The global burden of type 2 diabetes (T2D) is increasing, partially facilitated by a sharp increase in the disease in low and middle income countries (LMICs)1,2. LMICs not only show a high prevalence of T2D (8.7%), but have shown a much faster increase in this prevalence over the past 30 years when compared to high-income countries (HICs)1. Conventional risk factors for T2D in HICs, such as high body mass index (BMI)3, low levels of physical activity4, and poor dietary behaviours5, do not fully account for the greater increase in prevalence of T2D in LMICs2. Therefore, risk factors for T2D specifically within an LMIC context need to be determined.
Observational epidemiological analyses are currently being used to explain the rising prevalence and determine outstanding risk factors for T2D in LMICs, but even if designed well, such studies are prone to confounding, reverse causation and multiple sources of bias (e.g. selection, reporting and measurement)6. As such, these study designs can potentially generate unreliable estimates of causality between a risk factor and disease. A successful, more robust approach to overcome these limitations and improve causal inference is Mendelian randomization (MR)6,7.
Briefly, arising from instrumentable variable (IV) analyses in econometrics, MR exploits Mendel’s first and second laws of inheritance (i.e. the independent assortment and segregation of alleles that at leads to the random distribution of genotypes in the population) enabling the use of genetic variants to proxy for a clinically relevant (and usually modifiable) trait (e.g. BMI)6,7. Provided a number of key assumptions are met (Figure 1), these genetic IVs can then be used to derive the causal effect on a trait on disease or adverse health outcome, conferring multiple advantages over observationally-derived estimates of association.
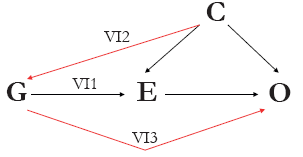
Figure 1 Directed acyclic graph (DAG) of the theory and key assumptions of Mendelian randomization. A genetic variant (or variants, G) can be used as instrumental variables for an exposure of interest (E) to assess the causal association between E and the outcome of interest (O) given that the following three assumptions hold: (IV1) G must be robustly associated with E; (IV2) G must not be associated with any measured or unmeasured confounding variable (C); and (IV3) there must be no independent association between G and O, given E and C.
Analogous to arms of a randomized control trial (RCT), genetic variants used in MR are largely independent of confounding factors due to the random nature of their allocation within a population. They are also not modified by the later development of disease or health outcome (Figure 2) and, with the advent of more accurate genotyping arrays, measurement error is largely reduced. Therefore, at a population level, the portion of variance in the modifiable trait explained by genetic variants used as an IV (unlike the direct measurement of the trait) is free of the limitations that would otherwise weaken causal inference in observational studies. MR provides a robust, unconfounded estimate of the casual association between a trait and disease6,7.
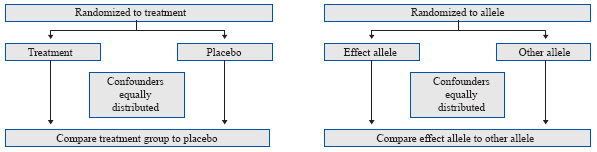
Figure 2 Mendelian randomization methodology compared to randomized controlled trials (RCTs). In an RCT, individuals are randomized to either the treatment or control (placebo) arms, which theoretically ensures that confounders are equally distributed among trial arms. Analogous to this, the random allocation and independent assortment of alleles at conception and meiosis, respectively ensure that confounders are equally distributed among genotype groups. Differences in individuals between genotype groups will differ only due to the genotype group; hence, we can use genetic variants as instrumental variables for exposures of interest in Mendelian randomization analyses.
In the context of HICs, MR has confirmed and identified endogenous risk factors for T2D including obesity, systemic inflammation and blood lipids, and exogenous risk factors including alcohol and dairy consumption8,9. For example, the use of MR in a recent comprehensive assessment of the causal association between BMI and T2D produced refined causal estimates suggesting that T2D risk increased by approximately 30% with each unit (kg/m2) in BMI (odds ratio (OR): 1.26; 95% confidence interval (CI): 1.19, 1.32; P=3.29x10-10)10. However, these findings cannot reliably be translated to LMICs. Until recently, the expense and availability of large samples of genetic and phenotypic data restricted MR analyses to HICs; a majority of which reside within populations of European ancestry. Therefore, whilst it is likely that higher adiposity may, at least in part, explain the growing prevalence of diabetes in LMICs, the estimate of association remains untested and currently only a few MR studies exist that assess the impact of any modifiable exposure on disease risk specifically in LMICs.
Specifically within the context of Latin America, Borges et al.11 investigated the causal effect of circulating homocysteine levels on blood pressure in the 1982 Pelotas Birth Cohort in Brazil using MR, then compared these results to those from a cohort of European individuals. For the European analysis, the authors used summary data from a recent meta-analysis of homocysteine genome-wide association studies (GWAS)12, (>44,000 European individuals) to generate an IV for circulating levels of homocysteine, of which they used to test the causal effect of homocysteine on blood pressure (BP) using summary data from the International Blood Pressure Consortium (IBCP) (>69,000 European individuals)13, in a two-sample MR approach. MR results showed that systolic blood pressure (SBP) decreased by 1.8mmHg (95% CI: -3.9, 0.4; P=0.11) in the Pelotas cohort but increased by 0.6mmHg (95% CI: -0.8, 1.9; P = 0.41) in the European population with each standard deviation (SD) increase in circulating log(homocysteine) levels. Similarly, diastolic blood pressure (DBP) increased by 0.1mmHg (95% CI: -1.5, 1.7; P=0.93) in the Pelotas cohort but increased by 1.1mmHg (95% CI: 0.2, 1.9; P=0.01) in the European population.
In another MR analysis using the same 1982 Pelotas Birth Cohort, Hartwig et al.14 found that lactase persistence (i.e. milk consumption) in adults was positively associated with BMI (effect estimate per 1dL/day increase in milk intake: 0.17kg/m2; 95% CI: 0.07, 0.27; P=0.001) and risk of obesity (OR per 1dL/day increase in milk intake: 1.09; 95% CI: 1.02, 1.17; P=0.015), contrary to observational estimates in the same cohort. Milk intake in Brazil (and potentially other similar Latin American countries) may predispose individuals to having a higher level of adiposity, a result that differs from inconsistent observational estimates derived from European populations.
These two examples alone underscore both the need for MR analyses to be conducted in LMICs, and the issues faced when dealing with genetic data. Firstly, large sample sizes are required for adequate statistical power in MR analyses due to the usually relatively small portion of variance explained in the risk factor by the genetic IV. The former described example, assessing the causal effect of circulating levels of homocysteine on BP, emphasises this particular point. Furthermore, a recent GWAS in over 340,000 individuals found that 66 genetic variants associated with BP in Europeans were also predictive of BP in 64,000 non-European samples; however, comparably large non-European sample sizes were necessitated to show this concordance between the direction of effect estimates and elucidate which genetic variants were associated with a specific ancestry group15. Therefore, the noticeable different sample size between the 1982 Pelotas Birth Cohort (N=3,701) and IBPC (N>69,000) in the aforementioned study, may partly explain the opposing direction of effect generated between the two populations. The relative cost of genotyping arrays and processing presents a risk of limited statistical power due to smaller sample sizes in MR analyses, particularly in LMICs, where appropriately sized samples may be relatively sparse.
Secondly, LMICs typically possess a multi-ethnic population structure (as seen in the Pelotas cohort in examples described above), showing a high level of genetic admixture and heterogeneity. The effect of risk factors on diseases that would typically affect one ancestral population (like Europeans, for example) might be irrelevant for an LMIC population as a result of this. In a recent study by Zanetti et al.16, global effect estimates for the association between SNPs and several common diseases, such as T2D, were typically in the same direction between different ancestral populations. However, the varying linkage disequilibrium structure (i.e. non-random association of genetic variants) between different ancestries largely influences the magnitude of effect between these populations. Ultimately, the difference in magnitude of the point estimates and likely heterogeneous population in Latin American countries underscores the extent to which MR analyses conducted in European populations may not represent the effects of the same exposures on outcomes in LMIC populations.
Whilst no studies have assessed the causal relevance of any exposure on T2D in LMICs using MR methodology, the described MR examples not only highlight their feasibility in LMICs, but also suggest that such studies will provide effect estimates more pertinent to LMICs. As more MR studies are published in LMICs, they could potentially refine population-specific IVs that take into account ancestral background, effect estimates and causal risk factors for relevant diseases such as T2D.
In order for future MR analyses in LMICs to be efficacious, there are certain properties, principals and limitations that should be taken into account, which have been outlined and discussed in detail previously6. Particular to LMIC settings, admixture and population heterogeneity due to multi-ethnic background can introduce genetic confounding and produce biased results. This can be addressed using methods of ancestral genomic control implemented in GWAS. Furthermore, selection bias and generalisability of findings (especially in populations where oversampling in low-socioeconomic status groups is likely) are of particular importance in practice. Finally, such studies will also rely on the availability of genetic data, high computational power and appropriate infrastructural facilities to store, maintain and analyse data required for MR analyses.
In the past decade or so, MR methodology has been increasingly applied to improve causal inference in a range of epidemiological contexts. Recent methodological developments building on the basic concept of MR, such as two-sample, two-step, network and multi-phenotype MR have made such complex analyses easily accessible for the research community, and provided more tools to dissect causal networks between traits with greater statistical power (Table 1)6,7.
Table 1 Methodological concepts, recent developments, strengths and limitations of Mendelian randomization analyses.
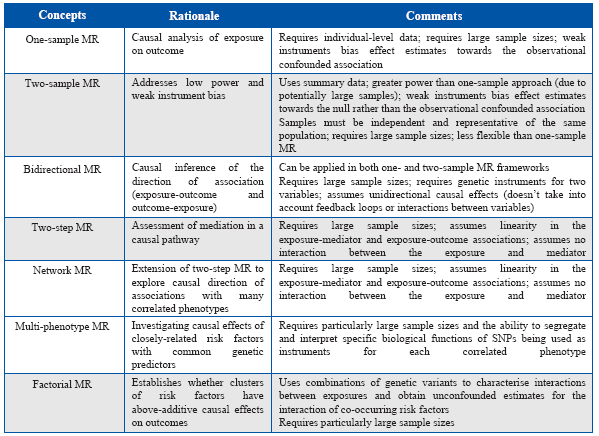
Given the high prevalence of T2D in Latin America and LMICs, it is important to generate greater understanding of the potentially modifiable risk factors of T2D (along with other high-prevalence diseases and adverse health outcomes). With economic growth, availability of human tissue, increasing cost-effectiveness of genotyping arrays and recent developments in MR methodology, MR analyses are becoming ever-more practicable and could prove to be of fundamental importance when attempting to find outstanding risk factors for T2D that are particularly pertinent in LMICs.