1. Introduction
In the context of the mobility and physical interaction restrictions implemented in response to the COVID-19 pandemic, digitization and automation in labor markets accelerated sharply. In particular, 2020 was characterized by the massive and abrupt implementation of the telework modality (remote work), which allowed continuity in certain jobs while reducing the health risk (ECLAC et al., 2020;WEF, 2020). It is clear then that the viability of teleworking is a key determinant of the economic and distributional impacts of a pandemic such as COVID-19 since those able to transfer their tasks to the digital realm might mitigate the impact of the shock by keeping their jobs and labor income (European Commission, 2020; Mongey et al., 2020).
This paper analyzes how the massive and abrupt adoption of teleworking has mitigated the impacts of the COVID-19 pandemic on the Argentine labor market by using the rotating panel structure of the Permanent Household Survey (EPH). Given that the use of teleworking before the pandemic is a poor indicator of how many jobs were able to adopt this modality in 2020, it is convenient to estimate the potential (or viability) of teleworking for occupations based on their characteristics. However, since there is no information on the composition of tasks and the work environment of occupations in Argentina, we estimate the potential for teleworking based on three different surveys: Occupational Information Network (O*NET), Program for the International Assessment of Adult Competencies (PIAAC), and Skills Towards Employability and Productivity (STEP), and then extrapolated it to the classification of occupations in Argentina.
We conducted two exercises to validate the different measurements of teleworking potential obtained. First, we found that the access and use of Information and Communication Technologies (ICT) are associated with an increase in the potential for teleworking. Second, the conditional correlation between the potential for teleworking and an indicator variable of whether the individual works at home is practically nil before the pandemic, but it becomes highly significant as of the second quarter of 2020. Overall, these results suggest that our estimates of teleworking potential are a good indicator of teleworking viability in Argentina.
Given this, it is possible to analyze how the viability of teleworking relates to job transitions and other labor market outcomes at the individual level. Although it is not possible to estimate causal effects, the identification strategy is based on two hypotheses. First, we measure the differential impact over time that the teleworking potential had in 2020. In particular, the teleworking potential should not be significantly correlated with changes in the labor market in “non-pandemic” periods. On the contrary, the mitigator effect would be expected to be relatively greater in those months when the restrictions and distancing policies were more severe due to the health situation. Second, we exploit the fact that the mitigator effect of teleworking potential should be minor (or null) for those jobs declared as “essential” during the pandemic.
We found that, for the period of peak restrictions (the second quarter of 2020), the potential for telework is positively correlated with the probability of being active and with the probability of being employed. These effects are greater in magnitude in the subsample of “non-essential” workers. In contrast, we found no systematic effects of teleworking potential in employment transitions between 2017 and 2018 and between 2018 and 2019 (“non-pandemic” periods), a reassuring result consistent with our identification strategy.
In this way, we contribute to the prolific literature that analyzes the economic consequences of the COVID-19 crisis, being the first empirical approximation of how the massive and abrupt implementation of teleworking has mitigated the impacts on the labor market of an unexpected shock of such magnitude, in the context of a developing country like Argentina. Furthermore, one contribution of this paper can be found in its use of panel data that consistently tracks the labor market transitions of the same individuals. It builds upon literature that measures the viability of teleworking by comparing estimates based on different skills surveys in the labor market.
The remainder of the work is organized as follows. In Section 2, we carry out a literature review. In Section 3, we discuss the data sources and validate the teleworking potential measurements. Likewise, we analyze the distribution of these measurements in various demographic and labor variables. In Section 4, we discuss some stylized facts regarding restrictions, activity level, and employment. Then, in Section 5, we analyze the relationship between teleworking and the Argentine labor market outcomes during the pandemic. The conclusions are presented in Section 6.
2. Literature review
This document contributes to various strands of literature. First, we contribute to the literature on the impacts of crises on labor markets (Fallon et al., 2002; Hoynes et al., 2012, Verick, 2011) and, in particular, in Argentina (McKenzie, 2004; Corbacho, 2007). Second, this paper is part of the prolific literature that analyzes the economic consequences of the crisis generated by the COVID-19 pandemic (Cajner et al., 2020; Hassan et al., 2020; Lewis et al, 2020; Coibion et al., 2020; Koebel & Pohler, 2020).
Third, we also contribute to the literature that measures the viability of teleworking in response to the shock posed by the pandemic. Telework refers to the performing of work tasks from a place outside the employer's home by using ICT. According to Weller (2020), the proportion of employment that can be carried out remotely depends on four factors: (i) the characteristics of the occupations; (ii) the characteristics of the productive structure; (iii) the level of informality; and (iv) the technological infrastructure, access to it, and the proportion of workers with the necessary skills.
Usually, the empirical literature restricts the analysis to the first point, that is, to the study of the characteristics of the tasks and the work environment of each occupation to determine the compatibility with remote work (Barbieri et al., 2020; Dingel & Neiman, 2020; Avdiu &Nayyar, 2020; Mongey et al., 2020; Leibovici etal., 2020).2
For example, remote work becomes practically impossible for those jobs that frequently require physical interaction with others, the use of machinery in a specific place, or working outside or onboard a vehicle. On the other hand, activities such as telephone assistance, data processing, or computer programming could easily be carried out by teleworking.
An influential paper in this literature is Dingel & Neiman (2020), in which the authors determine the feasibility of remote work in the US for more than 900 occupations. They estimate that 37% of jobs could be carried out remotely. However, since their estimate only considers factors that make teleworking impossible but does not consider factors that "hinder" its implementation, the authors suggest that their measurement is an upper limit of the feasibility of remote work.3
Building upon Dingel & Neiman (2020), various authors have replicated the exercise by extrapolating the teleworking potential of US occupations to other countries (Garrote Sánchez et al., 2020; Guntin, 2020). For Argentina, Albrieu (2020) and Bonavida Foschiatti & Gasparini (2020) estimate that between 26% and 29% of those employed are in occupations that could be carried out remotely (“teleworkable”). Likewise, this literature usually finds that teleworking potential is positively correlated with age, educational level, income, and job formality.
The main critique that these studies have received is that the content of the tasks varies depending on the development level and, therefore, it would not be correct to extrapolate estimates based on the United States to other countries, particularly emerging countries (Dicarlo etal, 2016; Lo Bello etal., 2019). Following the strategy of Dingel & Neiman (2020), Saltiel (2020) and Gottlieb et al. (2020) estimate the potential for teleworking in developing countries using occupational information from the same countries from the STEP surveys of the World Bank. The authors find that 12.9% of the workers in the sample could perform their work remotely, and there is substantial heterogeneity between countries. Hatayama et al. (2020) also use skills surveys to estimate the teleworking potential for a diverse set of 53 countries and find that their estimates positively correlate with Dingel & Neiman's (2020).
Some papers empirically estimate how the massive implementation of teleworking has mitigated the impacts of the pandemic on labor markets. Using the US Current Population Survey, Mongey et al. (2020) find that “excess” job losses, defined as the change in employment between February and March 2020 in relation to the average change between February and March from 2010-2019, suggest that occupations with a low potential for teleworking saw greater declines. Using real-time surveys in March and April 2020, Adams-Prassl et al. (2020b) studied the immediate impacts of the pandemic on the labor markets of the US, Great Britain, and Germany. In particular, the authors include in the questionnaire a question about the proportion of tasks that workers could do from home in their current or previous employment. They find that those who can do only a small part of their tasks remotely face a greater chance of losing their jobs and suffering income losses. However, their surveys do not follow the same individuals but rather include independent cross-sections. In this sense, this paper is the first of this type of empirical estimation for a developing country such as Argentina, and, unlike previous studies, it uses panel data that consistently follows the labor market transitions of the same individuals.
3. Data and sources of information
The main data source used in this study is the EPH microdata from the National Institute of Statistics and Censuses (INDEC). Specifically, we use the rotating panel scheme of the EPH4, identifying the subsample of individuals employed in 2019 who are interviewed again in 2020. In order to make year-on-year comparisons concerning the same period, the analysis is limited to those observed in 2019 that reappear in the same quarter of 2020.5 Additionally, we restricted the sample to those between 25 and 64 years of age in 2019 to avoid the influence of education and retirement decisions on labor participation.
A key input for the analysis is the measurement of the viability of remote work. Given that there is no information on the content of tasks and the work environment of occupations in Argentina, we adopt a strategy similar to that used in Albrieu (2020) and Bonavida Foschiatti & Gasparini (2020), estimating the teleworking potential of occupations based on skills surveys in the labor market of other countries following the methodology of Dingel and Neiman (2020). We will use three alternative surveys: (1) O*NET; (2) PIAAC; and (3) STEP. In comparative terms, (2) and (3) have the advantage of including economies with different levels of development in relation to (1), which is only for the US. However, the information on the task content and context work that arises from (1) is relatively more extensive and periodically updated, unlike (2) and (3).
In all three cases, the implicit assumption is that the characteristics of the occupations surveyed are similar to those of Argentina. This assumption would be incorrect if the same occupation in one country and another differ, for example, in the physical interaction they require, the technological intensity of the production process, the available infrastructure, etc. (Dicarlo etal, 2016; Lo Bello et al., 2019). Indeed, different levels of development are likely to crystallize into differences in these aspects. However, the comparison of measurements estimated in countries with different levels of development may suggest an idea of the bias when varying the composition of tasks of occupations. On the other hand, since other aspects, such as access and use of technological infrastructure, are not taken into account, these estimates should be interpreted as an upper limit of the potential for teleworking.
We first use the O*NET, which contains descriptions of more than 900 US occupations.6 The estimation strategy follows the methodology of Dingel & Neiman (2020), who determine the feasibility of remote work for US occupations using said survey. In particular, the authors use two questionnaires to classify occupations as compatible with remote work, one associated with the tasks that occur at work and the other on the physical and social factors of the work context. For example, teleworking becomes practically impossible for those jobs that frequently require physical interaction with others, the use of machinery in a specific place, working outside or onboard a vehicle. On the other hand, activities such as telephone assistance, data processing, or computer programming could easily be carried out remotely. Dingel & Neiman (2020) determine that an occupation is unfeasible for remote work if at least one of the conditions that would make it impossible is frequently observed.7
Second, we use the PIAAC survey conducted by the OECD in 35 countries. It is important to note that several of these economies present levels of development similar to those of Argentina (see Figure 1). Between 2011 and 2017, when these surveys were conducted, Argentina's average GDP per capita was similar to those evidenced by other countries such as Kazakhstan, Chile, Turkey, and Russia. Therefore, it may be much more reasonable for this group of countries to assume homogeneity in the composition of occupation tasks. Consequently, the potential for telework will be estimated based on the complete sample of 24 countries and a subsample that only considers the non-advanced economies of PIAAC.8 Regarding the information used to estimate teleworking potential, PIAAC has relatively less information than O*NET. Following Hatayama et al. (2020), among the available questions, we selected similar ones to those used by Dingel & Neiman (2020) in the O*NET.9 These include the frequency of doing physical work, using email, and interacting with the public by selling products or services.
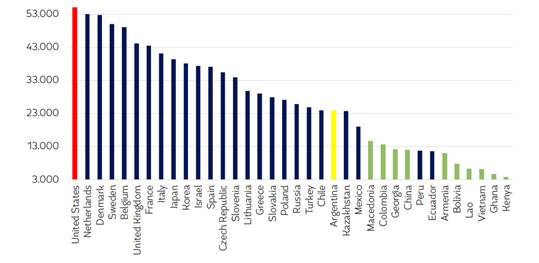
Own elaboration. The figure shows each country's average GDP per capita (constant 2011 international dollars in PPP) between 2011 and 2017. In green are the STEP countries; in blue, the countries in PIAAC; in yellow, Argentina; and the United States in red. The PIAAC and STEP samples are limited to those countries with occupational information at the ISCO level. The information from China in STEP refers to Yunnan Province.
Figure 1 Countries in O*NET, PIAAC and STEP
Finally, we also use the STEP survey carried out by the World Bank in 17 low- and middle-income countries.10 Unfortunately, Argentina has a GDP per capita much higher than that of the countries included in the STEP (see Figure 1), so it is well outside the income range that would be appropriate to extrapolate the teleworking potential based on these surveys (Gottlieb et al., 2020). However, the potential for telework will be estimated based on the complete sample and a second sample that only considers the STEP countries with the closest level of development to Argentina: Colombia and Macedonia. Unfortunately, for the latter, the small number of observations may represent a problem in terms of the precision of the estimates. STEP also has less information than O*NET. Following Saltiel (2020), we select those questions analogous to those used by Dingel & Neiman (2020) in the O*NET. These include the frequency of heavy lifting, repairing electronic equipment, operating heavy machinery, using a computer, and the intensity of contact with co-workers or clients.
In the three surveys, the objective is to add the estimate at the 2-digit level of the International Standard Classification of Occupations (ISCO), which has a correspondence with the two digits National Classification of Occupations (CNO) of Argentina constructed by INDEC. In the first case, O*NET classifies US occupations based on the Standard Occupational Classification (SOC) and provides a mapping between the SOC and the ISCO classifications. Meanwhile, in STEP and PIAAC, we need occupational information at the 2-digit level of ISCO to perform the aggregation.11 Due to the aggregation process between classifications, the application of this strategy results in a continuous variable W. e (0,1) at the level of each CNO occupation, which indicates the teleworking potential measured as the proportion of occupations within each family of occupations that can be performed remotely.12
In short, we have five alternative measurements of teleworking potential. A measurement based on O*NET information (US-O*NET); two measurements calculated from the PIAAC: one that considers the entire sample (PIAAC-24) and one that only includes the non-advanced PIAAC countries (PIAAC-EME); and two measurements estimated based on STEP: one that considers the entire sample (STEP-10) and one based on Colombia and Macedonia, the countries with the closest GDP per capita to Argentina (STEP-2).13 By working at the 2-digit ISCO level, we have 40 occupations. In Figure 2, we present the five variables for each occupation. Among the occupations with the greatest potential for teleworking are professionals and managers; those with the least include assemblers, cleaning personnel, agricultural, forestry, fishing workers, and machine operators. In comparative terms, US-O*NET estimates a greater potential for teleworking for practically all occupations with a measurement greater than 0.2, while for lower levels, it tends to estimate a lower potential than the rest of the variables.
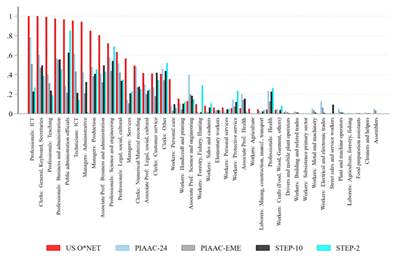
Own elaboration based on O*NET, PIAAC, STEP. The figure shows the estimates of teleworking potential at the 2-digit level of ISCO-08. US O*NET refers to the estimated measurement obtained based on the O*NET survey; PIAAC-24 countries refers to the estimated measurement on the complete PIAAC sample; PIAAC-EME corresponds to the estimated measurement on the non advanced countries of PIAAC; STEP-10 countries refers to the estimate obtained from the complete STEP sample; and STEP-2 countries corresponds to the measurement obtained based on Colombia and Macedonia, the countries with the level of GDP per capita closest to Argentina.
Figure 2 Measurements of the Teleworking Potential
Through the correspondence between the ISCO and the CNO of Argentina, it is possible to impute the estimates of teleworking potential to each individual employed in the EPH. Table 1 shows the correlation between the five estimates at the 2-digit level of ISCO-08 (Panel A) and after imputing the estimates to our EPH panel and using survey weights (Panel B). There is a high positive correlation between the different estimates exceeding 80% in virtually all cases, except for STEP-2. It is worth remembering that this variable is estimated based on only two countries; thus, the observations are much smaller, and it is probably less precise.
Table 1 Correlation between different measurements of teleworking potential
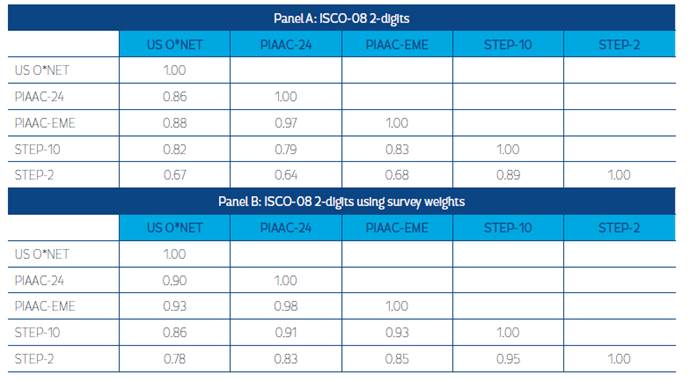
Own elaboration. Partial correlations between the different estimates of teleworking potential. In Panel A, the correlations are calculated at the 2-digit level of ISCO-08, while in Panel B, at the 2-digit level of ISCO08 using the EPH sample weights. US O*NET refers to the estimate obtained based on the O*NET survey; PIAAC-24 countries refers to the estimated measurement of the complete PIAAC sample; PIAAC-EME corresponds to the estimated measurement of the non-advanced countries of PIAAC; STEP-10 countries refers to the estimate obtained from the complete STEP sample; and STEP-2 countries corresponds to the measurement obtained based on Colombia and Macedonia, the countries with the level of GDP per capita closest to Argentina.
3.2 Validation of the teleworking potential measurements
We carried out two complementary exercises to validate the teleworking potential measurements. First, we analyzed the relationship between the potential of telework and the access and use of ICT, which is obtained from the “Module of access and use of information and communication technologies” of the EPH. Specifically, we estimated a multivariate regression of the potential for teleworking in the use and access to the internet and computer equipment, controlling for various labor and demographic variables at the individual level. The results are presented in Table 2, which includes a column for each teleworking potential variable. There is a high correlation between the ICT access and use variables, therefore, the estimates are likely to suffer from multicollinearity problems. Nonetheless, there is a positive relationship between ICT access and use and the potential for telework measured by the five different variables. Specifically, we found that having a computer at home is associated with an increase of 1 pp. on the potential of teleworking. Likewise, having internet access implies an increase in teleworking potential of between 1 and 2 pp., and using a computer is associated with a teleworking potential of between 3 and 8 pp. higher.
Table 2 Correlation between the teleworking potential and access and use of ICT
VARIABLES | (1) | (2) | (3) | (4) | (5) |
US O*NET | PIAAC-24 | PIAAC-EME | STEP-10 | STEP-2 | |
HH has computer | 0.010 | 0.009** | 0.006* | 0.004 | 0.004 |
(0.008) | (0.004) | (0.003) | (0.003) | (0.003) | |
HH has internet | 0.021*** | 0.015*** | 0.010*** | 0.010*** | 0.010*** |
(0.008) | (0.004) | (0.003) | (0.003) | (0.003) | |
Uses internet | 0.005 | 0.001 | 0.001 | 0.001 | 0.002 |
(0.008) | (0.005) | (0.003) | (0.004) | (0.003) | |
Uses computer (excluding internet) | 0.082*** | 0.049*** | 0.038*** | 0.038*** | 0.033*** |
(0.009) | (0.005) | (0.004) | (0.004) | (0.004) | |
Observations | 19,133 | 19,133 | 19,133 | 19,133 | 19,133 |
R2 | 0.490 | 0.471 | 0.468 | 0.462 | 0.465 |
Estimates obtained by OLS. Sample weights are used. Robust standard errors in parentheses. *** p <0.01, ** p <0.05, * p <0.1. The dependent variable is the teleworking potential of the individual's occupation. The variables of access and use of ICT belong to the “Module of access and use of information and communication technologies” of the fourth quarter of the EPH. Control variables include gender, age group, region, educational level, sector of activity, type of company (public, private, other), occupational category (employer, self-employed, formal salaried, informal salaried), size of the company, and income decile of the main occupation. The sample on which the estimates are performed corresponds to the fourth quarter of 2019. US O*NET refers to the estimate obtained based on the O*NET survey; PIAAC-24 countries refers to the estimated measurement of the complete PIAAC sample; PIAAC-EME corresponds to the estimated measurement of the non-advanced countries of PIAAC; STEP-10 countries refers to the estimate obtained from the complete STEP sample; and STEP-2 countries corresponds to the measurement obtained based on Colombia and Macedonia, the countries with the level of GDP per capita closest to Argentina.
Complementarily, we use responses to the EPH questions regarding the place where the worker mainly performs their tasks to construct a binary variable equal to 1 if the individual performs their work at home and zero otherwise. In the second and third quarters of 2020, the percentage of employed persons who worked from home reached 22%, representing an increase of 16 pp. in interannual terms (INDEC, 2020). Although this is not an indicator of telework in itself since, for example, it could be the case of a worker who has the workshop at home, it is expected that the correlation between this variable and the measurement of teleworking potential increases during the pandemic.
As shown in Figure 3, this is the case. The conditional correlation between working from home and teleworking potential is practically nil before the pandemic (from 2019q1 to 2020q1), while it becomes highly significant after the surge of the pandemic in Argentina in the second quarter of 2020.14 An increase of 1 pp. in the teleworking potential of an occupation is associated with an increase of between 0.23 pp. and 0.55 pp. in the probability of working from home in the second quarter, between 0.27 and 0.59 pp. in the third quarter, and between 0.19 and 0.40 pp. in the fourth quarter. The estimate of teleworking potential with the highest correlation with working from home during the pandemic is PIAAC-EME. Taken together, these results and those in Table 2 suggest that estimates of teleworking potential have a high correlation with access to and use of ICT infrastructure and with the probability of working from home during the pandemic; given this, they constitute a good indicator of teleworking potential in Argentina.
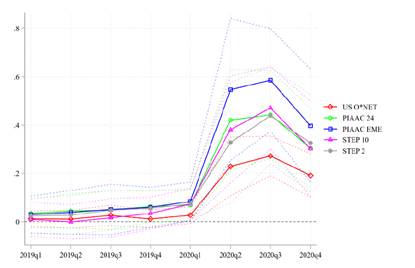
Estimates obtained by OLS. The figure shows the conditional correlation between a dummy variable that equals 1 if the individual works mainly in their home and 0 otherwise and the five teleworking potential indexes defined based on the occupation of individual , by quarter. Sample weights are used. The confidence intervals correspond to a confidence level of 95%. Control variables include gender, age group, region, educational level, sector of activity, type of company (public, private, other), occupational category (employer, self employed, formal salaried, informal salaried), size of the company, and income decile of the main occupation. US O*NET refers to the teleworking potential measurement obtained based on the O*NET survey; PIAAC-24 countries refers to the estimated measurement of the complete PIAAC sample; PIAAC-EME corresponds to the estimated measurement of the non-advanced countries of PIAAC; STEP- 10 countries refers to the estimate obtained from the complete STEP sample; and STEP-2 countries corresponds to the measurement obtained based on Colombia and Macedonia, the countries with the level of GDP per capita closest to Argentina.
Figure 3 Correlation between the measurements of teleworking potential and work from home
3.2 Teleworking potential and observables
To analyze how the viability of teleworking is distributed, we estimate the relationship between the potential for remote work and various labor and demographic variables at the baseline (the year 2019) by estimating the following equation:
where We (0,1) indicates the teleworking potential of the individual's i occupation; X. is a set of demographic and employment characteristics; and e i is the error term. Table 3 presents the results.
First, we find that being a man is negatively associated with the potential for teleworking. In relation to age, the oldest age group (55 to 64 years old) has significantly greater potential for teleworking than those between 25 and 34 years old. In regional terms, those who reside in the northeastern region show a potential for telework greater than GBA, while the opposite occurs with the Patagonia region. There are no statistically significant differences concerning the rest of the regions. A growing monotonic relationship is evidenced regarding education that is statistically significant after completing the secondary level. We also found that those with incomplete and complete primary education have a lower potential for teleworking than those who never entered the educational system. Being employed in an occupation classified as “essential” during the pandemic is not significantly related to the potential for teleworking. Regarding the activity sector and taking Public Administration as the base category, practically all sectors show less potential for telework, except for financial intermediation, where the teleworking potential is relatively higher. The sectors with the lowest potential for teleworking are health, construction, industry, hotels and restaurants, and other services. In terms of the type of company, there is no statistical difference between those who work in public and private companies. However, those who work in other types of companies show a greater potential for teleworking than those who work in a public company. In relation to the occupational category, employers have a greater potential for teleworking than non-employers and, to a greater extent, as compared to informal workers (self employed and informal salaried workers). The potential for teleworking is relatively higher in companies with more than five employees. Finally, we find a growing monotonic relationship between the potential for teleworking and the income decile of the main occupation.
In summary, the teleworking potential is relatively greater for women, the elderly, those with high qualifications, formal workers, and those who work in specific sectors of activity such as public administration or financial intermediation. These results align with previous literature (Mongey et al., 2020; Saltiel, 2020, Guntin, 2020; Hatayama et al, 2020). In addition, these results are in line with the findings of Bonavida Foschiatti and Gasparini (2020), who show that the least teleworkable occupations in Argentina are characterized by a higher proportion of informal workers, with lower levels of education, qualification, and wages. These groups are likely to be the most affected by the impacts of the pandemic by not being able to continue with their work responsibilities from home.
4. Stylized facts
Restrictions on mobility and physical interaction (quarantines and social distancing policies) implemented in response to the COVID-19 pandemic brought economic activity to a standstill around the world. In Argentina, on March 20, 2020, the Aislamiento Social Preventivo y Obligatorio (ASPO) began, which sought to minimize the mobilization of people and lasted until April 26, when territorially segmented measures were implemented. As shown in Figure 4, in March, there was already a drop in activity level, but April was the worst month of the year, with the economy falling 25% year on year. Thus, the most severe drop in activity occurred in the second quarter, when the economy fell 19% compared to the previous year. However, the impacts of the crisis are highly heterogeneous. The most affected sectors are those that require a high degree of physical proximity and therefore suffered the most from the restrictions: food and accommodation, construction, and personal, social, and community services. On the contrary, essential activities such as public administration, electricity, gas and water, health, and primary sectors experienced limited declines. Likewise, non-essential sectors such as education and financial intermediation also showed relatively minor drops in activity level.
The same patterns are observed in the recovery that began at the end of the second quarter and was accentuated in the second part of the year, in tandem with the easing of restrictions (see Figure 5). Some sectors such as food and accommodation, social and personal services, and transportation continued at very low levels due to restrictions on their operations. In contrast, others, such as financial intermediation, electricity, gas and water, commerce, industry, real estate, and business activities, began to reach levels close to those they had before the pandemic. However, certain activities such as education and public administration remain at low activity levels but with moderate drops because they were not fully allowed. Finally, the evolution of the sectors linked to primary activities (mainly fishing) has been quite independent of the supply and demand conditions linked to the pandemic, except for certain restrictions on the operation of the mining sector in March and April (BCRA, 2021).
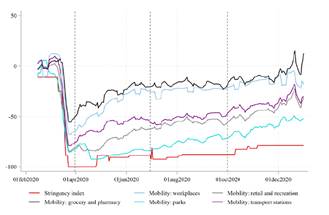
Own elaboration based on Blavatnik School of Government (Oxford University) and Google Global Mobility Trends. The Stringency Index of restrictions peaks at -100 and is a composite measurement of seven indicators that include information on public policy responses such as school closings, transportation bans, border closures, etc. (for more information, see Hale et al., (2021)). A 7-day moving average was calculated on the mobility series. The vertical dotted lines separate the quarters.
Figure 5 Stringency index and mobility
Regarding the labor market, in the second quarter, the employment rate fell 8.8 pp. compared to the first quarter, and 9.2 pp. compared to the same period in 2019. However, unemployment only increased 2.7 pp. compared to the first quarter and 2.5 pp. in interannual terms (INDEC, 2020). The activity rate fell 8.7 pp. in quarterly terms and 9.3 pp. year on year; as a result, the unemployment rate partially reflected the adjustments in the labor market during the pandemic (ILO, 2021). The easing of restrictions in the second semester was associated with an improvement in these indicators. In the third quarter, activity and employment rates increased by approximately 4 pp. compared to the second quarter, although they were 5 pp. below the same period of the previous year. Unemployment decreased 1.4 pp. quarterly, but increased 2 pp. compared to the third quarter of 2019.
When we impute the estimates on the potential for teleworking to our dataset, we found that 30% of those employed in 2019 were in a potentially “teleworkable” occupation (using the estimate of US O*NET), that is, compatible with teleworking (the occupation's teleworking potential is greater than or equal to 0.5). This result is similar to that obtained by Bonavida Foschiatti & Gasparini (2020) and Albrieu (2020) for Argentina. However, the potential for teleworking estimated in this way is much lower if we use the rest of the teleworking potential measurements. For example, according to PIAAC-24, only 12% of those employed in 2019 were in a “teleworkable” occupation.
As shown in Figure 6, the measurement of teleworking potential is positively correlated with the economic performance of the sectors during the ASPO. Except for essential activities, the sectors with occupations with a greater potential for teleworking are those that were able to transfer their tasks to this modality during the pandemic, such as financial intermediation, education, and public administration. The relationship is not clear in the case of primary activities, which is probably due to the fact that the EPH only covers urban areas; likewise, as already mentioned, the evolution of the sectors linked to primary activities has been quite independent of conditions linked to the pandemic.
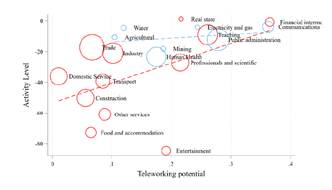
Own elaboration based on PIAAC, EPH, and INDEC. Sample weights are used. The teleworking measurement corresponds to the sectoral average for 2019 of the PIAAC-EME estimate. The activity level is defined as the year-on-year change in gross value added at constant prices in the second quarter of 2020. The size of the circle corresponds to the number of employed persons. The color blue (red) indicates sectors where more than (up to) 75% of employment is categorized as (not) essential during the pandemic. The dotted lines correspond to a regression of the change in the activity level in the teleworking potential by activity sector, for essential (blue) and non-essential (red).
Figure 6 Teleworking potential and economic activity
5. Empirical strategy and results
The empirical strategy consists of estimating a certain outcome variable as a function of the potential for teleworking and other control variables as follows:
where y i,q is the value of a certain outcome variable of the individual i in the quarter q of 2020 who was employed in the quarter q of 2019. The vector X i is a set of control variables at the individual level that includes gender, age, educational level, and covariates related to the occupation defined in the initial period, including the activity sector, the seniority of the position, the type and size of the company, the occupational category, and the income decile of the main occupation. Additionally, an indicator variable is included, essential i,q , that is equal to 1 if i is an “essential worker” who was thus not subject to restrictions during the social isolation measures.15 Finally, time fixed effects by quarter, T q , and regional fixed effects by urban area u r are included; and u, q is the unobservable error term.
The explanatory variable of interest is We (0,1), which refers to teleworking potential as defined by the occupation of individual i in quarter q of 2019. There are five estimates for this variable, which were described in Section 3, and represent a degree of each individual's exposure to the generalized shock that the pandemic represented.16 Those employed in occupations with the greatest potential for teleworking were likely to have been less exposed to loss of employment or earnings during the crisis.
Although we include a wide variety of covariates to avoid selection bias in observables, it is not possible to make a causal interpretation of these estimates since there may still be selection in unobservables. However, to support the identification strategy, W i is interacted with quarterly dummies based on the hypothesis of temporal heterogeneity of the effect of teleworking on labor market outcomes. First, the potential for teleworking should not be significantly correlated with changes in the labor market in “non-pandemic” periods (the first quarter of 2020). Second, the effect of teleworking should be greater in periods where restrictions have been relatively more severe. In this way, K7 indicates the expected change in y i,q in the face of an increase of 1 pp. in teleworking potential, in quarter q.17 In our dataset, those interviewed in the first quarter of 2019 and observed again in the first quarter of 2020 represent a placebo group. If the hypothesis is correct, the interaction term of the teleworking potential with the dummy for the first quarter of 2020 should not be statistically significant.18 On the other hand, due to the relaxation of restrictions in the second part of 2020, it is expected that the interaction coefficient with the second quarter will be greater in magnitude than those of the third and fourth quarters. In short, it is expected that Y2 > Y" > Y# > Y$.
The second hypothesis of the identification strategy is that we expect to find minor (or null) effects of teleworking potential in the subsample of workers declared “essential” during the pandemic since they were not subject to restrictions on mobility and physical interaction. Therefore, we estimate equation (2) on different subsamples according to the classification of essential activities during the COVID-19 pandemic in Argentina.
The dependent variables considered are: (i) the probability of being employed; (ii) the percentage change in hours worked in the main occupation; (iii) the percentage change in income from the main occupation; (iv) the percentage change in the hourly income of the main occupation; (v) the probability of being unemployed; and (vi) the probability of being active19. Except for (ii) - (iv), we estimate both linear probability models (MPL) and logistic models.
Descriptive statistics of both the dependent variables and the estimates of teleworking potential are reported in Table 4. Of those employed in 2019, 86% kept their job, 4% lost it, and 10% went into inactivity. On average, the hours worked fell 5% between 2019 and 2020. For those who kept their job in 2020, labor income grew in constant terms by 7%, while hourly labor income grew by 20%.21 On average, teleworking potential is the highest as measured by US O*NET, followed by PIAAC-24.
We cluster the standard errors at the ISCO-08 2-digit occupation level, that is, at the same level as the teleworking potential variable, to consider the potential correlation between unobservable characteristics of individuals employed in the same occupation. Alternatively, the estimates were repeated using robust standard errors, and the significance levels are practically identical and are available if necessary.
Table 4 Descriptive statistics
N | Mean | sd | min | p25 | p50 | p75 | max | |
Dependent Variables | ||||||||
Employed | 17,118 | 0.86 | 0.35 | 0 | ||||
% hours worked | 13,944 | -0.05 | 1.05 | -1 | -0.40 | -0.07 | 0.10 | 47.00 |
% labor income | 13,337 | 0.07 | 1.07 | -0.28 | -0.07 | 0.17 | 41.99 | |
% hourly labor income | 11,014 | 0.20 | 1.39 | -1 | -0.29 | -0.03 | 0.34 | 57.75 |
Unemployed | 17,118 | 0.04 | 0.19 | 0 | 0 | 0 | 0 | |
Active | 17,118 | 0.90 | 0.30 | 0 | 1 | 1 | 1 | 1 |
Teleworking potential scores | ||||||||
US O*NET | 17,118 | 0.32 | 0.40 | 0 | 0 | 0.08 | 0.80 | 1 |
PIAAC-24 | 17,118 | 0.20 | 0.22 | 0 | 0.02 | 0.05 | 0.40 | 0.78 |
PIAAC-EME | 17,118 | 0.14 | 0.17 | 0 | 0.01 | 0.03 | 0.31 | 0.55 |
STEP-10 | 17,118 | 0.16 | 0.17 | 0 | 0.01 | 0.06 | 0.24 | 0.63 |
STEP-2 | 17,118 | 0.14 | 0.16 | 0 | 0.01 | 0.11 | 0.23 | 0.85 |
Descriptive statistics of the dependent variables and of the five estimates of teleworking potential. Sample weights are used. US O*NET refers to the estimate obtained based on the O*NET survey; PIAAC-24 countries refers to the estimated measurement of the complete PIAAC sample; PIAAC-EME corresponds to the estimated measurement of the non-advanced countries of PIAAC; STEP-10 countries refers to the estimate obtained from the complete STEP sample; and STEP-2 countries corresponds to the measurement obtained based on Colombia and Macedonia, the countries with the level of GDP per capita closest to Argentina.
5.2 Results
For ease of reading, the results discussed below are included in the appendix. The results of estimating equation (2) for the complete sample are presented in Table 5. Specifically, we analyze the relationship between different outcome variables and the potential for teleworking during the COVID-19 pandemic in Argentina using the subsample of individuals employed in 2019 and interviewed again in the same quarter of 2020. We use five different estimates of teleworking potential that were described in Section 3. Therefore, Table 5 includes five panels where the coefficients of interest are reported.22
Considering the estimates of the OLS models, during the second quarter of 2020 (second row of each panel), an increase of 1 pp. in the potential for teleworking implied an increase in the probability of being employed of between 0.11 and 0.27 pp. (column 1). There is no statistically significant effect on hours worked (column 3). In terms of income, an increase of 1 pp. in the potential of teleworking implied an increase in the growth rate of labor income of between 0.21 and 0.43 pp. (column 4) and for hourly labor income between 0.35 and 0.64 pp. (column 5). On the contrary, the potential for teleworking was negatively associated with the probability of being unemployed (column 6). Finally, we found that an increase of 1 pp. in the potential for teleworking implied an increase in the probability of being active of between 0.08 and 0.21 pp. (column 8).
On the other hand, the coefficients of the interaction term with the third quarter (third row of each panel) are, in general, not statistically significant. However, the effect on labor income is statistically significant and larger in magnitude. In relation to the interaction term with the negative effects on employment and labor participation. Meanwhile, in practically all the estimates, we found that telework is inversely related during the first quarter (first row of each panel) in relation to the second quarter. Although the initial hypothesis suggested that this interaction should not be statistically significant, the change in the sign indicates that before the pandemic, there was a negative trend in employment and labor participation in occupations with high teleworking potential.
The results obtained using non-linear models, in general, are similar, although smaller in magnitude. For example, in the second quarter, an increase of 1 pp. in the potential for teleworking implied an increase in the probability of being employed of between 0.07 and 0.18 pp.
It is interesting to note that the coefficient of teleworking potential in the second quarter takes its minimum value when using the variable estimated from US data (US O*NET), while the maximum is obtained when using the variable considering the countries not advanced in PIAAC, which have a similar level of development to Argentina and therefore it is more reasonable to assume homogeneity of the composition of tasks between countries. Also, note that PIAAC-EME is the variable whose correlation with working at home is the highest (see Figure 3).
Table 6 statistically evaluates the differences between the coefficients of the interactions and confirms what is expected based on the identification strategy: Y2 > Y" > Y# > Y$. First, this suggests that due to the pandemic, the job profile of occupations with the greatest potential for teleworking changed dramatically between the first and second quarters of 2020, experiencing positive changes in activity, employment and income rates. Second, these effects tend to be diluted with the easing of restrictions during the second half of 2020.
To provide additional support to the identification strategy, Table 7 repeats the estimates in Table 5 for different subsamples based on the classification of essential activities during the COVID-19 pandemic in Argentina. We found that the mitigator effect of teleworking on employment and activity is greater in magnitude in the subsample of non-essential workers. Likewise, these effects are concentrated in the second quarter of 2020, when the restrictions were more severe. For example, in Panel A, an increase of 1 pp. in the potential for teleworking implied a 0.15 greater probability of remaining employed for non-essential workers, while 0.07 pp. higher for essential workers. On the other hand, the respective figures regarding the probability of being active are 0.10 pp. and 0.04 pp., the latter being not significant. Furthermore, the teleworking potential was associated with an increase in hours worked and in labor income, and with a negative effect on the probability of being unemployed only in the subsample of non-essential workers. Finally, the teleworking potential has a positive effect on hourly labor income for essential workers.
5.3 Placebo experiment
Table 8 repeats the exercise of estimating equation (2), but for the two years before the pandemic, a period for which finding significant effects of teleworking potential on the results of the labor market would not be expected. In particular, we evaluate the changes between 2017 and 2018 and between 2018 and 2019. We found no systematic effects of teleworking potential on employment transitions and labor participation. However, there are positive effects on income, which could be associated with the fact that occupations with greater teleworking potential are typically characterized by being formal jobs with high incomes and in sectors associated with technology and financial activities. These results represent additional evidence supporting the strategy to identify the mitigator effect of teleworking on employment and labor participation during the COVID-19 pandemic. At the same time, the effects of teleworking potential on earnings during the pandemic should be taken with caution.
5.4 Robustness checks
Four sensitivity exercises are carried out to guarantee the robustness of the results. First, as already mentioned, the sample is restricted to individuals employed in 2019 and interviewed again in the same quarter of 2020. The estimates would include some bias brought on by those individuals who changed their occupation (and teleworking potential) between 2019 and 2020. However, most of those observed remained in the same sector of activity. It was found that 21% of those employed changed their sector of activity between 2019 and 2020. As a robustness exercise, we repeat the baseline estimates but exclude these observations. Second, we replicate the estimates by eliminating the individuals who in 2019 were employed in primary activities (agriculture, mining, and fishing) since these sectors had an independent evolution of the supply and demand conditions linked to the pandemic. Third, in all estimates, standard errors were clustered at the ISCO-08 2-digit level. However, since some individuals may appear twice in the dataset, and since a household may include more than one worker, estimates are performed by correcting errors at the household level as another robustness exercise. Fourth, the findings shown in the paper are obtained using the sample weights corresponding to the year 2020. However, certain analysts have expressed concerns regarding the non-response adjustments made by the INDEC on these weights. For this reason, we repeat the estimates using the 2019 sample weights. Table 10 presents the results for the four robustness exercises. Additionally, in Panel A, the results of Table 5 (panel C) are reported for comparison. 23In general terms, the results remain practically unchanged regarding estimated coefficients and levels of significance.
5.5 Heterogeneous effects 24
As can be seen in Figure 7, restrictions on mobility evidenced great regional heterogeneities. Provinces such as CABA or Buenos Aires had very severe and prolonged quarantines, while in others such as Catamarca or Entre Ríos the restrictions were laxer, and after the initial drop at the beginning of the second quarter, they returned to “normal” mobility indicators. Consequently, to identify regional heterogeneities in the role of telework as a mitigator of the impacts of the pandemic, we estimate equation (2) for different geographical subsamples based on the level of “stringency” of the restrictions imposed in the face of the pandemic. Considering the changes in mobility to work, Argentine provinces are classified according to the de facto severity of quarantines in each quarter. Specifically, those provinces whose median decline in mobility is greater (in absolute terms) than the quarterly national median are classified as severe quarantine. In the second quarter, this group includes Buenos Aires, CABA, Neuquén, Río Negro, and Tierra del Fuego. In the third quarter, this group includes Buenos Aires, CABA, and Jujuy. And in the fourth quarter, it includes Buenos Aires, Chubut, CABA, Neuquén, and Tierra del Fuego.
The results for the geographic subsamples based on the severity of the restrictions can be found in Table 11. The potential for teleworking was positively correlated with the probability of being employed and active during the second quarter of 2020, but effects of greater relative magnitude are evidenced in the subsample of severely quarantined regions. For example, an increase of 1 pp. in the potential of teleworking implied an increase in the probability of remaining employed of 0.33 pp. in provinces with severe quarantines, while 0.17 pp. greater in light quarantine provinces. The difference is similar in relation to the probability of remaining in activity (0.26 pp. vs. 0.11 pp.). Meanwhile, the potential for teleworking was associated with a lower probability of being unemployed only in light quarantine regions. In terms of labor income, we found an effect in both subsamples, although it was larger in those with severe quarantines and significant for hourly labor income only in provinces with less severe quarantines.
Finally, to analyze the existence of heterogeneous effects at the individual level, we estimate equation (2) on different subsamples by gender and educational level. In terms of the latter, the sample is divided among those who, at least, have reached the incomplete higher education level (high qualification). As seen in Table 12, an increase of 1 pp. in the potential for teleworking implied a statistically significant increase in the probability of remaining employed, of 0.27 pp. and 0.26 pp. for women and men, respectively. Teleworking had a positive impact on labor income in both subsamples. Finally, for both, there is a significant effect on the probability of being active of 0.19 pp., and a negative effect on the probability of being unemployed only for women. Concerning the subsamples by educational level (Table 13), it is found that the positive effects of telework on employment and the activity rate are greater for those with a lower level of qualification; this group faced a probability of being employed that was 0.31 pp. greater, and a probability of being active that was 0.27 pp. greater. Meanwhile, for those with high qualifications, we found that an increase of 1 pp. in the potential for teleworking was associated with a 0.20 pp. greater probability of being employed and 0.10 pp. greater probability of being active, with the latter not being statistically significant. There are significant effects on income in both subsamples.
5.6 Correction of teleworking potential for access and use of ICT
One of the main limitations in implementing teleworking is access to ICT infrastructure. Garrote Sánchez et al. (2020) find that teleworking potential estimates are significantly reduced when considering internet access, particularly in low-income countries where internet access is relatively lower. The fourth quarter of the EPH includes a module containing information on ICT access so that the potential for telework could be corrected by taking this dimension into account. Specifically, those individuals whose households do not have internet or do not have a computer are assigned a zero in the corresponding teleworking potential measurement. Being only for the fourth quarter, the specification equals equation (2), but the teleworking potential is included without interacting. The results are presented in Table 14. There are no statistically significant effects on employment and labor participation, except for a positive effect on employment when using the STEP-2 variable. We found statistically significant effects on earnings with the five estimates of teleworking potential. It is important to bear in mind that for the base estimates, the interaction of teleworking potential with the fourth quarter, in general, is not statistically significant either. Even so, taking ICT access into account is crucial for a better estimate of the viability of teleworking in Argentina.
5.7 Attrition
There is a potential problem that can affect the estimates: attrition. This is the “breakdown” of the sample because some people are not surveyed again because they were not found in the same household or they did not want to answer. If there were a correlation between this loss of information and the potential for teleworking, this could bias the estimates. This loss of information is common in the rotating panel of the EPH and is usually around a third of the sample, but the pandemic likely exacerbated the problem. Indeed, 15,803 employed individuals were interviewed in 2019 and did not reappear for the 2020 interview. This figure accounted for 42% of the 2019 panel.
To assess whether there is a potential attrition problem, we estimate a regression of the probability of “disappearing from the panel in 2020” as a function of teleworking potential and various covariates. The dependent variable, then, equals one if individual i in quarter q of 2019 did not appear in quarter of 2020 and should have done so given the rotating panel structure of the EPH. As shown in Table 15, the relationship between teleworking potential and the probability of disappearing in 2020 is negative and statistically significant. An increase of 1 pp. in the potential of telework implied a fall in the probability of disappearing in 2020 of between 0.02 and 0.05 pp.
A plausible hypothesis behind this result is that those who dropped out of the survey in 2020 had relatively “worse” jobs, for example, informal ones, with less potential for teleworking, and faced a greater likelihood of losing their job and labor income during the pandemic. Consequently, if included in the survey, estimates of the mitigator effect of the potential for telework are likely to be greater in magnitude.
Table 15 Probability of disappearing from the panel in 2020
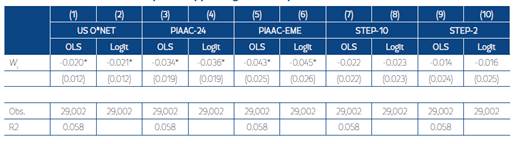
Estimates obtained by OLS (odd columns) and logistic model (even columns). Sample weights are used. The results of the logistic models are presented in terms of marginal effects. Cluster-corrected standard errors at the 2-digit level of ISCO-08 are in parentheses. *** p <0.01, ** p <0.05, * p <0.1. The dependent variable is a dummy that equals 1 if individual in quarter q of 2019 did not appear in quarter q of 2020 and should have done so given the rotating panel structure of the EPH. We (0,1) refers to the teleworking potential defined based on the occupation of individual i in quarter q. The estimates are performed on the subsample of individuals who were employed in 2019 and who are (or should have been) interviewed again in the same quarter of 2020. US O*NET refers to the estimate obtained based on the O*NET survey; PIAAC-24 countries refers to the estimated measurement on the complete PIAAC sample; PIAAC-EME corresponds to the estimated measurement of the non-advanced countries of PIAAC; STEP-10 countries refers to the estimate obtained from the complete STEP sample; and STEP-2 countries corresponds to the measurement obtained based on Colombia and Macedonia, the countries with the level of GDP per capita closest to Argentina. Controls include gender, age, educational level, and covariates related to occupation defined in the initial period, including activity sector, job seniority, type and size of the company, occupational category, and the income decile of the main occupation. Additionally, an indicator variable is included that equals 1 if i is an “essential” worker so that it was not subject to the restrictions during the measurements of social isolation. Finally, time fixed effects by quarter and regional by urban area are included.
6. Conclusions
We contribute to the prolific literature that analyzes the economic consequences of the COVID-19 crisis, offering the first empirical approximation of how the massive and abrupt implementation of telework has mitigated the impacts on the labor market of an unexpected shock and magnitude in the context of a developing country like Argentina. A further contribution of this work is the use of panel data that consistently tracks the labor market transitions of the same individuals. Finally, we contribute to the literature that measures the viability of teleworking by comparing estimates based on different skills surveyed in the labor market.
Given that the use of teleworking prior to the pandemic is a poor indicator of how many jobs could adopt this modality in 2020, it is convenient to estimate the potential (or viability) of teleworking for a given occupation based on its characteristics. However, since there is no information on the composition of tasks and the work environment of occupations in Argentina, we estimated the potential for teleworking based on three different surveys: O*NET, PIAAC, and STEP, and then extrapolated it to the Argentine classification of occupations. As an exercise to validate these estimates, we found that they have a high correlation with the access and use of the ICT infrastructure and with the probability of working from home during the pandemic and thus serve as a good indicator of the possibilities of teleworking in Argentina.
From there, it is possible to analyze how the viability of teleworking relates to job transitions and other labor market outcomes at the individual level. We found that, during the peak of restrictions in response to the pandemic (the second quarter of 2020), the potential for teleworking positively correlates with the probability of being active and employed. These effects are greater in magnitude in the subsample of non-essential workers, who were more subject to the restrictions imposed in response to the pandemic. An extensive battery of robustness exercises ensures the sensitivity of the findings.
In contrast, we found no systematic effects of teleworking potential in employment transitions between 2017 and 2018 and between 2018 and 2019 (“non-pandemic” periods), a reassuring result consistent with our identification strategy. However, there are positive effects on income, which could be associated with the fact that occupations with greater teleworking potential are typically characterized by being formal jobs with high incomes in sectors associated with technology and financial activities. Therefore, the effects of teleworking potential on earnings during the pandemic should be taken with caution.
The role of telework as a mitigator has been relatively greater in those geographic regions where restrictions on mobility and physical interaction have been more severe. In terms of gender, teleworking has played a key role in sustaining employment and labor participation for both women and men. Interestingly, we found that the positive effects of teleworking on employment and the activity rate are greater for those with low levels of qualification.
Notably, the potential for teleworking is a significant predictor of all these variables, taking into account that, in addition, it is correlated with a large part of the controls included (for example, educational level, informality, age, sector of activity, etc.) (Mongey etal., 2020; Bonavida Foschiatti & Gasparini (2020); Adams-Prassl et al., 2020a).
Our results are important to understand the critical role of the ability to work from home in the labor market. The pandemic exposed the relevance of promoting digital skills and expanding connectivity. In fact, it is likely that the demand for tech-skilled workers, the ability to change locations if needed, has undergone a permanent shift. This new scenario calls for policies that promote the acquisition of digital skills and facilitate access to fast connectivity, especially among the most vulnerable. As the recent pandemic has shown, these have become essential tools to foster the employability of workers and improve their chances of advancement towards better jobs.