Introduction
According to the World Health Organization, stroke is the second leading cause of death worldwide and the third most common in low-income countries, causing about 4.4 million deaths annually [1]. Although the most recent epidemiological studies show declining age-standardized mortality rates worldwide, the absolute number of people suffering a stroke and living with disability is increasing, creating emotional and socioeconomic consequences for individuals, their families, and health services [2,3]
Between 50% and 70% of stroke survivors regain functional independence. However, about 30% remain with physical, sensory, and intellectual impairments that result in disability, and approximately 20% require institutional care for functional dependence after hospital discharge following an acute episode [4].
The most common and widely recognized impairment caused by stroke is motor impairment [5]. Hemiparesis triggers gait disturbances mainly related to reduced propulsion and kinematic or spatiotemporal asymmetry, which increase energy expenditure, reduce speed and endurance during walking and increase the risk of falling in this population [6]. This results in increased functional dependence and the need for assistance in more than one third of activities of daily living, including self-care and social interaction [2,7].
Gait recovery is considered one of the main goals in rehabilitation [8]. From 65% to 95% of people with stroke learn to walk independently approximately six months after injury; compared to other individuals, they spend more time training gait than other activities and only 30-50% are able to ambulate in the community [9]. This has generated a considerable increase in rehabilitation research towards the development of new strategies to promote and enhance gait recovery after stroke [10].
Of these strategies, evidence in neurorehabilitation supports specific training with large doses and high intensities of practice as the main elements for improving mobility and gait speed after stroke [11], supporting findings in neuroscience that promote the incorporation of repetitive and variable practice for the improvement of neuroplasticity and motor learning processes post-stroke [12]. Thus, responding to the premise that training improves performance in terms of acquisition of new skills and refinement of previously learned skills after brain injury [13]. This means that for the central nervous system to act efficiently, rehabilitation programs must be guided by dependent plasticity processes such as task-oriented practice, focused attention, repetitive training, and feedback [14].
In recent years, feedback has been shown to be one of the variables that most impact gait recovery [15]. Extrinsic feedback systems determine task performance through the quantification of biomedical variables and inform the user directly or indirectly about a relevant variable such as force, myoelectric activity, temperature or joint torques, transforming this information into auditory, visual or haptic signals easily identifiable by the user [16,17]. Feedback is considered a key element for rehabilitation, given its ease of acquisition, manipulation and quantification in experimental settings [18].
Feedback provides information about performance and outcomes in the execution of a task, with the intention of reducing errors in execution and facilitating the achievement of goals in recovery [19]. Its application in stroke rehabilitation is mainly oriented to provide or represent proprioceptive sensory information that may be affected after the injury [6]. This type of feedback is used in gait rehabilitation, especially to improve step length and width, stance time, posture control, and weight transfer between limbs or on the trunk, which are mandatory steps for the improvement of such walking function [20].
The strategy used in this study is based on a dynamic robotic orthosis system that combines a weight-bearing method for the control of lower limb motion in the sagittal plane during gait training (Lokomat®) [21]. This system presents online extrinsic feedback during patient training, which allows evaluation of performance during gait. The information presented to the participant shows the interaction of the hip and knee joint torques with the robotic orthoses represented in a line graph visible on a monitor. The purpose of this information is to provide extrinsic feedback as a guide to performance at different phases in the gait cycle [10].
This study is designed to determine the effect of visual feedback on gait speed during robotic gait training, varying only the condition of provision and non-provision of such information during training, by applying a single-case design. In this type of study, each participant becomes his or her own control, reducing interference from confounding variables such as gender, age, socioeconomic status, and concurrent intervention [22].
Methodology
Study design
A single-case, randomized, nonconcurrent, multiple baseline study was conducted in two phases: baseline or control phase (A) and intervention phase (B). Subjects were randomized to receive 12 to 20 sessions with the control treatment (robotic gait training without feedback) before starting the intervention with robotic training with visual feedback. Randomization was conducted via the sealed envelope method.
Participants
Four persons with a history of stroke of no more than six months of evolution (three women, one man; mean age: 50 years; range 19 to 73 years) were recruited while participating in the interdisciplinary rehabilitation process PIR® of the Universidad de la Sabana Clinic in Chía, municipality of Cundinamarca, Colombia.
The inclusion criteria were: 1) to walk independently with or without the use of a support product for at least 10 meters, 2) to present a score of more than 20 points in the evaluation of the mini mental test.
Exclusion criteria: 1) presenting severe visual or hearing impairment, 2) presenting severe cardiovascular disease, 3) weighing more than 260 pounds, 4) presenting recent history of traumatic injury to the trunk or lower limbs, and 5) presenting lower limb discrepancy greater than 3 centimeters.
The study was endorsed by the Bioethics Committee of the Universidad Autónoma de Manizales (record 080 of 2018). Each of the participants accepted the intervention by signing the informed consent.
Table 1 presents the sociodemographic characteristics of the four participants, including educational level and cognitive status.
Materials and methods
Gait speed was assessed in all participants by applying the 10-meter walking test immediately after the end of each training session during the baseline (A) and intervention (B) phases. The 10-meter gait test has excellent test-retest reliability (ICC=0.95-0.99) and inter- and intra-rater reliability (ICC= 0.87- 0.88) for post-ACV persons in chronic stages [23]. In addition, it has excellent correlation with instruments for assessing independence in activities of daily living (r=0.76) [24].
Intervention
In the control and intervention phases, participants performed robotic gait training Lokomat 3 times per week for one hour, with weight support starting at 40% of body weight, progressing between 5 to 10% at each session, until 100% support tolerance was reached.
Gait speed was initially set at 1.5 km/hour with progressive increases of 0.2 km/hour at each session until a maximum speed of 3 km/hour was reached. The guiding force of the device was programmed at 100% at the beginning of the training with progressive reduction, until reaching 0% according to the participant's tolerance.
For the intervention phase, all participants also received visual feedback, through the observation of a monitor that presented information on the hip and knee joint torques required to maintain a predefined trajectory in each gait phase. By definition, a torque is positive when the participant's movement is corrected toward extension and negative when the movement is corrected toward flexion. Torques are the result of multiplying the participant's joint motion by the weight load on each joint, resulting in an arbitrary unit of measurement that is defined as the biofeedback unit [25]. This information is presented in a line graph that visualizes the participant and reports performance during the stance and swing phase (Figure 1). Training was performed by therapists certified in the handling of the robotic device, external to the research.
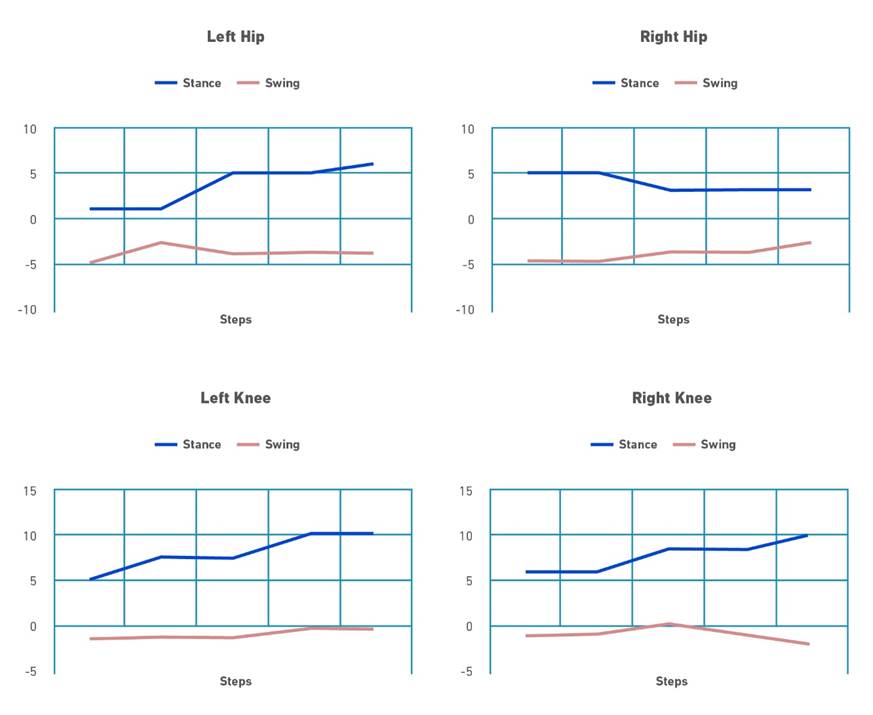
Note. In this Figure a positive feedback is provided for all joints, especially for the knees, during stance phase indicating that the patient actively moves joints according to the reference trajectories, while during the swing phase, particularly for the hips, patient dos not contribute to the walking movement than the robot has to exert torque in order to maintain the desired reference trajectory. Adapted from Tamburella F, Moreno JC, Sofía D, Valenzuela H, Pisotta I, Iosa M, et al. Influences of the biofeedback content on robotic post-stroke gait rehabilitation: electromyographic vs joint torque biofeedback. 2019;0:1-17.
Figure 1. Simulation of the standard display of the monitor implemented in the Lokomat( system for visual feedback delivery.
Data analysis
Data were analyzed according to the recommendations of the What Works Clearinghouse technical documentation for single-case designs and the publication guide for single-case designs - SCRIBE [26,27], using the web resource for visual analysis of single-case designs: https://manolov.shinyapps.io/Overlap [28]. Each of the phases was examined and compared for differences in level, trend of change, stability, immediate effect, and nonoverlap of data for each participant.
The median value of the data was used to determine the changes in level at each phase; the direction of the trend was calculated through the Theil- Sen estimator, also known as Sen's slope estimator or Kendall's fitting method [29].
Data stability was determined by calculating the percentage of data falling above or below 20% of the median data value, through the two standard deviations band method; a stability criterion is satisfied if 80% of the data fall in this range [30].
Finally, the nonoverlap of the data (PND) was estimated through the nonoverlap index. This index quantifies the proportion of data in the intervention phase (B) that do not overlap in the baseline phase (A). Non-overlap statistics are typically scaled as a percentage from 0 to 100. Thus, a percentage less than 50 denotes no effect of the intervention, a percentage between 50 and 70 demonstrates a questionable effect, and more than 70% suggests that the intervention was effective [31].
Results
Visual analysis
Figure 2-5 shows the graph of each participant's data used for visual analysis during the baseline phase (A) and the intervention phase (B), separated by the continuous vertical line. The x-axis represents the gait speed value and the y-axis the number of sessions for each of the phases.
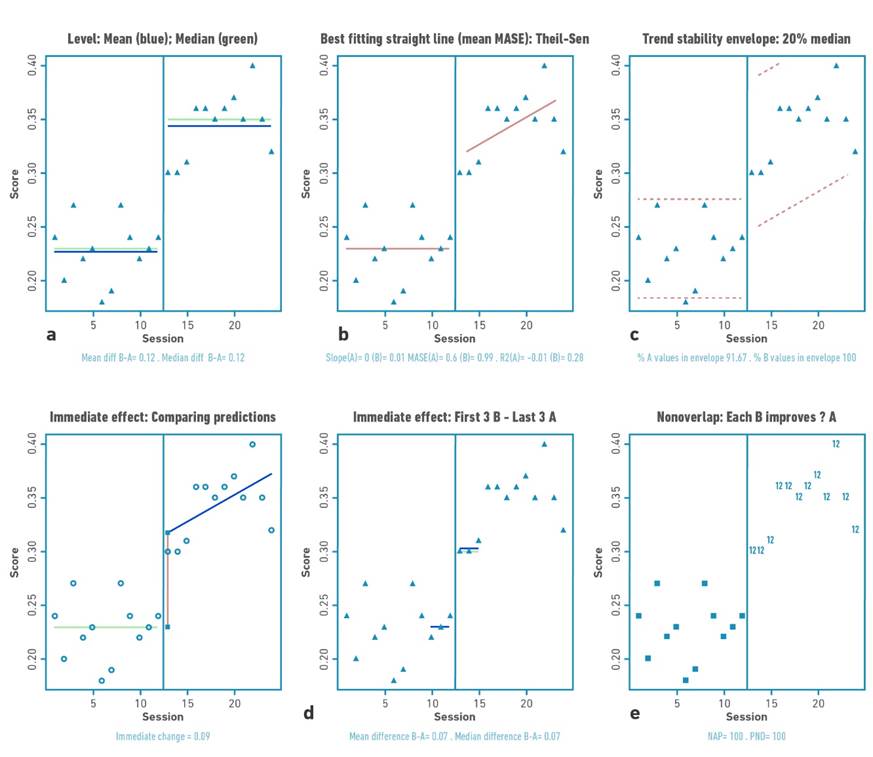
Figure 2 Visual analysis for participant 1 demonstrating: a) level, b) trend, c) data stability, d) immediate effect and e) nonoverlap (PND).
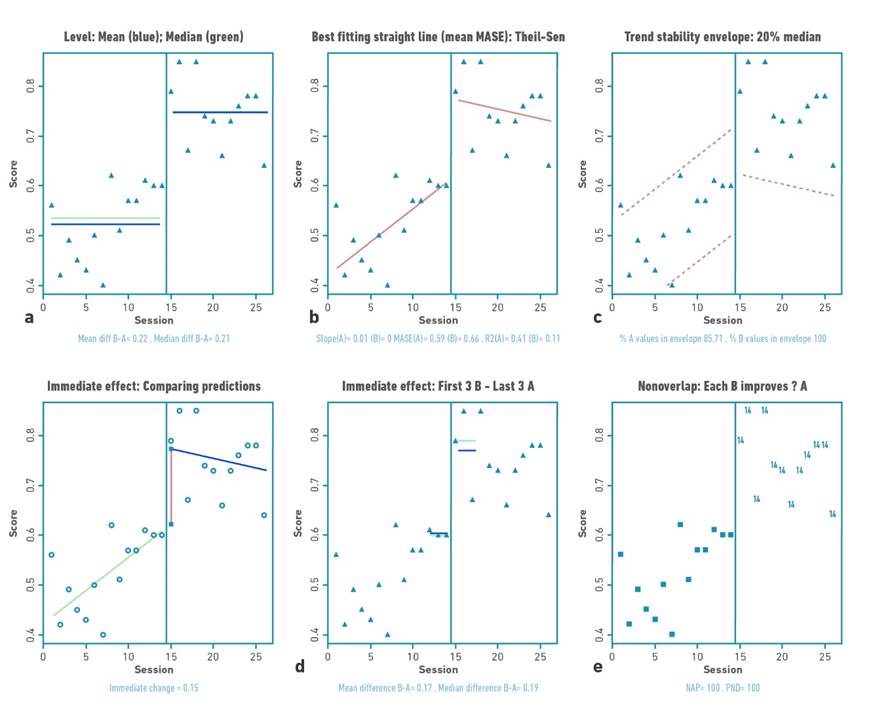
Figure 3 Visual analysis for participant 2 demonstrating: a) level, b) trend, c) data stability, d) immediate effect and e) nonoverlap (PND).
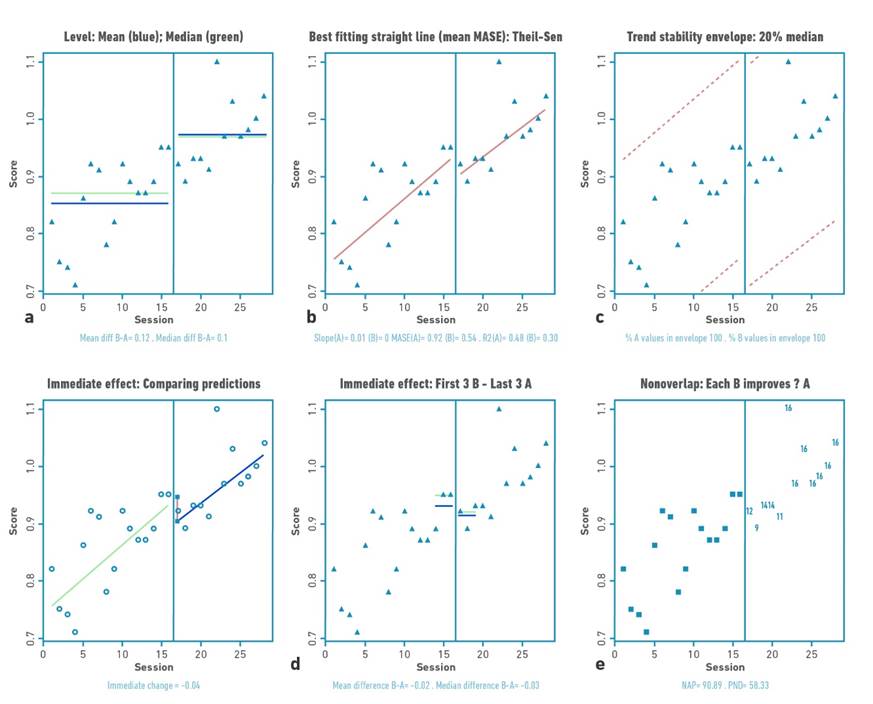
Figure 4 Visual analysis for participant 3 demonstrating: a) level, b) trend, c) data stability, d) immediate effect and e) nonoverlap (PND).
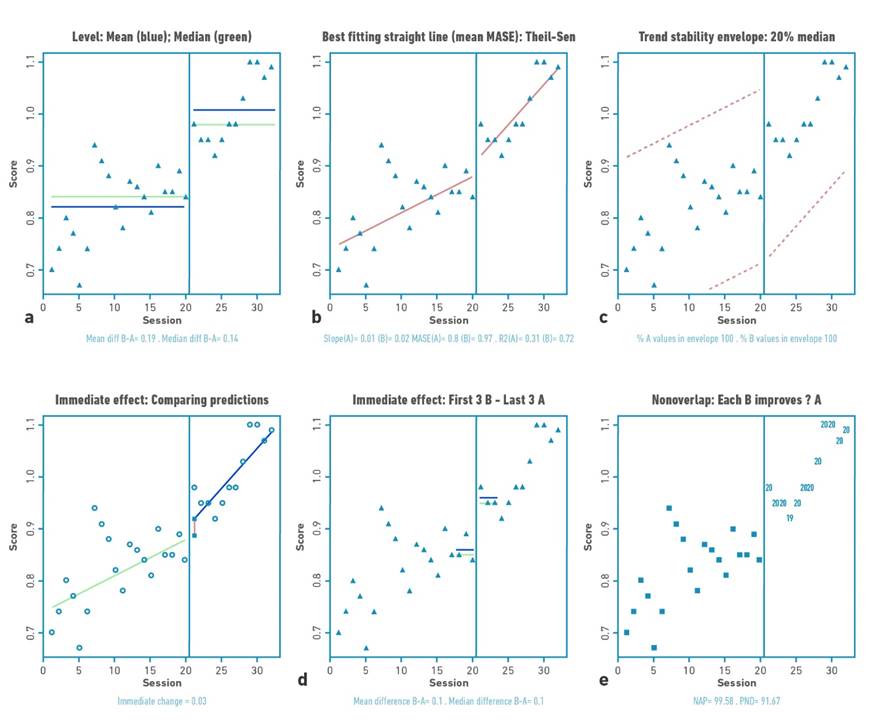
Figure 5 Visual analysis for participant 4 demonstrating: a) level, b) trend, c) data stability, d) immediate effect and e) nonoverlap (PND).
Changes in the level
Table 2 shows the changes in the level (median) and standard deviation of the data for the baseline and intervention phase for each participant. Positive changes in the level of the data were found for all participants. Gait velocity values were higher in all participants in the intervention phase compared to the baseline phase (mean:0.76 m/s); with difference in the median of the intervention and baseline phase between 0.1 and 0.21 m/s. The highest changes occurred in participants 1 and 2.
Changes in data trend
The trend of the data shows increases or decrease of the study variable in the visual analysis. For the present investigation, the trend of the data toward ascent shows improvement. Visual analysis of the trend demonstrates acceleration for the trend (increase in gait speed values over time) of the intervention line for participants P01, P03, and P04.
Statistical analysis
Data stability: two-standard deviation banding method
Each of the baseline (A) and intervention (B) for each participant met the stability criterion according to the two standard deviation banding method [32]. For all participants, more than 80% of the data remained within the established range, indicating stability of the data in both the baseline and intervention phases.
Immediate effect: patterns of change
Two patterns of change in response to the intervention were identified. The first pattern was an immediate change at the start of training (mean: 0.15 m/s), followed by further slight improvement throughout the intervention phase, but with decreasing data trend in this phase (no within-training effect), applicable for participant 2.
The second pattern was an immediate change, followed by a gradual and continuous improvement during the intervention phase (continuous effect during training), applicable for participants 1, 3 and 4. Participant 1 demonstrated a high immediate change pattern (mean: 0.09 m/s) with continuous effect during the intervention, while participants 3 and 4 were characterized by a more discrete immediate change pattern (mean: 0.04 and 0.03 m/s) with continuous effect during the intervention.
Percentage of non-overlapping data
For this study, an increase in outcome values would locate the points in the visual analysis higher in the intervention phase versus the baseline phase. The percentage of no overlap demonstrated intervention effect for participants 1, 2, and 4 (>91.67%) and questionable effect for participant 3 (58.3%).
Discussion
From neuroscience research, there are several training variables that determine the motor learning process. Most of them are related to the type of practice, repetition, taskoriented training, specificity and fundamentally feedback, the latter being one of the most critical variables for rehabilitation training [25]. Based on this concept, the present study aimed to determine the effect of visual feedback on gait speed during robotic training, by varying only the condition of supply and non-delivery of such information, applying a single-case design. The hypothesis stated in this study formulated that robotic training with visual feedback improved gait performance in temporal terms, specifically gait speed.
Consistent with the principles of analysis of single-case designs, the experimental criterion was moderated by demonstrating increases in gait speed in the first five days after the introduction of training for cases 1, 2, and 4, this associated with the pattern of change in the data reported for each case. The positive changes in gait speed are mainly related to the recognition of hip and knee joint movement, guided by the dynamic orthoses of the Lokomat®, system, which allows maintaining an adequate stride length, greater freedom in swing, stability in support and maintaining the direction of progression [14].
Pak and Lee [33] described similar effects in a study of visual feedback during squat training with gradual change in weight bearing on the paretic limb in adults with a history of stroke. The results demonstrate improvement in muscle activity, balance, and gait, indicating that visual markers have a primary effect on movement control and learning, which specifically improves weight transfer for balance control and stability of the affected limb [33].
For their part, Genthe et al [34] demonstrated changes in paretic leg propulsion after real-time visual feedback gait training in nine post-ACV subjects. The feedback training generated significant increase in the step length of the paretic limb, indicating that during gait the paretic limb was positioned further back during the terminal stance phase, which in turn modified patterns in muscle performance of the paretic limb and reduced offsets in the healthy limb [34]. During gait training, the use of real-time visual cues has shown favorable results in terms of weight distribution and symmetry in stance, which contributes positively to improved speed and balance during gait.
Kim and Oh [35] combined treadmill gait training with the use of real-time visual feedback in adults with hemiparesis secondary to stroke, demonstrating increases in stride length, stride length, and stance time. In addition, they reported improvement in balance assessment using the Timed get up and Go test. These findings suggest that task-oriented training with visual feedback favors not only the execution of the task itself, but also the transfer of learning into functional activities that share spatiotemporal characteristics during execution [35].
Based on these findings, the present study confirms the relevance of the application and use of motor learning principles for the rehabilitation of people with neurological deficits. In general, the reported neuroplastic changes in the central nervous system after injury are activity-dependent and are strongly associated with the application of motor learning principles in the rehabilitative setting.
Conclusions
The use of visual feedback during gait training has been widely investigated. However, some studies that present significant findings have some limitations: most of them compare intervention groups with conventional training and consider within the intervention additional strategies that have been proven to enhance learning, such as massive and repetitive practice and treadmill weight-bearing systems. This generates difficulties in determining the real effect of visual feedback during training [18].
On the other hand, the nature, amount and frequency of extrinsic feedback play an important role in its effectiveness. However, it has not been possible to determine conclusively which are the most appropriate and essential characteristics and types of feedback to favor the learning process [36].
The results presented in this study evidenced a positive effect of robotic gait training with visual feedback on gait speed in all participants in this research. Although the patterns of change and the immediate effect was not the same in all participants, it does highlight an improvement in the level of data during the intervention, demonstrating the importance of visual feedback as a performance coach in rehabilitative training for gait training. However, these should be viewed with caution, due to limitations derived from the non-replication of phases within the study and the study design itself, which has limitations compared to controlled clinical trials, such as sample size and the use of more extensive statistical analysis.
In relation to the results described, the lack of follow-up limits the possibility of describing possible long-term effects derived from the intervention presented. Likewise, all participants wandered independently prior to participation in the research, which makes it impossible to generalize the results to another type of population that does not share this characteristic.
On the other hand, the design used in this research is a design that in recent years has begun to be used and recognized in the rehabilitation sciences, so in most cases its reliability and validity is unknown. Therefore, the reader is recommended to review it in depth in order to understand how this type of design allows the generation of valid conclusions applicable to clinical practice.