1. Introduction
A negative social connotation has surrounded the term stigma. Generally defined as a mark of shame, it may seem that the base for the phenomenon of stigma is the idea that there is a generalized collective type, that is, the shared consciousness of the majority of society that makes the rules, whose infraction constitutes a criminal deviation (Durkheim, 1895-1982, p. 101), and the individuals who commit it are outsiders (H. Becker, 1966, p. 9). Thus, having a stigma is almost a curse for people who, due to their circumstances or qualities, have been stigmatized and must face particular challenges related to restricting their full participation in society, for example, through the exclusion of opportunities that generate income and employment (Rodríguez et al., 2015; Koschorke et al., 2014).
According to Goffman, stigma is “a special discrepancy between virtual and actual social identity [body, and individual character, among other categories and attributes]”; an undesirable difference between the “in fact” possessed and proved individual characteristics, and “the stereotype of what a given type of individual should be” (1963, p. 3). Now, the step from stigma to stigmatization relies on the reactions of the normals -people who do not depart negatively from the particular expectations at issue. That implies the practice of several types of discrimination that reduce life chances to those with a stigma and whose origin lies in their dehumanization.
The phenomenon of stigma and consequent stigmatization, discrimination, and social exclusion are dynamic. The meaning of the stigma is conditioned and influenced by the beliefs, attitudes, and social context of any given time; therefore, there are many variations in its meaning regarding existing geographical and cultural differences (Mason et al., 2001).
For instance, G. Becker (1957) provided the first approach from economics to address this kind of social issue with an economic model of competition, where individual utility-maximizing behavior may include tastebased discrimination. Phelps (1972) and Arrow (1973) also contributed with the term statistical discrimination, stating that due to limited information about job applicants’ skills, employers use observable characteristics such as gender or race to infer expected productivity. Based on social psychology, the economists Akerlof and Kranton (2000) considered that both identity and monetary incentives have a crucial role in decision-making and economic outcomes. According to them, identity economics provides an alternative approach to understanding human behavior and the nature of discrimination and suggests a new way to reduce disparities, e.g., equilibrium employment.
However, most economic research has focused on broad discriminatory actions and social exclusion measures, and although stigma and stigmatization are closely related to them, they are different constructs. Since discrimination is a resulting phenomenon, distinguishing these resulting actions from the origins of the thoughts underlying stigma and stigmatization emergence could provide a foundation for more complex interventions to address the root and reduce the levels of these social issues. In summary, the theoretical elaboration of stigma and stigmatization in economics requires an in-depth, multidisciplinary, and interdisciplinary approach to understand better the nature of their emergence and their close relation with other social phenomena.
Now, the neoclassical school of economics characterized human behavior based on the Homo economicus model, which refers to perfectly informed rational agents whose main objective is to optimize, i.e., to maximize their utility and benefit, taking into account their own preferences and interests, which implies “that agents have infinite cognitive abilities [. . .] and infinite willpower [. . .]” (Thaler, 2016, p. 1578). This idealization left aside the true human nature that, based on the evidence, shows the existence of the so-called “rationality failures” or inconsistency in the agents response to incentives (McFadden, 2009) and a limited capacity of the human mind.
Behavioral economics revolutionized mainstream economics. First, Simon (1957; 1976) introduces the concept of bounded rationality that emerged from empirically known human thought processes and choice architecture, particularly the limited human cognitive capacity in discovering alternatives, computing their consequences under certainty or uncertainty, and making comparisons between them. Later, in the 1970s, Amos Tversky and Daniel Kahneman, with their research in cognitive psychology, argued that the core characteristic of boundedly rational individuals was not poor reasoning, but that “intuition and reasoning are alternative ways to solve problems” (Kahneman, 2003, p. 1469). As a result, based on the idea that an intuitive system (fast and automatic) has a fundamental role in human cognition for decision-making, it appears essential to understand on a cognitive level how this system influences the attitudes and preferences that individuals adopt consciously and deliberately (rational system), e.g., in the case of this study for the emergence of stigma and stigmatization.
Behavioral economics1 offers a framework of analysis, i.e., a set of assumptions about human economic behavior that encompasses many research methods, e.g., experimental economics. Experimental economics is considered a methodological approach collaborative to the behavioral economics research program, and it can be defined based on experiments application as the primary research tool to address economic questions (Loewenstein, 1999). Behavioral economics enhanced neoclassical economics, taking into account insights from other disciplines such as psychology, cognitive sciences, social sciences, and empirically supported evidence on human behavior (Dhami, 2016) to improve the reliability of analyzing individuals’ decision-making process and explaining results in socio-economic situations.
These social phenomena are traditionally subject matter for social psychology, understood as the “scientific study of individuals feelings, thoughts, and behaviors” (Gilovich et al., 2016, p. 4). However, as a research program, behavioral economics addresses related issues through the lens of assumptions such as bounded rationality, social preferences, heuristics, and biases that founded its theoretical models.
Taking behavioral economics into consideration seems relevant to address the roots of these social phenomena, and based on a systematic review, this research intends to answer the following question: What are the cognitive factors underlying the decision-making process that influence the emergence of stigma and stigmatization in individuals, and how is this related with the experience of discrimination in their socio-economic lives? Notably, the research and current empirical evidence from the economic sciences reviewed herein were found to be insufficient, and there is no background record of the application of behavioral economics with the explicit purpose of gathering information with a cognitive insight that helps to clarify the complexity of this subject. Thus, this systematic review seeks to identify original studies, theoretical and methodological underpinnings in behavioral economics on the emergence of stigma and stigmatization within individuals relationship processes and their social structure.
2. Methodology
This systematic review uses PRISMA-P Group (2015) and PRISMA Group (2009) checklists, which describe the preferred reporting items for systematic reviews and meta-analyses (for protocols and reports). Likewise, the suggestions in guides and handbooks by The Cochrane Collaboration (Higgins et al., 2011), The Campbell Collaboration (2019), and The Joanna Briggs Institute(2014) -organizations with more than 20 years of experience producing systematic reviews (synthesis) of research evidence on the effectiveness of health and social interventions- are taking into account in the development of each item of the protocol. Moreover, it considers the recommendations and tools in methodological books of systematic reviews/research synthesis (Cooper et al., 2009; Petticrew & Roberts, 2006; Saini & Shlonsky, 2012). The protocol specifications (Appendix A [digital, available on request]) were established in advance. They included eligibility criteria, information sources, search strategy, study selection, data collection process, and analysis methods.
2.1 Eligibility Criteria
Only primary research studies, framed in behavioral economics, reported between 19402 and 2019 and written in English, Spanish, German, or French, with participants encompassing general child or adult human populations, were considered. No study design (quantitative, qualitative, and mixed methods approach), study settings, or publication status (published or gray literature) restrictions were imposed.
Studies were selected according to the following inclusion criteria. These criteria are known as PICO items (Participants/Phenomena, Intervention/Application, Comparators, and Outcomes), and the studies must fulfill one of the alternatives in each of the elements of PICO items to be included in the systematic review.
2.2 Information Sources
Studies were identified by searching electronic information sources: EBSCO (Academic Search Complete, Business Source Complete, eBook Collection, eBook Academic Collection, EconLit, Fuente Académica Premier, MasterFILE Premier, PsycARTICLES), Emeraldinsight, JSTOR, ProQuest (PRISMA Database with HAPI Index, ProQuest Central, ProQuest Dissertations and Theses A&I), Science Direct, Scopus, Springer, Taylor & Francis, Web of Science, Wiley Online Library, Google Scholar, and EconPapers RePEc. Further manual searches were conducted of the gray literature and selected scientific journals: American Behavioral Scientist (September 1957-February 2019), Experimental Economics (June 1998-March 2019), Journal of Behavioral and Experimental Economics (April 2014-April 2019), Journal of Socio-Economics (Spring 1991-February 2014), Journal of Behavioral Economics (1972-1990), Journal of Behavioral Decision Making (January/March 1988-January 2019), Journal of Economic Behavior and Organization (March 1980-March 2019), Journal of Economic Psychology (1981-June 2019), and Judgment and Decision Making (July 2006-January 2019).
2.3 Search strategy
The strategy included searching all the relevant literature from 1940 to 2019 (all years available in the electronic information sources except Web of Science [1940-2018], Scopus [after 1940], Google Scholar for search abstracts [articles added in the last year]), in the languages previously stipulated. The Evidence-Based Checklist for the Peer Review of Electronic Search Strategies Tool - PRESS (McGowan et al., 2016, pp. 39-40) was implemented in preparing the systematic review search strategy. The specific search strategies were developed and adapted to syntax and index terms for each database, using the following keywords: “behavioral economics”, “heuristics”, “biases”, “stigmatize”, “prejudices”, “stereotypes”, “discrimination”, “social exclusion”, “decision making”, “intuition”, “judgment”, “rational choice”, “bounded rationality”, “institutions”, “rules of thumb”, “prosocial”, “antisocial”, “equality”, “fairness”, “impartiality”, “status”, “social approval”, “social psychology”. The search was conducted between 14th December 2018 and 24th February 2019 (see Appendix A for full details of the search strategy employed in Web of Science).
Table 1 Inclusion Criteria
PICO items | Inclusion Criteria |
Participants/Phenomena | Stigmatization, discrimination, stereotypes, prejudices, social exclusion, and heuristics and biases. |
Intervention/Application | a) Addressed theoretically or methodologically, the nature of the emergence of the stigmatization (prejudices, stereotypes, or discrimination) and its effects on individuals’ relationship processes and social structure. b) Analyzed and evaluated the decision-making process and its influence on the emergence of (self-) stigmatization (prejudices, stereotypes, or discrimination). c) Investigated the relationship between heuristics and biases theory and stigmatization. |
Comparators | a) Direct stigmatization (prejudices, stereotypes, and discrimination) and selfstigmatization studies. For example, the existence of stigmatization compared with non-stigmatization, differentials (by category/attribute) in the levels of discrimination related to the same type of stigma, and comparison among different types of stigmas. b) Behavioral, labor market, health, government, or educational interventions or applications that influence the phenomena. |
Outcomes | a) Different definitions, models, and theories of stigmatization (prejudices, stereotypes, discrimination, heuristics, and biases) were considered. b) Simple and composite indicators or measures of experiences of stigma, health, and well-being, psychosocial stressors, performance outcomes, coping and social support, and public policy and social change (Frost, 2011, pp. 826-831). |
2.4 Study Selection and Data Collection Process
Literature search results identified with the search strategies were retrieved and imported to the reference management software EndNote to deduplicate records. Then, a three-phase manual screening was undertaken. In the initial stage, the remaining reference records following deduplication were uploaded in the Covidence software package, and one of the authors screened titles and abstracts against the inclusion criteria. In the second stage, for all the studies that appeared to meet or possibly meet the inclusion criteria, the full text was obtained in EndNote, and one of the authors screened to definitively determine whether the inclusion criteria were met or not. Lastly, the data in each of the remaining studies were appraised by one of the authors to determine their inclusion in the synthesis. Data extraction (Jesson et al., 2011; The Cochrane Developmental, Psychosocial and Learning Problems Review Group, 2014; Torgerson, 2003), critical appraisal (Armstrong & Shapiro, 1974), and risk of bias (Higgins et al., 2011) forms were designed and developed with the use of Qualtrics. Regarding the list and definition of variables, this study has considered the PICO items, the theoretical approach (e.g., concepts, theories, or models), and the methodological approach (e.g., sample selection, size, characteristics, variables of interest, and experimental design).
2.5 Planned Methods of Analysis and Risk of Bias Individual Studies
A combined data synthesis strategy was implemented as follows. First, a narrative synthesis that relies on the use of words and text, given the methodological and conceptual heterogeneity of included studies, is not appropriate to conduct meta-analyses or other formal methods of pooling data statistically (Davis et al., 2014). Nevertheless, this approach goes beyond simply summarizing the findings and requires exploration within and across studies of “the effects of interventions or applications and the factors shaping the implementation of the interventions” (Popay et al., 2006, p. 11).
Second, although meta-analyses are used to identify statistically significant results, the meta-synthesis approach is meant to generate theory (Finfgeld-Connet, 2018).
Accordingly, the data synthesis approach in this review followed the techniques of textual descriptions of studies, tabulation, groupings and clusters, vote counting as a descriptive tool, thematic and content analysis, coding, categorizing, and diagramming with the use of NVivo.
Now, studies that developed theoretical models were critically appraised with an adaptation of the framework proposed by Armstrong and Shapiro, which comprises the following components to evaluate the quality of these studies: reasonable and comprehensive assumptions, i.e., it can be tested against empirical evidence or judgment of experts; the logical structure of the models, i.e., the mathematics used to develop the model can be checked from the basics to the final form; the possibility to replicate the outputs of the models; and the value of the model’s outputs, e.g., identification of improved policies (1974, pp. 63-65).
Studies using experimental economics methods were assessed with the Cochrane risk of bias tool (Appendix A [digital, available on request]). Each outcome within each study was evaluated on five domains for risk of bias: selection bias, performance bias, detection bias, attrition bias, and reporting bias. Further, an additional item called “other sources of bias” was included in reporting problems not covered in the other domains. Finally, a judgment about the risk of bias (low risk, high risk, or unclear risk) for the outcomes within and across studies was stated (Higgins et al., 2011).
3. Results
3.1 Study Selection
A total of 3459 studies were identified for inclusion in the systematic review. The electronic databases provided 2914 records, and an additional 545 papers were identified through manual searching. Following the removal of duplicates, 1522 records remained.
After the title and abstract screening, 1428 studies were considered out-of-scope and discarded along with five duplicate records. The full text of the 89 studies retained was examined in more detail. Of these, 62 were rejected because they did not meet the inclusion criteria: 36 studies not addressed theoretically or methodologically -through the lens of the decision-making process or cognitive heuristics and biases theories- the nature of the emergence of the stigmatization (or related phenomena such as prejudices, stereotypes, or discrimination); instead, they were focused, for example, on the economic consequences derived from the interaction and social influence of groups regardless of race, gender, and sexual orientation; 20 were identified as secondary research and 6 as secondary data collection; one additional study was discarded because the full text of the study was not available.
The 26 studies included in the systematic review, which spans from 2002 to 2018 (with a peak of 5 in 2017), are all written in English, so no translation had to be conducted. Of these, 4 relevant studies from the gray literature (doctoral dissertations) were obtained, but after an updated search, two of them were replaced with their most recent and published version, as Figure 1 shows.
3.2 Study Characteristics
Among the 26 included studies, only three pertained to the development of theoretical models; the others were economic experiments. 52% of the studies contained experimental designs classified as laboratory experiments, 17% as field experiments, 17% as lab-in-the-field experiments, and the remaining 14% as internet-based or webbased experiments. The experiments had a duration between one day to one year; sessions lasted, on average, 76.25 minutes, and the number and frequency of sessions reported were between one time or weekly (monthly) over one month (year). Most of the studies conducted one experiment with various stages, but four involved two experiments, and another three carried out three, four, and six experiments, respectively.
A few studies reported the specific methods used to recruit participants, sampling, and treatment assignment. Further, almost half of the studies used control groups for treatments, i.e., the experimental design was within-subjects.
Of the studies, 77% involved behavioral economics interventions or applications. In particular, two studies developed models -one relied on a selective attention and learning process, and the other on similaritybased predictions- that provide possible explanations for the formation of stereotypes and discrimination of outgroups, minorities, and immigrants.
Thus, experimental designs are related to behavioral game theory, specifically with economic games (or modified versions). Economic games consist of strategic interactions among individuals (“players”) under a set of rules for the sequence of moves to choose strategies, the information the players have when they choose and how the payoffs of the game are related to the players’ utility (Camerer, 2003). For example, the Dictator game was the most used in experimental studies included in the synthesis. Its standard version requires the participation of two players, the dictator (the only player with an active role) and the responder. Then, the experimenter endows the dictators with an integer amount of monetary unit, and the dictators must decide how much to transfer -the offer can take values between zero and the total amount of the endowment- to the responder. The dictators’ offer is always implemented (Forsythe et al., 1994). As Camerer proposes, the “Behavioral game theory is about what players actually do. It expands analytical theory by adding emotion, mistakes, limited foresight, doubts about how smart others are, and learning to analytical game theory” (2003, p. 3).
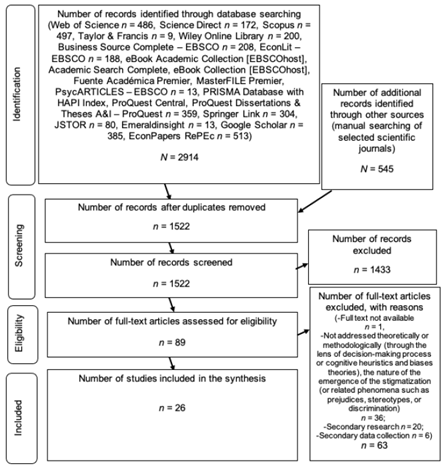
Note. PRISMA Flow Diagram. Adapted from “Preferred Reporting Items for Systematic Reviews and MetaAnalyses: The PRISMA Statement” by Moher et al. (2009).
Figure 1 Flowchart of Records Selection
Also, these studies take different approaches (socioeconomic and demographic surveys) aimed at testing their hypotheses. 15% of interventions studied the labor market, and 8% were dedicated to education sector interventions.
Concerning the labor market, one study was a model consistent with micro-labor studies on crime that characterizes an economic mechanism through which stigma could give rise to a socially inefficient equilibrium. The other three studies were experiments that directly examined bias in hiring, promotion, job assignments, working on a project, and willingness-to-accept (WTA) values to determine the cost of undesirable things through experimental designs where participants assumed the role of candidates, evaluators, or workers. Lastly, the two studies that conducted an application to the education sector were a lab-in-the-field and a field experiment, with real students and teachers as participants to assess perceptions of discrimination and discrimination in grading.
Table 2 Overview of Included Studies
Reference | Study type | Aims of the study | Phenomena | Intervention Application | Comparison | Outcome Measure |
---|---|---|---|---|---|---|
Ahmed (2007) | Experimental economics | To study how group identity, social distance, and intergroup bias may affect economic decision-making. | Discrimination | Behavioral economics | Direct study on discrimination | Experiences of discrimination (cooperate, stag, and dovish) |
Andreoni and Petri (2008) | Experimental economics | To explore the returns to beauty and gender. | Stereotypes | Behavioral economics | Direct study on stereotypes | Experiences of discrimination (average contribution and average payoff) |
Ben-Ner et al. (2009) | Experimental economics | To investigate the existence and relative strength of favoritism for in-group versus outgroup along with multiple identity categories. | Discrimination | Labor market | Direct study on discrimination | Experiences of discrimination (giving, sharing the office, work, and commute). |
Bjorvatn and Tungodden (2015) | Experimental economics | To study the impact of social identity on confidence and the role of disability identity in shaping confidence, preferences, and performance. | Stereotypes | Behavioral economics | Direct study on stereotypes | Experiences of (self) stereotyping (performance, confidence, risk-, time-, socialand competitiveness preferences) |
Bohnet et al. (2016) | Experimental economics | To examine whether evaluating candidates jointly rather than separately leads to individual performance playing a more important role than group stereotypes. | Stereotypes | Labor market | Labor market application that influences the phenomenon | Experiences of stereotyping and discrimination (candidates’ performance and evaluators’ choices) |
Candelo et al. (2017) | Experimental economics | To investigate how identity and social exclusion influence individual contributions to fund local public goods. | Social exclusion | Behavioral economics | Direct study on social exclusion | Public policy and social change (social identity, social exclusion, and amount contributed) |
Carlin and Love (2013) | Experimental economics | To advance a political theory of trust and reciprocity by testing dual-process strategies based on social identity and cognitive heuristics. | Stereotypes | Behavioral economics | Direct study on stereotypes | Experiences of discrimination (trust, reciprocity, partisanship, and amount sent) |
Chen et al. (2014) | Experimental economics | To investigate the effects of highlighting a common versus fragmenting identity on coordination and cooperation in a series of prisoner’s dilemmas and minimum effort games with varying incentives. | Discrimination | Behavioral economics | Direct study on discrimination | Public policy and social change (priming fragmenting identity versus common organization identity, coordination, and cooperation) |
Furuya (2002) | Theoretical model | To characterize an economic mechanism through which stigma could give rise to a socially inefficient equilibrium. | Stigmatization | Labor market | Direct study on stigmatization | Public policy and social change (welfare analysis -the marginal effect of social acceptance on social deviance and the size of the social cost relative to the possible private damage) |
Grimm et al. (2017) | Experimental economics | To investigate how and why people discriminate among different groups, including their own group and multiple outgroups. | Discrimination | Behavioral economics | Direct study on discrimination | Experiences of discrimination (transfers and beliefs) |
Grosskopf and Pearce (2017) | Experimental economics | To examine the discriminatory motives of the poorest people in England. | Discrimination | Behavioral economics | Direct study on discrimination | Experiences of discrimination (amount is given and closeness) |
Güth et al. (2009) | Experimental economics | To test whether the positive effect on the self from common group identity increased other-regarding behavior towards in-group members. | Discrimination | Behavioral economics | Direct study on discrimination | Experiences of discrimination (choices and beliefs -first and second-order- about average amount transferred) |
Halevy et al. (2012) | Experimental economics | To distinguish whether individual participation in intergroup conflict can be motivated by “in-group love”, “outgroup hate”, or both. | Discrimination | Behavioral economics | Direct study on discrimination | Public policy and social change (universal defection, intragroup cooperation, or intergroup competition - average amount kept or contributed-) |
Heinrich (2013) | Theoretical model | To get a picture of the forces driving negative stereotypes and to deduce policies to reduce them. | Stereotypes | Behavioral economics | Direct study on stereotypes and discrimination | Public policy and social change (average evaluation of group members by decision-makers and effects of affirmative action on in groupoutgroup and between-groups discrimination) |
Jenkins et al. (2018) | Experimental economics | To investigate how warmth and competence stereotypes influence social valuation and to what degree it is possible to predict people’s treatment of members of a wide variety of social groups. | Stereotypes | Behavioral economics | Direct study on stereotypes | Experiences of discrimination (social perception -warmth and competence stereotypes- and social valuation -equity preferences- according to the amount given) |
Meleady and Seger (2017) | Experimental economics | To examine the effect of imagined contact on cooperative behavior towards outgroup members. | Prejudices | Behavioral economics | Behavioral intervention/ application that influences the phenomenon | Experiences of discrimination (cooperative and competitive choices and trust) |
Ouazad and Page (2013) | Experimental economics | To estimate students’ perceptions of grading discrimination. | Discrimination | Education sector | Direct study on discrimination | Experiences of discrimination (students investment choice -number of questions bought- and grading practices -teacher and external examiner-) |
Pondorfer et al. (2017) | Experimental economics | To examine gender differences in stereotypes about risk preferences of men and women. | Stereotypes | Behavioral economics | Direct study on stereotypes | Experiences of stereotyping (risk preferences -own gamble choice and predictions of others’ gamble choices) |
Saccardo (2015) | Experimental economics | To present evidence of prejudice-based ethnic discrimination. | Prejudice | Behavioral economics | Direct study on prejudices and discrimination | Experiences of discrimination (prosocial and welfare-maximizing choices -payoffs- sending a false -a lie- or a truthful message, reject offers, beliefs about amount offered by subjects with different ethnicity names) |
Schwartzstein (2014) | Theoretical model | To draw out the consequences of selective attention in a model of an agent who makes forecasts based on available information. | Heuristics and biases | Behavioral economics | Direct study on heuristics and biases | Experiences of stereotyping and discrimination (systematically biased stereotypes and beliefs and limiting forecast) |
Shcherbakov (2016) | Experimental economics | To investigate stigma against individuals with ASD in the workplace. | Stigmatization | Labor market | Direct study on stigmatization | Experiences of discrimination (demographic questions, salary increase -willingness to accept-, social distance scale, knowledge of autism spectrum disorder [ASD], and taboo trade-off) |
Stanley et al. (2011) | Experimental economics | To investigate the relationship between implicit race attitude and social trust. | Heuristics and biases | Behavioral economics | Direct study on heuristics and/o biases | Relational performance (trustworthiness ratings, economic offers, and IAT scores). |
Tanaka and Camerer (2016) | Experimental economics | To measure the stereotyped attitudes of one group toward another. | Stereotypes | Behavioral economics | Direct study on stereotypes | Experiences of discrimination (impure altruism, altruistic punishment, reciprocity, trust, and coordination -according to the chosen amount in games-) |
Tsutsui and Zizzo (2014) | Experimental economics | To look at how changing relative group size and changing group status affects trust and trustworthiness. | Discrimination | Behavioral economics | Direct study on discrimination | Experiences of discrimination (trust -giving and return rates- and perceived value measurement) |
van Ewijk (2011) | Experimental economics | To examine whether ethnic majority teachers grade minority and majority students differently for the same work. | Discrimination | Education sector | Direct study on discrimination | Experiences of discrimination (grade and expectations for the essays attributed to different ethnicity students) |
Zeitzoff (2018) | Experimental economics | To measure how anger over past conflict influences intergroup relations. | Discrimination | Behavioral economics | Direct study on discrimination | Experiences of discrimination (altruism -monetary choices-) |
As an outcome measure, 81% (21/26) of the included studies had the experience of discrimination based primarily on stereotypes. During the experiments, the participants appeared biased, taking into account their treatment group identity. The decisions in economic games show more prosocial behaviors (trust, cooperation, and giving) to the in-group members than the outgroup members (see Appendix B).
Secondly, 15% (4/26) of the studies reported the potential of public policy and social change as an outcome. For example, stated implications for the resolution of real-world intergroup conflicts; recommended directions to generate full civic participation in mainstream society by immigrants and ethnic minorities; advocating for a mechanism to enforce participation in collective action (risk-sharing), to accept a stigmatized individual into the community for a positive general welfare effect.
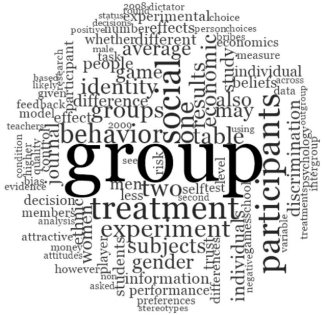
Note. This word cloud displays up to 100 words The criteria for the word frequency analysis were that the minimum length of characters in the word was three and the count of the number of times that the words occur included only exact matches, and excluded stop words, i.e., conjunctions or prepositions established by default NVivo.
Figure 2 Word Cloud of Included Studies
Lastly, one study established performance relational outcomes, i.e., identified implicit social bias as an independent factor contributing to the decision process. Although most of the studies are economic experiments, there was substantive heterogeneity across the interventions or applications, methods, comparators, and outcomes (see Table 2).
In terms of the quality of the three studies that presented theoretical models, all were comprehensively evaluated based on an adaptation of the framework proposed by Armstrong and Shapiro (1974), which comprises the following components: reasonable and comprehensive assumptions, the logical structure of the models, the possibility to replicate the outputs of the models, the value of the models outputs, identification of improved policies, among others.
Table 3 Search Strategies Keywords Frequency of Included Studies
Keywords | Frequency | Number of References |
Discrimination | 641 | 26 |
Bias | 501 | 25 |
Stereotype | 471 | 20 |
Status | 246 | 21 |
Equality | 208 | 23 |
Judgment | 184 | 12 |
Social psychology | 157 | 23 |
Stigma | 138 | 7 |
Social exclusion | 97 | 2 |
Prejudice | 88 | 15 |
Decision-making | 77 | 20 |
Prosocial | 73 | 5 |
Fairness | 69 | 17 |
Heuristic | 38 | 5 |
Behavioral economics | 36 | 14 |
Institutions | 35 | 16 |
Intuition | 29 | 6 |
Bounded rationality | 1 | 1 |
Rational choice | 1 | 1 |
Antisocial | 1 | 1 |
Impartiality | 1 | 1 |
Rules of thumb | 0 | 0 |
Social approval | 0 | 0 |
Note. The criteria to conduct the keyword frequency analysis in software NVivo were searching in all the 26 included studies; in the search for exact phrases, we enclose them in double quotation marks, e.g., “behavioral economics”, and the query included the search of words with the same stem, e.g., search for stigma and find and count stigmatization.
On the other hand, Figure 2 displays the Word Cloud3 resulting from the word frequency analysis using NVivo, and Table 3 shows the frequency of the keywords used in the search strategy across the included studies. Also, the risk of bias considerations within and across the 23 experimental studies resulted in an unclear risk of bias, given that all of them had one or more items judged as unclear. That is, there is no sufficient information to permit a judgment of “low risk” or “high risk”, using the Cochrane risk of bias tool (Higgins et al., 2011, pp. 8.7-8.8; Appendix A [digital, available on request]). Figure 3 shows the classification of studies for each one of the six items assessed.
3.3 Synthesis of Results
The included articles study how the behaviors of individuals -particularly in economic decision-making- towards others and themselves (Bjorvatn & Tungodden, 2015; Candelo et al., 2017) are significantly affected by their respective identities, intergroup bias, and social distance; for example, majority groups -high social status- versusminoritygroups-lowsocialstatus- (Shcherbakov, 2016; Tsutsui & Zizzo, 2014; van Ewijk, 2011).
Two studies show that in-group members’ favoritism triggers a risk of outgroup members facing discrimination (Ahmed, 2007; Halevy et al., 2012). However, Grosskopf and Pearce (2017) found the opposite: the existence of outgroup negativity. Estimating a structural model and counterfactual simulation performance revealed that individuals are willing to incur costs to avoid outgroup interactions. Therefore, the degree to which individuals’ identity with each other influences the extent of care about others; in other words, discrimination results from group-contingent social preferences.
Further, favoritism can be the reflection of guilt-aversion rather than group affiliation. Güth et al. (2009) elicited beliefs (expectations) before choices and partially proved this hypothesis. Specifically, in economic games, when the group identity is common and fully known. Through elicitation, individuals are aware and alert, and they may believe that in-group members have high expectations about them, that is, they expect to benefit from more generous actions during their interactions. Thus, individuals can show favoritism because they want to avoid the guilt of disappointing their ingroup members. However, if one individual can keep his identity hidden from the in-group members, his actions reveal that the in-group favoritism partly disappears. Also, some individuals, through indirect reciprocates expectation, can be more generous to the outgroup members if they expect that those individuals are generous to their in-group members (Grimm et al., 2017); or through perceived attitudes that can activate empathy such as warmth and competence (Tanaka & Camerer, 2016).
The ways that information is presented (framing effect) combined with selective attention and the process of learning under ambiguity (Heinrich, 2013; Schwartzstein, 2014) can influence the decision-making process, originating or overcoming stereotypes and discrimination (Bohnet et al., 2016).
In this context, Heinrich developed a model with the similarity-based approach to predict the result of collaborating with a specific group, where the individuals (decision-makers) do not have a priori group-specific preferences or beliefs (2013, p. 46). Thus, the ambiguity aversion4 of decision-makers and the quantity of information about a group influence the average evaluation of the group members. As a result, decision-makers prefer, on average, groups (members) they know well (in-groups, the majority, and the native group) to groups they know less well (outgroups, minorities, and immigrants).
Schwartzstein (2014) presented a model of an individual with selective attention, who learns to make predictions, for example, about others behaviors, based on ease of retrieval of available information. Then, the individual only attends to a variable that he believes is sufficiently predictive but has a confirmation bias that guides him to think that no matter which is the variable got his attention, it is important and informative. Thus, the formation of incorrect beliefs may rely on the persistent failure of individuals to learn whether a variable is worth attending to (omitted-variable bias), because their prior beliefs determine it. Consequently, if situational factors are not considered in the forecast, selective attention can lead to the other’s discrimination and stereotype, even when behavior may be context-dependent.
On the other hand, Furuya (2002) developed a model of community behavior, where the stigma -reflecting the fear of an incident due to criminal recidivism- acts as a barrier to obtaining employment for an individual with a history of deviance (criminal record). The author derived the two conditions under which stigma is likely to cause a socially inefficient equilibrium based on welfare analysis and micro-labor studies on crime. First, if for the potential employer the former criminal offender’s labor productivity is lower, and the expected damage to him (such as time lost from police interrogation or property damage) is greater; then, the certain expected social cost (ex-convict living on welfare or in the prison cell) increases with the denial of employment to the exconvict. Second, if the probability of recidivism is the same regardless of the ex-convicts employment status, then there would be a welfare gain from a public employment program.
In most studies, the main explanation behind these actions begins with social identity theory as the foundation -formulated by social psychologists Tajfel and Turner in the 70s and aligned with Goffman’s theory of stigma- and how the individual’s membership to a specific group is an essential source of pride and self-esteem. In an exercise of self-image, individuals enhance their groups status through social categorization (prejudices and stereotypes). Hence, prejudices and stereotypes mediated by the individuals identity appear to cause stigmatization and discrimination. However, given that individual social identity can be a compound of categories, there is no clear argument in the studies about the underlying cause of prejudices and stereotypes and what makes some categories more preferred or worse than others with the consequences of these social issues.
4. Discussion
Authors’ theoretical foundations about stigma and discrimination influence the formulation of their hypotheses, experimental designs, economic models, and findings. Therefore, the definitions used and the logical structure of their arguments need to be analyzed and critically assessed. In short, it appears that definitions are not clear enough, and the included studies are trapped in particular logical fallacies: “a type of argument that appears to be correct, but contains a mistake [or weakness] in reasoning” (Copi et al., 2014, p. 109).
4.1 Multiple and Vague Definitions
None of the included primary research starts with an explicit conceptual framework about their cited study phenomena, such as stigma, prejudices, stereotypes, discrimination, social exclusion, or biases. However, two possible explanations for this are as follows. First, it is possible to infer the definitions from their theoretical framework embedded in the introduction or background sections. Moreover, most of the included studies are experiments aimed at testing hypotheses and not at developing a new theory.
Because it is not possible to identify clear definitions, it could be deduced that a lack of clarity pervades the arguments produced and the research results, since the application of their findings could be ambiguous, at least, in the particular social phenomenon studied. Based on a logical framework, we analyze the conceptual strength by identifying the purpose and use of these specific definitions.
The mention of these social phenomena concepts in the included studies conforms to the purpose of stipulative and essentialist definitions, where they are the crucial elements for understanding social identity theory (Hughes & Lavery, 2008, pp. 43-44). For instance, a review of the stipulative definitions in social psychology about these concepts shows how they are closely related but still different. First, social identity is “that part of an individual’s self-concept which derives from his knowledge of his membership in a social group (or groups) together with the value or emotional significance attached to that membership” (Tajfel, 1984, p. 292). Second, the recognition of social identity is associated with a process of social comparison, social distance, and intergroup bias, along with social categories that can incorporate multiple attributes (Ben-Ner et al., 2009, p. 156).
Third, social distance is “the perceived affinity and nearness between people or groups” (Ahmed, 2007, p. 326). Furthermore, intergroup bias is the systematic tendency to evaluate own membership to a particular group and the in-group members in a more favorable way than the membership to the outgroup and its members (Ben-Ner et al., 2009; Hewstone et al., 2002). Simultaneously, associated phenomena emerge, such as stigma, ‘attribute that is deeply discrediting”; stereotypes, “beliefs or associations that link a whole group of people with certain traits or characteristics”; prejudices, “negative feelings about others because of their connection to a social group”; and discrimination, “negative behaviors directed against persons because of their membership in a particular group” (Kassin et al., 2014, p. 155).
On the other hand, “whatever their purpose, they [definitions] must enable the audience to know how to use the term following with its stipulated or essential meaning, and this means that they should not be obscure or circular” (Hughes & Lavery, 2008, pp. 49-50). In the included studies, some definitions fail to fulfill the authors’ purposes or have the appropriate essential details that support the set of definitions within the theory. For example, Furuya stated that stigma in his economic model “reflects each community members fear” (2002, pp. 281-283) that an incident exposes them to a private risk of suffering damage if they offer a job to an ex-convict and the ex-convict goes back to crime. In a hypothetical workplace scenario, Shcherbakov (2016) claimed that stigma is the presence of negative attitudes that comprise a system of beliefs that ultimately results in discrimination towards individuals with autism spectrum disorder (ASD). Although the sociological literature is the theoretical framework for both studies (Goffman, 1963; Link & Phelan, 2001), the stigma definitions mentioned before seem to necessarily imply the co-occurrence of other social phenomena closely related but distinct: the feeling of fear (prejudices) and the negative attitudes such as less likely to want to work with or to attend social events (discrimination).
Overall, the authors studied the phenomenon of discrimination and used social psychology literature as a theoretical framework, but they did not explicitly define the social phenomenon of discrimination, except for Ahmed (2007), who mentioned two definitions: discrimination-against and discrimination-in-favor. As the author proposed, discrimination-against is the “behavior observed when people treat anonymous people the same way they treat in-group members but treat outgroup members negatively”; discrimination-in-favor is “behavior observed when people treat anonymous people just as they treat outgroup members, but treat in-group members positively” (Ahmed, 2007, p. 35).
It may seem that authors confound the definition of discrimination with prejudices (Meleady & Seger, 2017; Saccardo, 2015) and with the meaning of intergroup bias associated with social identity theory. Social identity theory states that differential treatment of in-group and outgroup members is a natural human behavior essential for individuals’ identity. However, intergroup bias does not necessarily imply hostility, negativity, or discrimination against outgroups, i.e., favoritism towards the in-group may occur separately. As a result, authors focus their research on discrimination in subjects such as the following:
How and why people discriminate across multiple outgroups (Grimm et al., 2017; Ouazad & Page, 2013; Tsutsui & Zizzo, 2014; van Ewijk, 2011; Zeitzoff, 2018).
How discrimination is most likely a result of outgroup animosity rather than in-group favoritism (Grosskopf & Pearce, 2017).
How the occurrences of discrimination are a result of in-group favoritism [love] rather than outgroup hostility [hate] (Ahmed, 2007; Halevy et al., 2012) or guilt aversion is moderating the strength of in-group favoring (Güth et al., 2009).
How “family and kinship, political, religious and cultural beliefs and affiliations are important bases for discrimination in various contexts” (Ben-Ner et al., 2009, p. 167).
How priming or highlighting a common in-group identity can reduce or alleviate intergroup discrimination and improve overall cooperation (Chen et al., 2014), but social exclusion perceptions5 can negatively affect prosocial behaviors (Candelo et al., 2017).
Moreover, the authors did not state an explicit definition regarding stereotypes and used social identity theory (from social psychology) as a theoretical framework. Specifically, they argued that identity could shape the social perceptions or beliefs (stereotypes), and stereotypes can act as a mechanism to ease the categorization of people, highlighting features and differences between groups (Pondorfer et al., 2017), but also reinforcing intergroup bias depending on the social (stereotypical) perceptions towards outgroups in traits such as warmth and competence (Jenkins et al., 2018; Tanaka & Camerer, 2016).
As a complement to their theoretical framework, Andreoni and Petrie (2008), Bohnet et al. (2016), Carlin and Love (2013), Heinrich (2013), and Stanley et al. (2011) used the concepts of dual-process models, cognitive heuristics, and implicit and explicit social bias. According to the dual-process models, at a cognitive level, people have two modes of processing information for decision-making: system 1 (intuition) and system 2 (reason). As a result, the authors argued that stereotypes could act as cognitive heuristics or more intuitive and automatic decision-making mechanisms (implicit social bias) in situations with limited or minimal information or selective attention (Schwartzstein, 2014). They claimed that the amount of additional and available information allows a comparison about a stereotyped individual, which plays a crucial role in holding or eliminating a stereotype (social expectancy theory).
However, according to these studies, any social and individual perception or belief or intuitive judgment may seem to be a stereotype. Thus, the authors simultaneously confound stereotypes with a natural way of social categorization and social comparison. They also appear to concentrate only on negative stereotypes and assume that holding stereotypes is necessarily acting upon them. From that perspective, it is clear that this reinforces another misinterpretation mentioned before: intergroup bias and discrimination.
In sum, the included studies do not appear to have provided clear enough definitions or proposed circular definitions for these complex social phenomena, a definition that confounds two or more concepts around this kind of social issue. These limitations do not permit a correct understanding of the emergence of social stigma, stigmatization, and their relation to discrimination. Each phenomenon is associated with feelings, beliefs, reactions, or behaviors; for instance, at a cognitive level of analysis, it could have a single impact on the interpretation of the obtained economic outcomes in the experiments, but also for policy implications on how social identity can influence major life decisions in subjects such as cooperative behavior and prosocial acts (Ahmed, 2007, p. 336; Carlin & Love, 2013, p. 58; Grimm et al., 2017, p. 268; Grosskopf & Pearce, 2017, p. 41; Güth, 2009; Jenkins et al., 2018, p. 5; Tanaka & Camerer, 2016, pp. 18-20); gender gap (Andreoni & Petrie, 2008, p. 90); gender stereotypes and biases (Ouazad & Page, 2013, p. 129; Pondorfer et al., 2017, p. 13; van Ewijk, 2011, p. 1058); implicit social bias (Stanley, et al., 2011, p. 7714); persistent learning failures and disagreement, and the process of discovery (Schwartzstein, 2014, p. 1449); efficient job assignments, correct promotion practices, consequences of creating status-based group partitions, and fair treatment of people by organizations facing a diverse workforce (Ben-Ner et al., 2009, p. 167; Bjorvatn & Tungodden, 2015, p.277; Bohnet et al., 2016; Chen et al., 2014, p. 73; Shcherbakov, 2016, pp. 27-28; Tsutsui & Zizzo, 2014, p. 240); fighting market inequalities (Saccardo, 2015, p. 212); contributions to fund public goods (Candelo et al., 2017, p. 19); conflict resolution (Halevy et al., 2012, p. 194; Meleady & Seger, 2017, p. 14; Zeitzoff, 2018, pp. 413-417); antidiscriminatory policies (Heinrich, 2013, p. 53); and social inefficient equilibrium (Furuya, 2002, p. 289).
4.2 Where are the origins that matter?
It may seem there is a lack of conceptual clarity concerning the definitions of these social phenomena, limiting our understanding of their origins and change dynamics. Attempts to explain their interactions bring only more difficulties. For example, Ben-Ner et al. (2009) use a perspective based upon three theories: inclusive fitness theory, evolutionary theory, and social identity theory. They argued that these theories justify identity construction through differentiation among in-groups and outgroups.
For instance, regarding the inclusive fitness theory, Hamilton (1964) concludes that individuals behaviors toward others are context-dependent. Genetic relatedness and evolution through long-term affiliation constitute the most important relationships: family and kin, ethnicity, skin complexion, political and cultural views, and religion. Social species such as humans perform altruistic, spiteful, selfish, or mutually beneficial behaviors contributing to their inclusive fitness. Genes matched with a particular identity favor prosocial behaviors with those with a common genetic load.
According to this, the authors practice a kind of biological determinism, where the less the proportion of shared genes, the more social distance it can derive in discrimination. Supposing the authors chose the biological side, to make a comprehensive inquiry within the decision-making process framework, they had to analyze the existence, evolution, and role of human cognition and the possibility that the cognitive functions embedded in mental processes can significantly impact how is the emergence of these social phenomena.
Theoretical approaches derived in the above-explained context tend to (unintentionally) fall into the fallacy of composition, that is, it would be fallacious to argue that because this theory said that genes incline their bearers (applied in some organisms) to be altruistic toward those who share a percentage of genes, so a human being is also inclined to be altruistic toward those who share a given percentage of genes. Lastly, this argument does not consider the crucial role of social, cultural, and environmental factors. However, from those authors that only consider the sociological literature (Furuya, 2002, Shcherbakov, 2016) or social psychology literature with social identity theory (Ahmed, 2007; Candelo et al., 2017; Chen et al., 2014; Grimm et al., 2017; Grosskopf & Pearce, 2017; Güth et al., 2009; Halevy et al., 2012; Jenkins et al., 2018; Meleady & Seger, 2017; Ouazad & Page, 2013; Pondorfer et al., 2017; Saccardo, 2015; Tanaka & Camerer, 2016; Tsutsui & Zizzo, 2014; van Ewijk, 2011; Zeitzoff, 2018) to those authors that rely on dual-process models literature with heuristics or biases concepts combined with social expectancy theory (Andreoni & Petri, 2008; Bohnet et al., 2016; Carlin & Love, 2013; Heinrich, 2013; Stanley et al., 2011), the research synthesis analysis shows that it is not clear that the authors accomplished disentangling the roots of the emergence of these social phenomena, since, from the beginning, a misinterpretation of the theoretical framework can be deduced along with the confounding of definitions.
Finally, where are the origins that matter? Is human cognition exempt from each culture’s particular values or exempt from the influence of environmental and social factors? Could the functioning of the human mind’s dualsystem (intuition and reasoning) be generalized across subjects in all territories to explain the economic decisions that generate inequality in the world?
4.3 Circular Reasoning and Infinite Regress
We observe that the studies do not present a well-established sequence of causes and effects or consequences of these social phenomena, due to what seems to be circular reasoning and a hint of the problem of infinite regress. Several studies only considered experimentally testing theories developed in social psychology (social identity theory and intergroup bias) without critically revisiting the concepts and relationships involved.
Circular reasoning is a logical fallacy of presumption, also known as “begging the question” (or petitio principii, in Latin). The arguments are circular because the conclusion is included directly or indirectly in one of the premises to prove the conclusion. The general structure is A because of B, and Bbecause of A (Copi et al., 2014, pp. 140-142). In this case, the circular argument becomes less easily understood because the chains are a bit longer: discrimination exists because of stigmatization, stigmatization because of stigma, stigma because of prejudices, prejudices because of stereotypes, stereotypes because of social categorization, social categorization because of social identity.
The conclusion, in this logic, is usually restated as a premise in different words, or the premise that repeats the conclusion is suppressed, obscuring the fact that the conclusion depends on the suppressed premise (de Swart, 2018, pp. 504-505; Sinnott-Armstrong & Fogelin, 2015, pp. 323-324). Therefore, the key is in the last link in these social phenomena, that is, how discrimination ended explaining the social identity?
For example, Ahmed found that “discrimination occurs as an outcome of intergroup bias where discrimination is not a result of hostility toward outgroup members, rather being a result of in-group loving” (2007, pp. 335- 336), and argued that an explanation for the findings in his study could lie in social identity theory (Tajfel & Turner, 1986). According to that theory, recognizing social identity requires classifying people based on similar characteristics (social categorization). Consequently, the groups that individuals belong to are an essential source of pride and self-esteem.
As a result, social comparison and social distance appear to increase their self-image. Then, individuals enhance their group’s status, prestige, and success through intergroup bias. This bias includes behaviors (discrimination), reactions (stigmatization), feelings (prejudice), and beliefs (stereotypes) (Mackie & Smith, 1998; Tajfel & Turner, 1979; Wilder & Simon, 2001). In this context, stigmatization and discrimination contribute to recognizing individuals’ social identity and allowing them to emerge in society (Figure 4).
An infinite regress trail manifests in this series of related social phenomena, in which discrimination seems like the last member of the chain; however, the first member, social identity, does not fulfill the explanatory function that it is supposed to (Cameron, 2018; de Donato, 2011; Gratton, 2010). The circular arguments do not provide further insights into the nature of social stigma or discrimination.
Thus, there are currently no sufficient theoretical models or empirical evidence in economics that consider the existence, evolution, and role of human cognition itself around this subject. Interdisciplinary research is needed between economics and cognitive science on how implicit biases may lead to the reality of stigmatization, discrimination, and exclusion in which individuals and society live. Thus, what are the judgment and decisionmaking process factors that make all those beliefs, feelings, reactions, and behaviors emerge within humans and encourage them to face each other? If we assume that the emergence of these social phenomena is not spontaneous and that there is a factor or a set of characteristics that act as a trigger or cause, then the question appears to remain unanswered.
5. Limitations
According to PRISMA guidelines, at least two investigators must participate in the review process. Then the main limitation of this research is that the first author of this study was responsible for designing and writing its protocol and conducting all the stages for the systematic review (searching the literature, screening the included studies, extracting data, analyzing data, and writing the paper). However, as experts in economics and cognitive psychology, the second and third authors were responsible at each stage for discussing and refining search strategies, guiding, supervising, and reviewing the study selection, data analysis, and documents writing process. Also, this research was received feedback at academic meetings and conferences from colleagues and experts (whether in economics or behavioral and experimental economics).
On the other hand, the risk of bias assessment for all the included articles is not clear enough, due to the authors incomplete reporting of study designs in at least one of the items assessed.
6. Conclusions
To the best of the authors’ knowledge, this article represents the first systematic review that has sought to determine theoretical and methodological underpinnings in behavioral economics, trying to explain how stigmatization emerges within the relationship processes of individuals and their social structure. The review’s key findings are that most of the primary research identified was conducted through experimental methods and based on social psychology theories. Furthermore, it appears that economists’ misinterpretations of social identity theory have led them to confounding definitions associated with these social phenomena. This issue may obscure the differences and relations among stigma, stigmatization, stereotypes, prejudices, and discrimination and the factors underlying their emergence in society.
The analysis of the included studies from the behavioral economics literature shows that the research question that motivated this synthesis remains unanswered. Then, the research gap resides in applying the dual-system theory and the core behavioral economics concept of bounded rationality, heuristics, and biases connected with judgment and decision-making to disentangle the roots and relationships of these social phenomena.
Now, this is an opportunity to give rise to social innovation: new ways of thinking, strategies, tools, and projects from economic sciences that may help to solve the social problems facing the modern world. Thus, more research is needed to identify the cognitive processes behind the emergence and maintenance of these phenomena. Furthermore, there is ample scope to develop theoretical models that are embedded in this new insight with the potential to explore several experimental applications such as socio-economic reintegration (job market), migration, health-care discrimination, and institutionalized discrimination within the government.
We suggest these practical steps guide derived from the issues recognized in the discussion section when conducting research that implies an interdisciplinary approach: first, determine the relevant theories and their theoretical assumptions associated with each discipline to guide the research for the key variables and categories previously identified through a review; second, clearly define the key concepts or constructs within the context of the theories applied in each field of study; third, sketch the relations and the direction of dynamics between variables (concepts or constructs), and verify that the sequence of causes and effects did not fall in logical fallacies such as circular reasoning; fourth, describe and compare concepts, definitions, and theories that influence the social phenomena studied and explicitly state the contributions from each field in the interdisciplinary approach that will guide the analysis; finally, we encourage researchers to attend to the significance of well-established theoretical and conceptual frameworks to the quality and rigor required to verify and generalize interdisciplinary social phenomena study results.