the second and third moments than between the first and the third. Thus, results support the conclusion of the initial confidence bias already reported by extant research insofar as overconfidence predominates among low-achieving students (Hacker, Bol, Horgan, & Rakow, 2000). This is also the case in performance of students as the cognitive task becomes more difficult (Schraw & Roedel, 1994).
Recommendations and Implications for Research and Practice
This research tentatively underscores the importance of examining characteristics beyond those that are "cold" cognitive factors that contribute to learning. Results showed that perhaps researchers should delve into research not only of cognitive and metacognitive factors, but also of dispositional factors, such as cognitive styles and personality, as this also influences learning outcomes. Exploring the dynamic relationships between cognition, metacognition, and disposition (e.g., cognitive styles and personality) can provide a richer insight into individual learning differences, and it also has the potential to inform the development of personalized educational interventions that are more specific and less general/broad for more sustained learning effects. Indeed, recent research concluded that certain personality factors, namely conscientiousness and openness to new experiences, positively predict self-report metacognitive awareness (Gutierrez de Blume & Motoya-Londoño, 2020). Continued exploration of these issues should lead to the demolition of " theoretical silos" and encourage researchers to become more involved in a theoretical mix in already established frameworks that, thus far, seem to work in isolation from other relevant frameworks.
Avenues for Future Research
This exploratory research has opened the door to further examination of these topics. Additional descriptive studies should be developed that examine the relationship between cognition, metacognition, and dispositional factors such as cognitive styles and personality and how these influence learning processes and outcomes. Additional research on the instability or invariance of these constructs in more languages and cultures should also be conducted. Much of the research to date investigates phenomena in one language and employs samples from a single culture. Until researchers encourage more cross-cultural, cross-language research, the research community will have no additional confidence in the universality (or lack thereof) of the constructs examined. Future research efforts should also recruit larger samples to establish the stability of the results. Finally, it seems relevant to delve into the latent association that working memory may have in the explanation of this relationship, as some researchers have argued that in cognitive restructuring tasks used for evaluation of cognitive style (FDI), students with a FI style show better performance (Miyake, Witzki, & Emerson, 2001; Miyake, Friedman, Shah, Rettinger, & Hegarty, 2001).
Methodological Reflections and Limitations
No research involving humans is without error and, therefore, the reader should be aware of the limitations of this study. First, the study is exploratory in nature, as few studies have related metacognitive skills such as monitoring accuracy with cognitive styles. As such, it was not possible to develop a more robust research design with more specific research questions and hypotheses. Second, the sample was not only relatively small, but was also chosen for convenience, since the participants self-selected.
Despite these limitations, however, there are some strengths to the study. On the one hand, the performance, cognitive styles, and metacognitive monitoring measures were objective in nature, and therefore, the data do not employ self-report survey measures, increasing the validity of the conclusions drawn from the data. Furthermore, the innovative nature of this study will stimulate debate among researchers in these areas and help inform future research efforts. Finally, the present study investigated these constructs in a (Colombian) culture and (Spanish) language that differs from most research on these topics. Therefore, the present study, although exploratory, represents a contribution to the literature on these topics.
Conclusion
The present study sought to demystify the relationship between cognitive styles, confidence in performance judgments, and metacognitive monitoring accuracy. This represents an important advance because no research to date has examined these relationships, especially the use of objective measures without the inclusion of self-report surveys. In line with previous findings, individuals tend to exhibit poor monitoring accuracy (i.e., erroneous in their performance judgments) when they have not seen the task than when they have been exposed to it. Furthermore, participants tended to be more accurate in their judgments of relative versus absolute monitoring. Gender also played a role in that men tended to be more confident than women, a finding supported by previous work. Of importance to this study, individuals with a FD cognitive style were more confident and less accurate in their metacognitive monitoring judgments than individuals who were intermediate or FI. Therefore, this study highlights the need to consider cognitive, metacognitive, and dispositional factors, such as cognitive styles, to better understand how people learn and make judgments about their learning. These findings, and those stimulated by this research, have the potential to inform not only research and theory, but also the educational practice of educators in the classroom.