1. Introduction
People tend to view the ability to recognize and identify an individual face as a straightforward process. However, face recognition is a complex phenomenon. Several models have been developed to explain the mechanisms behind face recognition. For instance, the interactive activation and competition model (Burton et al., 1999) and the sequential model of face recognition (Bruce & Young, 1986) suggest that to successfully recognize a familiar face, one has to perceptually process the face, encode its visual proprieties, and create an integrated representation of its configural/holistic characteristics. Then, this integrated representation has to be matched with traces of visual features and semantic/episodic information stored in long-term memory. On the other hand, the Face-Space theory states that faces are represented on a multidimensional space and that each dimension of this space corresponds to a different face feature (face shape, hair color, and length). According to this theory, each known face is represented by a unique point in space. The precise location of a face is determined by the value that each of its features receives in the scale of each dimension. The perceptual difference between two faces is determined by the distance between their locations in space (Valentine et al., 2016).
Past investigations that studied the mechanisms that underlie face recognition suggested that different processes are involved in familiar and unfamiliar face recognition1 (Stacey et al., 2005). For instance, familiar faces are recognized more quickly and accurately than unfamiliar faces (Ramon et al., 2011; Stacey et al., 2005). Additionally, recognizing a familiar face activates a set of brain regions -that store semantic, episodic, and emotional information about a specific familiar person- that are not engaged in unfamiliar face processing (Johnstone & Edmonds, 2009; Natu & O’Toole, 2011). On the contrary, unfamiliar face recognition is achieved by visually processing early structural representations (Bruce & Young, 1986). Familiar and unfamiliar face recognition are also susceptible to different detrimental factors unfamiliar face recognition is impaired by poor lighting conditions, and changes in pose, expression, context, and viewpoint. However, these variables do not have a significant detrimental effect on familiar face recognition (Longmore et al., 2017; Natu & O’Toole, 2011).
This suggests that unfamiliar face processing is more reliant on pictorial information, which becomes less important as faces become more familiar. Additionally, it has been suggested that the internal features of the face (eyes, nose, mouth) are more relevant than external features (hair, ears, face contour) in familiar face recognition (Johnstone & Edmonds, 2009). No particular advantage has been attributed to external or internal features in unfamiliar face recognition (even though a few studies suggested that external features are more important to unfamiliar face recognition) (Bruce et al., 1999).
Several face categories -famous faces (Gosling & Eimer, 2011; Nessler et al., 2005), personally familiar faces (Leibenluft et al., 2004), and experimentally learned faces (Dubois et al., 1999)- have been used to assess differences in familiar and unfamiliar face processing. The use of famous faces to assess this phenomenon has the advantage of providing rich pictorial, semantic, and episodic information to participants that may facilitate the activation of semantic memory traces during face recognition. Additionally, the continued exposure to famous faces, through media, allows for robust recognition across viewpoints, lighting conditions, and poses (Natu & O’Toole, 2011). However, Ramon et al. (2011) argue that famous faces may bias the results of familiar face processing experiments for at least two reasons: (1) there may be a wide range of exposure to each famous face across participants, and (2) the images of famous faces used in these experiments are often ‘iconic’ pictures of celebrities, which can lead to image-based recognition instead of familiar face recognition (e.g., the participants may recognize the picture without being familiar with the famous face). Image-based recognition relies on different mechanisms than familiar face recognition. Thus, to avoid such a confounding effect, investigations that use famous faces to assess differences in familiar and unfamiliar face processing should select famous faces that are highly familiar to all participants and should not select iconic pictures that can be recognized based on other pictorial features instead of the famous face. Additionally, these investigations must take into account that the level of familiarity of each famous face is constrained by geographic and socio-cultural variables: (1) a highly familiar famous face in the UK is not necessarily universally recognized in France; (2) a face universally recognized in a sample of elderly adults may not be recognized by all participants in a sample of teenagers (Lima et al., 2021). The need to consider the influence of variables, such as the nationality of the participants, led to the development of several databases of famous faces in recent years. For instance, Bizzozero et al. (2007) developed a famous face database and collected normative data for the Italian population. Lima et al. (2021) and Marful et al. (2018) conducted similar endeavors for the Portuguese and Spanish population, respectively. These studies followed extremely rigorous norms and procedures, which ensure that their stimuli can be used to study the underpinnings of familiar face recognition with reduced bias in samples with similar characteristics to theirs. However, these studies did not control the low-level proprieties of their images.
The existence of a high degree of variance in the low-level proprieties (brightness, contrast, movement) of the stimuli used to evaluate differences between familiar and unfamiliar face processing may also bias the results of investigations that seek to disentangle the mechanisms that underpin these two phenomena (Andrews et al., 2015; Bainbridge & Oliva, 2015; McCourt & Foxe, 2003). For instance, some authors (Knebel et al., 2008; Willenbockel et al., 2010) suggested that temporal differences in the detection of the first event-related potentials (ERP) associated with face processing may be caused by different methods of controlling variance in the lowlevel proprieties of experimental stimuli. Additionally, this variance causes an increase in the latency of neuronal responses in the primary visual cortex (Brannan et al., 1998), and in the preliminary phases of thalamic processing (Heap et al., 2018). On the contrary, controlling brightness and contrast histograms, which can be achieved by equating the means and standard deviations of brightness and contrast distributions and by matching the number of pixels at each brightness and contrast level, reduces the variation in neuronal responses in the primary visual cortex (Bainbridge & Oliva, 2015; Willenbockel et al., 2010). For instance, Park et al. (2015) used fMRI (functional magnetic resonance imaging) to assess the impact of varying several properties of visual stimuli on several regions along the occipitaltemporal cortex. They found that the response of V1, an early visual area, is significantly smaller if the spectral energy of the stimuli is equated, and their brightness histograms present a skew value of 0. Additionally, salient low-level features may also cause a confounding effect in pupillometry -high spatial frequencies are associated with a smaller pupil size (Cocker et al., 1994)- and oculometry studies -stimuli with salient low-level features receive more fixations- (Orquin & Loose, 2013). Thus, investigations that seek to evaluate high-level cognitive functions, such as familiar and unfamiliar face recognition, must ensure that the variance in the low-level proprieties of their experimental stimuli is homogenized between and within experimental conditions. This will ensure that detected effects are explained by high-level properties of the stimuli, and not by possible confounding effects modulated by an elevated degree of variance in their low-level proprieties (Knebel et al., 2008; Lakens et al., 2013; Willenbockel et al., 2010).
In this article, we present a database comprising famous faces, whose variance in the low-level proprieties (brightness, size, resolution) was homogenized. Additionally, the familiarity of the famous faces was assessed by a sample of Portuguese college students. The database is available at https://osf.io/x3vsy/. The complete set of images can be freely downloaded, transformed, and used for research purposes.
2. Method
2.1 Participants
The sample of this study consisted of 48 unpaid volunteers who attended the first year of the undergraduate course in Psychology at a university in Portugal. The minimum sample size needed to get reliable familiarity scores was based on previous studies that evaluated the same phenomenon (Gosling & Eimer, 2011; Nessler et al., 2005). Our study was advertised through word of mouth. The sample was collected by convenience the participants were directly recruited at the campus of the University. Each participant signed an informed consent form prior to the data collection session. All participants (41 females, 7 males; aged: 17-30 years; mean age: 19.02 years) were of Portuguese nationality and had normal or corrected-to-normal vision. This study was approved by the Ethics and Deontology Committee of the University of Aveiro (N◦40-CED/2019, approved on January 22nd, 2020).
2.2 Stimuli
The database consists of 183 famous faces. These images were selected and downloaded from the Wikimedia Commons website. Every selected image was licensed under a Creative Commons License, which enables the free distribution of copyrighted work (UNESCO, 2018). The selected famous faces were considered to be widely known by the general Portuguese public. The database includes Portuguese and foreign actors/actresses, musicians, athletes, TV personalities, politicians, scientists, etc. Each image was converted to greyscale, resized (397 397 pixels), and rotated (to ensure that the nasal bone presented a 0◦ degree angle with the horizontal axis of the image) in GIMP (v2.10). The images were converted to Portable Network Graphics (PNG). Their resolution was also homogenized (every image presents a 96dpi 96dpi resolution). An oval mask was applied to every image to hide the maximal amount possible of external features of each face (hair, ears, face contour), without hiding any of its internal features (eyes, nose, mouth). Additionally, the brightness of the images was homogenized by a custom-algorithm developed in Matlab. To achieve this, our algorithm estimated the brightness of each image (sum of the grey value of each pixel of the image divided by the number of pixels of the image) and the mean brightness of the entire set of images. Then, the brightness of each image was subtracted from the mean brightness of the entire set. This difference was then applied to the grey value of each pixel of the respective images. This ensured that the brightness of each image did not differ significantly from the mean brightness of the entire set. Some examples of the final versions of the famous faces are presented in Figure 1. Our algorithm can be freely used for research purposes and downloaded at https://github.com/PauloJFSFRodrigues/lowlevel-features. The algorithm can be applied to other sets of images to homogenize their brightness values. A detailed description of the features of the algorithm will be presented in another article.
2.3 Procedure
A cross-sectional design was used to assess the familiarity level of the famous faces included in the FamFac database. Data collection procedures took place at the university. A single group of 48 participants evaluated the familiarity level of all of the famous faces included in the FamFac database in a single session. The participants completed a task programmed on E-Prime (v2.0) to assess the familiarity level of the famous faces. Each participant completed the task individually on a PC. At the beginning of the session, the investigators presented verbal instructions on how to complete the task. The task did not contain any practice trials. Throughout the task, each famous face included in the FamFac database was sequentially presented to the participants in random order. In each trial, a famous face was displayed at the center of the screen and a textbox was presented below the famous face. The participants were asked to type some personal information in the textbox if they were able to identify the famous face (e.g., name, or other unique piece of information about the person). We considered that a famous face was correctly identified if the personal information provided by the participants corresponded to the famous face that was depicted (correct responses received a score of 1). Otherwise, the response received a score of 0.
2.4 Data Preparation and Statistical Analyses
2.4.1 Familiarity Scores
The familiarity score of every famous face was calculated by multiplying the proportion of correct responses by 100. In other words, to compute the familiarity score of each face we divided the number of correct responses provided by all participants by the total number of participants (48) and multiplied the resulting score by 100. Thus, the familiarity score of the famous faces could range from 0 to 100. We used the same cutoff points employed by Gosling and Eimer (2011) and Nessler et al. (2005) to classify a face as highly familiar. These authors deemed a face as highly familiar if it received a mean familiarity score above 75 on the scales that they used in their studies.
2.4.1 Power Analysis
The number of stimuli (famous faces) needed to carry the inferential analyses was calculated based on a priori power analyses computed with G*Power (version 3.1.9.7; Faul et al., 2007). An a priori power analysis was computed for each inferential statistical test that we conducted (a one-sample t-test, a Mann-Whitney U test, an independent samples t-test, a Kruskal-Wallis H test, and a between-subjects one-way ANOVA). As no previous study has assessed the same relationships that we evaluated in this investigation, we used a medium effect size (d = .5 for tests in which two means were compared, and f = .25 for analyses in which the means of 3 groups are compared) and an alpha value of .05 to compute each power analysis (Prajapati et al., 2010). The highest result of the power analyses (obtained for the between-subjects one-way ANOVA) suggested that a total sample of 159 famous faces was required to achieve a statistical power of .80.
2.4.3 Statistical Analysis
The data collected in this experiment was prepared and analyzed in Microsoft Excel 2016 and IBM SPSS (v28). A one-sample t-test was employed to evaluate if the brightness of each image did not differ significantly from the mean brightness of the entire set.
As mentioned previously, we applied an oval mask to each famous face to remove the maximal amount possible of external features without hiding its internal features. However, the amount of external features that we were able to remove varied from face to face due to several factors (e.g., viewpoint, face size). One of the factors that contributed the most to these differences was the variability in the hairstyles of the famous faces included in the database. For example, we were able to completely remove the hair of some celebrities with short hair, but we had to keep some hair in the case of celebrities who wore bangs. To evaluate if the amount of hair depicted in each picture had a significant impact on the familiarity scores of the famous faces, we split the database into three categories: faces without hair (65 images), faces in which a small amount of hair was kept -e.g., pictures in which we were unable to remove the widow’s peak, a V-shaped point in the hairline in the center of the forehead- (88 images), and faces in which a great amount of hair was kept -e.g., famous faces with bangs- (30 images). A between-subjects one-way ANOVA and a Kruskal-Wallis H test were computed to evaluate if the amount of hair depicted in the pictures had a significant effect in the familiarity scores of the famous faces. Three Kolmogorov-Smirnov tests and a Levene’s test were computed to evaluate if the three groups of images presented normal distributions, and to check if the variance in these three categories was approximately equal. The result of the latter test suggested that the variances of the three groups were not homogenous, F (2, 180) = 3.50, p = .03. The results of the Kolmogorov-Smirnov tests suggested that the group of images in which a small amount of hair was kept, D(88) = .07, p = .20, and the images in which a great amount of hair was kept, D(30) = .13, p = .20, presented an approximately normal distribution. However, the famous faces that did not presented any hair did not present a normal distribution, D(65) = .11, p = 0.05. As both assumptions of normality and homoscedasticity were not met, we followed the recommendation of Fife-Schaw (2006) and computed a between-subjects one-way ANOVA (parametric test) and a Kruskal-Wallis H test (non-parametric test) to evaluate if the amount of hair depicted in the pictures had a significant effect in the familiarity scores of the famous faces. As both these tests supported similar conclusions, we choose to present the results of the parametric test, because this test is more robust, which decreases the probability of making a Type-I error.
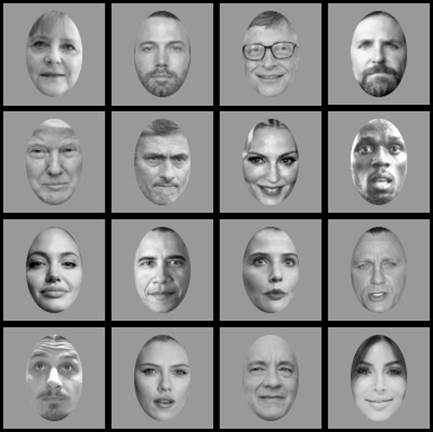
Note. First Row: Angela Merkel, Ben Affleck, Bill Gates, and Bradley Cooper; second row: Donald Trump, José Mourinho, Madonna, and Usain Bolt; third row: Angelina Jolie, Barack Obama, Cobie Smulders, and Daniel Craig; fourth row: Zlatan Ibrahimovi, Scarlett Johansson, Tom Hanks, and Kim Kardashian.
Figure 1 Examples of Famous Faces Included in the Database
Additionally, we wanted to ascertain if the participants were more familiarized with Portuguese/national famous faces or foreign/international famous faces. Thus, a Mann-Whitney U test and an independent-samples t-test were carried to assess the effect of type of nationality on the familiarity scores of the famous faces. Considering that the subset of Portuguese/national famous faces and the subset of international famous faces presented unequal sizes (N Portuguese famous faces = 17 vs. N International famous faces = 166), a Levene’s test was carried out to evaluate if the variance in the familiarity scores of the two subsets was approximately equal. The result of this test suggested that the variance in the familiarity scores of both subsets was approximately equal, F (1, 181)= 1.82, p = .18. Two Kolmogorov-Smirnov tests were carried to evaluate if the familiarity scores of the subset of Portuguese/national famous faces and the subset of international famous faces presented a normal distribution. The familiarity scores of the subset of Portuguese/national famous faces presented a normal distribution, D(17) = .13, p = .20. However, the same was not verified for the familiarity scores of the international famous faces, D(166) = .08, p = .02. As the assumption of normality was not met, we took the recommendation of Fife-Schaw (2006) into account once again and performed an independent samples t-test (parametric test) and a Mann-Whitney U test (non-parametric test). As both tests yielded similar results, we decided to present the results of the parametric test.
Effect sizes were estimated with Cohen’s d. The benchmarks suggested by Cohen (1988) were used to interpret the magnitude of these effects: small effect (d = .2), medium effect (d = .5), and large effect (d = .8).
3. Results
The brightness of each image was successfully homogenized by the algorithm developed on Matlab. Our results suggested that the brightness of each image did not differ significantly from the mean brightness of the entire stimuli set (M = 154.08, 95% CI [-.06, 0.06]), t(182) < .001, p > .99, d < .001, which indicates a small effect size. The brightness values of the stimuli are presented in the supplementary materials (see Table S1). An xlxs file with this data can be downloaded at https://osf.io/x3vsy/.
As previously mentioned, we used the cutoff point employed by Gosling and Eimer (2011) and Nessler et al. (2005)) to classify a face as highly familiar. Taking these metrics into account, 41 out of the 183 famous faces were highly familiar to our participants. The familiarity scores of the 183 famous faces are displayed in Table 1. This information can also be downloaded at https://osf.io/x3vsy/ in xlxs format.
The results of the between-subjects one-way ANOVA suggested that the amount of hair depicted in each image of the FamFac did not have a significant effect in the familiarity scores of the famous faces, F (2, 180) = .25, p = .78, η 2 < .01. The results of the post-hoc tests revealed that the familiarity scores of the images without hair (M = 47.88, 95% CI [41.22, 54.54]) did not differ significantly from the familiarity scores of the images in which a small amount of hair was kept (M = 50.42, 95% CI [45.11, 55.72]), or the images in which a great amount of hair was kept (M = 51.46, 95% CI [39.58, 63.35]) (all p > .05).
Additionally, the result of the independent-samples ttest, t(181) = 1.81, p = .07, d = .46, suggested that the familiarity scores of Portuguese/national famous faces (M = 60.83, 95% CI [48.57, 73.10]), and the familiarity scores of international famous faces (M = 48.55; 95% CI [44.43, 52.66]), did not differ significantly, although there was a tendency for Portuguese famous faces to have a higher familiarity score than international famous faces.
4. Discussion
The results of previous studies suggested that processing familiar and unfamiliar faces elicits different behavioral and electrophysiological responses (Bentin & Deouell, 2000; Gosling & Eimer, 2011; Ramon et al., 2011; Stacey et al., 2005). However, the results of electrophysiological and eye-tracking studies that seek to assess the different mechanisms involved in familiar and unfamiliar face processing may be confounded by several exogenous variables that exert a significant influence on the way that pictorial and structural codes of familiar and unfamiliar faces are processed and retrieved, such as the existence of significant variation in the low-level proprieties of the stimuli -e.g., brightness, contrast- (Knebel et al., 2008; Willenbockel et al., 2010); or the existence of varying degrees of familiarity with the faces presented to the participants (Ramon et al., 2011). Thus, investigations interested in disentangling the processes involved in familiar and unfamiliar face processing must ensure that each face presented to the participants is universally recognized, and that variation in the low-level proprieties of the stimuli is kept to a minimum. With these objectives in mind, we developed a database comprising 183 images of famous faces originally licensed under a Creative Commons License, whose low-level proprieties (brightness, size, resolution) were homogenized. The complete set of images is available at https://osf.io/x3vsy/ and can be freely downloaded, transformed, and used for research purposes.
We developed an algorithm in Matlab to automatically equate the brightness of the images included in the FamFac. The algorithm successfully homogenized the brightness of the set of stimuli -the brightness of each image did not differ significantly from the mean brightness of the entire set. Some studies suggested that some ERP components (e.g., P1 and N1) are sensitive to variations in the physical properties of stimuli, such as brightness (Schettino et al., 2016; Schindler et al., 2018). This effect is minimized when the variation between the low-level features of the stimuli is controlled for (Schettino et al., 2016). Considering that the brightness of each image included in the FamFac database was homogenized, it is possible to suggest that these stimuli should not elicit such confounding electrophysiological modulations. The algorithm used to equate the brightness of the images can be freely downloaded from https://github.com/PauloJFSFRodrigues/lowlevel-features. This code can be used to homogenize the brightness of other images sets.
As previously stated, investigations that use famous faces to study familiar face processing should ensure that the selected famous faces are highly familiar to all participants (Ramon et al., 2011). For instance, to guarantee that the famous faces that were going to be included in their main experiment were highly familiar, Gosling and Eimer (2011) asked eight participants to name and state the profession of a large pool of 129 famous persons. The famous faces that were included in their main experiment were those that were explicitly identified by at least six participants, which can be translated into a familiarity score of 75 on the scale that was used in our study. The same cutoff point was used by Nessler et al. (2005); these authors included a famous face in the set of stimuli that was used in their main experiment if its mean rating was higher than 3 (their familiarity scale ranged from 1 to 4). Taking these metrics into account, 41 out of the 183 famous faces included in the FamFac database were considered highly familiar by our sample. Our results also suggested that the familiarity scores of Portuguese/national famous faces and foreigner famous faces did not differ significantly, although approaching statistical significance (p = .07). Additionally, the subset of Portuguese/national famous faces consisted only of 17 stimuli. Thus, these results must be treated with caution. Ramon et al. (2011) suggested that varying degrees of familiarity with a given famous face between participants may lead to some additional variability in electrophysiological and ocular correlates elicited by this said famous face. Our results suggest that, in the case of the Portuguese population, there may be some advantage in using national famous faces to evaluate the mechanisms involved in familiar face processing, given that this subset presented higher familiarity scores than the subset comprising foreign faces. However, this difference did not achieve statistical significance.
Table 1 Familiarity Scores of the Famous Faces Included in the Database
Famous Face | Familiarity Score | Famous Face | Familiarity Score |
---|---|---|---|
Cristiano Ronaldo | 100.00 | John Travolta | 70.73 |
Ed Sheeran | 100.00 | Kim Kardashian | 70.73 |
Dwayne Johnson | 97.56 | Sandra Bullock | 70.73 |
Barack Obama | 97.56 | António Costa | 68.29 |
Justin Bieber | 97.56 | José Mourinho | 68.29 |
Miley Cyrus | 95.12 | David Schwimmer | 68.29 |
Albert Einstein | 95.12 | Jeremy Renner | 68.29 |
Donald Trump | 95.12 | Penelope Cruz | 68.29 |
Taylor Swift | 92.68 | Salvador Sobral | 65.85 |
Robert Pattinson | 92.68 | Sylvester Stallone | 65.85 |
Jennifer Aniston | 92.68 | Chris Pratt | 65.85 |
Zac Efron | 92.68 | Gigi Hadid | 65.85 |
Leonardo DiCaprio | 92.68 | Meghan Markle | 65.85 |
Diogo Amaral | 90.24 | Blake Lively | 65.85 |
Jackie Chan | 90.24 | Vera Kolodzig | 65.85 |
Emma Watson | 90.24 | Joana de Verona | 63.41 |
Katy Perry | 90.24 | Madonna | 63.41 |
Demi Lovato | 90.24 | Natalie Dormer | 63.41 |
Emma Stone | 87.80 | Sarah Jessica Parker | 63.41 |
Lady Gaga | 85.37 | Daniel Craig | 63.41 |
Angelina Jolie | 85.37 | Margot Robbie | 60.98 |
Kristen Stewart | 85.37 | Heath Ledger | 60.98 |
Selena Gomez | 85.37 | Luís Figo | 58.54 |
Ricardo Araújo Pereira | 82.93 | Hugh Jackman | 58.54 |
Scarlett Johansson | 82.93 | Paul Rudd | 58.54 |
Cara Delevingne | 82.93 | Kaley Cuoco | 58.54 |
Meryl Streep | 82.93 | Victoria Guerra | 56.10 |
Chris Hemsworth | 82.93 | Ezra Miller | 56.10 |
Rupert Grint | 80.49 | Vince Vaughn | 56.10 |
Anne Hathaway | 80.49 | Tom Hanks | 56.10 |
Dakota Johnson | 80.49 | Bradley Cooper | 56.10 |
Ellen DeGeneres | 80.49 | Alec Baldwin | 56.10 |
Ian Somerhalder | 80.49 | Ellie Goulding | 53.66 |
Maisie Williams | 78.05 | Danny Glover | 53.66 |
Eddie Redmayne | 78.05 | Cate Blanchett | 53.66 |
Michelle Obama | 78.05 | Ana Sofia Martins | 51.22 |
Débora Monteiro | 75.61 | Vladimir Putin | 51.22 |
Rui Unas | 75.61 | Felicity Jones | 51.22 |
Kanye West | 75.61 | Chris Rock | 51.22 |
Julianne Moore | 75.61 | Pope Francis | 51.22 |
Channing Tatum | 75.61 | Sofia Vergara | 51.22 |
Virgílio Castelo | 73.17 | Eva Longoria | 48.78 |
Angela Merkel | 73.17 | Christine Baranski | 48.78 |
Jason Momoa | 73.17 | Kate Winslet | 48.78 |
Ryan Gosling | 73.17 | Amy Adams | 48.78 |
Steve Jobs | 70.73 | Arnold Schwarzenegger | 46.34 |
Jason Statham | 70.73 | Claire Holt | 46.34 |
Kate Walsh | 46.34 | Miranda Kerr | 24.39 |
Maya Rudolph | 46.34 | Kate Mara | 24.39 |
Glenn Close | 46.34 | Jason Sudeikis | 24.39 |
Charlize Theron | 43.90 | Michael Douglas | 24.39 |
Jordana Brewster | 43.90 | George Bush | 24.39 |
Ben Kingsley | 43.90 | Sarah Hyland | 24.39 |
Olivia Wilde | 43.90 | Sacha Baron Cohen | 24.39 |
Kevin Costner | 43.90 | Carmen Electra | 21.95 |
António Guterres | 41.46 | Henry Cavill | 21.95 |
Octavia Spencer | 41.46 | Dan Reynolds | 21.95 |
Ashley Olsen | 41.46 | Stephen Merchant | 21.95 |
Ben Affleck | 41.46 | Howie Mandel | 21.95 |
Christian Bale | 41.46 | Clint Eastwood | 21.95 |
Jennifer Morrison | 41.46 | Evan Rachel Wood | 19.51 |
Kevin Bacon | 41.46 | Elon Musk | 19.51 |
Leighton Meester | 41.46 | LeBron James | 19.51 |
Dylan McDermott | 39.02 | Manuela Ferreira Leite | 17.07 |
Robert De Niro | 39.02 | Bill Murray | 17.07 |
Rachel Weisz | 39.02 | Monica Belluci | 17.07 |
Ewan McGregor | 36.59 | Dakota Fanning | 14.63 |
Victoria Justice | 36.59 | Ricky Martin | 14.63 |
Diane Kruger | 36.59 | Doutzen Kroes | 14.63 |
Giselle Bündchen | 36.59 | Christine Lagarde | 14.63 |
Susan Sarandon | 36.59 | Usain Bolt | 14.63 |
Zlatan Ibrahimovi | 36.59 | Claire Foy | 14.63 |
José Rodrigues Dos Santos | 34.15 | Ivanka Trump | 14.63 |
Chris Evans | 34.15 | Sienna Miller | 14.63 |
Cobie Smulders | 34.15 | Cynthia Nixon | 14.63 |
Mila Kunis | 34.15 | Carminho | 12.20 |
Bill Gates | 34.15 | Zidane | 12.20 |
Maggie Gyllenhaal | 34.15 | Theresa May | 12.20 |
Ashley Judd | 31.71 | Rafael Nadal | 12.20 |
Eric Bana | 31.71 | Christina Hendricks | 12.20 |
Meg Ryan | 31.71 | Shaquille O’Neal | 12.20 |
Tina Fey | 31.71 | Pep Guardiola | 9.76 |
Eric Stonestreet | 31.71 | Lake Bell | 9.76 |
Toni Kroos | 29.27 | Kevin Durant | 9.76 |
Jennifer Connelly | 29.27 | John Carpenter | 9.76 |
JK Simmons | 29.27 | Muhammad Ali | 7.32 |
Brendan Fraser | 29.27 | Sarah Silverman | 7.32 |
Conor McGregor | 29.27 | King Juan Carlos | 7.32 |
Demi Moore | 26.83 | Ashlee Simpson | 4.88 |
Sophie Turner | 26.83 | Ronnie O’Sullivan | 2.44 |
Sigourney Weaver | 26.83 | Jean-Claude Van Damme | .00 |
Naomie Harris | 24.39 |
For the sake of clarity, we will now discuss some of the limitations of this study. First of all, we would like to highlight that the number of famous faces with a familiarity score above 75 is relatively limited. We believe that this was due to the difficulty in finding a substantial set of high-quality images whose copyright license enabled us to freely use and transform them. For the same reason, the images included in the database have a low resolution (96dpi 96dpi). The oval mask that was superimposed on the faces to control for external and background features may also have harmed the familiarity scores of the famous faces. Nevertheless, we decided to keep the oval masks due to the high variability of the original images. We believe that keeping the original images without applying the oval mask would have introduced some bias in the familiarity scores because the participants could use the external features of the faces or other pictorial elements of the images to help them recognize the famous face, which could lead to image-based recognition instead of identity-based recognition. This was particularly true for our category of stimuli -famous faces- as some external features, such as hairstyles, could have been associated with the celebrity depicted. However, in the future, it would be interesting to evaluate if the familiarity scores would improve if the famous faces were presented without the oval masks. Importantly, the familiarity scores of this set of stimuli were assessed with a Portuguese sample. Thus, investigations that want to assess familiar face recognition with samples with different nationalities should evaluate the familiarity scores of the faces included in this database for these samples. Additionally, we recommend that investigations that intend to use this database to assess face recognition with Portuguese samples should choose their set of stimuli from the 41 famous faces that received a familiarity score higher than 75. The algorithm that we developed on Matlab successfully controlled the brightness of the images. However, several other low-level features (e.g., image complexity, movement, orientation, contrast) can cast a similar confounding effect in investigations that use electrophysiological or eye-tracking methods to evaluate high-level cognitive functions (Bainbridge & Oliva, 2015; Dragoi et al., 2000; Kamitani & Tong, 2006). Nonetheless, due to constrained nature of the present stimuli set (faces with ovals hiding all external and background features), we believe that those effects are limited in this set. Still, future studies with similar aims using stimuli with less constrained characteristics should ensure that the low-level features previously mentioned are also homogenized between their stimuli. The development of algorithms like the one we developed to control the brightness of visual stimuli could facilitate this procedure.
5. Conclusions
We believe that the materials made freely available for research purposes within this study (a famous face dataset anda brightness homogenizing algorithm) are particularly useful resources for studies of face processing, but can also be used in other studies which use visual stimuli and may need to control for low-level features. Furthermore, the original images wecollectedwere licensed under a Creative Commons License. Thus, any authors that wish to use this database can use the available stimuli, and transform them to fit the specific needs of their investigations.