Introduction
For human beings, the most common phenomena in nature, such as the varied landforms and the turbulence in the river, are unpredictable and lack rules. Since Pangu's founding, human beings have been tirelessly exploring this magical and wonderful nature (Bagley et al., 2017). In the process of understanding nature and transforming nature, human beings are uncovering their veil layer by layer to explore their true faces (Cai et al., 2016). It should be said that physicists spend a lot of energy in analyzing the universe and basic particles. With the creation of Newton's classical mechanics, Einstein's theory of relativity, and the development of quantum mechanics, human beings have made brilliant achievements in Natural Science (Liu & Jiang, 2020; Aranguren-Riano et al., 2018). Natural numbers, triangles, rectangles, squares, circles and so on, as practical techniques or tools of mathematics, are gradually created in the development of human social practice, and form arithmetic, algebra, geometry, trigonometry and calculus. Mathematics gradually matures from simple to complex (Yuan et al., 2016). In mathematics, geometry is the first subject to rise from empirical knowledge to theoretical knowledge, and its typical representative is Euclid's "geometric original" (Padisak et al., 2016). It is the first work in the history of mathematics to systematically discuss geometry by using axioms and methods. Since then, for quite a long time, geometry, as the science of describing the form of things, has been regarded as the only real mathematics and the model of scientific rigor (Gu et al., 2018; Hu et al., 2018; Kong et al., 2018; Zhang, 2020).
Euclidean geometry is a subject about intuitionistic spatial shape relations. Its studies ordered, smooth and feature length geometry (Gao et al., 2016). No matter Euclidean geometry, or Cartesian geometry analytic geometry and differential geometry developed later, the lines, curves and surfaces studied by them all have a common assumption that these lines and surfaces are smooth, meet the differentiability, and are abstract. However, the geometry of nature is complex. The points, lines and surfaces of nature are not all in the geometry category of Mathematicians (Riordan et al., 2016). Nature is more irregular, disordered and discontinuous. As long as people pay a little attention to a large number of non-linear irreversible phenomena in the surrounding environment, they will find that people know little about these phenomena and have no idea about many problems. Take the phenomenon that we are used to in the sky as an example. When you look up at the sky, you can often see a group of clouds floating in it, which is poetic (Ozguc, 2018). But if you look at the clouds with different telescopes, you will find that the shape of the clouds seems to have nothing to do with the magnification of the telescope. No matter how large the magnification, its shape is always the same (Li et al., 2017). For non-linear science, classical mechanics, quantum mechanics and relativity are useless. There must be new theories to study these scientific problems that all human wisdom can't solve.
In the mid-s, Mandelbrot, a researcher in the Physics Department of the corporate research center of the United States and a professor in the Mathematics Department of Harvard University, published an article on "how long is the coastline of Britain" in science magazine of the United States. In the paper, he stressed that the length of the UK coastline is uncertain and depends on the scale used in the survey (Skorek, 2018; Wu, 2018). The smaller the scale used to measure the length of coastline, the longer the corresponding length of coastline. Therefore, the traditional length scale is not a good measure of coastline, and a new parameter or measure must be found to better characterize the coastline. In the in-depth study of this problem, he brought out the concept of fractal. How long is the British coastline? It is considered to be the first epoch-making paper on fractal (Chen et al., 2016).
The term "Fractal" was first proposed by Mandelbrot. Its original meaning is "irregular, fractional and fragmented" object. At present, fractal is a frontier topic in nonlinear science. In different literatures, fractal is given different names, such as "Hausdorff measure set", "irregular set" and "set with fine structure", etc. Generally, fractal can be regarded as the state of large and small fragments gathering. Due to its rapid development in many sciences, fractal has become a discipline to describe the regularity of many irregular things in nature (Luo, 2016).
Based on the above background, this paper proposed the research method for heterogeneous fractal characteristics of landforms in the water of the Xisha Islandas based on multiple linear expression, and analyzes the heterogeneous fractal characteristics of landforms in the water of the Xisha Islands (Gao et al. 2017).
Materials and methods
Multiple linear regression analysis of projection pursuit based on extracting the factors of heterogeneous characteristics
The projection pursuit multiple linear regression analysis method based on the extraction of heterogeneous characteristics factors. Firstly, according to the common characteristics of landforms in the water of the Xisha Islands, this method divides the affiliation of independent variables to the heterogeneous characteristics (Lyu et al., 2018). Then, the projection pursuit technology is used to classify the independent variables into the characteristic factors. Finally, the heterogeneous characteristics factor is used as a new explanatory variable to complete the multiple linear regression analysis of landforms in the water of the Xisha Islands. The advantage of this method is to solve the multicollinearity problem in the case of small samples. At the same time, by extracting the factors of the independent variable, the independent variable screening is more consistent with the research significance of the problem itself, so as to distinguish the traditional independent variable screening method (Liang & Pei, 2016).
The affiliation division of independent characteristics
When modeling specific problems with multiple regression analysis, it often involves dozens or hundreds of independent variables because of the complexity and specialization of the problems. Traditional methods, such as correlation coefficient analysis and stepwise regression, can achieve a good fitting effect if they are only selected from the perspective of data analysis, but the regression model is often lacking persuasion and research significance. Aiming at the multi-level phenomenon that landforms in the water of the Xisha Islands show common characteristics when there are too many independent variables or they belong to a specific subject according to their professional meanings, the independent variables of landforms in the water of the Xisha Islands are classified as the heterogeneous characteristics factors according to their affiliation, which are the new explanatory variables of multiple regression analysis (Schuur et al., 2016).
Projection pursuit
The main feature of projection pursuit is to solve the problem of "dimension disaster" caused by the sparse high-dimensional spatial data of landforms in the water of the Xisha Islands. By projecting the high-dimensional data of landforms in the water of the Xisha Islands into the 1-3-dimensional subspace, it can maximize (or minimize) the projection index and find the projection that can reflect the original high-dimensional data structure or characteristics, so that the projection data is analyzed later (Abuzied & Alrefaee, 2017). The steps of dimensionality reduction for the high-dimensional data of landforms in the water of the Xisha Islands are as follows.
Step1: Data normalization of landforms in the water of the Xisha Islands.
Because there are variables in the water of the Xisha Islands sample data, there are great differences in the dimensions and orders of magnitude between variables, which makes the variable indicators lack consistency measurement. In order to facilitate the analysis, and ensure the equivalence and order of landforms in the water of the Xisha Islands data, it is necessary to normalize the original data of landforms in the water of the Xisha Islands. The normalization method of landforms in the water of the Xisha Islands is as follows.
As larger as better, the indicators are:
As smaller as better, the indicators are:
Where, x max( j) and x min( j) are maximum and minimum value of the jth indicator, x(i, j) is a sequence of normalized values of index eigenvalue.
Step 2: Construct projection index function Q (α).
PP method is to aggregate the P dimensional data { x ( i, j ); j =1,2,...p} of landforms in the water of the Xisha Islands into { α (1), α (2), α (3),..., α (p)} as the one-dimensional projection value z ( i ) of the projection direction:
Step 3: Construct projection index function.
In order to find the heterogeneity characteristics of landforms in the water of the Xisha Islands data in the high-dimensional data of landforms in the water of the Xisha Islands, when synthesizing the projection index values, it is required that the distribution characteristics of the projection values should meet the requirements that the local projection points should be as dense as possible, and it is better to agglomerate into several point clusters, while the whole projection point clusters should be as scattered as possible (Wilson et al., 2016). Therefore, the projection index function can be expressed as:
Where, S z is the standard deviation of the projection value; D z is the local density of the projection value z(i).
Where, E(z) is the average value of the sequence z(i); R is the window radius of the local density, which can be taken as 0.1; r (i, j) = |zi - zj|u () represents a unit order function, if R - r (i, j)≥0 its value is 1, then if R - r(i,j)<0 its value is 0.
Step 4: Optimize the projection index function to determine the best projection direction. Different projection directions reflect different characteristic structures of landforms in the water of the Xisha Islands. The best projection direction is to reflect the projection directions of some kind of characteristic structures of landforms in the water of the Xisha Islands as much as possible. Therefore, the best projection direction can be estimated by solving the problem of maximizing projection index function, namely:
This is a complex nonlinear optimization problem with optimization variables {α(j);j=1,2,...,p}, which is difficult to deal with by traditional optimization methods. Therefore, in this paper, real coded accelerated genetic algorithm (RAGA) is applied to solve the problem of high-dimensional global optimization of its heterogeneous characteristics of landforms in the water of the Xisha Islands.
RAGA
The standard genetic algorithm (SGA) code is binary, its process is tedious, and the accuracy is limited by the length of string, the calculation is large, and premature convergence may occur, which is not convenient to develop genetic operators for special problems. To solve this problem, this paper uses an improved accelerated genetic algorithm based on real coding, which greatly enhances the optimization performance of the algorithm (Oto-Peralias, 2018). The steps of RAGA modeling are as follows:
(1) The following linear transformation is used to optimize the real number coding of landforms variables in the water of the Xisha Islands:
Where f is the objective function of the optimized landforms in the water of the Xisha Islands, P is the number of optimized variables. Equation (9) corresponds the j-th variables to be optimized x (j) AAA and a (j) of the landforms in the water of the Xisha Islandson on the initial variable interval [0, 1] to the real number y (j) on the interval [0, 1]. y (j) is the genetic gene of RAGA.
(2) The initialization of the parents of landforms in the water of the Xisha Islands. Suppose that the size of the parents of landforms in the water of the Xisha Islands is n, then n groups uniform random number on the interval of [0,1] are generated. There are P in each group, namely {u(i,j);j = 1,2,...,P;i = 1,2,...,n}.The individual y(i,j) is substituted into equation (9) to obtain the optimal variable value x( i, j), and then the corresponding objective function value f (i) is obtained through equation (7). According to the order from small to large, {f (i);i = 1,2,...,n} corresponding individuals y(j, i) are sorted. The smaller the objective function value of landforms in the water of the Xisha Islands is, the stronger the individual's adaptability is. After sorting, the first individual is the excellent individual, so that it can enter the next generation directly.
(3) The fitness evaluation of the parents in the water of the Xisha Islands. The evaluation function based on the order is to distribute the regeneration according to the order of chromosome, which is not suitable for the actual target value. Set parameter α Є (0,1), and the evaluation function based on order is:
Here i = 1 means the chromosome is the best, i = N means the worst.
(4) The first generation y (j, i) of landforms in the water of the Xisha Islands was generated by selection. The selection process is based on rotating the wheel n times. Each rotation selects a chromosome for the new population. The selection process is described as follows:
The cumulative probability q.(i = 1,2,...,N) of each chromosome y( j, i ) is:
A random number r is generated from the interval [0,q i ]; If q i-1 < r ≤ q i y ( j, i), the i-th chromosome is selected. Repeat step 2 and step 3 for N times, so that the N duplicated chromosomes can be obtained and a new generation of individuals can be formed.
(5) The parent population of landforms in the water of the Xisha Islands was hybridized. To determine the parent of the crossover operation, repeat the following procedure from i = 1 to i = N: The random number r is generated from [0,1], if r < pc , then y ( j, i) is a parent. y'1( j, i) andy' 2 ( j, i) were used to represent the selected parents, and they were randomly matched. When the parents are odd, one chromosome can be removed or another chromosome can be selected to ensure pairing (Ginde et al., 2016). The arithmetic crossover method is adopted, i.e. firstly, a random number C is generated from (0, 1), then the crossover operation is carried out between y\(j, i) and y' 2 (j, i) according to the following form, and two offspring X and y are generated:
The second generation {y 2 (j, i);j=1,2,...,p; i=1,2,...,n} of landforms in the water of the Xisha Islands was obtained by the above hybridization.
(6) Mutation operation. The process of parent selection of mutation is similar to that of cross operation. From i = 1 to N, the following process is repeated:
The random number r is generated from the interval [1, 0]. If r<p m is selected, then chromosome y( j, i) is selected as the variant parent, and y( j, i) is selected as the variant parent to correspond to each selected parent, which is represented by y' 3 (j, i). Perform the variation as follows. In Rn, the direction of variation d was selected randomly, then y'(j, i) + Md. A new generation {yjd, i);j=1,2,...,p; i=1,2,...,n} of landforms in the water of the Xisha Islands was obtained by mutation.
(7) Evolutionary iteration. From the previous step, we will get 3N progeny individuals, and sort them according to their fitness function from large to small. The first (n-k) progeny is selected as the parent population of the new landforms in the water of the Xisha Islands. The algorithm turns to step (3) to carry out the next round of evolution, reevaluation, selection, hybridization and mutation. This is repeated until the objective function value of the optimal individual y n ( j, i) is less than a certain set value or the algorithm reaches a predetermined number of times of acceleration, and the algorithm ends. At this time, the optimal individual in the current population is regarded as the optimization result of RAGA, which are the heterogeneous characteristics of landforms in the water of the Xisha Islands (Zhuo, 2016).
Research method for fractal characteristics of Landforms Based on projection covering method
Basic principle of projection covering method
The projection covering method is to directly quantify the heterogeneous characteristics of landforms in the water of the Xisha islands by establishing the corresponding relationship between the surface of the water of the Xisha islands and the projection surface, projecting the landforms surface onto the two-dimensional plane. In this method, the complex landforms in the water of the Xisha Islands are projected vertically onto the projection plane of square grid with side length r (Juban et al., 2016). Let a, b, c and d be the four corners of any square grid on the projection plane of the water of the Xisha Islands, corresponding to the points on the surface at different heights. If the surface area enclosed by these four points is approximately obtained, the total area of the whole surface of landforms in the water of the Xisha Islands is approximately:
Where S(r) is the total surface area corresponding to the square grid side length r of the projections of the lands in the water of the Xisha Islands; S k (r) is the surface area corresponding to the k-th square grid in the projection surface of the Xisha Islands; N(r) is the total number of meshes projected from the ground surface of the water of the Xisha islands to the projection surface of the square grid; r is the mesh side length of the projection face of the square mesh; k is the mesh sequence number of the projection face.
When the side length r of the square grid of the planes in the water of the Xisha Islands is changed, the total surface area S(r) is also changed. Especially when r - 0, the real surface area of landforms in the water of the Xisha Islands is close to S(r). If the surface has fractal characteristics, according to the fractal theory, the relationship between S(r) and R is:
Where D s is the fractal dimension of the landforms surfaces in the water of the Xisha Islands.
Irregularity analysis of heterogeneous body of landforms in the water of the Xisha islands based on the landforms fractal dimension
The basic principle of the fractal dimension of lands surface in the water of the Xisha Islands is based on the projection covering method. Using the spatial analysis function provided by ArcGIS software, the DEM (tin format) of in the water of the Xisha Islands obtained from each measurement is transformed into grid data format (Grid format) of different sizes. Grid sizes are 3, 6, 9, 12, 15, 93, 96, 99mm, 33 species in total (Iacobucci et al., 2016). Then the surface area S(r) of the different grid sizes corresponding to the landforms in the water of the Xisha Islands is calculated. Finally, take In(r) as the abscissa and In[S(r)] as the ordinate, and collect the calculation results of different scales r into the double logarithmic coordinate system. The least square method is used to fit the straight line and determine the scale-free interval. The fractal dimension of the surface of landforms in the water of the Xisha Islands can be determined by the slope f of the fitting straight line, namely Ds = 2-β. Based on this, we can measure the irregularity of landforms in the water of the Xisha Islands. The implementation process is shown in Figure 1:
Results
Shidao is a Rock Island in the Xisha Islands, located in the northeast of Yongxing Island, the largest island in the Xisha Islands, with a distance of more than 1 km. With an altitude of 15 meters, it is the top of Xisha Islands. The rock on the island stands up, and the features of landforms on the eroded coast are very obvious, which is in sharp contrast with other grey sand islands surrounded by the crystal white jade belt beach. The geologists who set foot in Xisha have paid attention to its own unique characteristics, all of which focus on the geological history of the Xisha Islands since the late period. In this paper, the objective of the study is to study the terrestrial characteristics of landforms in the Shidao waters of the Xisha Islands.
Dynamic change of fractal dimension of landforms
Table 1 shows the surface fractal dimensions of the landforms in the Shidao waters of the Xisha Islands at different development stages calculated by this method:
Table 1 Fractal dimension calculation results of Xisha Islands water area based on projection coverage method
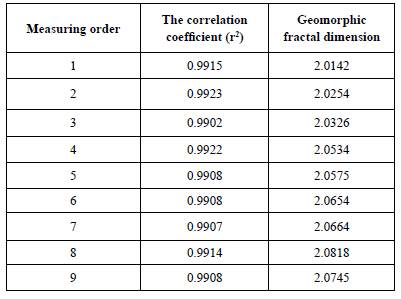
Note: 9 significance tests were all extremely significant.
It can be seen from the table that in the scale-free range, there is a good linear relationship between In[S(r)] and In(r), the correlation index r 2 is above 0.99, and the significance test reaches the extremely significant level (α=0.01). This shows that the landforms of the shisha Islands waters have obvious fractal characteristics in this region. With the change of measurement sequence, the surface fractal dimension of the landforms in the Shidao water area of the Xisha Islands increased from 2.0142 in the first measurement to 2.0818 in the eighth measurement, and the surface fractal dimension increased greatly from the first measurement to the fourth measurement, and then decreased. At the 9th measurement, the fractal dimension of the landforms surface in the Shidao waters of the Xisha Islands decreased to 2.0745, which was lower than that of the 8th measurement. This reflects that the dynamic evolution process of the simulation is an accurate expression of the dynamic changes of the overall characteristics of the landforms in the Shidao waters of the Xisha Islands.
Comparisons of fractal dimensions of landforms in the Shidao water area of the Xisha Islands by different methods
In order to further analyze the advantages of this method for the Shidao water area of the Xisha Islands, the fractal dimension of the landforms surface in the Shidao water area of the Xisha Islands calculated by the method in this paper is compared with the results obtained by the more commonly used calculation methods (Table 2). Among them, take the increment of landforms fractal dimension as the comparison object. That is to say, the fractal dimensions of the landforms in the Shidao water area of the Xisha Islands calculated by the above two methods are obtained by subtracting the corresponding geometric topological dimensions of the fractal objects. The corresponding fractal objects of this method and box counting dimension method are landforms surface and terrain contour, and the corresponding values of geometric topological dimension are 2 and 1.
Table 2 Fractal dimension increment comparison of the landforms in shidao waters of Xisha islands by different calculation methods
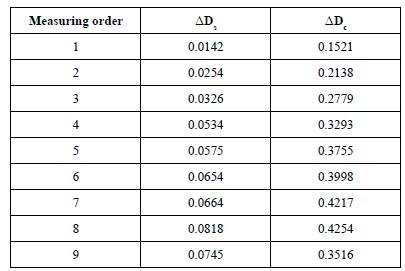
It can be seen from Table 2 that the maximum value of the fractal dimension increment of landforms calculated by the method in the Shidao water area of the Xisha Islands is 0.0818 and the minimum value is 0.0142. During the first to the eighth measurements, the average increment of fractal dimension of landforms in the Shidao water area of the Xisha Islands increased significantly. The maximum increment of fractal dimension of small watershed landforms calculated by box counting dimension method is 0.4254 and the minimum is 0.1521. It can be seen that the fractal feature quantification results of landforms in the Shidao water area of the Xisha Islands are more conducive to reveal the heterogeneity of the overall characteristics of landforms in different development stages.
Fractal dimension values of different regions
For a large number of statistical fractals existing in nature, they are not infinite generation like strict mathematical fractals, but can be established in a certain scale range. A certain form is closely related to a certain mechanism of action, and it is precisely because of the fractal characteristics of the multidimensional domain in the forms of landforms that provides an excellent opportunity for us to study the mode, intensity and scale range of the internal and external forces. Divide the Shidao water area of the Xisha Islands into six areas, and calculate the fractal dimension values of different areas by the method in this paper. See Table 3 for the calculation results of the fractal dimension values of different areas:
Table 3 Calculation results of fractal dimension values in different regions
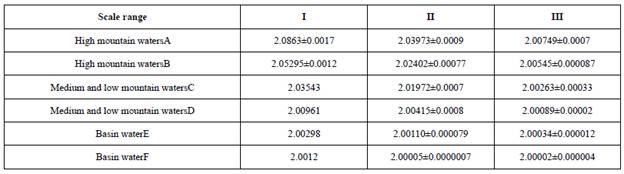
Figure 2 is the fractal structure of the multi-dimensional domain in the Shidao water area of the Xisha Islands.
From the above results, we can see that: In the Shidao water area of the Xisha Islands, each research area can be roughly divided into three obviously different linear intervals (scale from small to large is called I, II, III respectively), that is, multi-scale fractal. It shows the local self-similar or self-affine replication relationship in the Shidao water area of the Xisha Islands. With the increase of the observation scale, the fractal dimension value of each interval decreases in turn. This shows that with the increase of the observation scale, the roughness of the shape in the Shidao water area of the Xisha Islands is decreasing, and the fluctuation of the smaller scale is ignored. The process of controlling the action of landforms is from the dominant role of external forces, the struggle between internal and external forces, to the mechanism that is basically replaced by internal forces.
In the Shidao water area of the Xisha Islands, the fractal dimension values of different types of landforms in the corresponding interval are as follows: water area in high mountain area > water area in middle and low mountain area > water area in basin area, which reflects the difference of surface roughness or complexity of different types of landforms. Therefore, the fractal dimension value calculated by this method can be used as an important index of the erosion intensity of the surface external forces in the Shidao water area of the Xisha Islands.
In the Shidao water area of the Xisha Islands, the distribution of multifractal spectral values of different landforms is shown in Figure 3.
It can be seen from Figure 3 that in the Shidao water area of the Xisha Islands, the overall characteristics of the water area in the high mountain area > the water area in the middle and low mountain area > the water area in the basin area, thus reflecting the heterogeneity degree of the probability distribution of the surface area of different types of landforms in the Shidao water area of the Xisha Islands. In the Shidao water area of the Xisha Islands, different study areas of the same landforms also show different degree of uneven distribution of undulating surface area. Among them, the distribution of heterogeneity is significant in the C study area and the f study area of basin area in the middle and low mountain area, on the contrary, their corresponding D and e areas tend to be uniform and single fractal characteristics.
Discussion
In the Shidao water area of the Xisha Islands, the study of landforms can be said to be a new research field. We believe that the problems that need further improvement and in-depth study at present are mainly from the following aspects:
(1) The internal mechanism of the characteristics of the fractal segments of landforms
Many initial researches only focus on the discovery and description of the fractal phenomena in the landforms phenomenon. However, the internal formation mechanism or external dynamic conditions of the fractal properties of these landforms, as well as whether the fractal characteristics can reflect the nature of a particular landforms process, and how the regional characteristics of the fractal characteristics of landforms are, remain to be further explored. At the same time, the existing research shows that many dynamic factors of landforms, such as geography, geology, geophysical field (gravity field, etc.) and even geological structure (fault), can have fractal structure. On this basis, the landforms can also be fractal, so what is the mathematical relationship between these two kinds of fractal?
(2) The relationship between fractal dimension of landforms and landforms process
Many researches on the fractal characteristics of landforms reveal that the fractal law is only a static characteristic (i.e. the "instantaneous" state of landforms nowadays), while others try to associate the fractal characteristics with the development of landforms, but it is still difficult to explain the relationship between the fractal characteristics and the landforms process. Therefore, the following problems need to be studied:
1) Is the fractal dimension of landforms constant or changing?
2) How long can the fractal dimension of modern landforms represent the geo history stage?
3) The study shows that the climate and lithology conditions are basically the same, and the development of landforms reaches the basin in the middle age or old age (the area is large enough), and the fractal dimension value of the river is close to 0. Does this mean that a stable (balanced) landforms type fractal value tends to a definite value (constant)?
4) If the fractal dimension of landforms in a certain stage is a constant, how long does it take from the beginning of its development to reaching this constant?
5) Can fractal dimension reflect the stages of the development of landforms? That is, do different landforms have their own typical fractal dimension? What are the reasons?
6) Although fractal dimension can be used as the index of watershed erosion period division, what is the relationship between the division of water system development stage marked by the formation of fractal water network and the traditional landforms stage division based on landforms profile and other morphological factors?
7) Does the geological structure, lithology, climate and vegetation affect the fractal dimension of this stage?
(3) The relationship between the gradient free interval and the scale range of the main landforms processes
The fractal features of landforms are usually only statistical features in a certain scale range, and are this scale range related to the scale range of the main landforms processes? At the same time, the same landforms process or action is different on different scales, and the fractal feature is a kind of cross-scale geometric feature in a certain scale range (i.e. scale-free range). What does this fractal feature mean? What's more, does a certain kind of landforms follow the statistical fractal feature, which means that the mechanism of its leading landforms forces is scale-free or cross scale? The answer to this question is very important in the reliability judgment of the results of the indoor micro landforms experimental model and the determination of the extension or representative range (including common hydraulic engineering landforms simulation such as Gezhouba project, etc.).
(4) Deepening the research of landforms mode
There are many successful mathematical rules to simulate the generation of fractal terrain, but mathematicians also admit that this terrain does not reflect any information of the formation process of landforms. Other studies have found that the natural landforms landscape can be simulated by using a simple near linear rule. But what is the relationship between this simple rule and the multifactor complex process in the development of natural landforms? Does it reflect some characteristics of the complex landforms process, or just conform to some rules of people's visual judgment? How can we effectively use this mathematical rule to establish the landforms development and evolution model with the participation of internal and external dynamics? What is the difference between simulated terrain and natural terrain? Obviously, the interpretation of fractal landforms model is also an important aspect.
(5) The feasibility study on the application of time and space fractal law in the prediction of landforms process
Landforms events, such as earthquakes, floods, mudslides, etc., can be time fractal, which means that they have certain predictive significance. For example, if the distribution of flood water is time fractal, it means that the ratio of the number of floods with 100 year return period to the number of floods with 10 year return period can represent the ratio of the flood with 1000 year return period to the flood with 100 year return period.
Conclusions
Gouide, a famous landforms scientist, pointed out in his book the technology of landforms research that once a new technology can be used, the whole scientific process can develop around it and enter a higher level of research and interpretation. As a new technology, fractal theory is also applied to landforms. The application of fractal theory in the study of landforms can be very extensive, including the study of landforms of various landforms or watershed as a whole and the study of landforms process. Fractal theory provides a new research method for landformers, which enables them to discover the new cross-scale quantitative characteristics of the traditional complex and disordered landforms phenomenon. At the same time, fractal dimension has become a new parameter in landforms. The application of fractal theory also makes it possible for the research of landforms to connect the simple formula obtained from field observation with the complex landforms and establish the model. Therefore, fractal theory provides a possible way for landforms scholars to explain complex terrain.
Therefore, this paper puts forward the research method for heterogeneous fractal characteristics of Xisha Islands water lands based on multiple linear expression, and studies the forms of heterogeneous characteristics in the Shidao water area of the Xisha Islands. There is a good linear relationship between In[S(r)] and In(r), the correlation index r2 is above 0.99, and the significance test reaches the extremely significant level (α=0.01). This shows that the landforms of the shisha Islands waters have obvious fractal characteristics in this region. The Shidao water area of the Xisha Islands is divided into six regions, and the fractal dimension values of different regions are calculated by the method in this paper. The overall characteristics are as follows: mountain water area > middle and low mountain water area > basin water area. This shows the degree of heterogeneity of the probability distribution of rough surface area in different types of landforms.