1. Introduction
Frequently, human beings are confronted with a myriad of situations inducing fear. Fear is usually referred to as a primary emotion consisting of an aversive emotional state that allows an organism to cope with a real or potential threat (Ledoux, 2003). Environmental visual stimuli perceived as threatening tend to be processed and detected faster, enhancing physiological emotional responses in order to prompt an adaptive defense response (Rosa, 2014, 2015).
However, despite emotional activation -as seen by increased physiological reactions to threatening stimuli (e.g., increased peripheral autonomic activity such as heart rate or skin conductance)- is linked to human survival, high levels of arousal, in the absence of a “real” threat, may actually prevent subjects from adjusted behavior (Larson et al., 2006). In fact, this “so-thought” adaptive process can be maladaptive in many instances, easing the development and maintenance of excessive fear, as seen in Specific Phobias (SPs) (Rosa et al., 2011). The diagnostic features of the DSM-5 (5th ed.; American Psychiatric Association, 2013) for the SPs states that the critical feature is a deep and persistent fear caused by the presence or anticipation of an object or situation, resulting in symptoms of anxiety (American Psychiatric Association, 2013).
According to estimates, around 19.2 million adult Americans are afflicted by SPs (Kessler et al., 2005), and from those, 4.7% are affected by small Animal Phobias (APs) (Stinson et al., 2007). Identical prevalence (5%) was found among Europeans (Becker et al., 2007). Within SPs, APs, such as birds, frogs, spiders, bees are reported more frequently (Becker et al., 2007) and usually present a female/male prevalence ratio of 4:1 (Fredrikson et al., 1996). The most investigated APs are related to biologically fear-relevant stimuli, such as the snake (ophidiophobia) and the spider phobia (arachnophobia) (Kawai & He, 2016; Mayer et al., 2006).
Under an evolutionary perspective, snakes and spiders may evoke the same kind of threatening as large animals do, as they represent stimuli that threatened the survival of our ancestors, triggering physiological changes (e.g., heart rate, respiration, electrodermal activity, pupillary activity) with minimal input (Öhman, 1986; Rosa et al., 2017). However, studies regarding the fear of cockroaches (Katsaridaphobia) are scarce. According to research (Scandola et al., 2010), the fear of cockroaches it might not be related to a predator threat, but rather of harmful disease threat (Curtis & Biran, 2001). This may be a motive for not seeking treatment as these individuals usually assume that this fear is simple to overcome, capturing rarely the focus of clinical attention (Hood & Antony, 2012). In fact, between 60% and 80% of phobia sufferers do not seek treatment (Essau et al., 2000).
Different theoretical approaches can drive a wide range of treatments against Katsaridaphobia (Wolitzky-Taylor et al., 2008); however, Cognitive Behavioral Therapy (CBT) is one of the most efficient methods for treating APs. Graded Exposure (GE) is a traditional therapeutic approach to fear-related avoidance that can be conducted in vivo, imagined or through virtual reality (in vitro) (Gamito et al., 2014; Gamito et al., 2010). Despite the use of immersive Virtual Reality (VR) systems on GE, the use of head mounted displays (HMDs) or data gloves for personal viewing and interaction with a virtual environment (Sharples et al., 2008) might induce severe side-effects symptoms (e.g. cybersickness). Therefore, non-immersive VR systems, such 3D-TVs or desktops are a valuable option because they are cheaper and still effective, capable of altering all the emotional response dimensions, such as behavior, subjective experience, and physiological reactions, similarly to in vivo GE (Rosa et al., 2017).
Current research on emotion-driven VR has been focused on the assessment of the users emotional state and how it can be used as input in order to adjust the VR scenario (Moghimi et al., 2016). Facial expressions, postural actions, and psychophysiological indices have been the most used inputs for assessing users emotional states (Kim et al., 2004). However, within these, psychophysiological responses are believed to be more reliable, as they are hardly to be controlled by awareness (Li et al., 2016) Generally, a physiological-driven VR Environment is considered an adaptive VR Environment, as adjustments are made in the scenario depending on users emotional state (Anolli et al., 2010). The primary aim of a physiology-driven adaptive VR is to influence the user’s emotional state in a controlled manner by recognizing and responding to user’s emotional states while
he/she interacts with the scenario (Cosi´c et al., 2010). To this end, the physiology-driven adaptive VR environments must include a time-synchronized stimuli generation, the acquisition of the users physiological activity, the estimation of users emotional state, and an adaptive closed-loop control that leads to subsequent generation of new stimuli.
The physiology-driven adaptive VR environment strives to improve the advantages of VR environments that can be used for training and therapeutic purposes. Therefore, the application of GE through physiology-driven adaptive VR environments can be of particular importance in training and clinical contexts, where individuals present some kind of emotional regulation deficit. Given that user’s physiological activity can adjust (adapt) VR environments to his/her emotional state, new application opportunities for exposure techniques may emerge as an adaptive VR Environment can keep the user immersed in the scenario as the difficulty level adjustment is done using his/her psychophysiological measurements.
Several autonomic indices have been used for physio-logical input, such Heart Rate (HR), Electroencephalography (EEG) or Electrodermal Activity (e.g., Dekker & Champion, 2007; Sakurazawa et al., 2004; Wang et al., 2010). Nevertheless, HR is the most used because it minimizes the delay between physiological changes and the visual feedback information. A physiology-driven adaptive VR environment can provide the researcher with more complete information about the users emotional reactions during the VR exposure, allowing to reach better results than those obtained with simple VR exposure, yet, little research has been reported so far for adaptive non-immersive VR environments. The present study might therefore complement these earlier studies by using 3D-TV exposure combined with physiology to lead to a deeply personal experience by linking the VR environment to the users physiological state. The aim of this study was to develop and refine a physiology-driven application embedded in a non-immersive VR environment by assessing its ability to induce fear of cockroaches in individuals with different fear levels. It was hypothesized that our VR environment is valid for eliciting fear of cockroaches, as individuals with high fear of cockroaches will experience more fear during the scenario in comparison to individuals with low fear of cockroaches. More-over, we also hypothesized that our VR environment is sensitive to cardiac changes related to fear of cock-roaches, so a larger number of cockroaches will be presented in the VR scenario in individuals with high fear of cockroaches due to a pronounced cardiac acceleration.
2. Method
2.1 Sample
This study comprises a convenience of Portuguese university students (N=39) and the inclusion criteria were the following: 1) Age ≥ 18 years of age; 2) Normal and correct vision. The exclusion criteria included preexisting substance abuse, neuro/psychological disorders, at time psychotherapeutic process, psychopharmacological treatment or extreme fear of cockroaches. The extreme fear of cockroaches was assessed with the question “how would rate your fear of cockroaches?” (1- no fear at all; 9- Extreme fear). Preliminary analysis related to the exclusion criteria led to three participants being removed from the final analysis. Therefore, both results and sample characterization were performed based on a sample of 36 participants. The sample is predominantly composed by female participants (n = 25; 69.4%), ranging from 18 to 51 years (M=22.75, SD=7.75). Considering experience with Virtual Reality, most of the sample has little (n = 7; 20%) to none (n = 15; 42.9%) experience.
2.2 Instruments
For the purpose of this study, the following self-report measures included: (1) a socio-demographic questionnaire; (2) the Fear of Cockroaches Questionnaire (FCQ); (3) the Differential Emotions Scale (Izard, 1977); and (4) the Positive and Negative Affect Schedule (Watson et al., 1988).
Socio-demographic questionnaire. Participants answered a brief socio-demographic form to collect general information, such as gender, academic qualifications, and experience with VR, computers, and videogames.
The Fear of Cockroaches Questionnaire (FCQ), based on an 18-item measure that assesses participants avoidance of and fear of harm from cockroaches, an adaptation of the Fear of Spiders Questionnaire (FSQ) (Szymanski & O’Donohue, 1995). The internal consistency of this scale was high (Cronbachs alpha = .89) in our sample. Along with this scale, the level of fear and disgust of cockroaches were assessed via single item scale [Likert rating scale ranging from 1 (not at all) to 10 (extreme)] in two different questions specifically designed for the purpose of this study.
The Differential Emotions Scale (DES; Izard, 1977), based on the original 10 basic emotions, joy, surprise, anger, disgust, contempt, shame, guilt, fear, interest, and sadness, was translated to European Portuguese. The DES is a standardized instrument that reliably divides the individual’s description of emotion experience into validated, discrete categories of emotion. The DES was formulated to gouge the emotional state of individuals at that specific point in time when they are responding to the instrument using the 5-point Likert scale (1- Not at All; 5-Extremely).
The Positive and Negative Affect Schedule (PANAS; Watson et al., 1988) was used to assess affect. The PANAS comprises 20 items, divided in 10 items for positive affect and 10 items for negative affect. In this study, only the negative affect scale was used, because we predicted that the cockroaches would elicit negative emotions of fear and disgust. Responses from participants are rated on a five-point Likert-type scale that ranges from 1 (very slightly or not at all) to 5 (extremely). The total score for this instrument was computed through the average of the responses to the 10 items comprising the negative affect scale. In a present study the internal consistency of the negative affect scale was high (α = .88).
2.3 Apparatus
2.3.1 Application development
A physiology-driven adaptive VR app, such as the one we developed, requires knowledge and expertise in game design, game programming, psychology, and data analysis. Likewise, the application development required a physical setup for user monitoring using biosensors, a 3D digital environment, a 3D-TV calibration, and the user design interaction. This section briefly summarizes the development and integration of these components. Using expertise from Environment Design (3D digital modeling), Game Programming, and Game Design, it was modeled an interior space to simulate a room that could give a sense of presence of the patient into a new area of interaction. The space was developed in low-poly resolution 3D and exported into a game engine (Unity). Furthermore, the textures were adjusted with techniques of baking light and Ambient Occlusion to create an aesthetic digital space, comparatively lifelike, although optimized for a better computing performance and, consequently, adapted for the active 3D-TV system (1080P/60 Hz). If the level of immersion is defined by the physical properties of the environment (Slater, 2009), such as agency, visual representation or surrounding sound, then, using a desaturated and monochromatic screen color spectrum could notably create a smooth and engaging environment. Due to the fact the user starts the simulation seated in front of a television, then the images projected on the TV screen were also quiet, to avoid any tension or anxiety on the experience of apparent disembodiment.
The following milestone required has consisted of 3D environment validation into a non-immersive VR system based on a Samsung 3D-TV. Consequently, after the debugging process, the game programmers released the prealpha demo, being further validated by the psychologist researchers. The artificial intelligence of the cockroach system was adapted to reactions of each bug in the environment (collisions, occlusions, scale, movement, among others), which was dependent on cardiac data input (See Figure 1). This physiology-driven adaptive VR environment was designed to evoke stress and even mild discomfort when cockroaches are displayed. As this device is not compatible with the Unity graphics engine by plug-and-play, it was decided to create an application that could transmit the information acquired by the sensors Biosignalsplux directly to Unity in order to reduce the latency of the simulation.
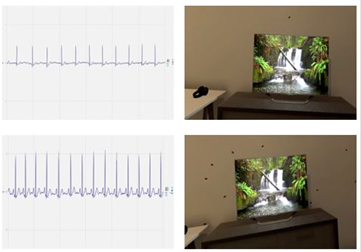
Figure 1 Users cardiac activity (left side) used as input by the VR system to determine the number of cockroaches within the scenario (right side)
However, for a better optimization in the software development process, we developed a software application in JAVA to connect the data captured by the Biosignalsplux sensors with Unity, to what we call BiosignalsPlux Unity Integration software (Figure 2). The Java application reads the data from the device, sends it to a remote database (SQL Server) so that Unity can read it. The latency of reading the data from the graphics engine, whether within the database network or external network, was averaging only 10 milliseconds. Despite this limitation, the implementation of data reading remotely has three major advantages: a) Reading and interpreting data can be done in separate locations; b) All data can be saved and accessed on a secure server; c) There can be multiple accesses to the data, updated in real time or later through the database.
A new debugging test session was developed, running the simulation displayed on a Samsung 3D-TV with BiosignalsPlux Unity Integration software to capture physiological data. With the low latency checked as also the liability of software and hardware architecture design, the pre-alpha release was evaluated into new proof of concept (alpha release). After the battery of tests made it at MovLab facilities (Laboratory of Interactions and Interfaces from University Lusófona) with several users from different research domains (Videogames, Biomechanics, Sound, Psychology, Film, and Communication Sciences), it was validated the ability to capture physiological data, the user interface design, and the exposure of users to the non-immersive VR system. The milestone achieved was a new software release to the Beta version of Cockroach VR environment for the participant-testing stage.
2.3.2 Cardiac Activity Recording and Processing
Regarding the physiological recording, we used, as mentioned above, the BiosignalsPlux, a Wireless realtime biosignals acquisition unit with multimodal sensors for recording cardiac activity data from participants (Figure 3).
Electrocardiography (ECG) was performed using a 2-lead system configured to Biosignalsplux, sampled at 1000 Hz. Disposable surface ECG electrodes were placed 2cm below the clavicle and 2cm medially from the anterior medial edge of the deltoid, and the other, half the distance between the final rib and the superior iliac crest. ECG data were downsampled to 60 Hz and exported from the biofeedback app to MS Excel in order to be analyzed in IBM-SPSS. ECG data was then trimmed with offline filters based on absolute and difference criteria. Specifically, artifacts were removed for heart rate values above 150 BPM (Beats Per Minute) or below 40 BPM; and for differences larger than 35 BPM between consecutive sample points for each 1000ms time window. The baseline was calculated for the first 60 seconds of exposure and then compared to each 30s epoch during the 4 minutes of exposure. Positive difference values indicated a BMP rise, whereas negative differences represented a decrease. After baseline recording, two cockroaches were presented in the environment, and if a difference of at least +10 bmp in relation to baseline was verified, cockroaches would be introduced gradually to reach the limit of 1000. In case of a BMP decrease, cockroaches would be gradually removed from the VR environment, indicating that the user could control their HR up to the initial average values.
2.4 Procedure
After ethical approval by the Ethics and Deontology Committee for Scientific Research (CEDIC), participants were recruited as part of a Cyberpsychology Course experimental demonstration. Each student invited to participate had the option do decline their participation at any stage of the experiment, since the participation was not mandatory or involved in the course grading. After reading and given their written consent, each participant would then complete the first part of the self-report measures. Once concluded, they were directed to a room with a 3D-TV setup (Samsung active 3D 46-inch SmartTV Plasma TV, Model UE46F800) of the virtual room environment and start being monitored for their HR, as seen in Figure 4.
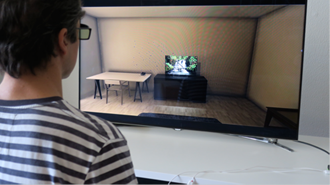
Figure 4 Laboratory setup for recording cardiac data from a participant interacting with our VR scenario via 3D-TV
Participants were informed their physiological activity would have an impact on the number of the cock-roaches. During the first minute, there was no exposure to stimuli, so that it was possible to establish a baseline for each participant. After this initial minute, for the following 4 minutes each participant was exposed to the non-immersive VR simulation of a room in which cock-roaches would appear based on the level of physiological activation (based on HR). After this 4-minute period, the participant would be directed to another room to complete the second part of the self-report measures and after completion, a 5 minute debrief about the experience.
The experiment was conducted in the Laboratory of Experimental Psychology (Labpsiexp). The experiment took around 20 minutes.
3. Statistical Analysis
Statistical procedures were based on descriptive and inferential analyses. Firstly, descriptive statistics were conducted to describe the data collected through the self-reports. Secondly, the correlations between self-report data with the activation through the heart rate at the first, second, third, and fourth minute of exposure were also conducted using the Pearson (r) coefficient.
Inferential statistics were then performed to: 1) analyze whether there were differences on self-report measures across the different groups of fear of cockroaches via a non-parametric ANOVA; 2) examine whether HR differ during the 3D-TV exposure to the cockroach stimuli between different fear groups; and 3) analyze whether the introduction of cockroaches in the VR scenario due to cardiac acceleration (yes vs. no) is associated with the fear of cockroaches. The HR analysis was performed via Generalized Estimating Equations (GEE) with one within-subjects factor with four levels representing the HR average for the first, second, third, and fourth minute of exposure; cockroach fear group as the between-subjects factor, while controlling for the emotional experience (PANAS) during the virtual reality exposure. The GEE approach was preferred due to its robust properties in parameter estimation in within subject designs and it did not require the outcome variable to have a particular distribution (Rosa et al., 2019; Ziegler, 2011). A GEE with gamma distribution with log link was chosen due a positive skewness of data. A M-dependent (M=3) working correlation matrix was estimated. This type of correlation structure was preferred as the Correct Quasi-likelihood under the Independence model Criterion (QQIC) was the lowest (Cui & Qian, 2007). As the HR baseline values did not significantly differ between fear groups, the outcome measure was examined in absolute values. Sidak correction was applied to all post-hoc tests. A chi-square of independence was performed to examine the association between the fear of cockroaches (3 groups) and the introduction of cockroaches in the VR scenario (yes vs. no), due to BPM increasing. In case of a statistically significant chi-square, the adjusted standardized residuals were computed to identify the cells with the largest deviations from expected values, using a cut-off of +/- 1.96 as recommended by Sharpe (2015). All statistical procedures were performed with IBM SPSS v.25 (SPSS Inc., Chicago, IL, USA) with a level of significance of 5%.
4. Results
Descriptive statistics, such as minimum, maximum, mean, and standard deviation was examined for each self-report measure under this study (Table 1).
Table 1 Descriptive statistics for self-report data
M | SD | Min | Max | |
FCQ | 0.91 | 0.85 | 0.00 | 2.94 |
Fear of cockroaches -single item | 3.25 | 2.37 | 1.00 | 8.00 |
Disgust of cockroaches -single item | 5.81 | 2.74 | 1.00 | 10.00 |
PANAS (negative affect) | 1.18 | 0.63 | 0.00 | 2.50 |
Disgust felt during the exposure | 2.25 | 2.02 | 1.00 | 9.00 |
In regards to the association between HR and fear of cockroaches, a significant and moderate relation was found at the third moment of assessment (r = .343; p = .047), indicating that a higher level of fear of cockroaches is associated with higher HR activation at this moment of exposure.
Furthermore, the FCQ score was used to set apart 3 groups, according to the 33.3 and 66.6 percentiles of this distribution (P 33.3 = 5; P 66.6 = 20, rounded up to the nearest whole number). As expected, non-parametric ANOVAs showed significant differences on both negative affect and fear of cockroaches during the exposure (single item) between fear groups (low vs. moderate vs. high self-reported fear of cockroaches) (p < .05). However, no differences were found for disgust, indicating that each group was distinctive in terms of experienced sensations along the exposure.
In regards to HR at different moments of the 3D-TV exposure between different groups of fear of cockroaches, results have shown only a main effect of fear group [Wald χ 2(2) = 6.02, p = .049], showing that high fear group had more pronounced HR activity than the low fear group. However, no other significant differences were found between fear groups (see Figure 4). Neither a main effect of moment [Wald χ 2(3) = 3.90, p = .273], nor an interaction effect [Wald χ 2(6) = 11.20, p = .082] on HR were found.
As concerned to introduction of cockroaches into the VR scenario due to HR, chi-square results showed a significant relationship between the introduction of cock-roaches and the fear group χ 2(2) = 6.98, p = .031 (Figure 5). A more detailed analysis indicated that the high fear group had a higher percentage of cases with introduction of cockroaches into the scenario (Z adj = 2.6) than in the low fear group (Z adj = −1.7) and moderate fear group (Z adj = −.9).
5. Discussion
This study was intended to serve as a proof of principle that measurement of HR is sensitive enough to emotional response (fear), from which it is possible to adjust a VR environment in real-time. According to the results obtained, our physiology-driven adaptive VR environment was effective in eliciting fear of cockroaches, as the group with high fear of cockroaches presented higher HR and reported the VR environment more negative in terms of affective impact than the group with low fear of cockroaches. These results corroborate our first hypothesis, supporting the use of our VR environment for fear elicitation. The findings also showed that individuals with higher levels of fear of cockroaches were more likely to trigger cockroaches into the scenario, demonstrating that HR is a sensitive physiological index to measure changes associated with a fear emotional response, which is in line with our second hypothesis.
From a technical standpoint, measuring HR using the Biosignalplux system presented some advantages. First, HR is a physiological measure that minimizes the
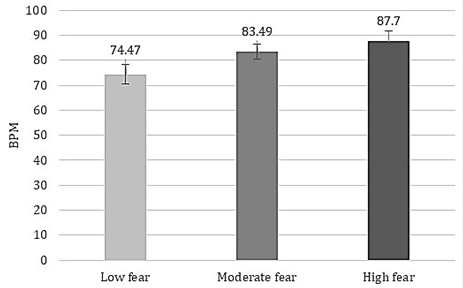
Figure 5 Heart Rate in VR Environment as a function of the fear group after controlling the emotional experience
delay between physiological changes and the adjustment made in the VR environment. Second, it is more available in real life through portable devices such as smart-phones or pulse watches. Besides, the Biosignalplux system is an affordable recording system with numerous sensors (e.g., EMG, EDA), with an extremely compact size, modularity, and several APIs that can be freely used, potentially making physiology-driven adaptive VR environments more cost effective for pilot studies or researchers/therapists with limited funding. The use of 3D-TV is also advantageous, especially these kind of VR environments can be used in the comfort and intimacy of users home. Moreover, 3D-TVs have usually a refresh rate of a minimal 240 Hz, which minimizes movement blur while interacting with the VR scenario.
The physiological capture we have presented could be beneficial for intelligent systems that may be able to recognize and react according to users (trainee or patient) biological activation, and designed to promote emotional regulation (Gamito et al., 2011a, 2011b). We find our results promising considering the number of cockroaches introduced into the scenario was dependent of users HR acceleration, thus our adaptive VR environment could also be part of wider biofeedback-enhanced VR systems for enhancing self-control, by teaching individuals how to monitor their own emotional/psychophysiological responses, which is known as an efficient method of emotional regulation (Peira et al., 2014). Further-more, eye movements can be easily recorded not only in non-immersive VR environments (e.g., Rosa et al., 2019), but also in immersive environments as well (e.g., HTC VIVE Pro Eye or VARJO VR-2 Pro), providing rich information regarding attentional processes. Thus, physiology-driven adaptive VR Environments could be also developed for eye input integration (e.g., displaying an arousing object/element in the VR scenario when the user shows fatigue or distraction via blink rate/blink duration analysis (Margalhos and Rosa, 2016, Rosa et al., 2015, Rosa et al., 2017).
Despite the interesting results, some limitations should be addressed. Firstly, the sample is unbalanced in terms of gender, which is related to a small representation of males students in the Psychology course. Secondly, this study considered a 4m VR session and did not cover long-term effects. Additionally, there is a possibility that a larger clinically meaningful difference in fear levels between both groups (control vs. phobia of cockroaches) would lead to a different HR response to cockroaches during our task. Altogether, our results point out that our physiology-driven adaptive VR environment is a valuable technique, with good acceptance by students (non-clinical sample), but it is also important to conduct future studies with clinical samples. Under a micro perspective, the findings reinforce the value of using VR stimuli associated with physiological recording (van Rooij et al., 2016). Under a macro-perspective, our physiology-driven adaptive VR environment has a potential use within the biofeedback-enhanced VR techniques, which has a possible future application in the health care customization.