1. Introduction
There is now a broad consensus in the literature that corruption, commonly defined as an abuse of public power for private gains, negatively impacts on the development of a country (Aidt, 2009). It is also widely agreed that this development requires a set of institutions and policies that stimulate both political and economic inclusion in order to facilitate growth and a sustainable development (Rodrik, Subramanian, & Trebbi, 2002; Acemoglu & Robinson, 2012; and Baland, Moene, & Robinson, 2010).
Corruption undermines development at two levels. On the one hand, corruption can be used to circumvent the existing rules, e.g. through bribes or favours, or by embezzling funds or manipulating information. On the other hand, corrupt practices can be used as a means to directly capture and influence the rules of the game. Indeed, the policies and institutions required for sustainable development are themselves to a large degree the result of a political process that is at risk of being influenced by interest groups. The fact that many countries struggle with the implementation of such "good" institutions and policies usually does not seem to be the consequence of a lack of knowledge, but rather the result of the influence exerted by certain actors who distort the policy making processes in order to safeguard and foster their own narrow interest in the status quo (Grossman & Helpman, 1994).
With the growing body of quantitative and qualitative evidence of these negative impacts of corruption, the importance of minimizing corruption has gained in importance both in the policy arena and in research. During the last 24 years, since the launch in 1995 of Transparency International's Corruption Perceptions Index (CPI), the research on corruption increased impressively.3 Perhaps one of the most fertile areas has been the cross-country empirical research into the causes and consequences of corruption (Lambsdorff, 2006). The findings have contributed significantly to improving our understanding of the phenomenon, but there are still many contested issues. For instance, the roles of the size of government, of cultural and historical factors, or of the level of democracy as determinants of corruption remain unclear.
Perhaps Danila Serra has undertaken the most thorough analysis into of the determinants of corruption (Serra 2006). The author conducts a global sensitivity analysis based on Leamer's Extreme-Bounds Analysis in order to detect which variables commonly used in the literature as determinants of corruption survive this robustness check. Her analysis finds that only five variables are robustly related to corruption. Countries with more years of continuous exposure to democracy, a higher degree of political stability, higher income per capita levels, and a higher share of protestant population, have lower levels of corruption. In addition, the colonial heritage (British) significantly predicts the present level of corruption of a country.
In turn, Billger & Goel (2009) contributed to the literature on the determinants of corruption by looking at the conditional distribution of the phenomenon across countries. Specifically, they wondered if determinants of corruption are different in highly corrupt countries and in countries with low levels of corruption. The answer to this question is relevant both in improving our understanding of corruption and from a policy perspective. If there are differences according to the level of corruption, then the policies adopted to controlling the phenomenon should also be differentiated4.
To answer their research question, Billger & Goel (2009) used a sample of 99 countries and a quantile regression model. Their results confirm some of the findings in previous contributions to the literature, but they also added new insights. For instance, the authors find that changes in the level of economic freedom do not have the expected corruption-reducing effects in countries with high levels of corruption. In turn, the level of democracy does reduce corruption level in the most corrupt countries, while the effect of the size of government is ambiguous. However, the authors did not include some variables that from a theoretical point of view could be relevant in explaining corrupt levels; and limited the data set to information from the years 2001 to 2003. We furthermore argue that some of the variables they used were not specified adequately, as we will explain in more detail below.
Therefore, our contribution aims at answering the question raised by Billger & Goel (2009). To do this we improve the model by adding additional variables empirically found relevant by Serra (2006), increase econometric rigour and use a more representative data set. Our database updated for the year of 2018 and comprising 170 countries is, to our best knowledge, currently the broadest dataset used in this type of research.
Our findings confirm that there are differences in the determinants of corruption across countries according to their levels of corruption. The variables size of governments and proportion of protestant population are good predictors of corruption only for countries with specific levels of corruption. In contrast to Billger & Goel (2009), we find that the level of democracy and the level of economic freedom are strongly significant determinants of corruption for all levels of corruption. Finally, and in contrast to the majority of related studies, the British colonial heritage does not turn out to be significant in any of our quantiles. Possibly, this is due to the extensive dataset we used, where corrupt countries that were former British colony not taken into account in previous research were included. However, here we do not aim to expand on the empirical results we obtained, leaving this for future research.
The paper is structured as follows. In the next section, we review the variables that are brought forward by both theory and empirical studies as determinants of corruption levels. Sections three and four explain in detail the data and the methodology we used. Section five displays and discusses our results; section six concludes.
2. Corruption and its determinants
Since the mid-1990s, a broad strand of literature aims at exploring the determinants of corruption both theoretically and empirically. Following Serra (2006), Lambsdorff (2006) and Dreher, Kotsogiannis, & McCorriston (2007), it is possible to classify the variables that explain corruption levels in four groups.
Group A: Political and economic institutions. This group contains variables explaining corruption that are related to the characteristics of the political regime of the countries, the effectivity of the justice system, and the level of economic freedom. The hypothesis is that corruption levels might be caused by weaknesses in these areas. Sandholtz & Koetzle, (2000) for instance, study the variation of perceived levels of corruption in a sample of 50 countries by proposing a set of variables related to the political and economic structure of the countries, democracy levels, and the degree of integration into the international economy. The authors find that corruption levels are higher with weak democratic institutions.
Group B: History. Theory suggests that the past strongly determine the institutional, political and cultural characteristics of a country today (North, 1990, p 93). The empirical literature on determinants of corruption has focussed on the influence of the colonial history as an indicator of historical components. Some studies such as La Porta (1999) and Treisman (2000) show that countries that have been a British colony tend to have lower levels of corruption, supposedly because of the British influence on democratic traditions and the characteristics of the judicial institutions.
Group C: Culture. This group contains social and cultural variables that could influence corruption levels. Most of studies have focused on the impact of religious beliefs as an important fundamental of social attitudes and family values that could explain the level of tolerance vis-à-vis corrupt practices. Most studies argue that religions that are more rigid in their structure tend to favour the conservation of the status quo and may thus be more tolerant with respect to corruption. Therefore, countries with a higher share of Protestants could be expected to exhibit lower levels of corruption. Various studies indeed find that the percentage of Protestants or the fact of having a majority of Protestants is in a good predictor of corruption levels (Sandholtz & Koetzle (2000), Treisman (2000), Pellegrini & Gerlagh (2007) and Picón (2012).
Group D: Economy. Finally, there are a series of economic variables that have been put forward in explaining levels of corruption. Studies typically consider the level of a country's integration into the international economy, the economic development of a country, as well as the size of government and its influence on the economy (Lambsdorff, 2007, p. 4). The empirical evidence suggests that the lower the income per capita level, the lower the integration of the country into the world economy and the bigger the size of its government, the higher the level of corruption tend to be (Serra (2006), Lambsdorff (2006), Picón (2011) and Picón (2012).
3. Data
For our study, we use the Corruption Perceptions Index (CPI) published by Transparency International for 2018 as our dependent variable. We multiply the CPI by -1 to obtain an index that permits a more intuitive interpretation of the results in which a higher score reflects a higher level of perceived corruption. The use of the CPI as a proxy for the level of corruption is justified due to the strong correlation of this index with other, comparable, indicators (Alesina & Weder (2002), Picón (2012).
Concerning the explanatory variables, we use the following data and classify them according to the groups presented in the previous section (see table 1).
Table 1 Data description and sources
VARIABLE | DESCRIPTION | SOURCE |
---|---|---|
Corruption | Corruption Perception Index 2018, multiplied with -1 to indicate that the index increases with increasing levels of perceived corruption (-100 least corrupt; 0 most corrupt). | Transparency International |
Economic Prosperity | Gross National Income per capita 2018 (PPP, prices 2011). Taken from the World Bank DataBank. For North Korea an estimate based on Maddison (2001) and published by the CIA were used. | World Bank and Central Intelligence Agency (CIA) |
Democracy | Sum of the 2018 indicators for Political Rights and Civil Liberties from "The Freedom in the World". Each country is scored from 2 to 14, where 2 represent the highest freedom and 14 the lowest freedom. The scores are multiplied with -1 to indicate that the index rises with increasing levels of "democracy" measured this way (-14 least democratic countries; -2 most democratic countries). | Freedom House |
Economic Freedom | Index of Economic Freedom, calculated as the average of the following indicators: Business Freedom, Labor Freedom, Monetary Freedom, Trade Freedom, Investment Freedom, and Financial Freedom, all from 2018. | Authors based on indicators by The Heritage Foundation and The Wall Street Journal |
Size of Government | Index of Government Expenditures 2018. | The Heritage Foundation and The Wall Street Journal |
Protestants | Proportion of persons with Protestant religion per country, according to the available data for 2018. | Central Intelligence Agency (CIA) and Religious Freedom Report by U.S. State Department |
British Colony | Dichotomous variable with value 1 if the country has been a British colony, 0 if not. | Central Intelligence Agency (CIA) and World Statesmen |
Source: Authors
Group A: Political and economic institutions. Just as Billger & Goel (2009), in the study we include as a measure of the level of democracy the sum of the indicators of Political Rights and Civil Liberties used by Freedom house's "Freedom in the World 2018" report. Each country is assigned a value between 2 and 14, where 2 represents the freest countries (most democratic) and 14 the least free (least democratic). Again, we multiply the values by -1 to obtain an index that increases with the level of "democracy" measured this way. Concerning economic freedom, (Billger & Goel, 2009), we use the Index of Economic Freedom published by The Heritage Foundation and The Wall Street Journal. However, the use of this index is problematic here considering that some of its indicators, such as the level of government expenditures, could entail problems of multicollinearity with the variable Size of Government, which is also used by the authors and is measured by dividing public spending by GDP. This decision may explain the ambiguous results obtained by the authors with respect to the role played by the size of government in explaining corruption levels. In our study, we try to be closer to the objective of the index and its methodology and use the average of its following indicators: Business Freedom, Labor Freedom, Monetary Freedom, Trade Freedom, Investment Freedom, and Financial Freedom, all of them for the year of 2018. By doing so, we avoid the problem mentioned before and can expand the list of countries used in our sample.
Group B: History. To capture the historical factor of colonial heritage, we use a di-chotomous variable taking the value of 1 if the country was a British colony and 0 if not. This variable was generated based on information available at the Central Intelligence Agency (The World Factbook) and complementary information published by the organization World Statesmen.
Group C: Culture. As a cultural variable, we take the proportion of Protestants in each country according to the available data for 2018. The variable was generated based on information by the Central Intelligence Agency (The World Factbook) and the report on Religious Freedom issued by the U.S. Department of State.
Group D: Economy. As an indicator for economic prosperity, we use the Gross National Income per capita 2018 (PPP, prices for 2011). The main source of information used was the Human Development Report data. For North Korea, we used as a proxy an extrapolation of the results from Maddison (2001) published by the CIA World Factbook as this country does not present official data on aggregated production or income. As an indicator for the size of government, in turn, we use the index for Government Spending 2018 published by The Heritage Foundation and The Wall Street Journal. This index is based on the level of public expenditures, including government consumption and transfers as percentage of GDP, and does not try to identify an optimal level of Government Spending as this may vary from country to country depending on culture, geography and development. According to the methodology, the scale of this index is non-lineal: countries that have expenditures close to zero are slightly penalized, while level of expenditures superior to 30% of GDP receive a score much worse as a the result of the quadratic function used. Therefore, only very big governments receive low scores.
To our knowledge, the database we use is, with information from 170 countries from 2018, the broadest and most recent database used to date in similar studies. For the first time, we include countries such as North Korea and some African countries, such as Lesotho, Gambia, Sudan, Sierra Leone and Swaziland that are usually not included due to lack of data. In our case, it was still impossible to include Somalia for that reason.
4. Methodology
In order to answer the research question, it is necessary to analyse the values of the different determinants for each level of corruption. However, the great majority of studies use econometric tools based on least square regression that do not allow for such type of analysis. Picón (2011) and Picón (2012) classify countries by quartiles according to their level of corruption using neural networks to identify which explanatory variables are doing best in classifying the countries. However, neural networks present limitations in terms of the characteristics of the coefficients produced by these classifications.
In this study, we therefore use a regression by quantiles5, allowing us to carefully analyze the determinants of corruption over the whole range of the levels of corruption, paying particular attention to the most and to the least corrupt countries.
According to Koenker & Bassett (1978), a general quantile regression model can be expressed as:
Where 0 < q < 1 designates the proportion of the population with scores below the quantile qth. Therefore, for each observation i, let εί be the residual:
Thus, a regression for a specific quantile qth (with q є (0,1)) is the solution of:
The algorithm to solve this problem is taken from Hunter & Lange (2000) and implemented in STATA statistical software by sqreg command, which estimates simultaneous-quantile regression and obtains an estimate of the variance covariance matrix via bootstrapping, including between-quantile blocks. Consequently, we can test and build confidence intervals associating coefficients describing different quantiles.
Table 2 Determinants of corruption OLS regression and regression by quantiles 2018
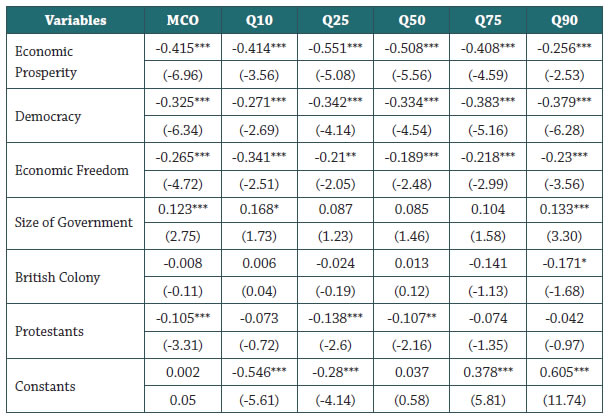
Notes: The dependent variable is the inverted Corruption Perceptions Index (Corruption in Table 1).
The model includes a sample of 170 countries with data from 2018.
The results from the quantile regression are based on 1000 bootstrap repetitions.
The lower quantiles (for example Q10) represent the countries with the lowest levels of perceived corruption. All the quantitative variables have been standardized with mean 0 and standard deviation 1.
The absolute values of the t statistics are given in parenthesis. ***, ** and * denote the levels of significance of the coefficients at 0.01, 0.05 and 0.1 respectively.
5. Results
First, we can observe that for the OLS estimation all proposed variables with exception of being a British colony are significant. Being a British colony also does not seem to be an important factor in the conditional distribution by quantiles. A possible explanation could be the size of our sample; by including countries with high levels of perceived corruption that used to be a colony of the British Empire but were excluded in former studies, the result stating a positive influence of British colonial history vanishes6.
Second, our results confirm the relevance of indicators related to economic prosperity such as income per capita levels. In this case, income per capita results turns out to be highly significant both on average and over the whole distribution in quantiles.
Third, in contrast to the results obtained by Billger & Goel (2009), we find that democracy and economic freedom are highly significant in when explaining levels of perceived corruption over the whole distribution and not only for countries with the highest levels of corruption. Countries with low levels of democracy and economic freedom tend to have higher levels of corruption over the whole sample. Again, the difference between the results from Billger & Goel (2009) and ours may be explained by the size of our sample.
Fourth, the variable Size of Government is significant on average but not over the whole distribution of quantiles of corruption levels. Indeed, the size of the government seems to be relevant in when explaining levels of corruption for the most corrupt countries and the least corrupt countries, but not for the countries with intermediate levels of corruption. This seems to indicate that increasing government spending is a risk in particular for the most corrupt countries, where such an increase would probably come along with a further increase in their level of corruption.
Finally, the proportion of Protestants is significant on average as indicated by the OLS regression. However, when looking at the quantile results, we can observe that this is not consistent over the whole distribution. The variable is only significant only for intermediate levels of corruption and the least corrupt countries. Before speculating on possible explanations for this result, in our view the most plausible one is that the result depends more upon the distribution of the countries as than on a real causal relationship between both variables.
6. Conclusions
We revisited an interesting research question put forward by Billger & Goel (2009) using a broader sample of countries and improving the specifications of some variables. We find that indeed there are differences in the relevant determinants of corruption between countries with different levels of corruption.
The size of the government and the proportion of Protestants in the population are only good predictors of corruption only for specific levels of corruption. The size of the government is relevant only for the most and the least corrupt countries, but not for the countries with intermediate levels of corruption. In addition, the proportion of Protestants significantly predicts levels of corruption only for intermediate levels of corruption and the least corrupt countries.
Our results differ from those published by Billger & Goel (2009) with respect to the importance of democracy and economic freedom. The authors found that the level of democracy predicts corruption only for the most corrupt countries, while the level of economic freedom is relevant in explaining corruption only for the least corrupt countries. Our results show that these two variables are highly significant for all levels of corruption.
Finally, in contrast to most other contributions to this strand of literature, in particular the extreme bounds analysis by Serra (2006), we do not find the British colonial legacy to be significant for any level of corruption. We suggest that our finding can be explained by the broad sample; we were able to include countries with high levels of corruption and British colonial history that were excluded in previous studies.
Despite the number of contributions on determinants of corruption, we think that there is still room for exploring in more depth the causes of corruption by exploiting differences in corruption levels and by using more countries and more recent datasets. The empiric specification of the variables suggested by theory can also be improved as new indexes emerge. Our findings here in particular suggest that there is room for increasing our understanding about the historical characteristics of the countries, as the colonial heritage alone seems to be a poor proxy, at best.