Introduction
Pedestrian traffic data must be a fundamental base for the decision process related to sustainable mobility. However, according to [1], pedestrian mobility and cycling are the two modes of transportation more understudied.
Pedestrian monitoring is required to generate necessary inputs for an informed decision-making process to benefit the population. For instance, to warrant a pedestrian traffic signal on a specific location, it is necessary to have a minimum number of pedestrians crossing the street during a period. Usually, the warrant refers to the pedestrian traffic peak hours; therefore, it is necessary to understand the temporary variations of pedestrian volumes.
In many jurisdictions, short-duration counts can be applied to reduce monitoring costs, and the information collected can be adjusted using expansion factors from places with similar temporal patterns to obtain daily or annual pedestrian traffic estimates, which could allow jurisdictions, for example, to determine the population who benefited from a specific improvement.
Despite this, there is only one study available regarding the temporal variation of pedestrian volumes in Costa Rica [2] and, the monitoring efforts have been historically focused on motorized traffic that provokes an underestimation of non-motorized traffic [1].
There is minimal knowledge of the geographical and temporal variation of pedestrian traffic [3; pp. 4-31], even though pedestrian data must be the base for the evaluation of active mobility projects, transportation planning, and pedestrian exposure analysis [4]. This lack of information limits the ability of agencies to adequately improve and manage the non-motorized infrastructure and improve pedestrian safety efficiently [5], even though the positive impacts of non-motorized modes of transportation have been recently acknowledged [6].
If information is not collected for a specific road user, there is a probability that it could not be adequately included in the management of a city. Therefore, pedestrian volumes are a valuable source of information; however, they have not been extensively explored in Costa Rica. There is a lack of pedestrian mobility databases in the country, and most of the traffic monitoring effort is related to motorized traffic.
Suppose a pedestrian study is performed in the country based on peak hour manual counts. In that case, there is no reliability on the expansion factors used to predict average daily pedestrian traffic estimates because there is minimal knowledge regarding the temporal variation of these volumes.
Similarly, [3] indicates that in the United States, there is a predisposition to apply very short duration counts for pedestrian traffic monitoring (pp. 4-1). Furthermore, [7] indicated that the most common source for pedestrian data is manual counts, even though annual estimates from short-term manual counts could generate errors between 30 and 60 percent. Additionally, [8] indicate that the research related to the study of temporal factors for non-motorized traffic is scarce.
This article explores a specific urban area through cluster analysis to develop temporal pattern groups. The methodology proposed could be replicated in other jurisdictions to have a better understanding of pedestrian behavior.
This study aims to establish specific distinct pattern groups for the temporal variation of weekday pedestrian volumes applying cluster analysis in the central business district of Guadalupe in San José, Costa Rica.
Background
In a study related to the use of automatic counters to extrapolate volumes from manual counts, [9] argued that it is crucial to consider aspects like weather, hour, and location to establish hourly factors. These hourly factors can be used to expand short-term counts. In their study, they indicated the importance of characterizing these factors for future application. [4] applied three different methods to expand short-duration counts: assuming that there are no hourly variations in pedestrian traffic, applying temporal factors obtained from motorized traffic, and relating expansion factors from cities with similar characteristics. They concluded that applying expansion factors from other jurisdictions could generate more significant errors than the other analyzed methods.
Typically, cluster analysis has been used as a method to characterize temporal traffic patterns. For example, cluster analysis has different applications: to characterize truck flows [10]-[13] or traffic patterns [14], to classify roads according to their use [15], or to identify unusual patterns or nonrecurrent events [16], [17]. Additionally, [18] characterized the stations of a public bicycle system according to entrance and exit. Also, they related their station classification with the geographic location of the stations. [19] applied clustering techniques to identify possible variables that could influence the pedestrian injury severity.
In Costa Rica, clustering analysis has been used to characterize temporal factors for motorized traffic [20]; in this study, routes are classified according to the temporal distribution of traffic. The methodology proposed by [20] has been adopted in this study for non-motorized traffic, using the data collected in a previous study [2]. On the other hand, [21] found that commercial and service developments and the area of walkways available affect pedestrians' volume.
Study area, data collection, and counting sites
The study area comprises the Central Business District (CBD) of Guadalupe in the Municipality of Goicoechea, in the province of San Jose, Costa Rica. Guadalupe is one of the seven districts of Goicoechea. Statistics regarding Guadalupe and Goicoechea are in Table 1. This information can be helpful to evaluate the applicability of this research in similar cities outside Costa Rica. The district of Guadalupe was selected due to similarities to other urban areas in Costa Rica: a predominant commercial land use, its streets and avenues forming a grid, and the presence of sidewalks.
Table 1 Characteristics of the Canton of Goicoechea and District of Guadalupe
Characteristic | Goicoechea | Guadalupe | Source |
---|---|---|---|
Area (square km) | 31.65 | 2.39 | [22] |
Population | 136,112 | 22,520 | [23] |
Percentage of urban population (2011) | 98.5 | - | [24] |
Literacy rate (2011) | 99.0 | - | [24] |
Percentage of population with a disability (2011) | 11.7 | - | [24] |
Average number of years of education received by people aged 25-49 (2011) | 10.5 | - | [24] |
Average number of years of education received by people aged 50 and older (2011) | 8.7 | - | [24] |
Homicide rate per 10,000 habitants (2019) | - | 0.4 | [25] |
Road traffic crashes with victims (injured or dead) (2017) | 385 | 176 | [26] |
Source: Own elaboration
Additionally, [2] mentioned that in Guadalupe, several transit routes converge; therefore, the study area attracts and generates several pedestrian trips. Expressly, the study area is limited by avenues 27 and 35 and streets 39 and 67, the national routes 218 and 201 shown in Fig. 1. Route 218 has an Average Daily Traffic (ADT) of 36379 vehicles per day, and Route 201 has an ADT of 21,490 vehicles per day. Both ADT estimates are for the year 2015 [27].
Forty-seven bus routes cover Guadalupe-Moravia's sector, most of them use National Route 218, and more than 200,000 passengers use these routes every day [28].
Materials and methods
The data were collected at 46 different sites, distributed as shown in Fig. 1. The number of sites was determined to have enough spatial coverage in the study area and the number of available sites to place the pedestrian counter. The automatic pedestrian counter must be attached to a pole or a traffic sign, limiting the number of available spots where the counter can be placed. Counts duration was between eight and sixty days between the end of April and October 2016. At one point, the pedestrian data were collected permanently during five months. Additionally, for verification purposes, manual two-hour counts were performed in seven different points.
Regarding the pedestrian counts, at every counting site, an automatic pedestrian counter was placed. The counter has a passive infrared sensor with a high-precision lens. Generally, the sensor was attached to a utility post, over some time (usually one week), at the border of the sidewalk. The device registered pedestrian volumes at 15-minute intervals.
All 46 counting sites were included in the cluster analysis. The temporal variation of pedestrian traffic at every counting site was scrutinized. Three different sites presented unique characteristics and based on them, and some decisions were made:
The Counting site 16, next to a bus stop that connects different districts between Moravia and Desamparados, presented a different behavior during Mondays and Thursdays; therefore, for analysis purposes, this site was divided as site 16.1 and site 16.2.
Similarly, site 45 was considered three different sites: 45.1 (May 23rd and 25th), 45.2 (May 24th and 26th), and 45.3 corresponding to May 29th, 30th, and 31st.
Site 22 presented two different patterns, so it was considered two different sites: 22.1 and 22.2.
For every counting site, the hourly factors, which are the proportion of the daily pedestrian traffic, were estimated using eq. 1:
Where:
FH i : Hourly factor, for the hour "i" at counting site "p."
(VH) p : Pedestrian volume, at the hour "i" at the counting site "p."
(VD) p : Pedestrian daily traffic volume at the counting site "p."
The cluster method proposed by [29] was implemented to hierarchical grouping the vectors of hourly factors from the different counting sites.
This method groups elements by minimizing the Euclidean distance among elements of the same group and maximizing the distances from other groups. The different elements are hierarchically grouped; the decision to join the elements to a specific group is defined by choosing the minimum Euclidean distance to a specific group. Once the element is included within a group, the Euclidean distances are recalculated, and the process is repeated until only one group, which contains all elements, remains [30]-[31].
This process can be visualized through a dendrogram, where each element is assigned to a group; they are grouped until only one is formed. The vertical axis in the graph represents the Euclidean distance; therefore, the shortest the length of the vertical lines, the closest the grouping, indicating that these are the most similar elements comprising them. To establish the practical number of pattern groups, an adaptation of the hybrid approach proposed by [13] was adopted.
Once the groups have been established through cluster analysis, this classification is related to the location of the different facilities, categorized by land use, that may be sources of pedestrian trips, for which the possible attractors are defined below.
Table 2 Land use categories applied in the study
Category | Description | Number of locations |
---|---|---|
Municipal and public services | Includes the Municipality, the entities of payments of public services, and the municipal services | 7 |
Health services | A clinic, the red cross headquarters, and a hospital | 3 |
Banks | All financial facilities in the area | 14 |
Recreational | Parks | 2 |
Supermarkets | Small size grocery and convenience stores are not included | 7 |
Churches | Contains churches from different Christian denominations | 16 |
Restaurants | This category includes all the restaurants and “sodas” of the place that open at daylight-hours | 43 |
Night recreational | Includes restaurants, bars, and theaters that are open during the night. | 25 |
Schools | Academic and artistic schools | 8 |
Source: Own elaboration
This is an exploratory analysis because many facilities from different types are in the same block. This study attempts to identify the impact of each location type on pedestrian traffic. Fig. 2 shows the different facilities considered in the present study.
The different facilities were located on the map, and influence zones were established for each category at different distances (50, 75, 100, 125, and 150 m), and the counting sites found in each influence area were classified according to their group. The influence zone for each facility was established by the pedestrian travel path from the facility's front door. Distances longer than 150 m were not considered due to an overlap between many of the counting points, and considering the size of the study area and distances shorter than 50 m would exclude many facilities from the analysis.
A hypothesis test was considered: There is a relationship between the pedestrian temporal pattern groups and the land use in the surrounding areas.
A test of independence between the clusters and the different areas of influence is performed to prove this hypothesis.
One limitation of this study is that the interaction of different location types in the influence area of a site was not considered. Other aspects not considered in the present study are related to the width or accessibility (for example, presence of ramps for people with limited mobility) of the sidewalks in the study area; further details regarding the characteristics of the sidewalk can be found in [2].
Results
Cluster analysis
The cluster analysis was performed using R-Studio, and six different pedestrian pattern groups were obtained. Fig. 3 shows the dendrogram with the 46 counting sites on the horizontal axis. Additionally, based on the hybrid approach proposed by [13], pedestrian pattern groups C and D were divided into two groups for practical purposes:
■ Pedestrian Pattern Group CI includes sites 21, 26 and 32
■ Pedestrian Pattern Group C2 includes sites 2, 5 and 45.2
■ Pedestrian Pattern Group D1 includes sites 42 and 45.1
■ Pedestrian Pattern Group D2 includes sites 7, 8, 9, 10, 11, 20, 23, 24, 25, 28, 29, 30, 31, 43, and 44.
Fig. 4 shows the spatial distribution of the different pattern groups. Groups D2 and F have 15 and 19 counting sites, respectively, comprising more than two-thirds of the sites considered in the study. Groups A, C2, and E presented fewer counting sites on nearby locations.
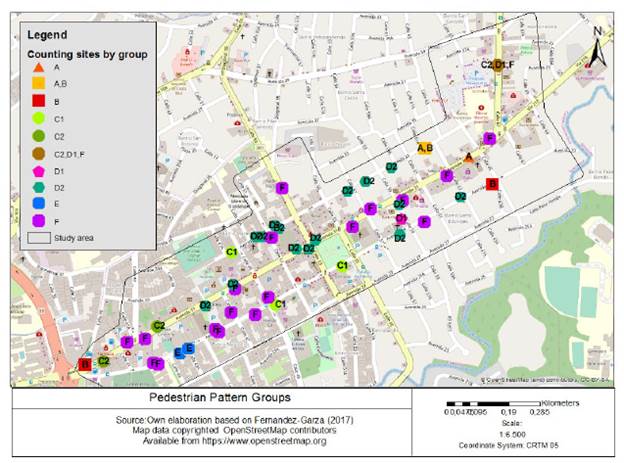
Source: Own elaboration based on [32], [33]
Fig. 4 Location of the counting sites by pedestrian pattern group assigned.
Fig. 5 depicts the eight patterns obtained. Every graph contains the hourly distribution for the counting sites in the group. Additionally, the dashed lines represent the average value for each group. Table 3 shows the average hourly factors obtained for each group.
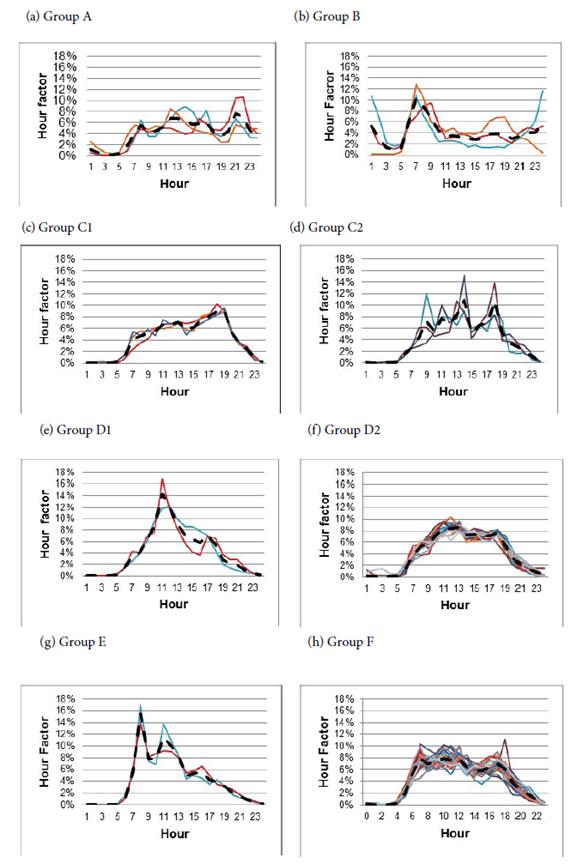
Source: Own elaboration Note. The dashed line shows the average values.
Fig. 5 Pedestrian pattern groups.
Table 3 Average hourly factors, in percentage, for each pedestrian pattern group
Hour | Group A | Group B | Group C1 | Group C2 | Group D1 | Group D2 | Group E | Group F |
---|---|---|---|---|---|---|---|---|
0 | 1.15 | 5.26 | 0.04 | 0.14 | 0.07 | 0.25 | 0.05 | 0.12 |
1 | 0.53 | 3.07 | 0.05 | 0.03 | 0.04 | 0.15 | 0.06 | 0.10 |
2 | 0.20 | 1.38 | 0.11 | 0.11 | 0.02 | 0.15 | 0.03 | 0.06 |
3 | 0.13 | 0.99 | 0.02 | 0.16 | 0.07 | 0.09 | 0.05 | 0.07 |
4 | 0.38 | 1.40 | 0.25 | 0.18 | 0.25 | 0.25 | 0.12 | 0.36 |
5 | 1.81 | 6.03 | 1.22 | 1.40 | 1.31 | 1.41 | 1.37 | 1.96 |
6 | 4.06 | 10.32 | 4.20 | 2.67 | 3.41 | 3.76 | 5.71 | 5.46 |
7 | 5.56 | 8.87 | 4.62 | 4.43 | 4.01 | 5.32 | 15.50 | 7.85 |
8 | 4.19 | 6.78 | 5.15 | 7.25 | 6.44 | 5.79 | 7.80 | 7.02 |
9 | 4.49 | 4.44 | 5.54 | 5.47 | 8.60 | 7.29 | 7.80 | 7.45 |
10 | 5.19 | 3.36 | 6.76 | 7.71 | 14.31 | 8.34 | 11.43 | 7.83 |
11 | 6.71 | 3.58 | 6.62 | 6.71 | 11.91 | 8.41 | 9.91 | 7.67 |
12 | 6.72 | 3.31 | 7.13 | 8.39 | 9.18 | 8.64 | 8.00 | 7.90 |
13 | 6.26 | 3.02 | 5.84 | 10.91 | 7.13 | 7.23 | 4.89 | 6.31 |
14 | 5.69 | 2.84 | 6.23 | 5.16 | 6.39 | 7.32 | 5.40 | 5.86 |
15 | 5.74 | 3.05 | 7.57 | 6.58 | 5.76 | 7.18 | 5.52 | 5.87 |
16 | 6.02 | 3.78 | 8.10 | 6.97 | 7.03 | 7.09 | 4.29 | 6.39 |
17 | 4.19 | 4.02 | 8.97 | 10.77 | 5.53 | 7.60 | 3.86 | 7.02 |
18 | 3.55 | 3.74 | 9.26 | 5.25 | 2.93 | 5.67 | 3.12 | 6.12 |
19 | 4.38 | 3.06 | 5.36 | 3.53 | 2.13 | 3.36 | 2.14 | 3.74 |
20 | 7.60 | 3.44 | 3.50 | 2.83 | 1.88 | 2.03 | 1.47 | 2.32 |
21 | 6.94 | 4.13 | 2.44 | 2.10 | 0.97 | 1.40 | 0.85 | 1.70 |
22 | 4.48 | 4.14 | 0.82 | 0.98 | 0.45 | 0.81 | 0.48 | 0.76 |
23 | 4.01 | 5.81 | 0.20 | 0.26 | 0.17 | 0.44 | 0.15 | 0.25 |
Source: Own elaboration
The following paragraphs describe the temporal variations of pedestrian traffic for each group, and some pictures are added to provide a context of some sites; the analysis of location categories and pedestrian flow patterns is explained further below.
Group A: Sites 16.1, 16.2, and 22.1. This group does not present well-defined peak hours. Even though the counting site 16 was separated in two, the cluster analysis joined them due to the pattern differences between Mondays and Thursdays because these differences are less significant than the differences among other sites. There is a variation of traffic, around four percent of total daily traffic, between 8 am and 11 pm (Fig. 6).
Group B: Sites 4, 19, and 22.2. All counting sites in the group presented a very high morning peak between 7 and 9 am. On the other hand, site 19 presents high pedestrian percentages late at night. This behavior might be explained by the location of a nearby bar with night activity.
Group C1: Sites 21, 26, and 32. These counting sites presented a very similar hourly distribution. Between1 and 5 am, the pedestrian volumes are practically null. After 5 am, there is a sharp increase in pedestrian traffic between five and seven. The number of pedestrians increases as the time of the day increases until 7 pm. The counting sites 21 and 26 are close to bus stops for busses coming from the city center that could explain the peak at 7 pm, due to workers commuting back to their homes (Fig. 7).
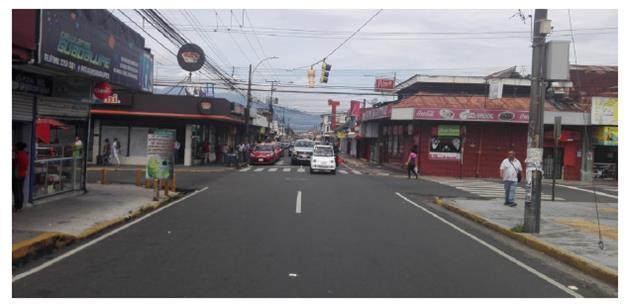
Source: Taken by the author
Fig. 7 Northwestern corner of Parque de Guadalupe, Goicoechea. San José, Costa Rica.
Group C2: Sites 2, 5, and 45.2. The hourly distribution of traffic shows short peaks. Two prominent peaks are predominant, one at 2 pm and another at 6 pm. These peaks could be explained by the location of a commercial plaza in front of site 45.
Group D1: Sites 42 and 45.1. These two sites presented a very similar hourly distribution of pedestrian traffic with a very high peak at eleven in the morning; however, they are in different locations, and their average weekday pedestrian traffic differs (705 and 2411 pedestrians for sites 42 and 45, respectively).
Group D2: Sites 7, 8, 9, 10, 11, 20, 23, 24, 25, 28, 29, 30, 31, 43, and 44. The hourly distribution for all counting sites is very similar, and they practically follow the average distribution from the group. This hourly distribution presents a constant increase in pedestrian traffic between 6 am until a peak is reached at 11 am, then traffic slowly decreases until 6 pm. After six, there is a sharp decrease in the percentage of daily traffic until 10 pm. Most sites are located on avenues 31 and 33. These sites also present a wide range of daily traffic, from 276 pedestrians per day, on-site 44, to 5215 pedestrians per day, on-site 7 (Fig. 8).
Group E: Sites 33 and 36. These two sites practically do not present pedestrian volumes between 1, and 5 am. At 6 am, pedestrian traffic increases at these sites, with a very high peak around 8 am (approximately 16 % of the daily traffic) and another peak at 11 am. Nearby, the Fernando Centeno Guell School, an educational center for people with disabilities, may influence the hourly variation of pedestrian traffic at these locations (Fig. 9).
Group F: Sites 1, 3, 6, 12, 13, 14, 15, 17, 18, 27, 34, 35, 37, 38, 39, 40, 41, 45.3, and 46. Group 6 includes the biggest number of counting locations. There are no abrupt changes in the hourly factors over the day. The pedestrian traffic is practically null between midnight and 4 am; then, the traffic increases until it reaches a plateau between 7 am and 5 pm when the pedestrian traffic drops. The daily traffic significantly varies among the counting sites from 353 pedestrians per day to 8067 pedestrians per day, at counting sites 34 and 1 respectively (Fig. 10).
Relation between groups and land use
Because some groups have very few counting points assigned, it was decided to redefine the pattern groups as D2, F, and others (others include groups A, B, C1, C2, D1, E) to have a more robust hypothesis test. Once this new notation is established, Fisher's exact hypothesis test is performed to analyze the independence of the data.
It also includes an analysis of the average weekday traffic (TPD ES) and its relationship with the established clusters groups, and all points are included.
Relationship between the influence of each facility type and the land use categories for each group
In this approach, the eight groups found are maintained through differences in time factors; for six, it is impossible to observe significant relationships between zones of influence and the different land uses considered since they have very few points. It is expected that at a greater distance, the number of points per group that the different establishments increase influence; this is only true for groups D2 and F, which have a more significant number of points.
Fig. 11 shows the result obtained for group D2. It is observed that the establishments that influence a higher percentage of the points are in the upper part of the graph in blue tones, that is: banks, restaurants, public services (municipal), bus stops, night recreation and churches and in red the categories that have less influence for this group (schools, parks, and supermarkets).
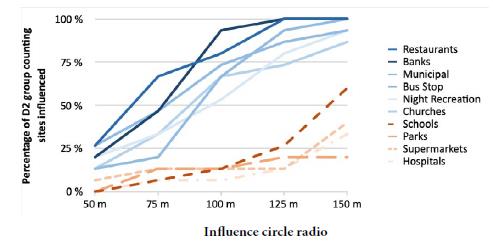
Source: Own elaboration
Fig. 11 Percentage of pedestrian pattern group D2's counting sites influenced by different land uses.
This behavior found for group D2 is repeated in all groups, considering that the land use is mixed and the study area is small.
Relationship between the influence circle radio and the clusters groups for each establishment
As mentioned previously, it was required to join some of the eight groups established through clusters at this study stage to achieve the independence test.
For each land use assigned to each location, the relationship between the distances from the other land developments and the number of counting sites of each category that enter each influence circle is obtained, and a Fisher's exact independence test is performed with a significance of α = 0.05.
According to the results shown in Table 4, only for the case of banks and public services, the null hypothesis is rejected; that is, the established groups depend on the distance they are from the banks and public services.
Table 4 Fisher's exact independence test results
Case | p-Value |
---|---|
Banks | 0.0011 |
Public Services | 0.0052 |
Schools | 0.1136 |
Night Recreation | 0.2117 |
Bus Stop | 0.2702 |
Hospitals | 0.3439 |
Supermarkets | 0.4506 |
Parks | 0.5632 |
Restaurants | 0.5497 |
Churches | 0.8180 |
Source: Own elaboration
As shown in Figs. 12 and 13 for both banks and public services, the percentage of points influenced in each group increases concerning distance. In both cases, group D2 is the most influenced by them.
Conclusions
The cluster analysis proposed in this study was appropriate to identify sites with similar temporal distributions of pedestrian traffic. However, this method does not explain the different temporal patterns obtained.
Therefore, a test was performed to relate the groups obtained with the land use in the surrounding areas; however, no relationship between them was found. It appears that the groups have a mixed influence from the different land uses. Further research is required because only the influence of banks and public services is suggested for Group D2.
This study shows how the temporal distribution of pedestrian traffic could vary significantly even in the same CBD. Nonetheless, two groups dominated the study area: Group F with 19 counting sites and Group D2 with 15 sites. These two groups contain more than two-thirds of the total sites included in the study. The variation of pedestrian traffic is similar for both groups. Based on their variations, the pedestrian volume is null, or very low, between midnight and five in the morning in the study area.
Additionally, the peak pedestrian traffic is around midday. After this period of the day, the number of pedestrians slowly decreases until 6 pm. After sunset, which is around 6 pm, the pedestrian traffic decreases significantly.
A limitation in the study is the number of counting sites, and these are not distributed uniformly as not all sidewalks within the study area have the necessary characteristics to place counters properly. Due to the implementation of exclusive transit lanes during the peak periods in May 2019, additional research is necessary to determine the effect of this measure on pedestrian behavior.
Additional research is recommended to determine explicative variables for the differences in the patterns found. For example, other studies have found relationships between weather and pedestrian behavior [1], [34] or the effect of urban density in walking activity [35]. The diversity of the patterns found indicates the need for further research and analysis better to understand a complex phenomenon such as pedestrian mobility. The interaction of different variables like transit facilities, services, and the weather should be included in future studies. Extreme precaution is recommended in using expansion factors for short-term counts; due to the heterogeneity of patterns based on the collected data.
This project was developed at LanammeU-CR as part of the activities related to Law 8114, as amended.