Musaceae (banana and plantain) are part of the main food products in the world, these crops play an important role in the socioeconomic growth of developing countries located in tropical and subtropical regions. According to FAO in 2019 the world production of bananas was 116 million tons and of plantain 41 million tons.
In Colombia, banana production in 2020 exceeded 2.4 million tons with a harvested area of more than 103,000 hectares, and with 86% of the national production destined for export. The average yield per hectare of bananas was 24.4 t ha-1 in 2020. The department of Valle del Cauca had a total banana production in 2019 of around 68,300 tons, being the third department with the highest production nationwide and an average yield of 14 t ha-1, at the same time, it should be noted that in terms of production for the national consumption, it occupies the first place with a 25% stake (MADR, 2021a).
According to the MADR (2021b) (Ministry of Agriculture and Rural Development from Colombia by its acronym in Spanish) in 2019, plantain production in Colombia exceeded 4.3 million tons with a planted area of more than 450,000 hectares, in this way, the plantain became the most planted crop in the country, and the most important in food security. In Colombia, the average yield per hectare of plantain has been 8.3 t ha-1 for the year 2019, a higher yield than the world average, which for 2018 was calculated at 7 t ha-1. For its part, the department of Valle del Cauca for 2019 had a total production of about 320,000 tons, being the fourth department with the highest production nationwide and an average yield of 11 t ha-1.
This research has the interest of studying the department of Valle del Cauca since according to the results of the last National Agricultural Census of 2014, the food production of it was 3.2 million tons, while the national production was around 33.2 million tons, placing Valle del Cauca in the first place of production with a stake of 9.6% (DANE, 2015).
In this way, to promote and increase the productivity of Musaceae crops in the department of Valle del Cauca, economic and technical resources are necessary that lead to the modernization of the production chain. According to Yang and Zhu (2013) agricultural modernization implies increasing the efficiency in the use of natural resources, monitoring and subsequent improvement of the organization of the production process and the active implementation of innovative technologies, therefore large investments and financial and productive resources are necessary.
Agricultural production is related to the period that elapses from the initial investments, the purchase of the inputs required for the establishment, and subsequent maintenance of the crop until the time of harvest and/or marketing of the products, said period includes stages of risk and uncertainty for the production process (Seven and Tumen, 2020). Therefore, access to agricultural credit programs can play a crucial role in the possible management of these risks, thus sustainably achieving growth in agricultural productivity and supporting decisions during the production process (Eswaran and Kotwal, 1986). Likewise, the main objective of granting credit is not only to improve the production and commercialization of the agricultural sector, is also to promote technological change (Fernández Moreno et al., 2011).
For the reasons mentioned above, many authors have used different methods and models to evaluate the impact that accesses to an agricultural credit program generates for a certain group of people on productivity, quality of life, and others.
International authors as Ciaian et al. (2012) estimated how access to agricultural credit affected input requirements and agricultural efficiency in CEE transition countries (Central and Eastern Europe). To do so, they turned to a farm-level single-panel dataset with 37,409 observations and used a matching estimator. Within their results, they found that access to agricultural credit increases total factor productivity by up to 1.9% for every 1,000 euros of additional credit, this in turn is based on a negative effect of access to credit on labor, suggesting that these two are substitutes.
Chandio et al. (2019) examined the impact of agricultural credit and farm size on the technical efficiency of rice productivity in Sindh, Pakistan. For that, they collected data from 180 rice farmers using a cross-sectional random sampling technique and did analysis through Maximum Likelihood Estimation (MLE). Among their results they found that credit, farm size, fertilizers, and labor have positive and significantly influenced rice productivity.
It is also important to mention that credit restrictions are of special interest when evaluating the access that a farmer may have, for example, Seck (2021) applied an endogenous commutation regression model to examine heterogeneous credit constraints and their effect on the productivity of small farmers in Senegal, obtaining results that indicate that credit restrictions hinder the productive performance of farmers.
A study conducted for Elahi et al. (2018) in 48 villages in the Sargodha district of Punjab, Pakistan, analyzed farmers access to agricultural advisory and financial services, and their impact on wheat productivity using Propensity Score Matching methodology (PSM). They found results showing that access to farm advisory services improves wheat productivity, as well as significant differences between farmers who had simultaneous access to farm advisory and financial services compared to those who had access to one or neither, were also found.
Owusu, (2017) applied also PSM methodology to assess the effect of access to credit on agricultural productivity of cassava in Ghana. The results showed that access to credit was determined by different factors such as: age, gender, level of education, size of the household and of the farm, agricultural experience, and extension service, as well as the hired labor and the distance between the farmer and the lender. Finally, the author found that credit has a positive and significant effect on cassava productivity.
Contrary to what was previously presented, other authors such as Nakano and Magezi (2020) found results in which financing is not necessarily related to increased productivity. They analyzed the impact of microcredit on technology adoption and rice crop productivity in Tanzania by conducting a randomized control trial (RCT) to estimate the intention-to-treat (ITT) effect as well as local average treatment effect (LATE) of microcredit, using treatment status as instrumental variable (IV). They obtained results in which it is evident that the financing programs granted do not result in an increase in rice yields, profits from rice cultivation or family income.
In the case of Colombia, Echavarría, Villamizar-Villegas, and Mcallister (2017) evaluated the impact of credit in the coffee sector, using a panel data model with fixed effects and instruments, together with common support given by estimated propensity scores, their results suggest that credit has a beneficial and significant effect on outcome variables. Echavarría, Villamizar-Villegas, Restrepo-Tamayo et al. (2017) through a Propensity Score Matching (PSM) analysis, studied the effect on some variables such as farm yield, and the Multidimensional Poverty Index (MPI) for long and short cycle crops. In general, the results suggested that the various types of credit have a positive and significant effect on yield (between 3% and 28%).
The main purpose of this research is to investigate the question of how access to agricultural credit affects agricultural productivity in Musaceae crops (plantain and bananas) in the department of Valle del Cauca (Colombia). To answer this question, data from the 2014 national agricultural census was used, and econometric methods were applied to analyze the relationship between access to agricultural credit and two productivity indices considered as production per hectare and production per employee. Following the existing literature, the main contributions of this study be listed below: it is the first study that has been developed that assesses the impact of agricultural credit at the departmental level, analyzing the crops of banana and plantain in specific way. Second, in this study two important factors or productive resources are considered to evaluate and analyze the efficiency and effectiveness in agricultural productivity, which are the surface of the land in which the crop is established and the labor. Third, this study considers the possible endogenous problems caused by the "selection bias" of the sample, so the non-parametric Propensity Score Matching (PSM) method was applied to estimate the impact of access to different credit programs in agricultural productivity in Musaceae crops.
MATERIALS AND METHODS
Data
This research will be based on survey data published by the National Administrative Department of Statistics (known by its acronym in Spanish DANE) in 2017, obtained from the National Agricultural Census (CNA for its acronym in Spanish) carried out in 2014 in Colombia; said census is identified at the level of the Agricultural Production Unit (UPA for its acronym in Spanish respectively)1. Therefore, UPA was the analysis unit for this research. Moreover, the results of CNA questionnaire, were structured in several modules where each one of them provided unique information. For this reason, files related to the characteristics of UPAs, people, crops, machinery, and infrastructure were of interest.
As CNA was carried out in 2014, all the information about agricultural production and earned credit correspond to 2013. Therefore, the results found express the changes in productivity of that year based on access to credit in that year.
The CNA covered a total of 2,370,099 UPAs nationwide. Valle del Cauca, which is the zone of interest for this study located in the southwest of the country has 3.2% of the UPAs registered (76,874). To meet the objectives proposed in this research, the data base was filtered, and it was reduced to banana producers (1,501), plantain producers (4,232) and farmers with both crops (880).
Treatment variable
Initially, the agricultural credit as "treatment variable" responds to the CNA question: "Was the requested credit or financing approved?", it is a dichotomous variable in which if the credit was approved the value it takes is 1 and 0 if it was not obtained. As the credit acceptability rate is quite high, since in average 87% of the Musaceae producers obtained the credit (DANE, 2017), this becomes a limitation since the application of the PSM methodology requires a big population to form the control group, therefore it is proposed to include those farmers who did not request financing or credit in the control group and manage the possibly systematic differences produced with the implementation of said methodology. The credits granted come from any entity such as banks, cooperatives, individuals or moneylenders, government programs, or warehouses of agricultural and agro-industrial inputs.
Dependent variable (Productivity Index 1 and 2)
The variable of interest or result was agricultural productivity measured in two indices proposed in this study. The first productivity index PI 1 was calculated as the natural logarithm of the division of production (in tons) by the harvested area (in hectares), the second index PI2 was calculated as the natural logarithm of the division of production (in tons) by the total number of employees in the farm (including permanent and daily employees belonging or not to the family, that is, all the labor available for the agricultural activity).
In general, in this research, the natural logarithm was applied to some variables, as can be seen in Tables 1 and 2 (including productivity indices), since for the econometric analysis, applying the natural logarithm, the effect of the units of the variables on the coefficients is eliminated, and given the properties of the logarithms, some complex mathematical operations are facilitated.
Method
Because credits are not awarded randomly among farmers, there is a selection bias problem, which will cause ordinary least squares (OLS) estimate to produce a biased effect of the impact of credit on agricultural productivity. Consequently, this research applies the Propensity Score Matching PSM methodology to obtain the causal effect of the granting of agricultural credit, however, its application assumes of conditional independence, which establishes that the selection of the treatment is given exclusively by observable variables.
According to Vinha (2006) the general idea of this methodology is to evaluate the impact of estimating the counterfactual without treatment using the information of those individuals who have received the treatment and similar individuals who did not receive. In the same way, Heinrich et al. (2010) point out that the PSM solves the question of what would have happened to the participating individuals in the absence of treatment using information from the group of those individuals who did not participate. For this study, the objective is to form a control group with farmers who have a propensity scores (PSCORE) or probability b(x) similar with those who received agricultural credit. By comparing how results differ between participating and nonparticipating individuals who have equivalent observable characteristics (control variables), the intervention effect is estimated by averaging the differences between the participants and their matched comparison cases. In this way, the PSM methodology allows to calculate the Average Treatment Effect on the Treated (ATT). Therefore, the impact of the agricultural credit on productivity indices studied can be given by:
Where, treatment condition (With Agricultural Credit) is denoted by WAC =1 and WAC = 0 otherwise, and the impact variable (PI
1 and PI
2) of interest is denoted by ,
if the credit was received, and
,
otherwise.
It is worth to mentioning the weaknesses of this method as Hoz Aguilar, (2019) did, who summarizes the following limitations: PSM technique requires large databases, one of its conditions to be used is that the region of common support between the treated and untreated must be met and also it is not possible to establish or demonstrate that there are no differences in unobserved variables, since these can affect both the probability of participation and the results.
Control variables
The control variables included two main categories. The first category included factors that can affect the agricultural productivity as systems or technology used in the crops, for this research it was taken into account if the farmer implements a pressure irrigation system (drip, sprinkler, pumping) or a surface system by gravity or manual, another indicator of the modernization of agricultural work can be the existence or not of agricultural machinery, use of fertilizer organic, chemical or other method to improve the soil. Other determinants that can affect productivity were the area (in hectares) allocated to agricultural infrastructure such as warehouses, ponds, silos, wells, etc., the land area (in hectares) occupied by the UPA and if the farmer received technical assistance.
And the second category covers the personal characteristics of the farmers including level of education calculated as percentage of people without education living in the farm, as well as their race, understanding by majority those who do not belong to an indigenous, gypsy, raizal or black groups, and if farmers use of permanent and/or temporary employees including family members.
Thus, these variables become control variables, since they can systematically generate different groups. This will be corroborated in the following analysis and results section (Descriptive Statistics), where it will be possible to observe if there are significant differences between the group made up of farmers who accessed agricultural credit and those who did not, according to each of the control variables.
RESULTS AND DISCUSSION
Descriptive statistics
This section presents the descriptive statistics corresponding to the sample used to estimate the impact of agricultural credit. According to the results of the descriptive statistics alone (Table 1 and Table 2), when the agricultural credit is approved, the average value of the PI 1 for plantain crops and PI 2 for plantain and banana crops are higher and significantly different, this suggests a possible positive correlation between access to credit and the productivity indexes studied. However, in the case of PI 1 for banana crop, although it presents higher average values for the treated group, the difference is not statistically significant, suggesting that there is no positive effect of agricultural financing on this.
Table 1 Definition and descriptive statistics of dependent variables with and without agricultural credit.
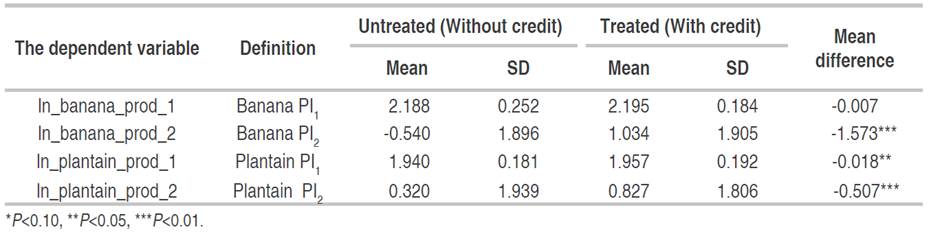
Table 2 Definition of control variables obtained from questionnaire of CNA and descriptive statistics with and without agricultural credit.
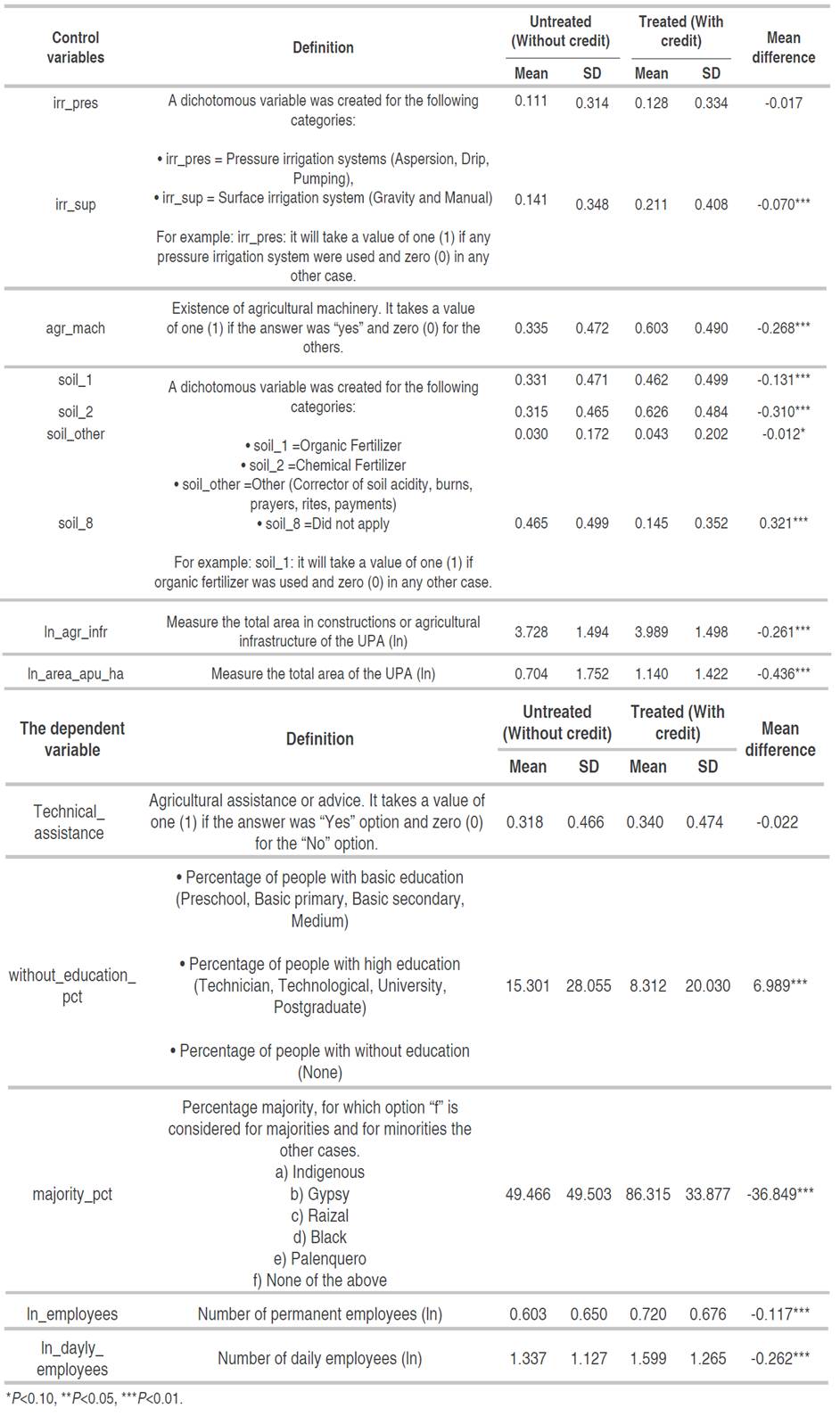
Another interesting result is about technical assistance, which does not have significant differences between the average values of the two groups but has a higher value in the treatment group than in the control group. However, technical assistance was considered within the group of control variables, since according to the census questionnaire, advice on credit and financing was part of the technical assistance received by some of the farmers.
There are significant differences between the control and treatment groups if the farmer implements some type of innovation on the farm, such as the use of agricultural machinery, application of some method to improve soil fertility, or if an irrigation system is installed. On average, who accessed an agricultural credit has the highest percentage of the population with owns agricultural machinery (61%), implements chemical fertilization (63%) over organic fertilization (46.2%), and uses irrigation systems (on average, the surface irrigation system is more implemented than pressurized).
The group that did not receive any type of credit have an average of 15.3% of its population without education (basic or high) while those who received the credit, only 8.3%. On the other hand, on average 86% of people who received some types of agricultural credit do not belong to some ethnic minority group, while those who did not receive it, have an average of 50% minorities.
In general, as can be seen in Table 1 and Table 2, the groups have different means in the group that had access to agricultural credit and in those that did not receive it, which leads to the conclusion that they are not randomly distributed. Therefore, these differences confirm the need to create a control group that is comparable to the group that accessed agricultural credit and to apply the methodology proposed in this research.
Common support
The common support shows the probabilities of participation for both UPAs with credit and those without. As can be seen in Figure 1, exist a common support, since each UPA with credit and with a defined probability can be associated or "matched" to a unit without credit with a similar probability.
Balance test
Before using PSM, the samples must pass the balance test, which ensures that there is no systematic difference between the treatment group and the control group after pairing. In other words, it is necessary to verify that the post-pairing rocking has corrected the problem of selection in observables, which was evidenced by the tests of differences of means carried out in the item "Descriptive statistics". As can see below, Table 3 and Table 4 show balance test results for each crop.
According to balance test (Table 3, Table 4), there is evidence of a reduction in the selection bias in observables. Therefore, it is argued that the consistency of the results through the balancing test is an indication of the robustness and reliability of the results found.
Banana productivity
As can be seen in Table 5, the impact of agricultural credit on PI 1 is positive and significant (8.4%). Therefore, it can be affirmed that banana producers who have access to agricultural credit, they achieve an average 8.4% increase in tons produced per hectare, contrary what was initially suggested in basic analysis of descriptive statistics, where a possible non-relationship between access to agricultural credit and the PI 1 was intuited. To get an idea of the magnitude of the effect calculated with PSM methodology in terms of the unit of measurement of the result variable, an average an increasing the yield from 8.8 to 9.5 t ha-1 is expected, in other words an increase of almost 1 t ha-1.
Table 5 Results of propensity score matching for banana crops applying 4-nearest neighbor matching and its standard error.
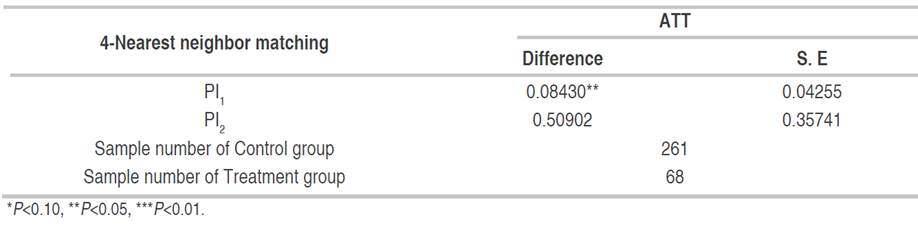
As mentioned in the introduction, the production of bananas in Valle del Cauca is destined for national consumption, however, this crop at the national level mainly has an export market. According of this, FINAGRO (2018) (Financial entity for the Colombian agricultural that provides resources through banks, cooperatives, and microfinance intermediaries) affirm that for export-type bananas, systems production of high technological level is required. However, according to the results of the CNA, only 2% of the resources obtained by agricultural credit were invested in post-harvest processing, and other 16% were destined to the purchase of machinery.
Regarding PI 2, this had a positive but statistically non-significant effect with high SE value (35%) obtained. Therefore, for this index, accurate results are not obtained that allow affirming the causal effect of agricultural credit in the labor productivity. According to CNA, approximately 20% of the resources obtained from the granting of agricultural credit is used to pay the labor, the third highest items in which it is invested (32% is invested in inputs and 20% in installation of the crop). To improve PI 2 with the existing labor, the employee should receive training and use tools that allow optimize work such as specialized machinery or the application of best agricultural practices.
Relating the results obtained between the two productivity indices, it is found that if there is no significant increasing in PI 2 with the increase in PI 1 when a credit is obtained, it is probably because the employees continue to have the same performance generating a low variation in the PI 2. For this reason, especially in this case, it can be said that PI 2 is an indicator that the farmer would be working inefficiently, that is, the human resources are not being optimized.
At this point it should be noted that the Colombian rural labor market presents great challenges related to increasing the quality of jobs, increasing formality, and female participation (Parra-Peña, Puyana, and Yepes Chica, 2021). All these factors mentioned, play an essential role in improving agricultural productivity. According to Otero-Cortés (2019) during the 2010-2019 period in rural areas, the labor informality has rates significantly higher than urban ones; female labor participation continues very low in rural areas compared to that of men and the unemployment rate for them is higher than in the capitals; on the other hand, child labor continues to present high levels.
Dulal and Kattel (2020) mention another important point of view that should be noted within this research, they in their study carried out in Nepal affirm that there were no more opportunities to increase banana production by investing in land preparation, labor, and fertilizers, instead, suggest that an insurance scheme helps improve banana production and income, as they make farmers take risks, market more and seek more business opportunities. For the present study, this information was not accessed, but it is exhorted that the entities that oversee granting credits or financing, offer a suitable insurance scheme, as well as to raise awareness in the producer of the benefits of this.
Plantain productivity
As can be seen in Table 6, for PI 1 a negative effect that suggest a decrease in productivity equivalent on 0.08% was found, and for PI 2 a positive coefficient interpretated as a productivity increase of 19.13% was obtained; however, both were non-significant results. Therefore, there is not enough statistic evidence to demonstrate any effect of access to agricultural credit in the indexes evaluated for this crop.
Table 6 Results of propensity score matching for plantain crops applying 4-nearest neighbor matching and its standard error.
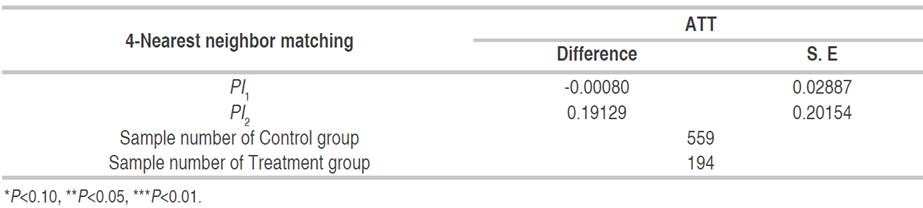
As stated in the introduction, plantain crops currently work as a crop destined for national consumption with important growth projections, however, the results obtained have shown that the financing is not generating positive results in the productive improvement of plantain in the farmers from Valle del Cauca. For his part, Jesús Alberto Rodríguez Paz, (2020) points out in his work on the impact of agricultural credit on productivity at the national level, that some negative results may be since farmers in Colombia allocate the resources obtained to maintain the crop and not to improve the productivity.
According to CNA data, the plantain producers use the resources mainly to pay for agricultural inputs, labor, and installation of crops. However, no investment has been made in agricultural infrastructure or post-harvest processes, variables that in practice have been considered essential to increase productivity. There are some factors that influence decision-making on the advisability of investing in the variables mentioned above, such as specialized advice, knowledge, the farmer's openness to new technologies, among others.
The negative and indifferent effect of credit on productivity may also be due to the time the survey was carried out, since the crop could be in sowing, and only until the time of harvest are the results of the investment expected to be obtained, as is explained by Echavarría et al, (2017) who in their study at the national level, found positive and significant effects of credit in transitory or short-cycle crops and a negative effect in permanent and annual crops.
On the other hand, Dépigny (2019) also highlight that the high cost of crop plantain is particularly due to the necessary inputs, this is considered by farmers as one of the main reasons for the low success of the crop. In the case of plantain producers in Valle del Cauca, around 35% of the resources obtained through agricultural financing are used for agricultural inputs (seeds, fertilizers, insecticides, pesticides).
Finally, it should be noted that in Valle del Cauca only 13% of Musaceae producers apply for credit, even though 87% are approved by some financial entity, which indicates that the main problem is not access to credit specifically. The difficulty may rather lie in the conflicts over land in Colombia, which can be summarized as: armed conflict, land with an agricultural vocation dedicated to other activities, and social inequality (Deininger, Castagnini, and González, 2013).
CONCLUSION
The impact of agricultural credit on the productivity of Musaceae crops in Valle de Cauca Colombia was analyzed. For banana crops, a positive and significant effect of credit on the tons produced per hectare was obtained (increase of 8.4%), however, significant results regarding the effect of credit on tons produced per employee were not found. Relating the results found from PI 1 and PI 2, it can be concluded that the farmer has not optimized and managed the available human resource. On the other hand, there was no statistical evidence of an increase or decrease in plantain productivity as consequence of the credit access.
Considering the results of the agricultural census, the investment of agricultural credit in both crops differs mainly in two items:
The low percentage of investment in post-harvest processes in plantain (0.6%) while in banana it was 2%.
There is 4% more investment in inputs in plantain crops than in banana crops.
As mentioned in the analysis of results, when agricultural credit has a maintenance approach and not productivity improvements, it has a direct impact on the results obtained; however, considering the Valle del Cauca banana market and the differences in investment of the credit in each crop, can be the reasons for the difference in the results obtained for both crops, however, is invited to develop researches that consider the effects of the credit programs on agricultural productivity when is investing in specific items.
As the impact evaluations are valuable tools for the design of good public policies, helping to reveal their quality and effect. The most important contribution of this research was to reveal the impact of accessing agricultural credit in Musaceae producers from Valle del Cauca, considering two transcendental productive factors: land and labor. In addition, it was shown what items were being invested in once accessed it and the openness that the farmer had to agricultural credit. In other words, through this research was understanded the investment priorities of the farmer and the rate of participation in said credit programs, demonstrating that despite the high rate of credit granting compared to the low participation, it opens the door to the discussion of the weaknesses of financing policies to reach all farmers. In addition, with the results obtained once again, the high costs of inputs that the farmer faces and that prevent investing in other items that allow them to increase productivity efficiently are demonstrated.
Additionally, it is invited to compare the impact of credit programs on agricultural productivity of other crops of departmental and/or national interest with different methods and approaches. Moreover, it is considered important to take into account how credit programs are designed and its target population.