INTRODUCTION
Diseases caused by foodborne pathogens are prevalent, even in developed countries. Staphylococcus aureus is a facultative, non-spore-forming, round-shaped Gram-positive bacterium. When this bacterium reaches a population of approximately 106 CFU/g in a person, it can produce an enterotoxin responsible for severe symptoms such as vomiting, a weak pulse, abdominal cramping, shallow respiration, and nausea (Aljasir & D’Amico, 2020; Rubab et al. 2018).
Staphylococcal food poisoning is often associated with protein-rich foods, such as meat and dairy products (Lin et al. 2018). Dairy products are susceptible to microbiological contamination due to their nutritional composition, pH, and high moisture content. Cheese, particularly fresh and minimally ripened handmade varieties made from unpasteurized milk, is regarded as a potential carrier of foodborne disease. The Costeño Cheese (CC) is an artisanal raw milk cheese manufactured by enzymatic coagulation without the addition of starter cultures, and it is highly consumed in the Colombian Caribbean region. During its fermentation, undesirable microbial species can proliferate. Among these pathogens, S. aureus is the most common contaminant in artisanal cheese (Valík et al. 2018).
In recent times, there has been a growing interest in the use of natural polymers to create active films, which are produced by incorporating natural additives with various features such as antioxidant or antimicrobial properties. The literature suggests the use of plant extracts (Lotha et al. 2018) to eliminate foodborne pathogens. Due to the vast diversity of plant species, there is a constant demand for exploring new sources of substances with antibacterial and antifungal properties. Natural additives can be extracted from numerous plant origins. Schinopsis balansae, commonly known as quebracho, has not been previously employed in active film manufacturing. However, Salvat et al. (2001) reported that the extract of S. balansae exhibited a weak but significant bacteriostatic activity.
Predictive microbiology is a scientific discipline focused on study microbial behavior in food environments, and it involves the development of mathematical models that can be used to predict food poisoning under specific conditions (Cai et al. 2023). Predictive models have been developed employing data obtained from pure culture experiments in microbiological media. These models are employed to provide reliable predictions of microbial growth in food matrices in response to environmental conditions such as temperature, pH and water activity (Ding et al. 2011). Predictive models can be valuable for risk assessment, especially estimating changes in the bacterial population in a food chain. Moreover, the microbial prediction is considered an interesting alternative to prevent food poisoning. Different validation indices such as root mean square error (RMSE), accuracy factor (Af) and bias (Bf) have been proposed to evaluate the quality fit of one model (Geitenes et al. 2013). There are primary and secondary models widely used in predictive microbiology.
Primary models describe variations in bacterial cells as a function of time, while secondary models pertain to the responses of growth parameters resulting from fluctuations in environmental conditions. Mathematical models describing the effect of temperature on S. aureus growth have been published (Kim et al. 2018). However, the impact of active film manufactured using an aqueous extract of S. balansae on the growth of S. aureus in cheese has not yet been reported. Therefore, the purpose of this study is to develop and validate a secondary model for S. aureus growth in cheese packaged with active film.
MATERIAL AND METHODS
Bacterial strains and growth conditions. S. aureus was obtained from the food microbiology laboratory at the Universidad de Cartagena, Colombia. A 1 mL aliquot of the culture was mixed with 10 mL of tryptic soy broth (TSB) and incubated at 37 °C for 24 hours to activate the inoculum. Subsequently, a 1 mL portion of the activated strain underwent a second activation in TSB (at 37 °C for 24 hours) to achieve a viable bacterial population of approximately 2 log CFU/mL.
Steam distillation system. The distillation process for obtaining the aqueous extract of S. balansae was carried out through a system composed by a volumetric flask container (VFC) as a heat source to generate steam by boiling water. The VFC is linked to another spherical glass container (SGC) with two entrances, the upper entrance receives the VFC, and the bottom entrance is coupled to a glass condenser. A glass collector recovers the aqueous extract from vegetal material into the condenser. The leaves (30 g) of S. balansae were placed in the SGC flask and water in the VFC. Finally, water is heated, and the vapor passes through the leaves in the SGC dragging active principles that then are condensed and recovered as aqueous extract in the glass collector (González-Cuello et al. 2018).
Gas chromatography - mass spectrometry. 50 μL of aqueous extract of S. balansae along with 450 μL of dichloromethane were injected into a gas chromatograph (Agilent Technologies 7890A), coupled to a mass spectrometer (Agilent Technologies 5975C) and HP Chem Station data system using a DB-5MS capillary column (J & W Scientific, Folsom, USA) with a stationary phase of 5% phenylpolymethylsiloxane (60 m x 0.25 mm, D.I. x 0.25 μm) for the separation. Oven temperature ranged from 45 ºC (5 min) to 150 ºC (2 min) with ramps of 4 ºC/min.
Edible film formation. 0.5 % (w/v) of low acyl gellan (LAG) (Modernist Pantry. EEUU) was directly dissolved in deionized water at 80 °C. Once it was completely dissolved, a plasticizer (8 % w/v) was added. Subsequently, the dissolved LAG solution was air-conditioned and 10 mL of aqueous extract of S. balansae was incorporated. Then, the dispersions were spread over Petri dishes and dried for 48 h at 34 °C. Dried films were peeled intact from the casting surface.
Inoculation and enumeration of S. aureus. Samples of CC were purchased from a local supermarket (Cartagena, Colombia). A total of 5 kg of cheese was cut into 11 g portions. Then, 100 µL of the S. aureus (2 log CFU/g) were uniformly added to the cheese surface employing a micropipette. Then these samples (11 g) were packaged with edible film in different trays and stored at different temperatures (6, 12, 18 and 21 °C) during various days. Likewise, blank samples (cheese without covering) were used as a control. For obtaining each growth curve, the inoculated samples at each temperature were mixed with 99 mL of peptone water and homogenized in a stomacher. S. aureus was enumerated on Baird-Parker agar (Merck, Darmstadt, Germany) after 48 h incubation at 37 °C. The results were expressed as log CFU/g. For each storage temperature at least two biological replicates were carried out (two growth curves obtained from each temperature).
Primary modeling. The experimental data of S. aureus in various packaged CC was fitted to the Huang model (Huang, 2013) (Equation 1).
Where y0, ymax and y(t) are the bacterial concentrations at the initial, maximum, and at time t respectively; µmax represents the maximum growth rate ENT#091;(log CFU/g)/hENT#093; and λ represents the lag-phase.
Secondary modeling. The maximum growth rates (µmax) obtained from the Huang model were modelled as a function of temperature using the polynomial model (Equation 2).
Where, log x is the logarithm of the growth rate; a, b, and c are constants and T is temperature.
Validation of predictive models. The accuracy factor (Af), bias factor (Bf) and root mean square error (RMSE) were used in this study to evaluate the accuracy of the predicted models. The equations 3 and 4 for Af, and the equation 5 for RMSE.
where, the variable factors obs, pred, and n are the observed values, predicted values, and repetition number of the observed data, respectively.
Statistical analysis. The results for growth parameters are expressed as means plus/minus standard deviation. Microbial counts were expressed as log values for statistical analysis. The influence of the active film application on µmax was evaluated through an analysis of variance (ANOVA-one way) along with post hoc tests (LSD test) were employed to determine the homogeneous groups (p < 0.05) using SPSS software version 23.0.
RESULTS AND DISCUSSION
Gas chromatography - mass spectrometry (GC/MS). Leaves of S. balansae were submitted to steam distillation. GC/MS analyses were performed on the aqueous extract displaying the presence of 4 compounds (69.71 %). Table 1 shows the principals compounds identified, the retention time and the concentration area. The major compounds were: 9.12.15-octa catrienoic acid (30.75 %), followed by 2.4.6-cycloheptatrienone (20.48 %), pyrocatechol (12.63 %) and 3-amino-2-phenazinol (5.85 %).
On the other hand, Venter et al. (2012) reported that extracts of Schinopsis lorentzii and S. balansae contained complex compounds, with pyrocatechol being the primary constituent of the aqueous extract. Pasch et al. (2001) also indicated that quebracho had a similar composition, primarily based on combinations of resorcinol, catechol, and pyrogallol building blocks. Villanueva & Marrugo (2014) reported that 9,12,15-octadecatrienoic acid has anti-inflammatory and antimicrobial properties, thus corroborating the information provided by Saraiva et al. (2013), where it was found that the Schinopsis genus exhibits antimicrobial properties against S. aureus, Klebsiella pneumoniae, and Pseudomonas aeruginosa. Therefore, our results can contribute to the control of the growth of pathogenic bacteria. However, further investigation needs to be performed to confirm these hypotheses.
Fitting Huang model. The suitability of models used for bacterial prediction depends on various factors, such as the type of microorganisms, the food, and environmental conditions. In the present study, the primary predictive model developed by Huang was applied to S. aureus growth on cheese covered with active films, as illustrated in figure 1. The results depicted in Figure 1 indicate that the initial concentration of S. aureus for all samples was approximately 2 log CFU/g. The growth pattern of S. aureus in cheese was similar to that reported in high-protein foods such as milk, meat, and egg products at various storage temperatures (Choi et al. 2019), exhibiting the three initial growth phases (lag phase, logarithmic phase, and stationary phase).
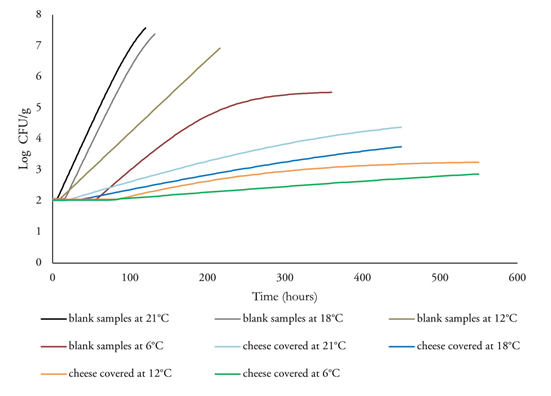
Figure 1 Growth of Staphylococcus aureus in costeño cheese with or without active film of Schinopsis balansae and stored at different temperatures.
The S. aureus population tended to increase at all temperatures (6 to 21 °C) in the blank samples (without covering). The rate of increase was reduced when active films were applied to cover the cheese samples. Therefore, the S. aureus population was lower in the cheese samples covered with active films than in those that were not covered, as shown in figure 1. These results indicate that S. aureus growth was significantly affected by both temperature changes and the application of active films. This behavior may be attributed to the presence of antimicrobial compounds in the aqueous extract of S. balansae. Therefore, the inhibition of S. aureus growth in the covered cheese is due to the antimicrobial compounds present in the aqueous extract.
The Huang model was adjusted to the experimental data to determine the growth parameters, such as initial cell count (Y0), maximum growth rate (μmax), lag phase (λ), and maximum cell population (Ymax), as shown in table 2.The Y0 values did not change significantly (p > 0.05), indicating that this parameter may be controlled when the bacteria are incorporated into the food system. Values of Y0 were similar for all analyzed temperatures, ranging between 2.010 and 2.075 log CFU/g. In other words, the application of the active film does not affect the initial count of S. aureus. The initial count of S. aureus is a critical safety indicator in artisanal dairy production, especially at the beginning of milk and young cheese fermentation (Aĉai et al. 2014).
Table 2 Growth parameters of Staphylococcus aureus in costeño cheese with or without active film of Schinopsis balansae at different temperatures using the Huang model.
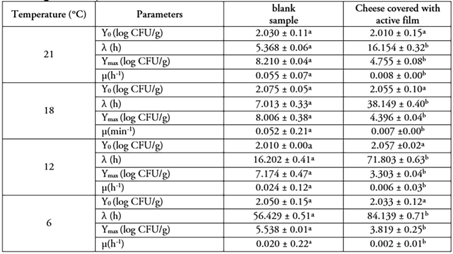
Rows with no common letter showed a statistically significant difference (p < 0.05) based on LSD test.
The lag phase (λ) represents the time necessary for cells to adapt to new environmental conditions. λ exhibited a tendency to decrease as the temperature increased in all samples. Higher values were observed in CC samples packaged with active film and stored at 6°C (84.139 h), 12 °C (71.803 h), 18 °C (38.149 h), and 21 °C (16.154 h) compared to cheese samples without covering, which were stored at 6 °C (56.429 h), 12°C (16.202 h), 18 °C (7.013 h), and 21 °C (5.368 h). The results indicate that the λ in samples covered with active film was longer than in the blank samples, confirming the inhibitory effect of the aqueous extract. Therefore, it seems that both the application of active film and low temperatures are required to increase the λ of S. aureus in CC samples. Similar results were reported by Lin et al. (2018), who found that λ of S. aureus in cooked pork sausage containing preservatives such as sodium nitrite (6.103 h), nisin (7.204 h), potassium sorbate (7.347 h) and sodium lactate (18.255 h) was longer than samples without preservatives (3.942 h). On the contrary, Cai et al. (2023) calculated λ values ranging from 0.803 to 3.941 h using the primary Gompertz model for the growth of S. aureus in in acid-curd cheese. However, it must be highlighted that these values were obtained at higher temperatures, ranging from 32 to 44 °C, and without the use of antimicrobial substances.
Another important parameter is μmax, which was significantly (p < 0.05) modified by the presence of the active film. In blank samples, the highest values were obtained at 21 °C (0.055 h-1), followed by those samples stored at 18 °C (0.052 h-1), while the lowest values were found at 6 °C (0.023 h-1) and 12 °C (0.024 h-1). These results align with those reported by Gomes de Araújo et al. (2017), who observed μmax values ranging from 0.003 to 0.048 h-1 for S. aureus when cultured on fresh and minimally ripened handmade cheeses made from raw milk. On the other hand, Cai et al. (2023) reported the following μmax values: 0.688 (at 44 °C), 1.011 (at 32 °C), 1.059 (at 40 °C), 1.256 (at 34 °C), and 1.401 log10 CFU/h (at 36 °C) using the modified Gompertz model for S. aureus growth in acid-curd cheese. According to the Food and Drug Administration (FDA, 2022), the optimal growth temperature for S. aureus is 35 °C. The experimental results verified that fermentation temperature is the most important influencing factor for μmax.
When the active film was applied to CC samples, the μmax values were as follows: at 21 °C (0.008 h-1), 18 °C (0.007 h-1), 12°C (0.006 h-1) and 6 °C (0.008 h-1). The μmax increased when the temperature increased, which is consistent with previous reports (Gomes de Araújo et al. 2017) indicating that temperature as a major environmental factor affecting microbial growth kinetics in foods. Thus, the non-application of active film along with mild temperatures, may lead to rapid microbial growth. The results of this study suggest that the Huang model can well fit the growth of S. aureus in the fermentation stage.
The last parameter calculated by the Huang model was the maximum bacterial concentration reached by S. aureus at the end of the logarithmic phase (Ymax). This parameter is relevant considering that some foodborne bacteria produce toxins when they reach a certain bacterial population.
Covering the CC with active film of S. balansae significantly affects (p < 0.05) Ymax of S. aureus. In general terms, Ymax values were higher in blank of CC samples (ranging from 8.210 to 5.538 log CFU/g) compared to the samples covered with active film (ranging from 4.755 to 3.819 log CFU/g). Cai et al. (2023) found Ymax values between 3.935 and 6.257 log CFU/g for S. aureus growing over a temperature range from 32 to 44 °C without the addition of antimicrobial compounds. This difference may be due to differences in cheese production, fermentation conditions, and types of S. aureus. Based on the above-mentioned findings, it can be concluded that the aqueous extract of S. balansae used as active film can significantly inhibit S. aureus in CC.
Secondary model for S. aureus. The secondary model equation was developed using the µmax values calculated from the Huang model. A polynomial equation was applied to illustrate the effect of storage temperature and the application of active film on S. aureus growth. The µmax changes in CC samples according to temperature and packaging conditions are illustrated in figure 2. The µmax values were directly proportional to the storage temperature. However, this increase was less significant (p < 0.05) when active films were used to cover the CC.
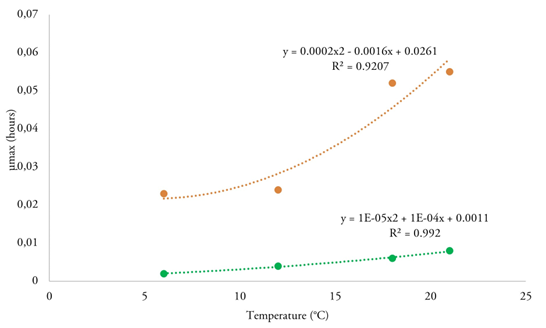
Figure 2 Effect of temperature on maximum growth rates of Staphylococcus aureus in costeño cheese calculated from Huang model. Green line covered with Schinopsis balansae film, orange line without film.
Considering that most secondary models are constructed under real and extreme storage temperature conditions, it is important to conduct a validation process to assess their prediction accuracy. Validation indices such as Af and Bf have been proposed to validate polynomial models (Baranyi et al. 1999; López et al. 2006). Af represents the sum of absolute differences between predicted and observed data of one parameter measured in the secondary model. Bf is the relative deviation among predicted and observed values and it is used to determine whether the model over- or under-predicts bacterial growth. For example, if the value calculated for Bf is outside the range (0.7 - 1.5), the model is considered unsuitable; whereby, a perfect agreement between predictions and observations indicates values of Af and Bf equal to 1.0 (Choi et al. 2019; Ross, 1999). The last parameter proposed is RMSE, which compares the observed values in the experiment with those predicted values calculated in the predictive bacterial model at the same temperature. The closer the calculated value is to zero, the more acceptable is the model.
The validation process of the secondary model for the growth of S. aureus is summarized in table 3. These values confirmed the ability of the polynomial model to predict the µmax of S. aureus during milk fermentation, as Bf values were close to 1.0, indicating that the predicted value is good. Af is also an important index of the model's prediction performance that Bf cannot comprehensively evaluate. The closer Af is to 1, the better the model is. In addition, Braun & Sutherland (2003) proposed that Af values between 1.1 and 1.9 can be recognized. The results reported in this study are like those published by Yu et al. (2020), who reported values of Af (1.06 - 1.13), Bf (0.91 - 1.00) and RMSE (0.00 - 0.68) in the development and validation of secondary models for growth factors of S. aureus in raw beef under various packaging conditions. On the other hand, Cai et al. (2023) validated a secondary model based on artificial neural networks using the same indices (Af, Bf, and RMSE) and obtained the following values: Af = 1.064, Bf = 1.037 and, RMSE = 0.067.
Table 3 Mathematical validation of the secondary model to describe the response of Staphylococcus aureus in Colombian costeño cheese, with or without active film of Schinopsis balansae
The result reported in the current research regarding the validation of secondary model is acceptable for describing the growth of S. aureus in CC covered with an active film of S. balansae. It should be noted that real CC samples were used instead of laboratory culture media; hence, the obtained results could be considered more reliable for practical applications. Furthermore, when microbial growth is estimated in artificial microbiological media, models tend to overestimate predictions, which may be overly conservative for some practical cases (Pérez & Valero, 2013).
In this study, a secondary model using a polynomial equation was developed to describe the response of S. aureus on cheese covered with an active film of S. balansae. These films were manufactured using an aqueous extract of S. balansae. The main compounds found in the aqueous extract of S. balansae were 9,12,15-octatrienoic acid, 2,4,6-cycloheptatrienone, and pyrocatechol, which may exhibit bacteriostatic activity against S. aureus.
The active film had a significant influence on growth parameters such as µmax, λ, and Ymax. The secondary model had Af and Bf values close to one, indicating that polynomial models can effectively predict S. aureus growth in CC covered with an active film. Therefore, the model may be valid for preventing the growth of S. aureus capable of producing enterotoxins responsible for food poisoning. Naturally fermented matrices, such as dairy products, are the most suitable environments in which these models can be applied.