1. Introduction
Recent research has suggested that the human brain is a type of complex system in which individual regions constantly interact rather than function independently. This observation has led to attempts to understand the brain from a network perspective (Bullmore & Sporns, 2009). Discussions about brain connectivity also drew attention to the resting-state network, which is active even in the resting state, and led to the development of brain network analysis, a recent integrative perspective. This analysis is based on graph theory, and the brain network refers to the integrative network that continuously processes and transmits information between structurally and functionally connected brain regions (Van Den Heuvel & Pol, 2010).
Watts and Strogatz (1998) explored the characteristics of networks by classifying them as random, lattice, or small-world networks. In a random network, the node links are randomly determined, and while information transmission is extremely fast, the separation of information is low due to the low level of node clustering. In contrast, a lattice network is characterized by a regular pattern of links, and while it has a high level of node clustering, it takes a long time to transmit information to distant nodes. The human brain has the characteristics of a small-world network, which features somewhere between random and lattice networks. This means it is possible to minimize the connection cost and to process information efficiently as it maintains an optimal state between functional integration and separation (Kim & Choi, 2016). In network analysis, the small-world network can be defined as having both high global efficiency (GE) and local efficiency (LE), both which support parallel information processing, meaning that processing of multiple features occurs simultaneously (Latora & Marchiori, 2001, 2003). In other words, the human brain is likely to maintain high levels of both types of efficiency.
Autism spectrum disorders and schizophrenia are the disorders most typically associated with impairments in social interaction and social cognition (Green & Horan, 2010; Pinkham et al., 2008). The difficulties experienced by individuals with these mental illnesses seem to be associated with impaired neural networks in the brain regions usually associated with social cognition, such the amygdala and orbitofrontal cortex (OFC) (Pinkham et al., 2008; Sabbagh, 2004; Welchew & et al., 2005). Such problems in brain functioning have also been identified in the studies that employed integrative indicators of brain network activity and schizophrenia group clearly showed lower GE and LE (Kennedy et al., 2006; Liu & et al., 2008). Both indicators were found to have significantly decreased in the brain network of infants with high risk of autism (Lewis et al., 2014). In the study by Rudie and et al. (2013), individuals with ASD groups showed lower levels of separation than controls in OFC, medial prefrontal cortex, fusiform gyrus, and secondary visual area, resulting in lower local efficiency. However, these studies were mostly conducted with patients who were suspected of having sustained brain damage, and research on the relationship between brain network activity and social functioning is particularly lacking. Therefore, an investigation of whether specific network characteristics do in fact reflect individuals social competence in the community requires investigation in a normal population.
Most people live in the context of social relationships, which change according to factors such as age, current roles, and the environment (Van Tilburg, 1998). Late adulthood is a period associated with less social support and relationship due to a variety of factors, such as the loss of a spouse, retirement, and disease (B. Cornwell et al., 2008; Dugan & Kivett, 1994; Prince et al., 1997; Van Tilburg, 1998). This characteristic of late adulthood may become more pronounced due to rigidity in the elderly as demonstrated through discomfort with new things, which often leads to social isolation (Cornwell & Waite, 2009). Therefore, late adulthood is characterized to lack of resources for sustaining and developing social relationships. Consequently, maintaining and developing relationships requires proactive behaviors as well as development of roles (Van Tilburg, 1998). Unfortunately, the typical aging process is accompanied by natural cognitive decline. Considering that effective and adaptive interactions with others requires complex brain functioning, such as cognitive calculation and inferences (Winningham & Pike, 2007; Ybarra et al., 2008), developing ties with others is likely to be the result of utilizing individual (cognitive) resources or abilities, rather than the result of environment. In summary, late adulthood is suspected to be a crucial period in which proactive efforts requiring integrate brain function are needed. The elderly population with varying degrees of cognitive decline due to aging is well suited to the study the relationship between brain ability and human relationships.
Researchers have commonly used the concept of social networks to understand the social relationships of individuals, referring to social relationships as where individuals continuously interact (Dunkle et al., 2001). The most basic unit of analysis in research on group phenomena and ties with others in social networks can be specified as a triad-relationship (Figure 1), which is divided into a “closed triad,” where all three-A, B, and C-are linked, and an “open triad,” where there are links between A and B and A and C, but no link between B and C (Huang et al., 2014, April). The two concepts stem from the social phenomena referred to as “closure” and “hole”, and explain the respective social roles and patterns.
A social relationship with a structural hole can be considered economical in that individual serves as a bridge in the relationship in terms of information transmission and control (Burt, 1992). However, as the bridging individual acquires the power to control and regulate information from a more advantageous position, mutual trust with other members may have limitations (Bae & Kim, 2006). In contrast, in a closed-type relationship, a horizontal relationship is assumed, the social bond is emphasized, and mutual transactions and trust may increase (Gargiulo & Benassi, 2000). It is assumed that the formation of a closed triad, a type of closed relationship, requires individual to interact with a sense of intimacy, and it is predicted that the sense of trust increases in the relationship accordingly.
Recent studies have employed the size of social network (i.e., the total number of members in a network) or the outdegree centrality when trying to determine the social relationships individuals (Barrera et al., 1981; Costenbaderm & Valente, 2003). However, quantity of social relationship is insufficient to represent ones interaction and actual attitudes. Qualitative aspect, such as intimacy and social support, should be considered. Therefore, social network analysis requires the use of variables that represent both quantitative and qualitative aspects (Fiori et al., 2006). The social network measure in the present study incorporated both qualitative and quantitative aspects of interpersonal relationships by setting a criterion for the level of conversation when considering whether a tie exists, while counting the number of closed triads.
As discussed earlier, the human brain is thought to be closely involved in social functioning. The findings of recent studies suggest that the problems in brain network activity are related to deficits in social functioning and abilities. In many cases, old adult tends to have fewer interaction and be more likely to experience social isolation. This study hypothesizes that interpersonal relationship in late adulthood is influenced by brain network. We assumed that an increase and growth in interpersonal relationships in late adulthood would be manifested as a higher score on the closed triad index. The change in the closed triad index was measured by counting the number of closed triads at two time points separated by an interval. Study participants were classified into two groupsone group where the number of relationships increased and another group where the number of relationships was maintained or decreased between the first and second time point. The comparison of the brain network activity of the two groups was conducted by performing an independent sample t-test on resting-state fMRI (rs-fMRI) data.
2. Materials and methods
2.1 Participants
This study was a subsample from the Korean Social Life, Health and Aging Project (KSHAP). The KSHAP is a community-based cohort study that collected information from an entire population of older adults in Township K (Youm et al., 2014). In the first wave investigation we identified people who were 60 years old or older and their spouses among the 1864 residents of Township K (Kwak et al., 2018). In the wave 1 (2011) and wave 2 (2012) of the study, participants completed a social network survey. This studys aim to compare with brain network and social network, so we adapted following exclusion criteria: under 65years old, having a history of losing consciousness due to head trauma, infarction or stroke, lefthanded, ambidexter and K-CDR score 0.5 to 3.0. Therefore, we needed to apply neuropsy chological tests for exclusion criteria. Only 194 were administered neuropsychological tests in wave 3 (2015). Consequently, we used only 194 participants datasets. Also, we excluded participants with any history of neuropsychiatric or neurocognitive disorders. The remaining 52 healthy participants (31 females) were included in the final dataset. The study was approved by the Institutional Review Board of Yonsei University, and all participants provided written informed consent for the research procedures.
2.2 Questionnaires
2.2.1 Closed triad index
The KSHAP respondents were also asked how often they talk to each network member (include their spouse). It has eight-point scale, ranging from “every day” to “less than once per year”. With this data we judge connection only if their score was four or more (up to two weeks a day). Based on these data, we calculated the number of closed triads.
2.2.2 Korean-Clinical Dementia Rating Scale; K-CDR
The CDR assesses three domains of cognition (memory, orientation, and judgment/problem solving) and three domains of function (community affairs, home/hobbies, and personal care). The six CDR domains were rated on a 5-point scale (0=no impairment; 0.5=questionable impairment; 1=mild impairment; 2=moderate impairment; 3=severe impairment; 4=profound impairment; 5= terminal impairment).
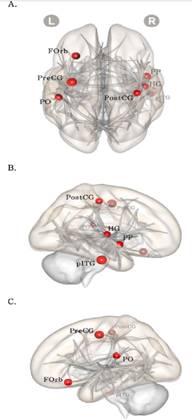
Note. The red color node means values of increase group is larger than values of decreased/sustained group in the closed triad relationship. The nodes size is proportional to the difference between groups; A= superior view, B= right view, C= left view; FOrb= frontal orbital cortex, PO= parietal operculum cortex, pITG= posterior inferior temporal gyrus.
Figure 2 The result of local efficiency t-test with Increased group and Decreased/sustained group (cost 0.16)
2.3 Image acquisition
MRI images were acquired using a 3-Telsa MAGNE-TOM Trio 32 channel coil. Whole-brain T1-weighted images were reconstructed from 224 sagittal slices of 1mm thickness using an MPRAGE sequence with the following parameters: TR=2.3 s, TE=2.36 ms, FOV=256×256 mm2 and FA=9 . To estimate change of signal we get EPI protocol; TR=2.0 s, TE=30 ms, FOV=240×240 mm2, FA=7. Voxel size 3×3×3 mm, gap = 1 mm
2.4 Data analysis
2.4.1 Image data
Image preprocessing was carried out using tools implemented in Statistical Parametric Mapping software (SP-M12; Wellcome Department of Imaging Neuroscience, London, UK) and analyzed with CONN 17.c Toolbox (http://www.nitrc.org/projects/conn). All images were smoothed using an 8-mm full-width at half-maximum Gaussian kernel. The images were analyzed using SPM 12. The functional images were motion corrected, spatially normalized in the Montreal Neurological Institute (MNI) space, resampled to 3.0mm x 3.0mm x 3.0mm voxel size, and then spatially smoothed using a 6mm full-width half-maximum Gaussian kernel.
Data were analyzed using a seed-driven approach with the Conn-fMRI functional connectivity toolbox that was designed to work with both resting state scans and block designs. After images were preprocessed using SPM12, temporal connectivity correlations between the BOLD signal from the seed regions and that at every other brain voxel during the entire acquisition period provided seed-to-voxel connectivity estimations. Before averaging of individual voxel data, the waveform of each brain voxel was filtered using a bandpass filter (.008Hz < f < .09Hz) to reduce the effect of low-frequency drift and high-frequency noise. Realignment parameters and main session effects were defined as the first level covariates.
2.4.2 The Default Mode Network analysis
The choice of the threshold may have a strong effect on the network parameters, so analysis using a variety range of thresholds is performed (Sala-Llonch & et al., 2014). This study used threshold for the correlations to keep the 16% strongest connections of all the possible connections of edges. We analyzed data supposing all participants have the same number of edges.
2.5 Network definition
We used 105’s region of interest (ROI) in cerebral defined by Harvard-Oxford Atlas (HOA). Using participants ROI, we constitute 105×105 adjacency matrix that calculated from functional connection with mean of timeseries signal’s correlation. In this study, we just consider positive correlation coefficient, which is normally used in network analysis. We used binary network: edge is coded 1 if it has a value above the threshold; edge is coded 0 if it has a value below the threshold. Also, we used global efficiency (GE) and local efficiency (LE) for network measurements, which is generally used for small-world network (Rubinov & Sporns, 2010).
2.5.1 Characteristic path length
Characteristic path length is the average of the shortest path lengths of all pairs of nodes in brain. L is characteristic path length when d ij is the shortest path length between node i and j. Shorter path length between each node means better efficiency.
3. Results
52 elderly residents of a rural village in South Korea completed a survey, neuropsychological assessments and MRI scans. We divided closed triad into an increased group (male; 7, female; 16) and a decreased or sustained group (male; 12, female; 15). In Table 1, both groups’ age and education were not significantly different.
The global efficiency of the Increased group and Decreased/Sustained group were not significantly different in .05∼.20 network threshold. The result was showed as supplemental material.
The local efficiency of the Increased group and Decreased or sustained group was significantly different in 16% network threshold (p<.05). Increased group showed higher local efficiency than other group, especially in right frontal orbital cortex, left inferior temporal gyrus, and left parietal operculum cortex (Table 2, Figure 2).
4. Discussion
This study using resting-state brain network to determine that relationship building pattern in late adulthood was influenced by brain network integration. Although previous studies have demonstrated an association between brain network activity and social functioning, few of these findings can be generalized to the normal population because they were conducted with patients with extreme impairments to social functioning. In the present study, 105 brain regions were designated to represent the whole brain and the brain network index was estimated based on GE and LE as the indicator of the small-world network to determine the brain network efficiency of participants. The indicator of interpersonal relationship building in late adulthood was the closed triad, a social network variable used in sociology. This indicator was measured interval between two time points to properly represent the change in closed triad in late adulthood. The results of the analyses are described below.
First, the results of the two-group comparison in the change in the number of closed triads showed no group difference for GE, but a significant difference for LE. The group with an increase in the number of closed triads showed higher LE in the entire brain network. More specifically, this group showed higher LE in the right OFC, left parietal operculum, and left inferior temporal gyrus, compared to the group in which closed triads were maintained or decreased in number. In other words, the brain network was more locally efficient in the group that showed an increase in building interpersonal relationships than the group that showed either maintenance or a reduction in building relationships. LE differences between the two groups were observed in OFC, parietal operculum, and inferior temporal gyrus. This study suggests that brain network integration in OFC is significantly associated with intimate interpersonal relationship. Previous study demonstrated that OFC is the primary region involved in various social interaction (Brothers, 2001; Frith & Frith, 2001), is related to decision-making processes (Bechara et al., 2000), and in particular, is activated when social skills, such as empathy, are required (Hynes et al., 2006). Our study’s findings corroborate those of Rudie and et al. (2013), who found that LE was significantly lower in regions including the OFC in those with autism-spectrum disorders and who show lower levels of social functioning. The parietal operculum is classified as part of the somatosensory cortex and activated when imagining the pain, although its role needs to be clarified further (Decety & Grèzes, 2006; Ruben et al., 2001). The inferior temporal gyrus has been reported to play a major role in language proficiency (Scheff et al., 2011). Although the regions other than the OFC have rarely been reported to have a direct relationship with social functioning, parietal operculum is considered to represent socio-cognitive characteristic, such as thinking and predicting about others perspective. The inferior temporal gyrus is considered to reflect a high level of vocabulary, which would be support communication. Because of this, both areas could be needed to keeping relationship.
Second, the significant difference between the groups was found only for LE. The finding of low LE in the group with a low score for social relationships is consistent with some previous studies that have compared individuals with autistic spectrum disorders and schizophrenia with normal controls (Liu & et al., 2008; Rudie & et al., 2013). However, Kim and Choi (2016)) noted that a reduction in GE is observed in elderly people during the normal aging process while the results for LE are somewhat inconsistent. Unlike previous studies that did not take into account changes in social relations, this study has found that LE is closely related to changes in social relations. The significant increase in LE likely represents greater social functioning.
Table 1 Demographical results
Increased group (n = 25) | Decreased/or sustained group (n = 27) | t | p | |||
Mean | sd | Mean | sd | |||
Age | 73.00 | 4.87 | 74.37 | 4.80 | -1.020 | .313 |
Education | 6.00 | 2.40 | 6.70 | 5.04 | -.651 | .519 |
Sex(male/female) | 9/16 | 12/15 | ||||
Job (farmer/self- | 18/0/2/5 | 19/1/1/6 | ||||
employed/etc./none) |
Note: ***p < .001, **p < .01, *p < .05.
Table 2 The local efficiency t-test results
Region | Increased Group M(SD) | Decreased or sustained group M(SD) | beta | T | dof | p |
Whole network | .73(.02) | .71(.02) | .01 | 2.12 | 50 | .03* |
Frontal orbital cortex; Right | .75(.15) | .61(.20) | .15 | 2.93 | 46 | .00*** |
Parietal operculum cortex; Left | .79(.05) | .75(.07) | .04 | 2.47 | 50 | .01** |
Inferior temporal gyrus; posterior | .76(.15) | .59(.33) | .17 | 2.04 | 42 | .04* |
division Left |
Note: ***p < .001, **p < .01, *p < .05.
Our study’s findings highlight the importance of social relationships as a major factor in successful aging and suggest that brain network activity is potentially important for those who age successfully. In a previous study, we found results about the importance of brain function in individuals with dementia and mental illnesses. However, our results have demonstrated that a lower level of brain network functioning in normal individuals cause detrimental effect in the development of interpersonal relationships. This study showed it is possible that individuals with lower LE in their brain network activity are socially isolated or stagnant, and, therefore, proactive management of brain function likely plays a major role in maintaining health late adulthood.
We note several limitations to this study. First, it is difficult to verify any causality associated with the difference between the two groups as brain imaging and the collection of social network data did not take place at the same time. Second, demographic variables such as physical and cognitive functioning were not controlled. Nevertheless, the study offers a unique contribution to the literature as an exploratory study of the relationship between the pattern of interpersonal relationship-building and brain network activity in late adulthood. Previous studies have shown that social activities and interactions, such as establishing and maintaining relationships, have a positive effect on maintaining cognitive function in older adults (Holtzman et al., 2004; Zunzunegui et al., 2003), and those without social ties, such as the elderly living alone, are 1.5 times more higher to develop dementia after three-years follow up (Fratiglioni et al., 2000). In this study, findings of higher brain efficiency in older adults who maintain and increase social ties are important in that they can take into account various possibilities for preserving brain efficiency. Of course, preservation of brain function relies on various activities including physical and cognitive activities, rather than based on one single activity (Bherer et al., 2013; Rolland et al., 2010). The results of this study suggest that the formation and maintenance of interpersonal relationships are one of the variables that positively affects brain efficiency and aging. Further research is needed to address the limitations of this study, employing analytic techniques to determine whether a specific brain region is involved in interpersonal relationship-building, and investigating whether an intervention in interpersonal relationship-building improves the brain network index in late adulthood. Third, while the CTI used in this study meaningfully reflects group activities, it seems difficult to represent various groups because they are single variables. Accordingly, it will be necessary to use various variables that effectively reflect positive changes in human relations. Fourth, the results derived from this study are uncorrected p < .05, not performed multiple tests, so further studies will be needed for more validated results. Finally, careful interpretation will be needed as there are constant debates over the use and interpretation of graph matrices (de Vico Fallani et al., 2014; Petrella, 2011).
In conclusion, we employed a novel approach to the investigation of the variation in the pattern of interpersonal relationship-building in late adulthood by conducting a brain network analysis from the perspective of brain efficiency. This study considered qualitative aspect as well as quantitative aspect of social network. Late adulthood is commonly associated with social isolation due to retirement and disease. However, the finding of this study suggests that old adults who have high network efficiency would develop relationship. Based on this, we empirically prove that the role of brain is fundamental in interpersonal relationship in old adults.